1. Real-time Anomaly Detection
Advanced machine learning models can continuously analyze sensor data (e.g., temperature, humidity, shock, vibration) to spot deviations from expected patterns, allowing for immediate corrective actions.
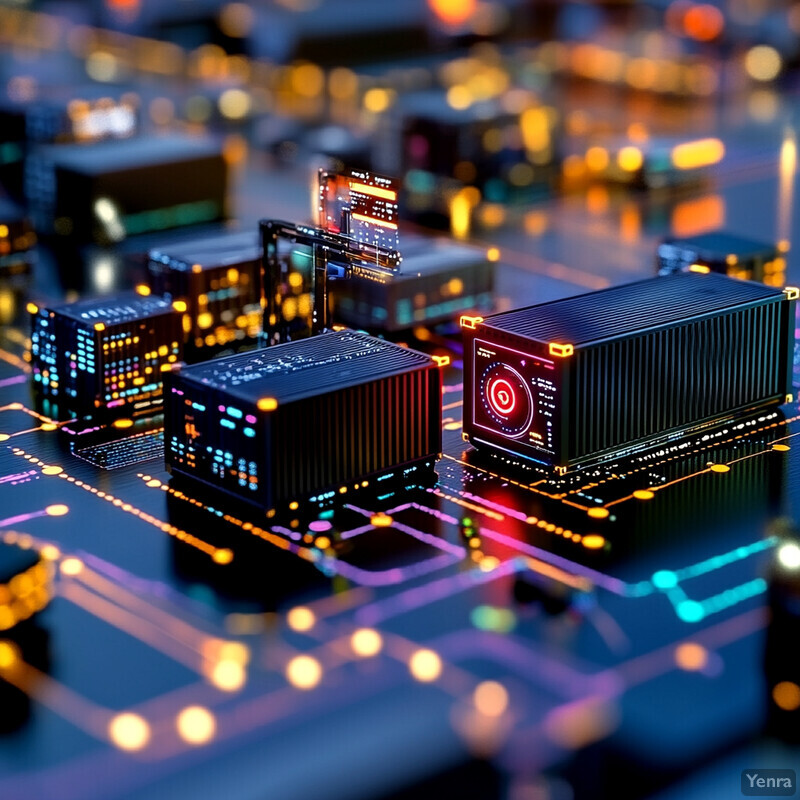
AI-driven real-time anomaly detection involves the use of advanced machine learning algorithms that continuously ingest and analyze streams of sensor data—such as temperature, vibration, humidity, and pressure readings—from various points along the supply chain. These systems learn the expected operational ranges and patterns for different types of cargo under normal conditions. When a sensor reports data that falls outside these learned norms, the AI flags the event, allowing stakeholders to intervene promptly. For example, if a perishable goods shipment’s temperature unexpectedly spikes, the AI alert can prompt immediate actions such as adjusting the refrigeration settings, rerouting the shipment, or inspecting the container for mechanical issues. This proactive response capability helps prevent small irregularities from escalating into full-scale damage, thereby safeguarding the cargo’s integrity and value.
2. Predictive Spoilage Analytics
By learning from historical data and environmental conditions, AI can predict when perishables are likely to spoil or degrade, enabling proactive adjustments to logistics and storage conditions.
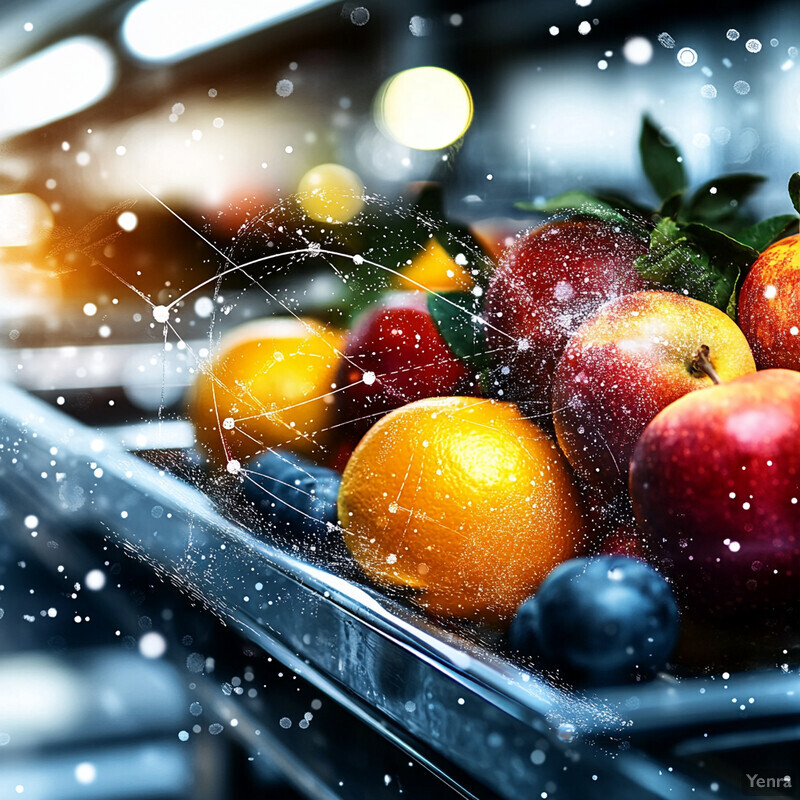
Through complex predictive modeling, AI can estimate the remaining shelf life and freshness of perishable cargo long before it visibly deteriorates. By integrating historical data on perishables—such as fruits, vegetables, dairy, or pharmaceuticals—with current and forecasted conditions (temperature, humidity, handling methods, and transit times), AI systems calculate the likelihood and timing of spoilage. This predictive insight empowers logistics planners to make informed decisions about when to accelerate deliveries, adjust storage conditions, or redistribute items that may degrade sooner. In turn, this leads to minimized waste, better quality control, and improved customer satisfaction, as products arrive in optimal condition.
3. Intelligent Sensor Fusion
AI algorithms can integrate and interpret data from multiple sensors—such as temperature, CO₂ levels, humidity, and GPS trackers—providing a comprehensive understanding of cargo integrity.
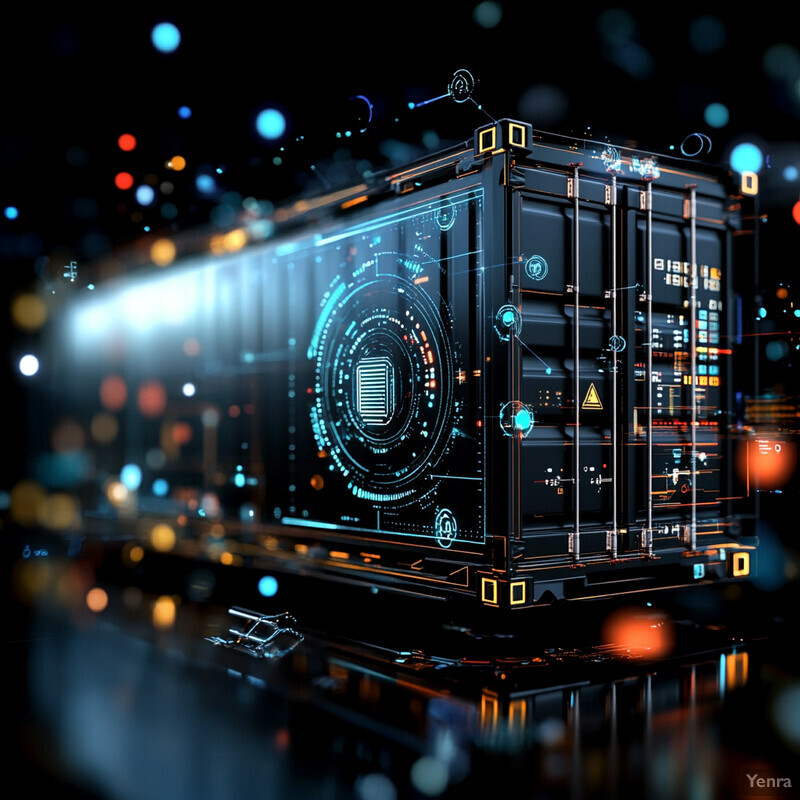
Intelligent sensor fusion involves the use of AI to combine and interpret data from a wide variety of sensors, including environmental monitors, GPS units, vibration detectors, and chemical analyzers. Rather than relying on a single data stream, the AI system contextualizes readings against one another to provide a more holistic picture of cargo conditions. This approach reduces the likelihood of misinterpretation that could arise from isolated sensor signals. For example, a slightly elevated temperature reading might be less alarming if accompanied by stable humidity and airflow metrics. By unifying data sources, AI-driven sensor fusion produces richer insights that inform more accurate decision-making, ensure cargo integrity, and lower the incidence of false alarms.
4. Computer Vision Damage Detection
Advanced image recognition technologies can identify physical damage or tampering of containers and pallets, reducing the need for manual inspections.
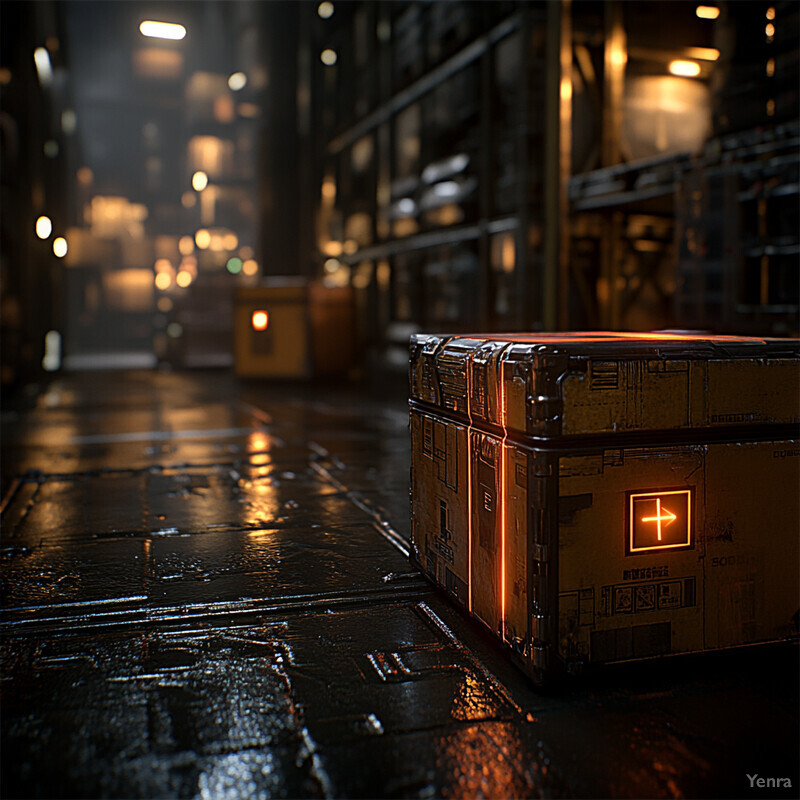
Using sophisticated image and video analytics, computer vision—backed by AI—can automatically detect signs of physical damage, tampering, or unauthorized openings in cargo containers and packages. By comparing real-time camera feeds or photographs against reference images of undamaged cargo, these algorithms can spot dents, tears in packaging, compromised seals, or missing items. This is especially useful when human inspectors are limited or when round-the-clock surveillance is required. The ability to catch damage early reduces the likelihood that compromised shipments will move further downstream, thereby preventing losses, claims, and dissatisfied customers. Additionally, computer vision can continually improve as it processes more imagery, refining its accuracy over time.
5. Automated Quality Assessments
Through continuous monitoring, AI systems can classify cargo conditions (e.g., fresh, borderline, spoiled) in real-time, helping handlers maintain quality standards and comply with regulations.
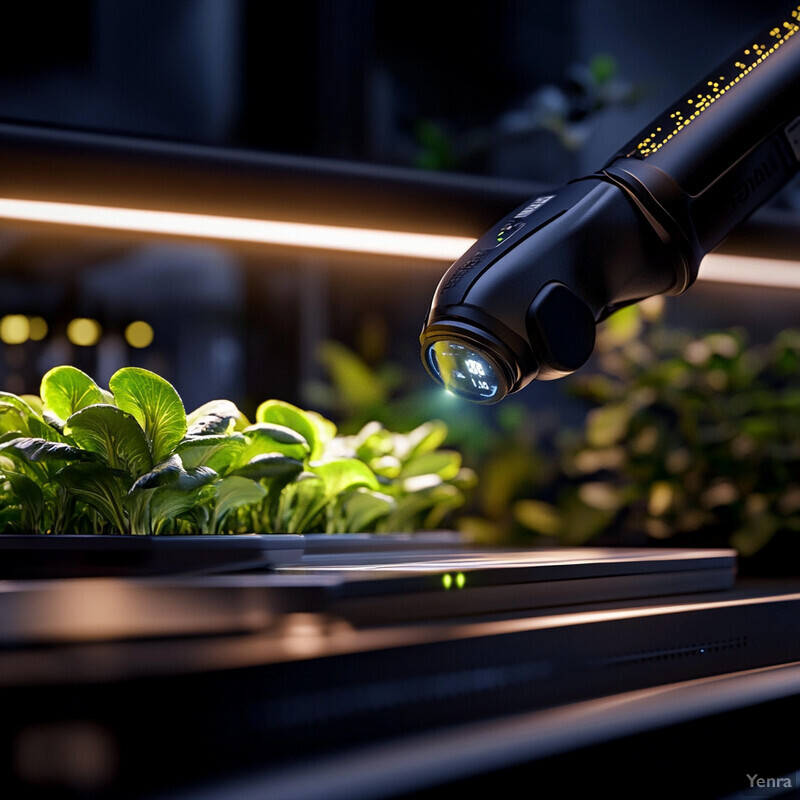
AI systems can classify and evaluate the quality of cargo automatically by analyzing sensor inputs and visual cues. For products with strict quality standards—like pharmaceuticals that must remain within certain temperature ranges, or produce that should not bruise—AI can continuously score shipments on a quality index. If quality dips below a predefined threshold, handlers receive alerts, allowing them to adjust conditions, remove compromised items, or expedite deliveries. Such automated quality assessments ensure that logistics providers maintain compliance with quality mandates and streamline their operational workflows, ultimately protecting brand reputation and enhancing consumer trust in product integrity.
6. Adaptive Threshold Setting
Instead of relying on static temperature or humidity limits, AI models can dynamically adjust thresholds based on current environmental conditions and cargo types, improving responsiveness to potential issues.
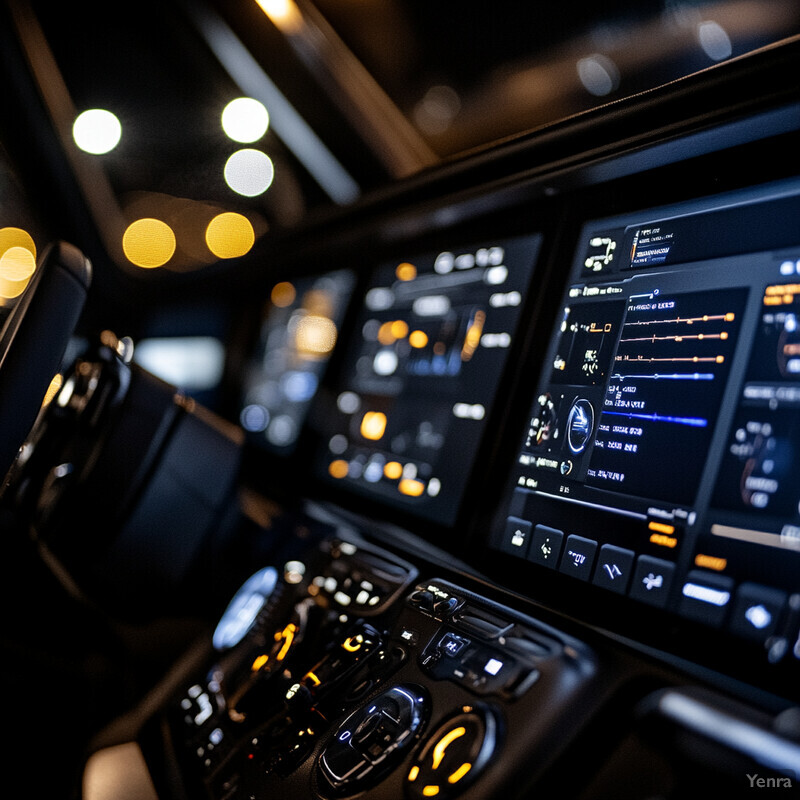
Instead of relying on rigid, one-size-fits-all thresholds for temperature, humidity, or vibration, AI enables thresholds to adapt dynamically to the context. For instance, what may be a normal internal temperature for one cargo type could be detrimental to another. Machine learning models continuously learn optimal conditions for each type of shipment, factoring in route, climate, season, and historical performance. As these models refine their understanding, they autonomously adjust acceptable ranges. This reduces the frequency of unnecessary alarms and allows handlers to focus on true risks, ensuring that interventions are both precise and timely.
7. Predictive Maintenance for Equipment
AI insights into the performance of refrigeration units and related equipment help schedule preventative maintenance, reducing downtime and preventing cargo deterioration.
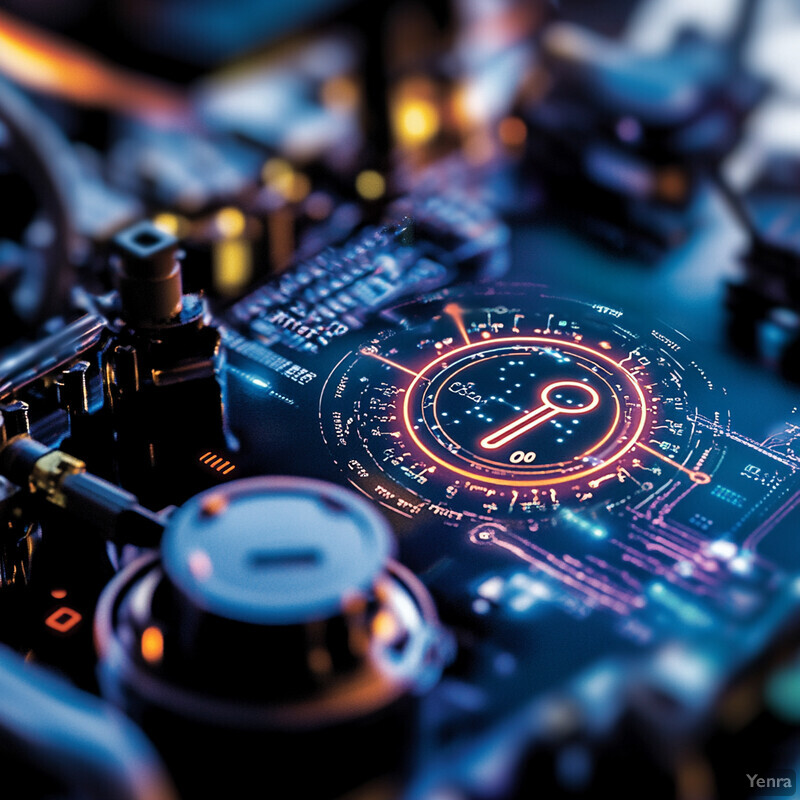
One of the indirect but critical ways AI enhances cargo condition monitoring is by predicting when the equipment that maintains cargo conditions—like refrigeration units, ventilation systems, or cargo-handling machinery—might fail. By monitoring energy consumption patterns, vibration profiles, and historical downtime records, AI models can forecast potential breakdowns before they occur. Logistics operators can then schedule timely inspections and repairs, minimizing unexpected failures that could compromise an entire shipment. Predictive maintenance not only helps preserve cargo quality but also optimizes operational efficiency, resource allocation, and cost management.
8. Smart Alert Prioritization
Algorithms can rank alerts based on severity, historical patterns, and contextual data, ensuring that handlers focus on the most critical issues first.
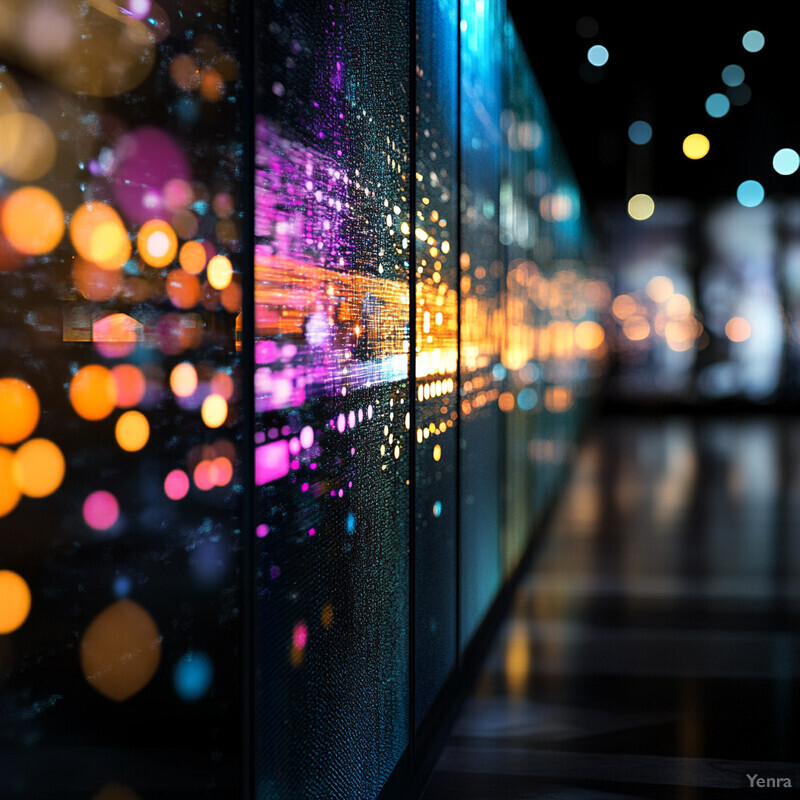
Cargo monitoring can generate a steady stream of alerts, not all of which demand immediate action. AI can sift through these alarms, weighting their urgency and severity based on historical outcomes, cargo type sensitivity, and environmental context. By intelligently prioritizing alerts, AI ensures that human operators aren’t overwhelmed by trivial or non-urgent notifications. Instead, they can focus on high-impact situations—like a sudden drop in oxygen levels for live animal shipments or a rapid temperature rise in a pharmaceutical container. The net effect is increased efficiency and more effective decision-making, as operations teams can swiftly address the most pressing matters first.
9. Supply Chain Risk Management
By analyzing environmental, geopolitical, and seasonal trends, AI can forecast disruptions and recommend alternate routes or storage conditions to preserve cargo quality.
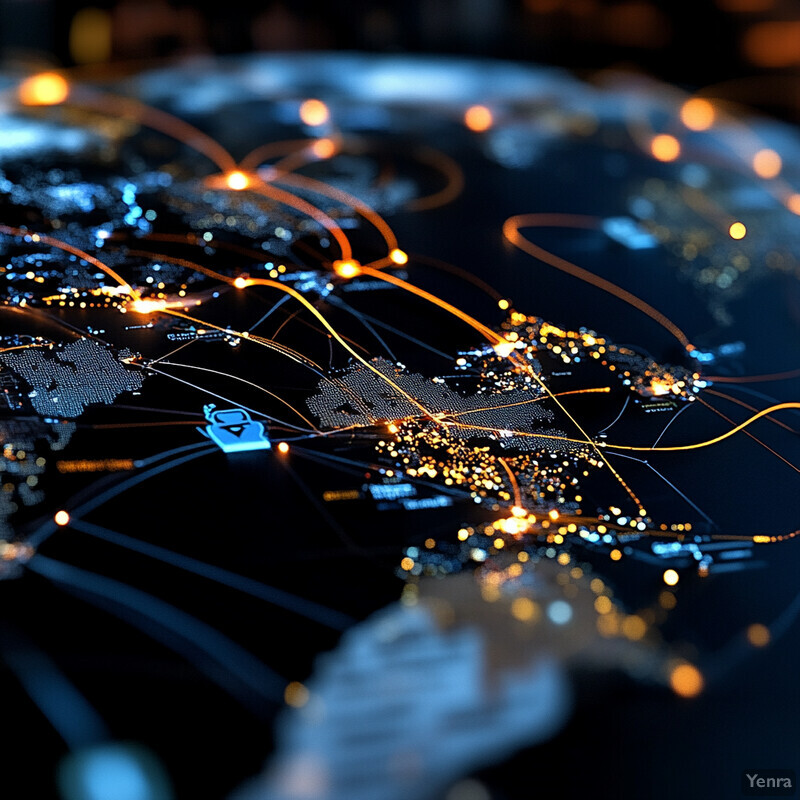
AI models can assess a wide range of external factors that affect cargo conditions, from geopolitical instability to seasonal weather changes and port congestion. By integrating real-time transportation data with predictive analytics, AI can anticipate disruptions along the route and recommend contingency plans. For example, if severe storms are predicted along a shipping lane, the system might propose an alternate route or suggest reinforcing refrigeration systems to maintain cargo temperatures during delays. This holistic approach to risk management prevents unexpected environmental factors from diminishing cargo quality and helps maintain schedule integrity.
10. Enhanced Compliance Monitoring
Automated analysis of sensor logs and documentation supports adherence to safety and regulatory standards (e.g., FDA, HACCP), reducing the risk of penalties or recalls.
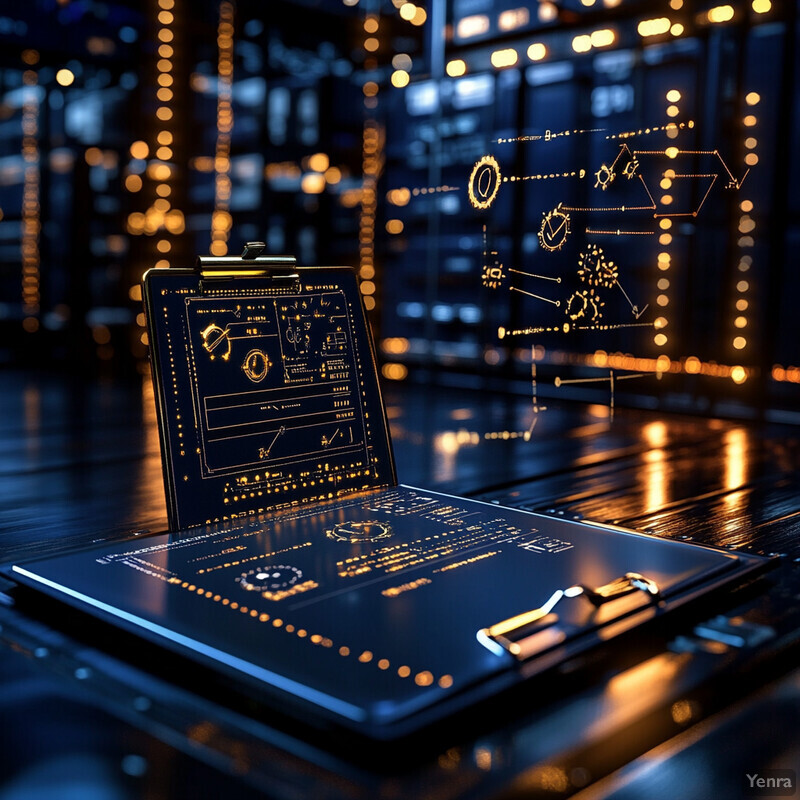
Regulatory standards for cargo, especially for sensitive goods like pharmaceuticals or hazardous materials, are stringent. AI aids compliance by automatically reviewing sensor logs, comparing conditions against regulatory benchmarks, and flagging any periods of deviation. It can also streamline audit processes by generating comprehensive compliance reports, complete with timestamps, sensor readings, and corrective actions taken. This level of automated oversight ensures that organizations meet regulatory mandates, maintain certifications, and reduce the likelihood of costly fines, recalls, or legal disputes stemming from non-compliance.
11. Dynamic Route Optimization
AI can factor in weather forecasts, traffic conditions, and cargo fragility to select transport routes that minimize damage and maintain optimal conditions.
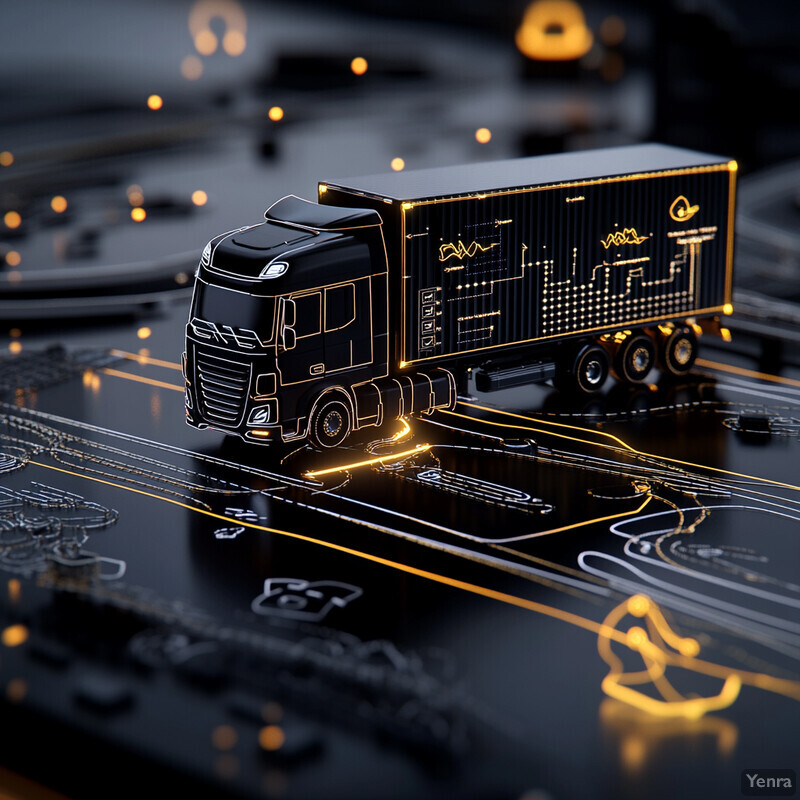
AI-driven route optimization tools factor in cargo conditions, weather forecasts, traffic patterns, and even real-time handling delays at ports or warehouses. By forecasting environmental conditions en route, these systems can propose adjustments—such as changing a delivery path to avoid extreme heat or humidity. The recommended route might ensure better temperature stability for sensitive shipments, reduce fuel consumption by avoiding congestion, and shorten overall transit times. With AI-powered routing, logistics providers can maintain the ideal state of their cargo throughout the journey, mitigating risks of spoilage or damage.
12. Scenario Simulation with Digital Twins
By creating digital replicas of cargo, containers, and supply chain routes, AI can simulate 'what-if' scenarios, testing interventions before implementing them in the real world.
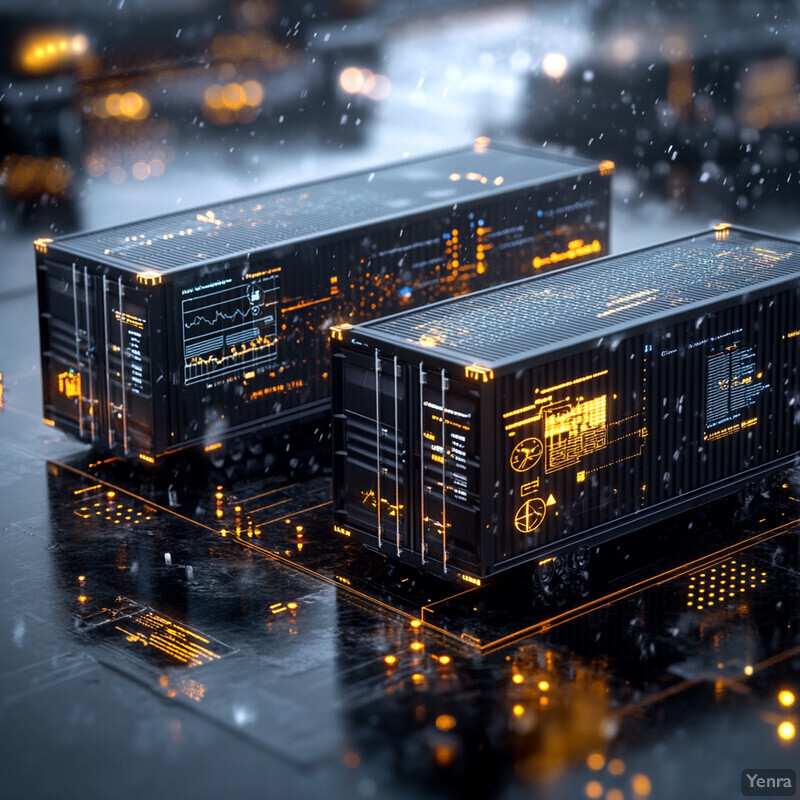
Digital twins—virtual replicas of physical cargo containers, routes, and supply chain environments—allow logistics teams to test various “what-if” scenarios. By applying AI-driven simulations, they can see how cargo conditions might change if temperature control systems fail, routes are delayed, or sensors malfunction. These simulations empower operators to pre-emptively devise contingency plans and resilience strategies before a real event occurs. Digital twins facilitate continuous improvement in cargo handling and monitoring protocols, ensuring that logistics providers stay a step ahead of potential disruptions.
13. Context-Aware Environmental Controls
Intelligent systems can autonomously adjust temperature, ventilation, or humidity settings on refrigerated trucks or containers to maintain peak quality of the cargo.
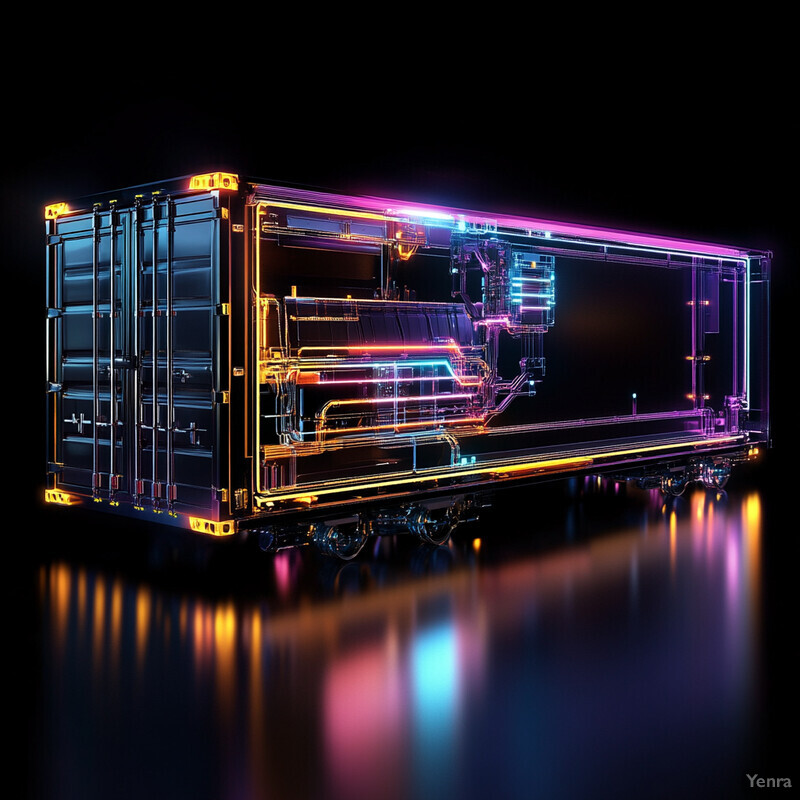
AI can interface directly with environmental control systems—such as refrigeration units and ventilation controls—to dynamically adjust them based on current and predicted cargo conditions. For example, if sensors detect that the internal container temperature is nearing an upper threshold, the AI can lower the set point of the refrigeration system. If certain humidity-sensitive cargo is en route through a humid climate, the system may increase ventilation. By continually recalibrating environmental conditions, AI ensures that cargo is maintained in an optimal state, reducing the risk of spoilage and maintaining product quality throughout transit.
14. Fraud and Tampering Detection
Data-driven anomaly detection can alert stakeholders to unauthorized container openings, unplanned stops, or sensor manipulations that suggest tampering.
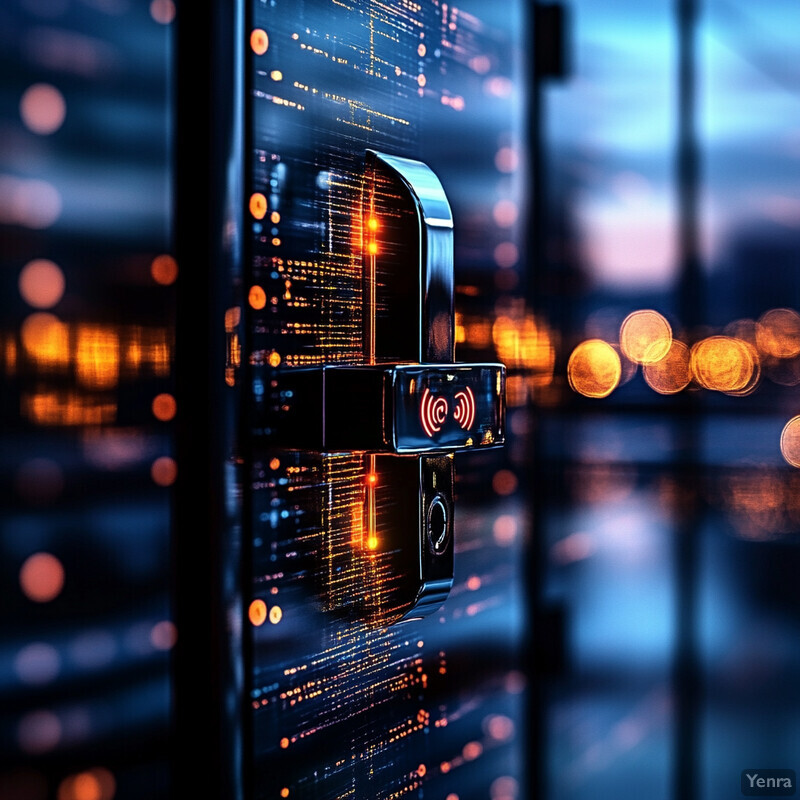
Through anomaly detection algorithms and sensor data analysis, AI can identify suspicious activities, such as unexpected container door openings, sudden deviations in route, or unusual movements. Combined with geolocation and timeline data, these insights can indicate possible theft or tampering. AI can then alert security teams or trigger automated responses, such as locking mechanisms or activating onboard cameras. As a result, logistics providers gain an added layer of security and integrity in their supply chains, protecting valuable cargo and reducing insurance costs related to theft or adulteration.
15. Shelf-Life Forecasting
Through continuous learning, AI can refine predictions about how long a cargo will remain saleable under given conditions, improving inventory and logistics planning.
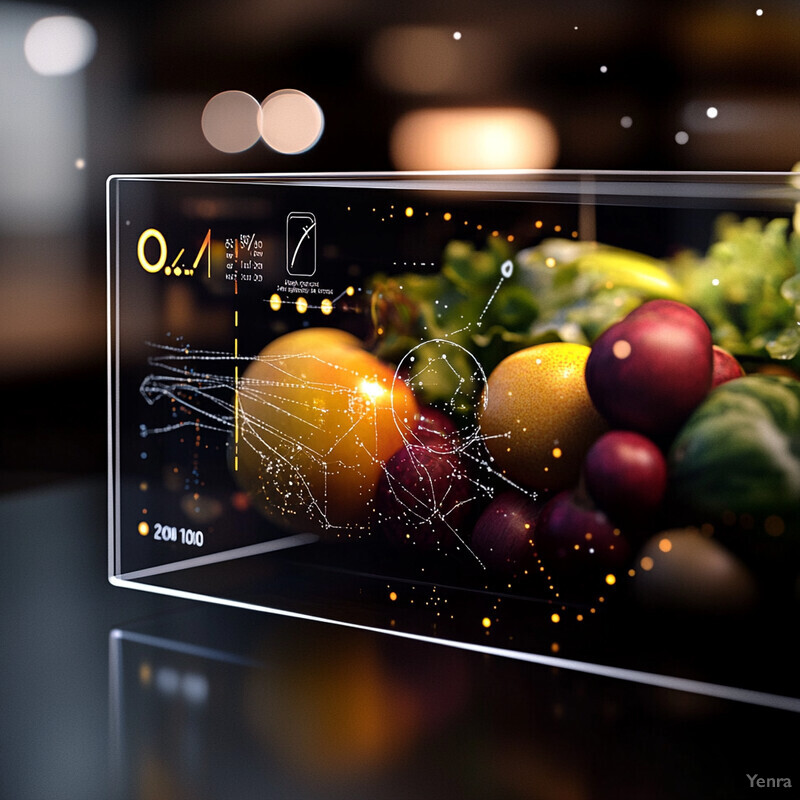
With AI, it’s possible to forecast the shelf life of products under given conditions more accurately than ever. By integrating real-time sensor data with product-specific spoilage models, AI can predict when a batch of perishable goods will no longer meet quality standards. Logistics teams can use this information to make informed decisions, such as prioritizing the delivery of items with shorter remaining shelf life, redistributing stock to nearby markets, or even adjusting environmental parameters to extend shelf life. This level of foresight leads to better inventory management, reduced waste, and improved profit margins.
16. Real-Time Condition Dashboards
AI-driven analytics populate dashboards with live, actionable intelligence, offering stakeholders an at-a-glance understanding of the entire cargo network’s status.
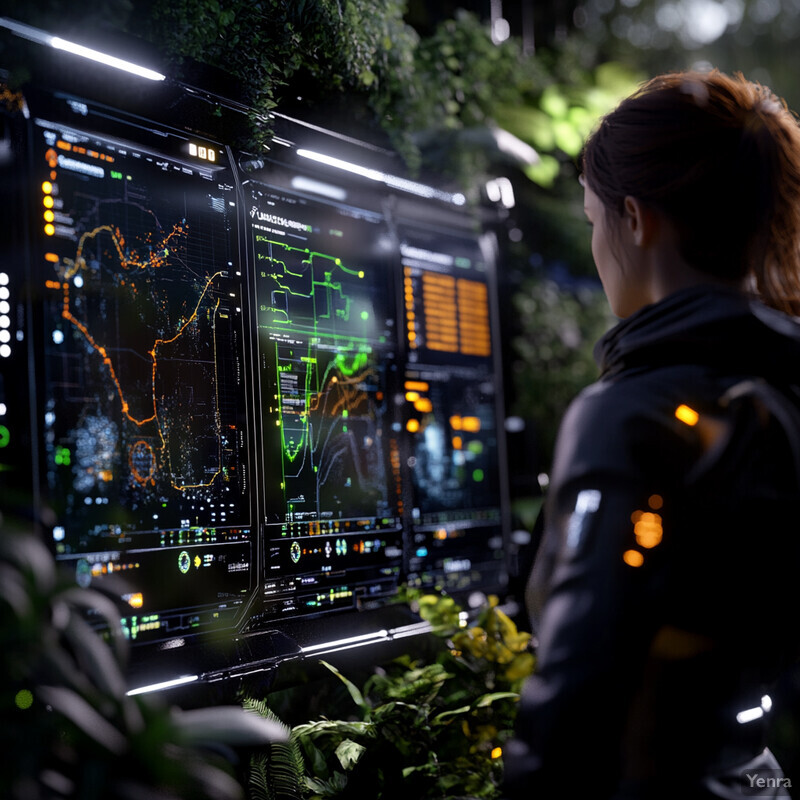
AI can continuously feed, aggregate, and interpret complex sets of sensor and contextual data, presenting the distilled insights on intuitive dashboards. These dashboards offer logistics managers, drivers, and quality assurance teams a real-time view of cargo conditions throughout the supply chain. Rich data visualizations—such as color-coded maps, trend charts, and alert panels—allow swift interpretation and responsive decision-making. By providing stakeholders with a single pane of glass that reflects the true state of their cargo, these dashboards improve transparency, accountability, and operational clarity.
17. Automated Documentation and Reporting
Natural Language Generation (NLG) can produce summaries of cargo conditions and supply chain events, streamlining administrative tasks and record-keeping.
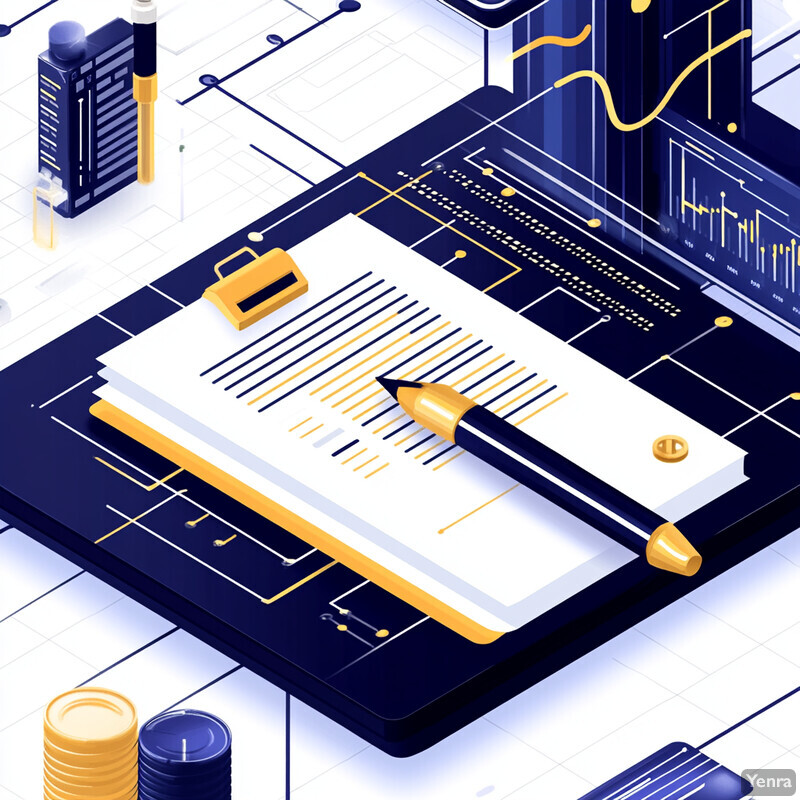
Through natural language generation (NLG) and automated report compilation, AI can produce detailed summaries, compliance documents, and quality reports without manual effort. By pulling from sensor logs, historical data, and event registries, the system can generate human-readable narratives that explain what occurred during transit, whether conditions were consistently maintained, and if any exceptions took place. Automated documentation ensures that record-keeping is accurate, timely, and consistent, reducing administrative burdens, simplifying regulatory audits, and maintaining a verifiable archive of cargo conditions.
18. Continuous Improvement Through Feedback Loops
AI models learn from every shipment, refining their predictions and recommendations to improve long-term accuracy and efficiency.
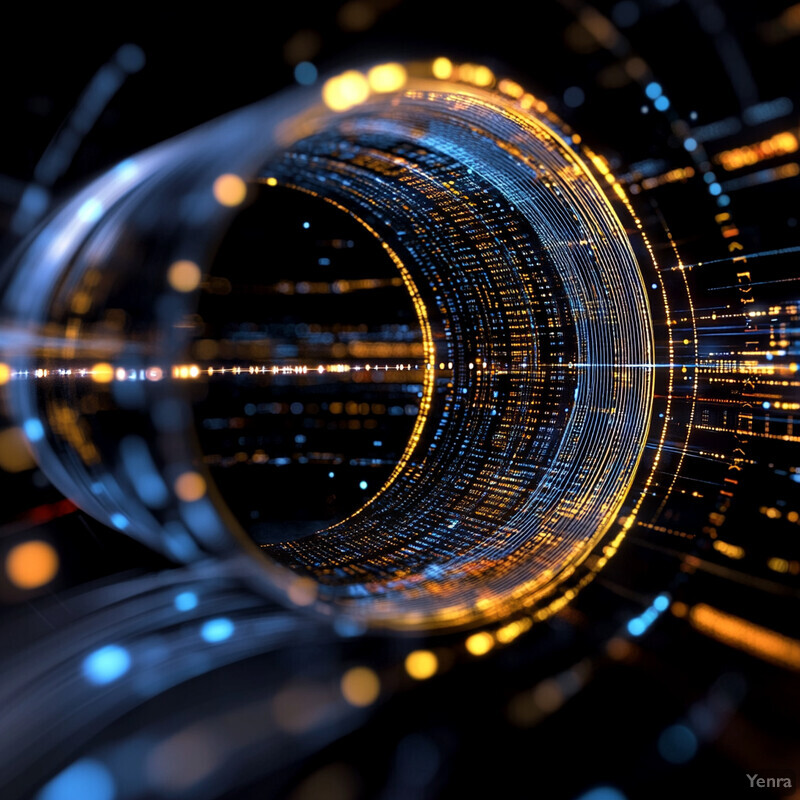
AI models thrive on data and feedback. Every shipment that passes through a monitoring system offers a new dataset with insights about cargo conditions, successful interventions, and mistakes to avoid. By continuously retraining and refining models with fresh data, the AI system becomes increasingly adept at predicting issues, optimizing thresholds, and advising on best practices. Over time, these iterative improvements lead to more stable supply chains, fewer losses, and enhanced confidence in the technology’s recommendations, ultimately driving higher operational standards and customer satisfaction.
19. Efficient Space Utilization
By predicting temperature and humidity gradients within containers, AI can assist in optimal loading patterns that improve air circulation and reduce spoilage risk.
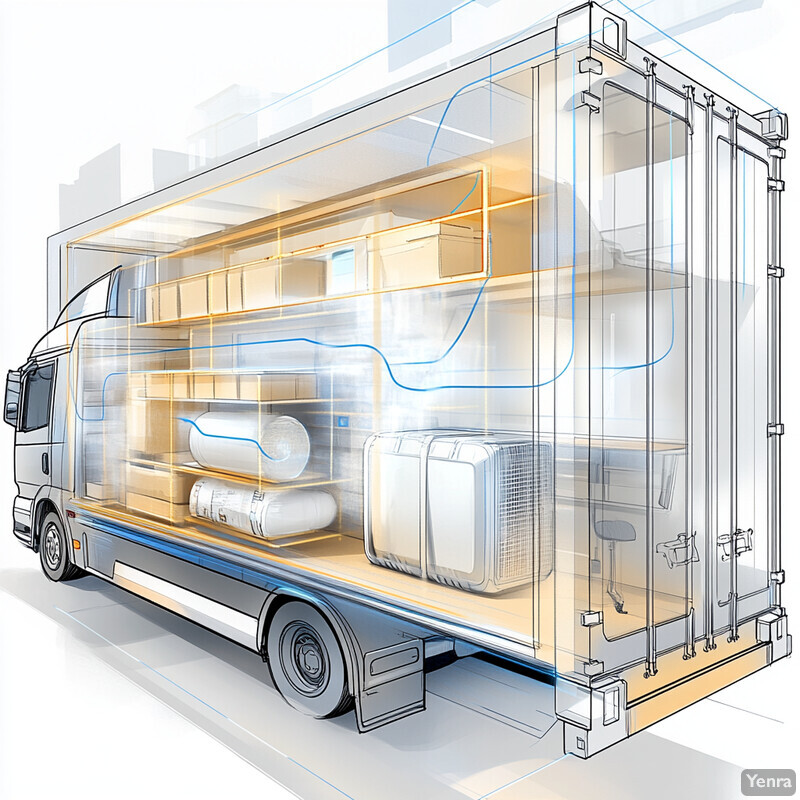
By analyzing how different loading configurations affect airflow, temperature distribution, and humidity levels, AI can help optimize how cargo is arranged within containers. For instance, it may suggest stacking patterns or positioning temperature-sensitive goods near cooling vents. AI-driven spatial optimization ensures even environmental conditions, reduces hotspots, and limits areas where spoilage might occur. This efficiency in loading not only preserves product quality but also maximizes space utilization, potentially lowering transportation costs and improving overall logistics efficiency.
20. Integration with Blockchain and IoT
When combined with IoT sensors and blockchain ledgers, AI ensures secure, transparent, and verifiable cargo condition histories, enhancing trust among all supply chain partners.
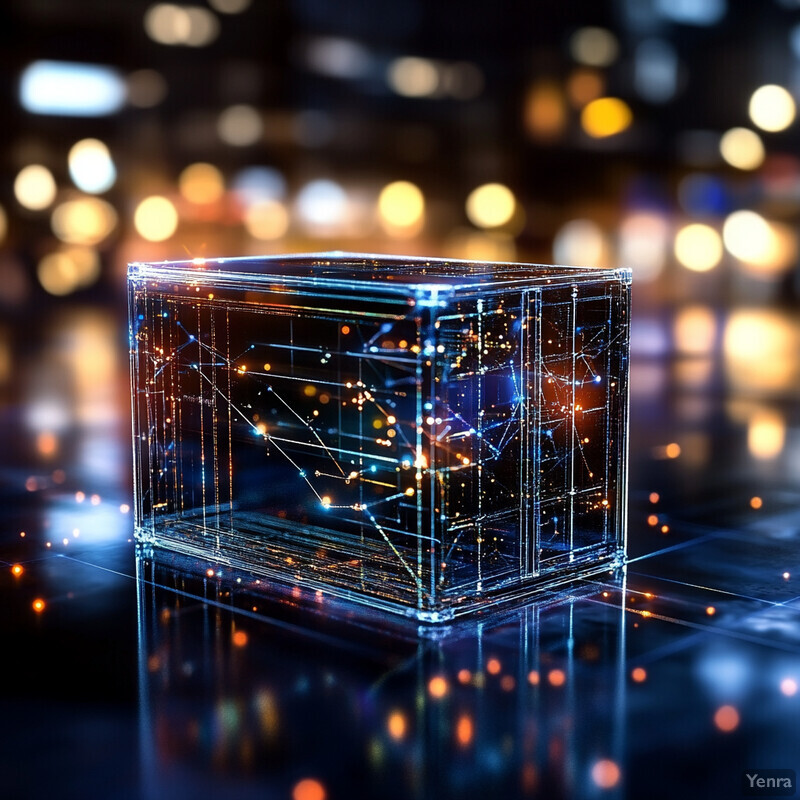
When AI is combined with Internet of Things (IoT) sensors and blockchain ledgers, the transparency and trustworthiness of the entire cargo lifecycle increase dramatically. IoT devices continuously feed accurate, real-time data into AI-driven analytics platforms, while blockchain ensures that the resulting data and records are tamper-proof and easily auditable. This integration provides a verifiable chain of custody, guaranteeing that cargo conditions were maintained as claimed. Stakeholders—ranging from manufacturers and distributors to regulators and end customers—can trust the integrity of the supply chain, knowing that data-driven insights are both reliable and secure.