1. Automated Tumor Segmentation in Imaging
AI algorithms, especially deep learning models, can accurately and rapidly identify and delineate tumor boundaries from CT, MRI, and PET scans, reducing the time and variability associated with manual contouring.
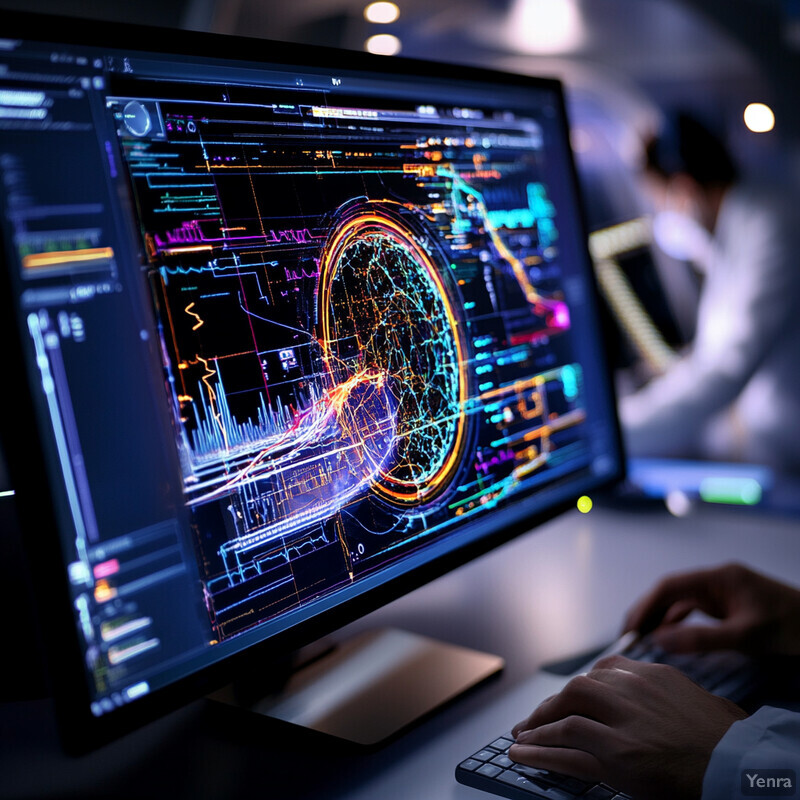
Traditional approaches to delineating tumor boundaries on diagnostic imaging scans—such as CT, MRI, and PET—often require time-consuming manual effort by highly trained radiologists or radiation oncologists. Variability in how different clinicians interpret these images can lead to inconsistencies in treatment planning. AI-powered segmentation tools, based primarily on deep learning models, can rapidly and accurately identify tumor margins, reducing the time needed for initial planning. By standardizing the segmentation process, these tools help ensure more reliable inputs into the treatment planning system, thereby improving both the precision and reproducibility of care.
2. Personalized Treatment Recommendations
By analyzing patients’ genetic profiles, medical histories, and treatment outcomes, AI-driven decision support tools can recommend the most effective therapies tailored to an individual’s specific cancer subtype and biomarkers.
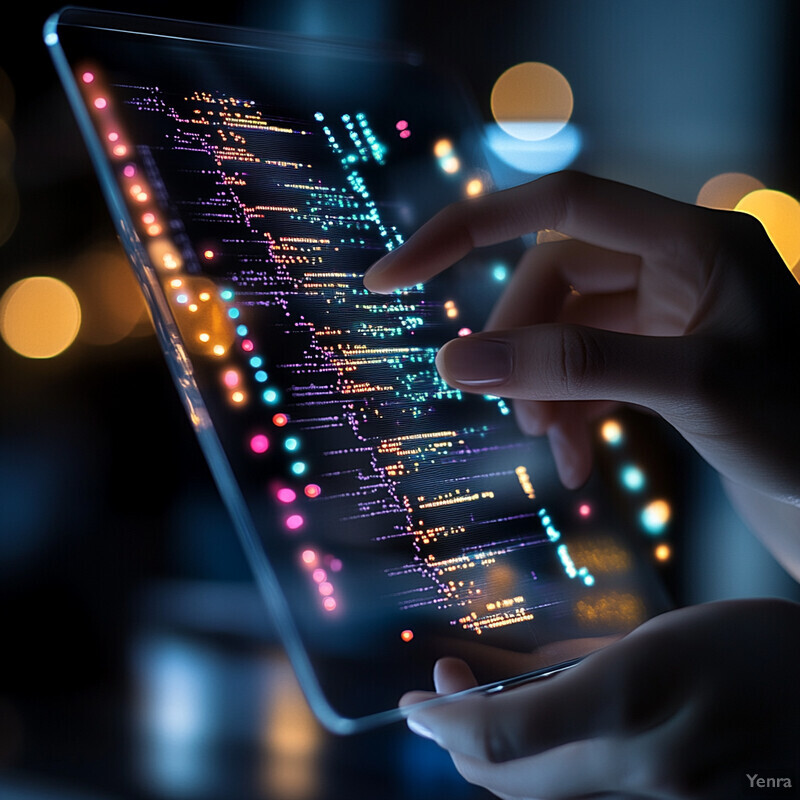
One-size-fits-all approaches to cancer treatment have given way to the promise of precision oncology, where therapy is tailored to the patient’s specific tumor genetics, biomarker profiles, and overall clinical context. AI algorithms can integrate and analyze this multidimensional data—from DNA sequencing results to patient demographics—and predict which treatment protocols are most likely to be effective. By comparing a patient’s unique molecular fingerprint with large datasets of past cases and outcomes, AI-driven decision support systems can guide oncologists toward targeted therapies, immunotherapies, or other personalized regimens, increasing the likelihood of better outcomes and fewer unnecessary interventions.
3. Adaptive Radiotherapy Planning
AI can continuously analyze imaging and clinical data during radiotherapy, adapting the treatment plan in real-time to account for tumor shrinkage, patient movement, or changes in tissue density, thus improving treatment accuracy and minimizing side effects.
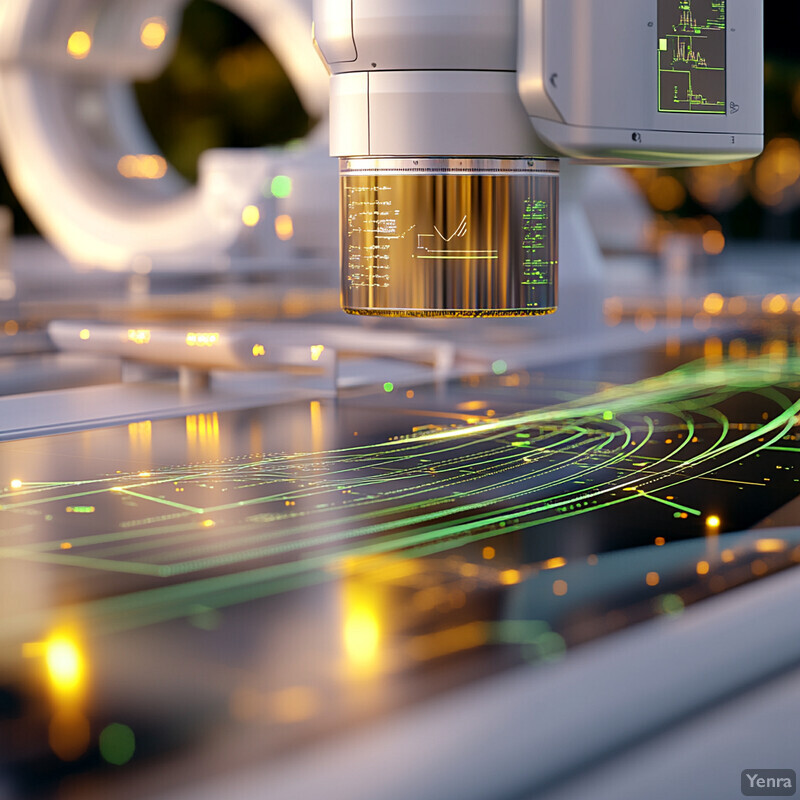
Radiotherapy plans, once set at the beginning of a treatment course, historically remained relatively static. However, patient anatomy, tumor size, and tumor position can change over the course of treatment. AI-enabled adaptive radiotherapy allows for continuous assessment of imaging data and ongoing patient monitoring. These intelligent systems can automatically adjust beam intensities, angles, and other parameters based on real-time feedback, ensuring optimal dose delivery to the tumor while minimizing radiation exposure to healthy tissues. In doing so, adaptive planning not only enhances the efficacy of treatment but also reduces complications and improves patient comfort.
4. Integrating Multi-Omics Data
Advanced machine learning models can incorporate genomic, proteomic, transcriptomic, and metabolomic data, helping clinicians identify novel molecular targets and predictive biomarkers that guide more effective treatment planning.
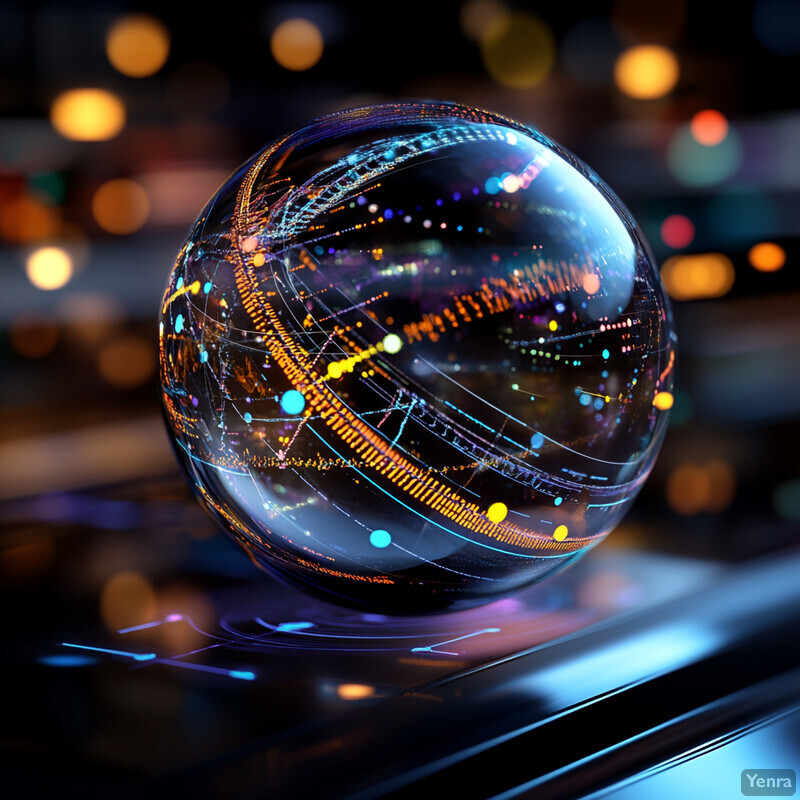
Cancer is driven by complex interactions at the genetic, proteomic, transcriptomic, and metabolic levels. Combining these “omics” datasets into a coherent picture used to be a daunting task. Advanced AI models now excel at integrating and interpreting these vast, heterogeneous datasets. By doing so, these models can identify novel molecular markers, uncover hidden patterns indicative of certain cancer subtypes, and guide clinicians in selecting targeted treatments aligned with a tumor’s unique molecular characteristics. This holistic view of the tumor’s biology empowers a more nuanced and effective treatment planning process.
5. Predictive Modeling of Treatment Response
Using large datasets of past cases, AI systems can predict how a particular patient’s tumor will respond to various therapies, helping oncologists choose the best treatment strategy upfront.
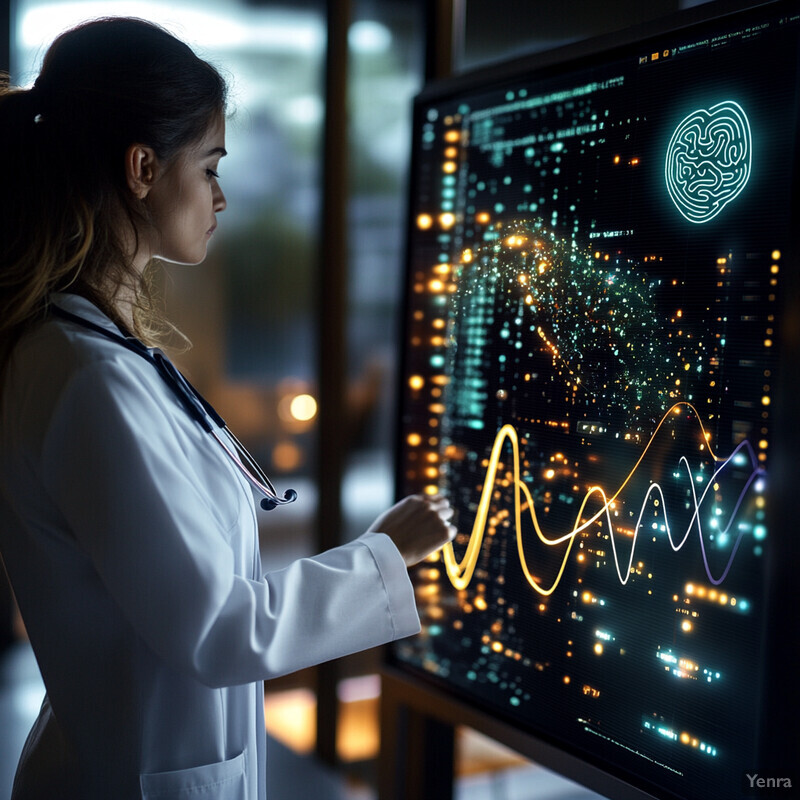
Oncologists often face uncertainty in predicting how a given tumor will respond to a particular therapy. AI-driven predictive models, trained on thousands of historical patient cases and outcomes, can forecast the likely effectiveness of chemotherapy, immunotherapy, radiation, or combination treatments. These models consider factors such as tumor stage, genetic mutations, and patient comorbidities. By having a data-driven estimate of how well a patient is likely to respond, clinicians can select the most promising therapeutic avenues upfront, potentially reducing time spent on ineffective treatments and minimizing unnecessary side effects.
6. Risk Stratification and Prognostication
Sophisticated predictive models can estimate survival times, recurrence risks, and the likelihood of treatment-related toxicities, enabling clinicians to counsel patients more effectively and consider prophylactic interventions when needed.
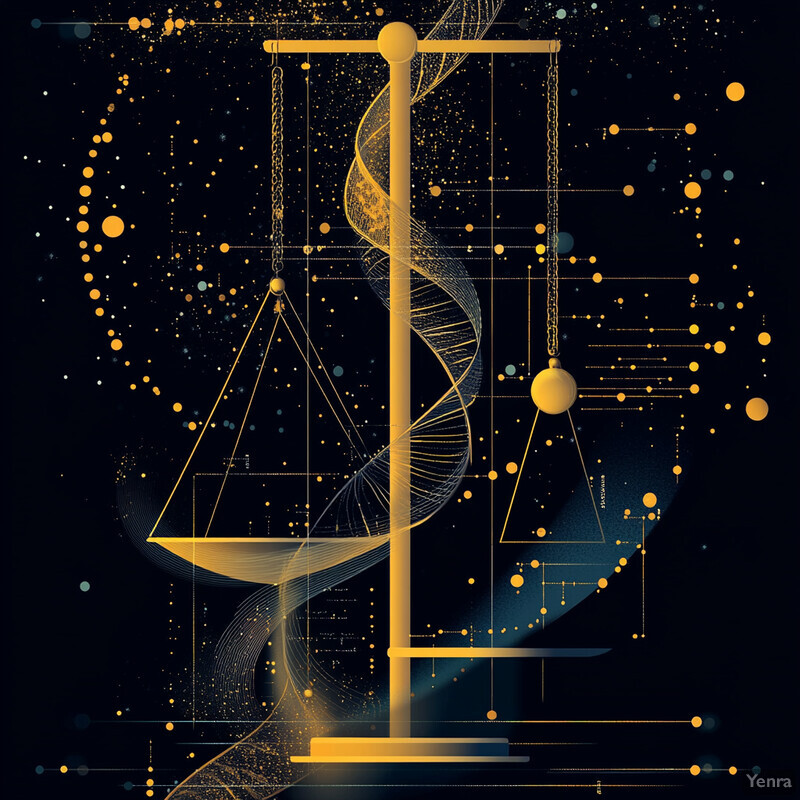
Understanding a patient’s risk profile is crucial for setting expectations and guiding treatment intensity. AI models can assess the likelihood of tumor recurrence, metastasis, or treatment-related side effects by analyzing large datasets of past patient outcomes in combination with clinical, pathological, and imaging data. These prognostic tools help physicians stratify patients into risk categories, informing how aggressively to treat and enabling timely interventions for those at higher risk. Ultimately, AI-driven risk stratification supports more individualized patient counseling, better resource allocation, and improved overall patient management.
7. Optimizing Drug Dosing and Schedules
Through reinforcement learning and simulation, AI can determine optimal chemotherapy or targeted therapy dosing regimens and scheduling, aiming to maximize efficacy while minimizing harmful side effects.
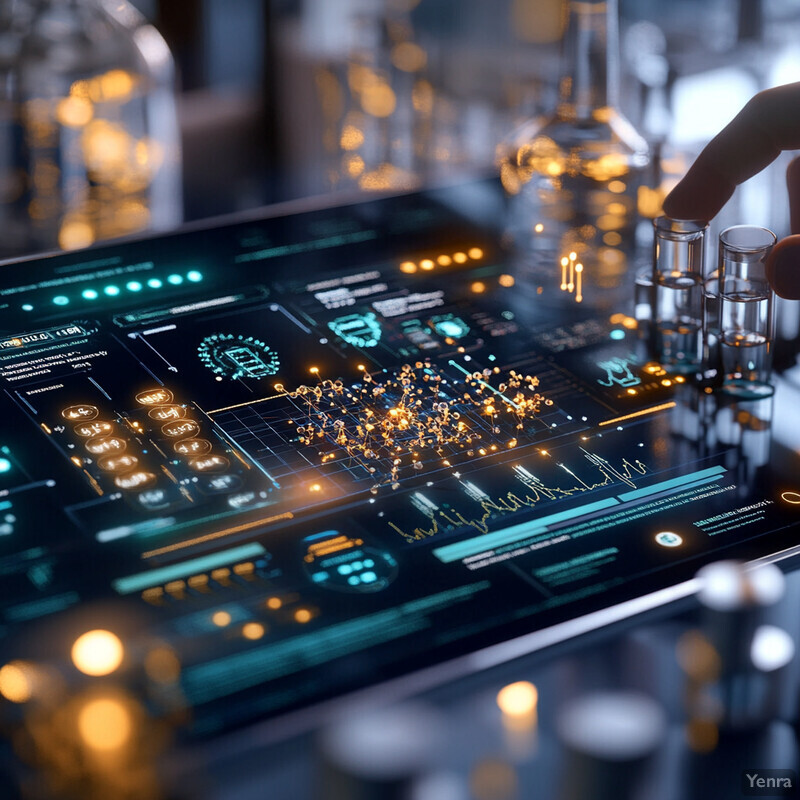
Determining the correct dosage and scheduling of anticancer drugs can be challenging, as clinicians must balance efficacy against toxicity. AI algorithms, including reinforcement learning models, can explore treatment scenarios virtually, adjusting dosage and timing to maximize patient benefit and minimize side effects. By continuously refining their strategies as more patient data becomes available, these models help oncologists arrive at treatment regimens that are both safer and more effective. This data-driven approach reduces trial-and-error in dosing decisions, improving patient quality of life and therapy outcomes.
8. Quality Assurance in Treatment Planning
Machine learning models can serve as an independent check of human-generated treatment plans, flagging potential errors in radiation dosing, target delineation, or beam arrangement before treatment is delivered.
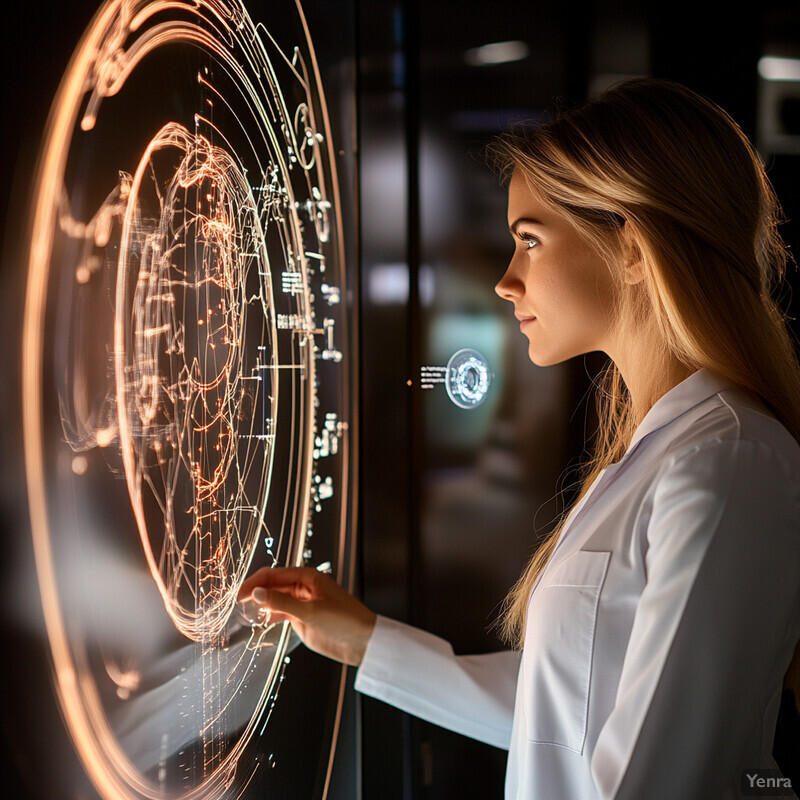
Even the most experienced clinicians can introduce errors or oversights during the planning phase. AI-based quality assurance systems serve as a valuable second check, reviewing radiation dose distributions, target volumes, and organ-at-risk delineations. These systems can flag potential anomalies, discrepancies, or suboptimal plan parameters, prompting the treatment team to make necessary adjustments before therapy begins. By acting as an intelligent safety net, AI helps maintain high standards of care, safeguarding patients against preventable treatment errors.
9. Virtual Patient Simulations
AI-powered virtual patient models can simulate outcomes under multiple treatment plans, allowing oncologists and dosimetrists to 'test drive' different strategies and pick the one with the best projected outcome.
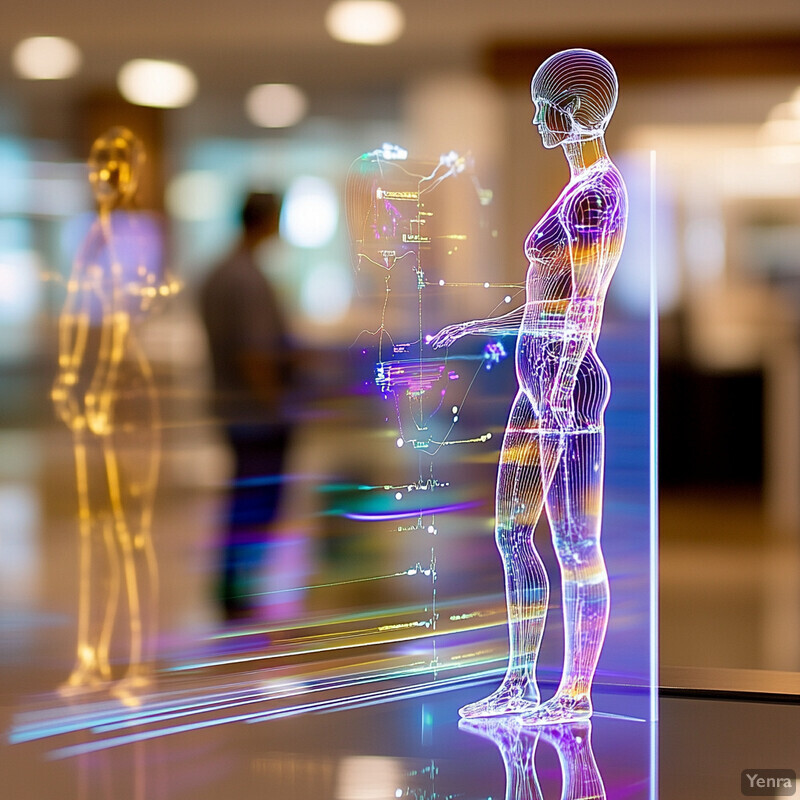
AI-driven virtual patient models allow oncologists and dosimetrists to simulate various “what-if” scenarios before finalizing a treatment plan. These models incorporate patient anatomy, tumor characteristics, and therapy modalities to predict potential outcomes. For example, clinicians can compare projected responses to different radiation beam configurations or chemotherapy combinations. By evaluating multiple plans without putting the patient at risk, these simulations streamline the selection process, leading to more confident decisions and treatments that are better aligned with the patient’s unique situation.
10. Natural Language Processing of Clinical Notes
By extracting meaningful insights from unstructured patient records, research publications, and clinical guidelines, AI can help ensure that the latest evidence and treatment options are considered in the planning phase.
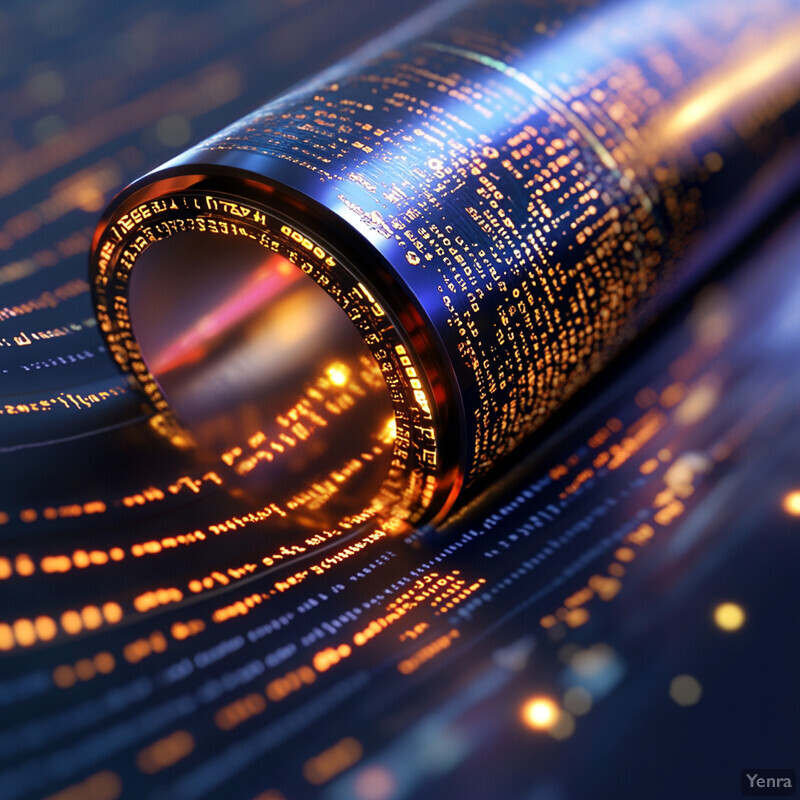
A wealth of valuable clinical information is contained in unstructured formats, such as physician notes, pathology reports, and published research articles. AI-driven natural language processing (NLP) tools can extract and organize critical insights from these narrative documents. By identifying key concepts, treatment guidelines, and evidence-based recommendations in real-time, NLP systems ensure that clinicians have immediate access to the most current and comprehensive knowledge base. This enables a more informed and evidence-guided treatment planning process.
11. Clinical Trial Matching
AI can rapidly assess patient characteristics against complex trial eligibility criteria, ensuring that patients are offered the most cutting-edge treatment options available through clinical research studies.
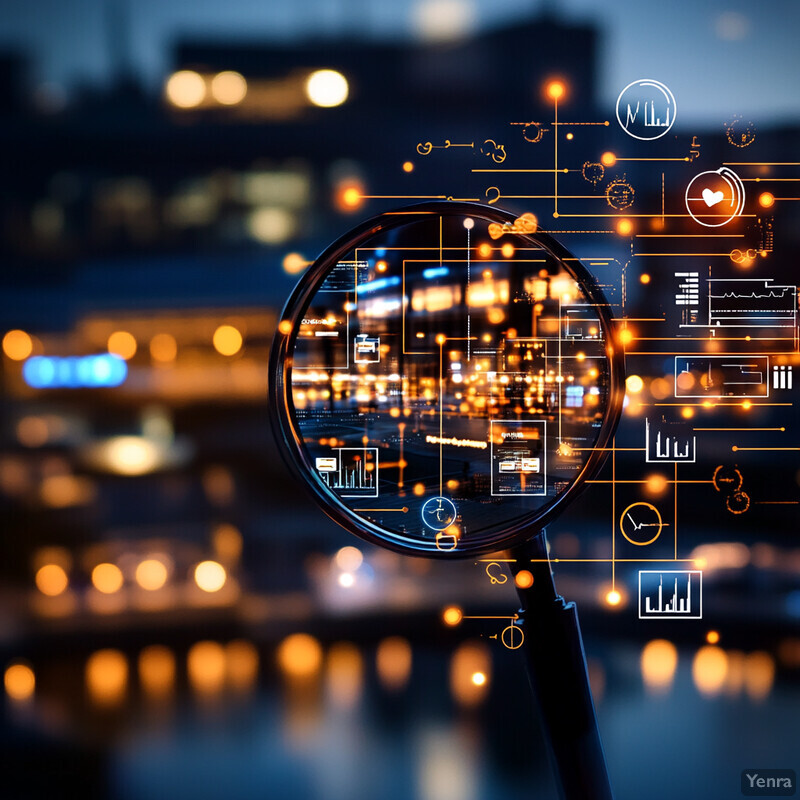
Matching patients to suitable clinical trials can be a painstaking and complex task, as eligibility criteria often involve subtle combinations of genetic, clinical, and demographic factors. AI can rapidly evaluate a patient’s data against trial requirements—something that would take a human expert hours or days. By more efficiently identifying eligible patients, these systems enhance access to cutting-edge therapies, expedite the recruitment process for clinical studies, and ensure patients receive opportunities to benefit from the latest advances in cancer treatment.
12. Integrating Imaging, Pathology, and Clinical Data
AI can fuse radiological imaging findings, histopathological analysis, and patient-specific variables into a unified platform, offering a more holistic view of the patient’s disease and optimal treatment approach.
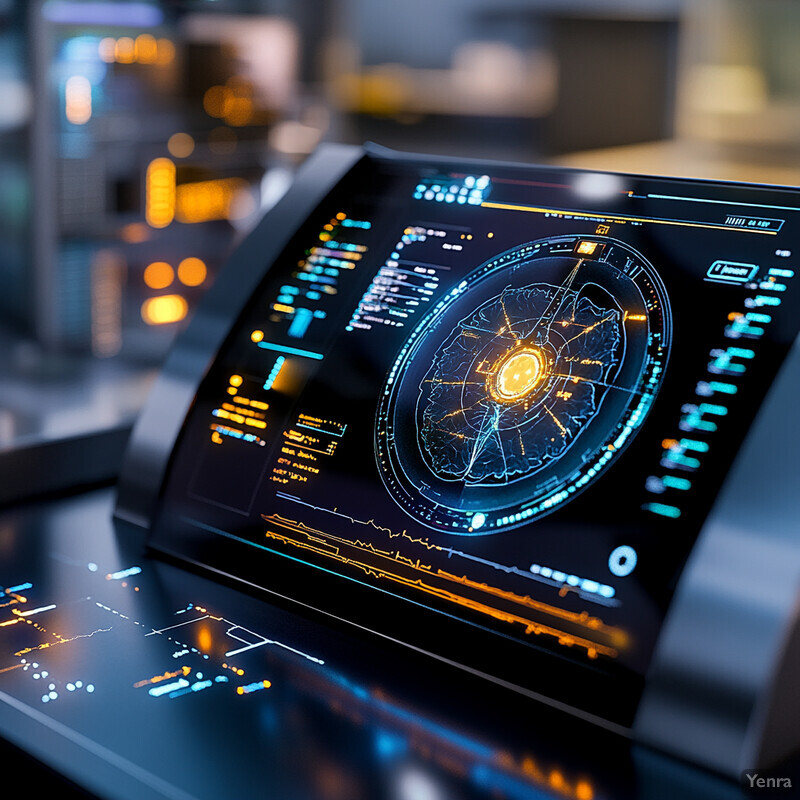
Cancer treatment planning requires input from multiple specialties: radiology, pathology, medical oncology, and more. AI excels at fusing this diverse information—imaging findings, histopathological results, lab values, patient-reported symptoms, and past treatment history—into a single coherent framework. By providing a comprehensive, integrated view of the patient’s case, AI helps multidisciplinary teams collaborate more effectively and make better-informed decisions about treatment strategies, potentially leading to more precise and personalized care.
13. Auto-Contouring of Organs-at-Risk
Beyond tumors, AI can swiftly identify and delineate healthy tissues like the spinal cord, lungs, or salivary glands, ensuring that radiation therapy plans minimize damage to these critical structures.
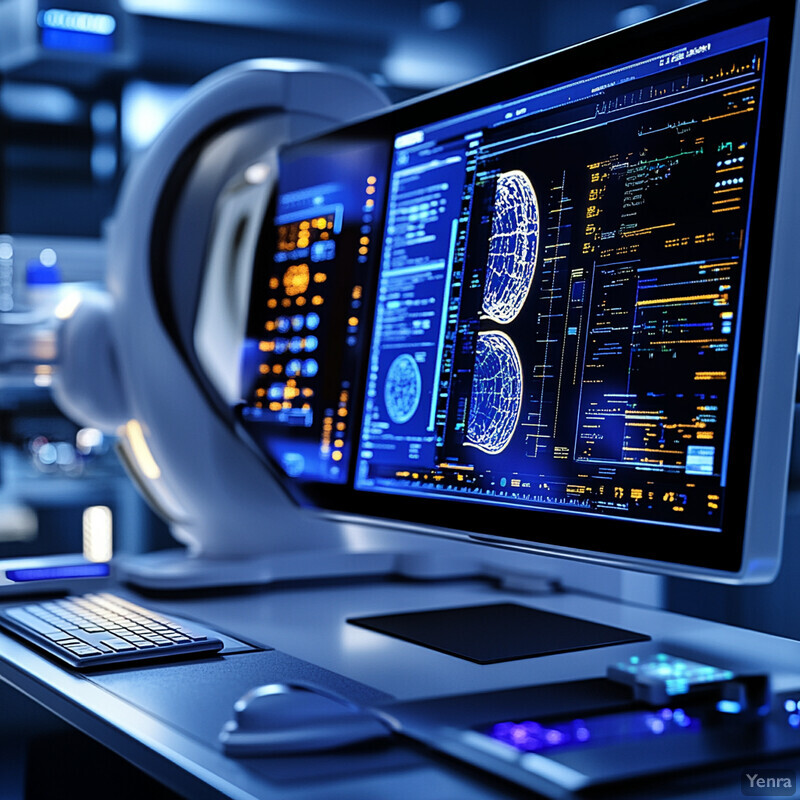
Beyond identifying tumors, it’s critical to accurately map out surrounding healthy organs that must be protected during radiation treatment. Manually outlining organs-at-risk can be labor-intensive and prone to variability. AI-driven auto-contouring tools can quickly and consistently delineate these structures, significantly reducing the time required for treatment planning. The improved accuracy and consistency ensure that radiation dose is carefully calibrated, minimizing potential damage to healthy tissue and improving patient outcomes.
14. Optimizing Surgical Planning
AI can help surgeons by integrating imaging data to map the precise boundaries of tumors and critical structures, assisting in planning minimally invasive procedures that reduce complications and recovery time.
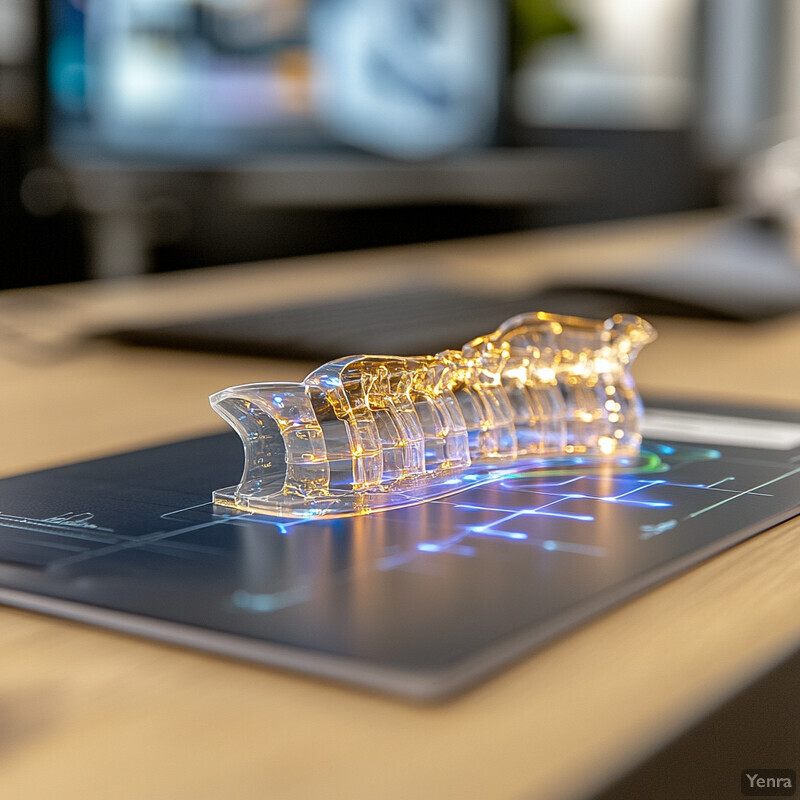
For surgical oncology, precise knowledge of a tumor’s location, size, and its relationship to vital anatomical structures is paramount. AI can integrate preoperative imaging data—such as MRI and CT scans—and construct detailed 3D models that guide the surgeon in planning minimally invasive approaches. Such planning reduces unnecessary tissue removal, shortens operation times, and may improve postoperative recovery. AI tools can also predict surgical outcomes, helping surgeons choose the best operative techniques for each patient.
15. Guiding Combination Therapy Selection
By predicting synergistic effects between drugs, radiation, and immunotherapies, AI can help identify combination treatments more likely to improve patient outcomes.
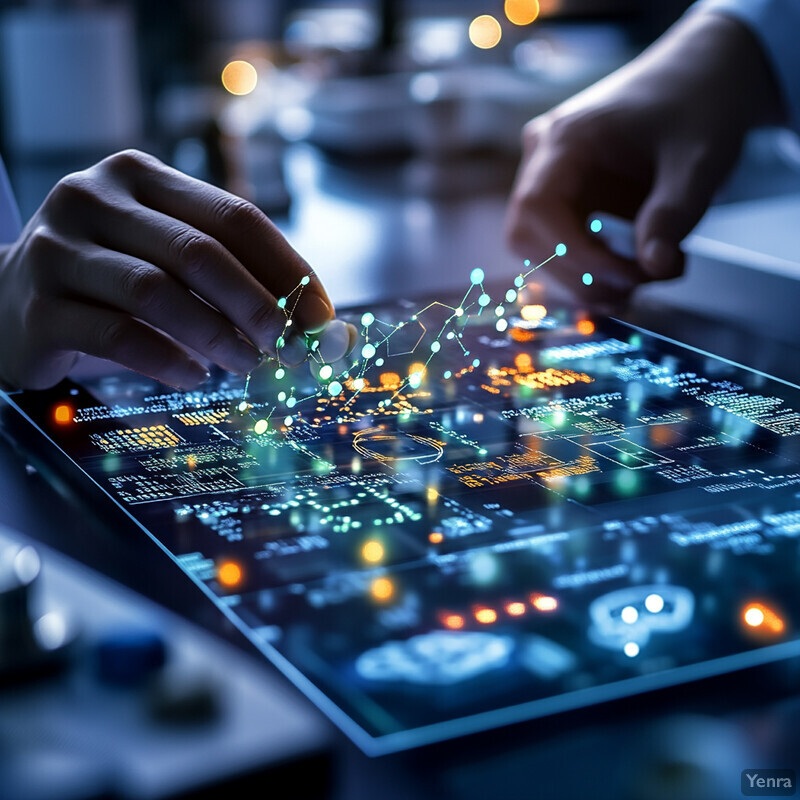
Today’s oncologists have many treatment modalities at their disposal, including chemotherapy, targeted therapies, immunotherapies, and radiation. Determining the best combination can be challenging due to complex interactions and potential toxicities. AI models can analyze large datasets to identify patterns of synergistic benefit or antagonism between agents. By suggesting combination treatments that have historically yielded better survival rates or fewer side effects, these models enable clinicians to craft more effective multimodal treatment plans.
16. Shortening Time to Treatment Start
Automated workflows, from imaging interpretation to treatment plan generation, can reduce administrative and planning bottlenecks, ensuring patients begin their treatment sooner.
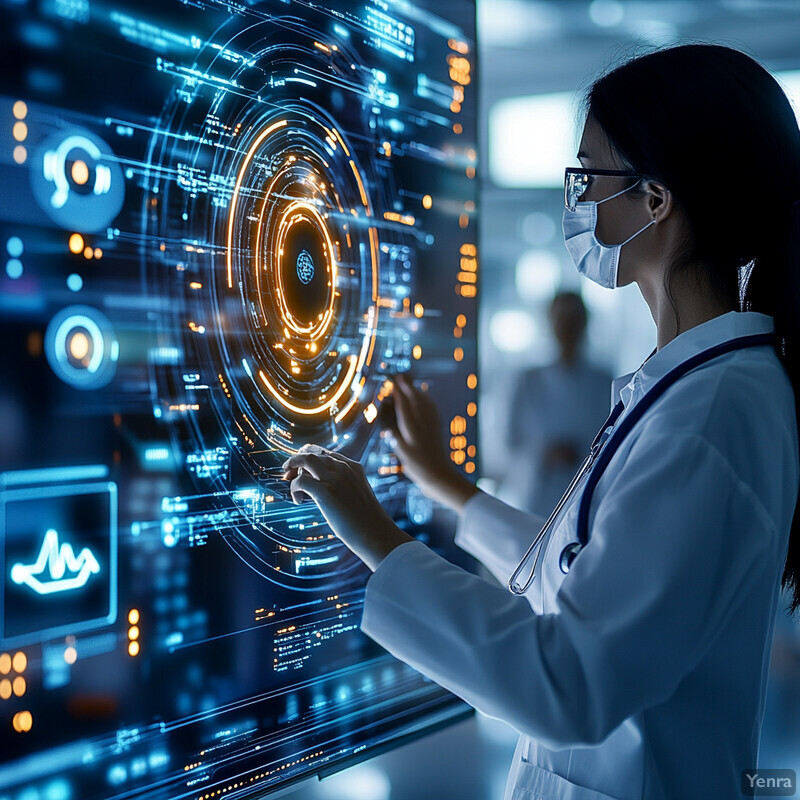
Administrative delays in treatment planning—such as manual image contouring, repeated consultations, and refining radiation beam arrangements—can postpone the start of therapy. AI-powered tools accelerate these processes, automating repetitive steps and providing rapid, data-backed recommendations. By streamlining workflow, AI helps patients begin their treatments sooner, potentially improving outcomes and reducing anxiety associated with waiting.
17. Machine Learning-Based Radiomics
Advanced feature extraction from images (radiomics) combined with AI can help classify tumor subtypes, grade aggressiveness, and provide insights for more nuanced treatment planning.
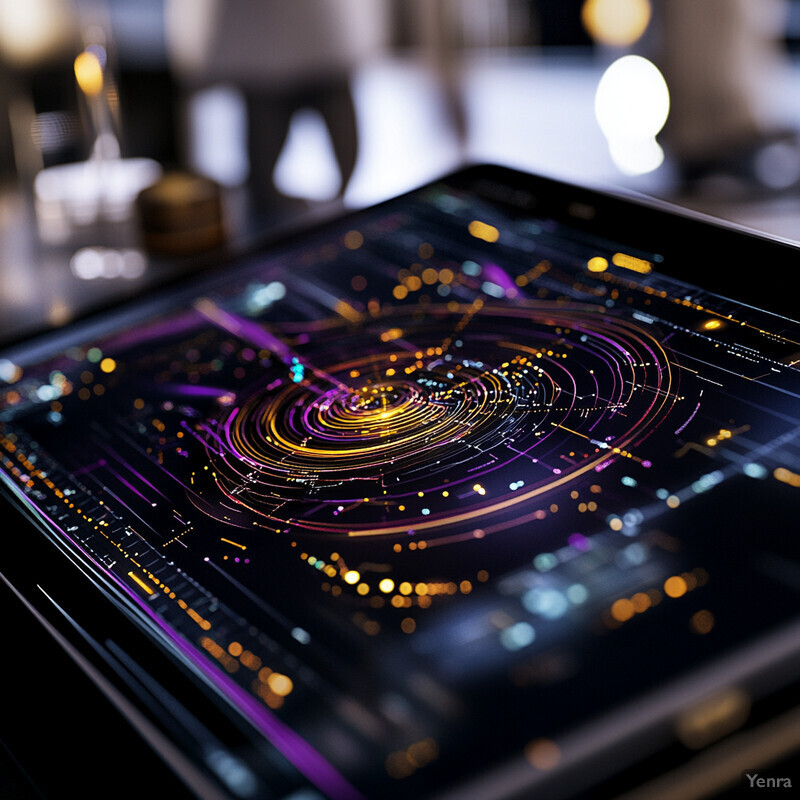
Radiomics involves extracting a large number of quantitative features from medical images, capturing subtle patterns not discernible to the naked eye. AI can analyze these features and link them to tumor phenotype, aggressiveness, and likely response to specific therapies. Integrating radiomics into treatment planning provides clinicians with deeper insights into tumor behavior, guiding more informed treatment decisions. This approach moves beyond simple anatomical imaging, turning each scan into a rich source of predictive information.
18. Continuous Learning from Outcomes
AI systems can continuously incorporate new patient outcomes, refining models over time, ensuring that future treatment plans benefit from the growing body of real-world data and experience.
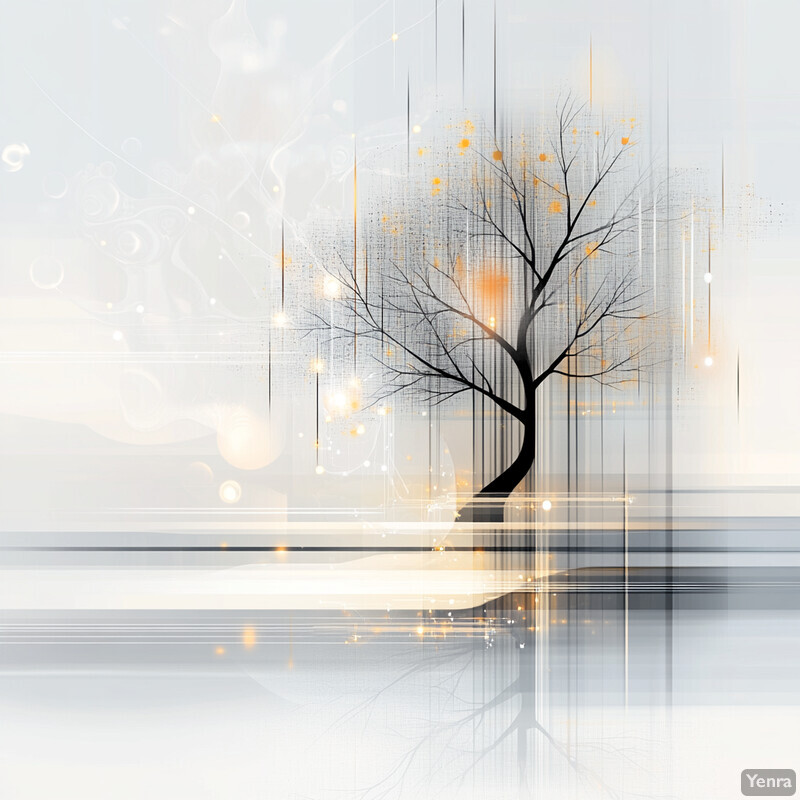
AI systems do not remain static; they evolve as they encounter new data. After a patient completes treatment, the outcome—whether successful tumor control, side effects, or recurrence—is fed back into the model. Over time, this feedback loop allows the AI to refine its predictions and recommendations. Continuous learning ensures that treatment planning benefits from the most current evidence and real-world outcomes, improving the accuracy and utility of AI tools for future patients.
19. Cost-Effective Treatment Strategies
By predicting which treatments are most likely to yield positive results, AI can potentially reduce ineffective interventions, thus trimming costs and improving resource allocation within oncology care settings.
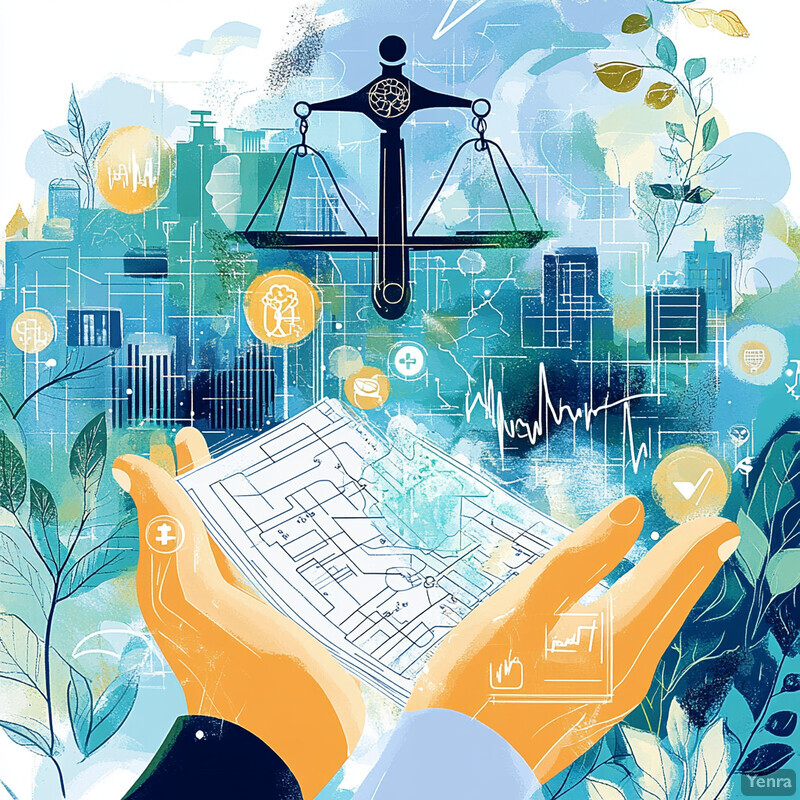
Balancing the need for advanced therapies with the realities of healthcare budgets is a persistent challenge. By predicting which treatments are more likely to yield favorable results and identifying patients unlikely to benefit from expensive options, AI can guide cost-effective decisions. Reducing the use of ineffective treatments or unnecessary diagnostic tests ultimately helps manage limited resources more efficiently. The result is a more sustainable approach that preserves the quality of care and ensures patients receive the most appropriate therapies.
20. Enhanced Communication Tools for Patients
AI-driven decision support systems can generate simplified explanations and visualizations of proposed treatment plans, helping patients understand their options and engage more fully in shared decision-making with their care team.
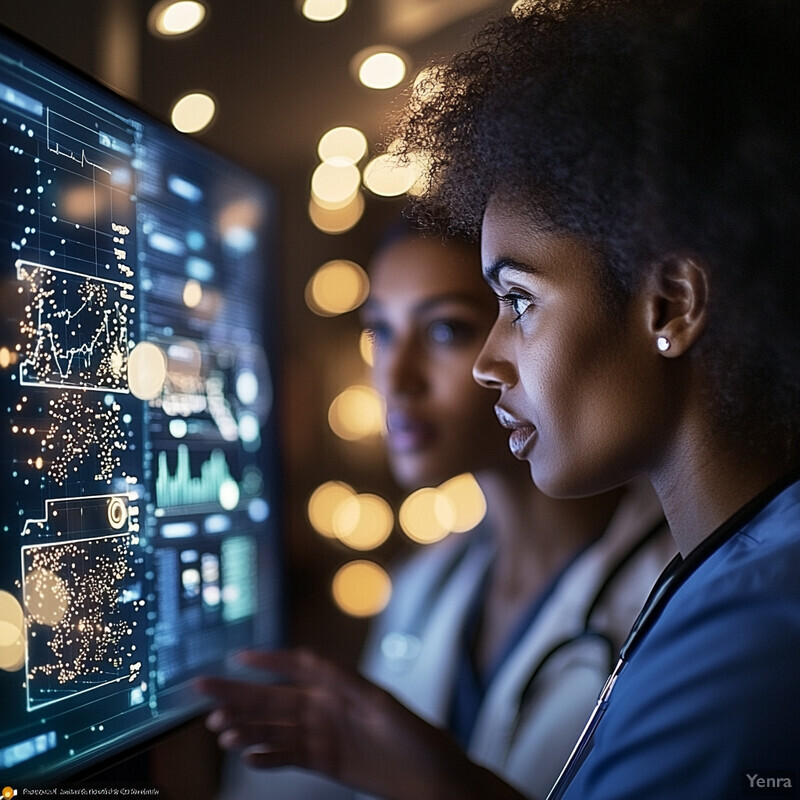
Effective treatment planning extends beyond selecting a therapy—it also involves helping patients understand their options. AI-driven decision support systems can generate patient-friendly explanations, use visualization tools, and provide evidence-based rationales for recommended treatment plans. By making complex medical information more accessible and comprehensible, these systems empower patients to engage in shared decision-making, improving satisfaction and adherence to the chosen treatment regimen.