1. High-throughput Data Analysis
AI-driven algorithms can handle massive volumes of genomic, transcriptomic, proteomic, and metabolomic data, enabling researchers to identify potential biomarkers that would be missed by traditional methods.
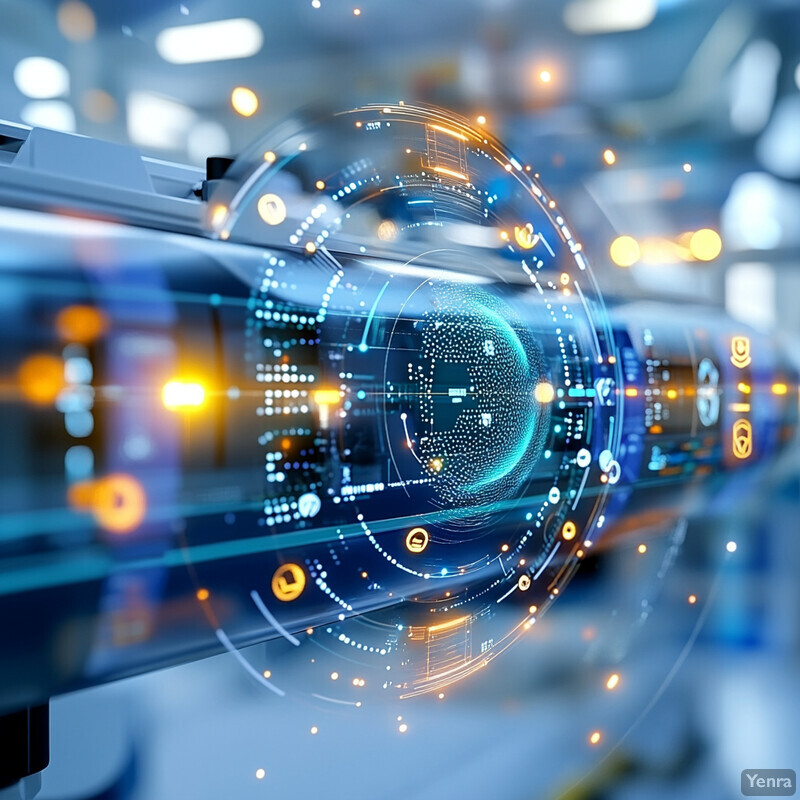
Traditional biomarker discovery often involves manually sifting through complex, multidimensional biological data to find patterns of interest. AI-driven high-throughput data analysis automates and accelerates this process by applying sophisticated machine learning algorithms to massive datasets generated from next-generation sequencing, mass spectrometry, and other omics technologies. By doing so, AI helps researchers detect subtle correlations and associations between biomarkers and disease states. This capability enables scientists to prioritize leads and focus on the most promising candidates, greatly reducing the time and labor required to move from raw data to actionable insights. Ultimately, AI-assisted high-throughput analysis can translate into faster biomarker identification, improving the efficiency and success rate of diagnostic and therapeutic discovery efforts.
2. Feature Selection in Complex Datasets
Machine learning models can efficiently sift through thousands of features, zeroing in on a smaller subset of highly predictive biomarkers for disease classification or prognosis.
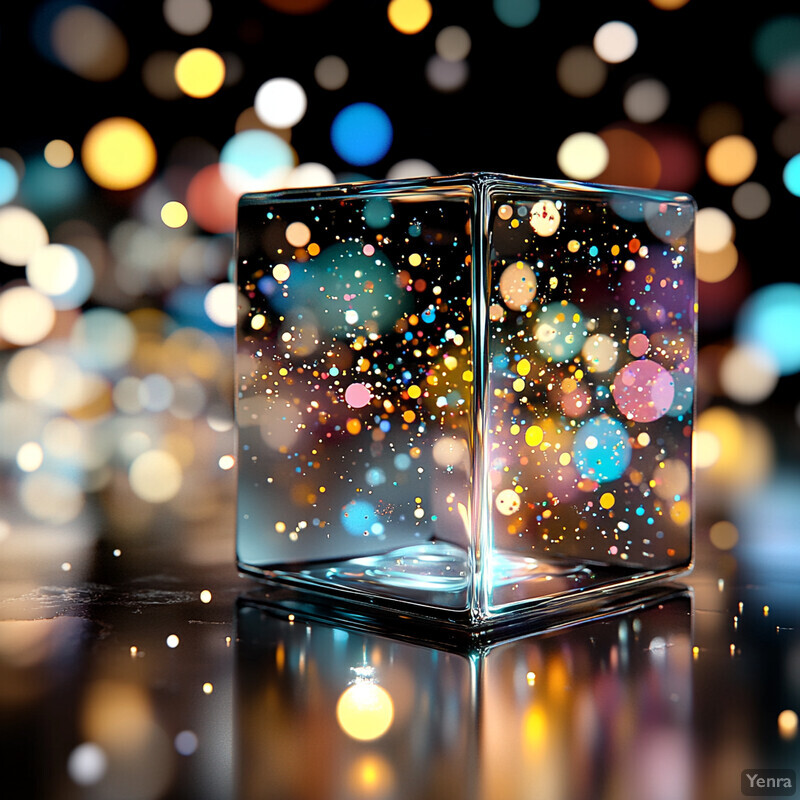
Modern biomedical research often involves extremely large and complex datasets containing thousands of potential features—genes, proteins, metabolites, clinical parameters—many of which may not be informative. Machine learning algorithms excel at feature selection, applying mathematical techniques to distinguish signal from noise. By iteratively testing and refining the relevance of each variable, AI helps researchers pinpoint a subset of features that accurately predict disease states or outcomes. This reduction in dimensionality not only makes modeling more manageable and interpretable but also saves valuable time and resources. Ultimately, this leads to a more efficient path toward robust biomarker signatures that hold diagnostic, prognostic, or therapeutic value.
3. Integration of Multi-Omics Data
AI can integrate genetic, proteomic, metabolomic, and clinical data into unified models, uncovering biomarkers that emerge only when multiple data modalities are combined.
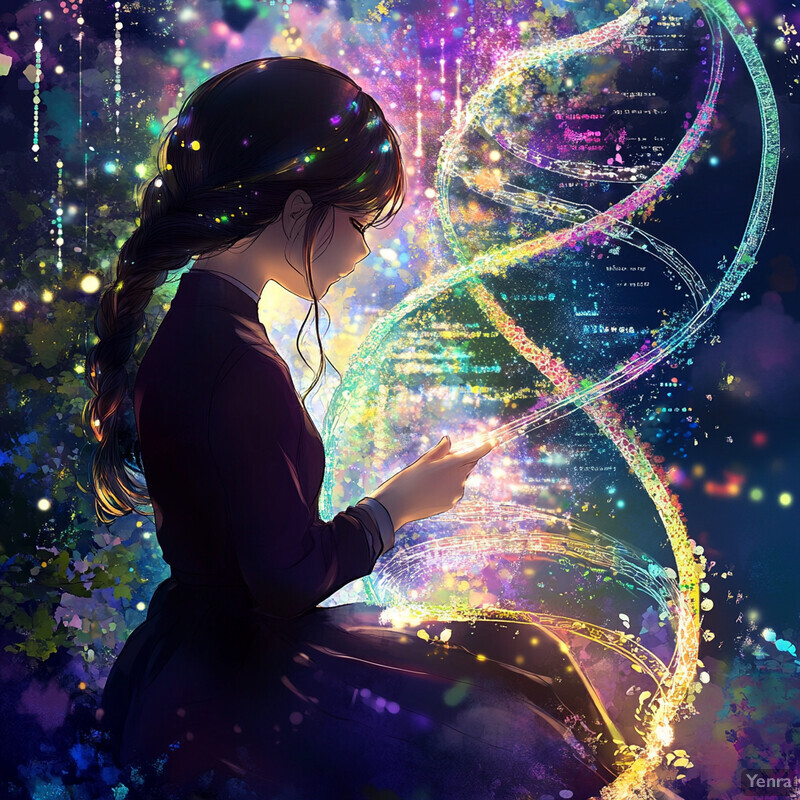
Diseases are multifactorial, arising from complex interactions at the genomic, transcriptomic, proteomic, metabolomic, and even environmental levels. AI-driven integrative modeling allows researchers to combine these diverse data streams into a single cohesive analytical framework. By identifying patterns that only emerge when multiple data types are considered simultaneously, AI can uncover biomarkers that would remain hidden if each data source were analyzed separately. Such integrative approaches promote a holistic understanding of disease mechanisms, revealing biomarkers that are both biologically meaningful and clinically actionable. As a result, multi-omics integration facilitated by AI is paving the way for precision medicine, where diagnostic tests and treatments can be tailored to an individual’s unique molecular profile.
4. Predictive Modeling of Disease Outcomes
Advanced modeling techniques, such as deep learning, can predict patient outcomes based on candidate biomarkers, helping clinicians choose the most meaningful biomarkers for diagnostics and prognostics.
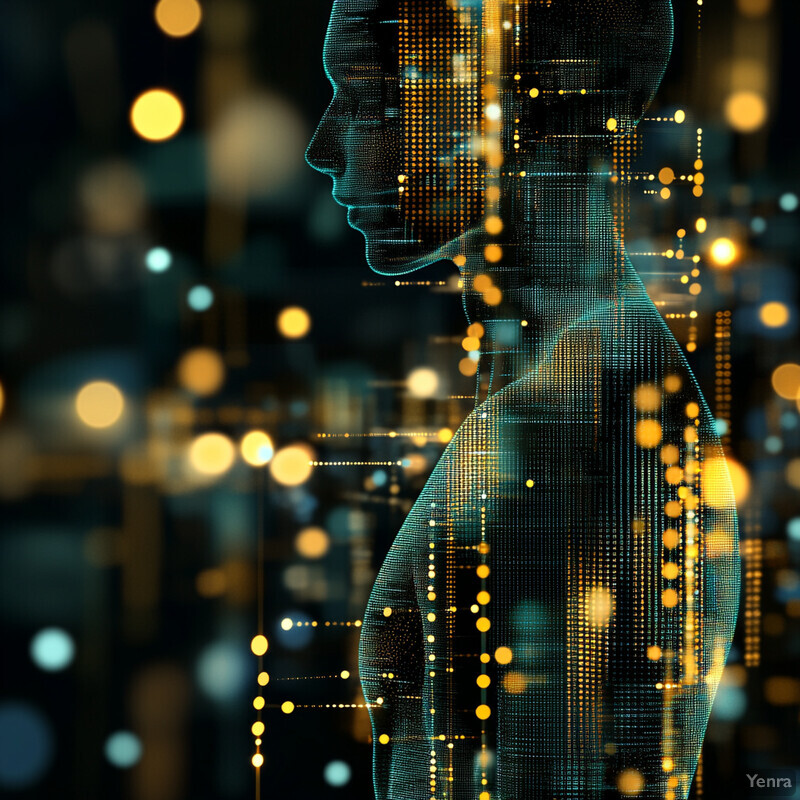
Beyond merely identifying biomarkers associated with a particular condition, AI-driven predictive models can determine how well these biomarkers forecast disease outcomes. For example, deep learning algorithms can learn complex relationships between biomarker signatures and patient trajectories, predicting disease progression, treatment responses, or the likelihood of adverse events. When validated clinically, these predictive models guide physicians in treatment decisions and patient management, ultimately improving care. By continually refining these models as new data become available, AI ensures that predictive biomarkers remain current and valuable, enabling dynamic, informed decision-making in patient care.
5. Advanced Imaging Biomarkers
Computer vision and deep learning can analyze imaging data (MRI, CT, PET) to detect subtle patterns indicative of disease, allowing the discovery of imaging biomarkers that correlate with molecular changes.
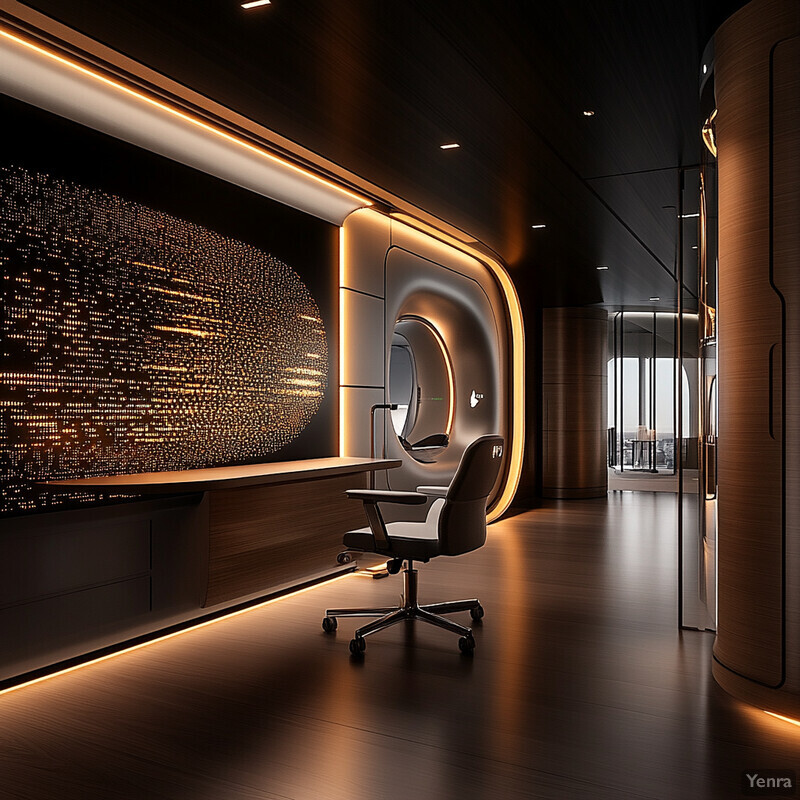
Medical imaging—MRI, CT scans, PET scans—provides a rich source of data that can reveal structural and functional abnormalities associated with diseases. AI-driven computer vision and deep learning algorithms excel at detecting subtle image features that may be invisible to the human eye. These image-based biomarkers can reflect underlying molecular changes or disease states, such as patterns in tumor vasculature or subtle tissue density shifts in neurological disorders. The discovery of imaging biomarkers through AI reduces the need for invasive procedures and complements molecular biomarker panels. As a result, radiologists and clinicians gain powerful, noninvasive tools for early detection, monitoring, and personalized treatment planning.
6. Accelerated Hypothesis Testing
AI can rapidly test numerous hypothetical biomarkers in silico, drastically reducing the time and cost associated with traditional experimental validation.
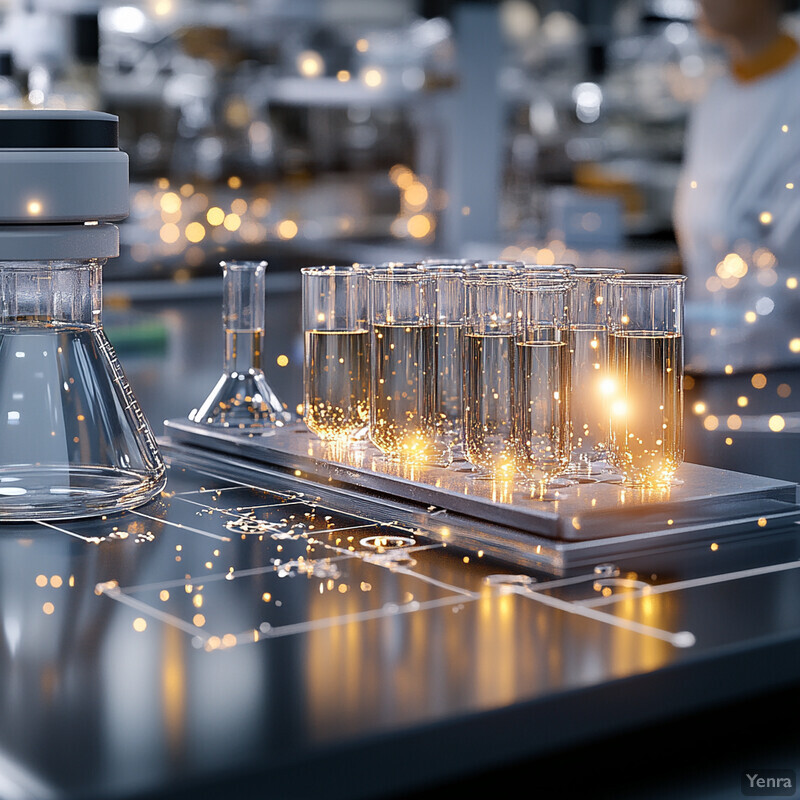
Traditional biomarker research often involves a long and costly process of hypothesizing candidate biomarkers and then validating them experimentally. AI offers a computational shortcut by enabling in silico hypothesis testing. Using machine learning, researchers can rapidly test large numbers of candidate biomarkers and refine their lists based on predictive power and reproducibility. This computational pre-screening dramatically reduces the number of fruitless laboratory experiments and clinical trials, saving time and resources. By guiding scientists toward the most promising candidates more quickly, AI-driven hypothesis testing supports a more efficient research pipeline and accelerates the path to clinical validation.
7. Biomarker Prioritization for Clinical Trials
Machine learning models can prioritize which biomarkers are most likely to be clinically relevant, streamlining patient stratification for more effective clinical trials.
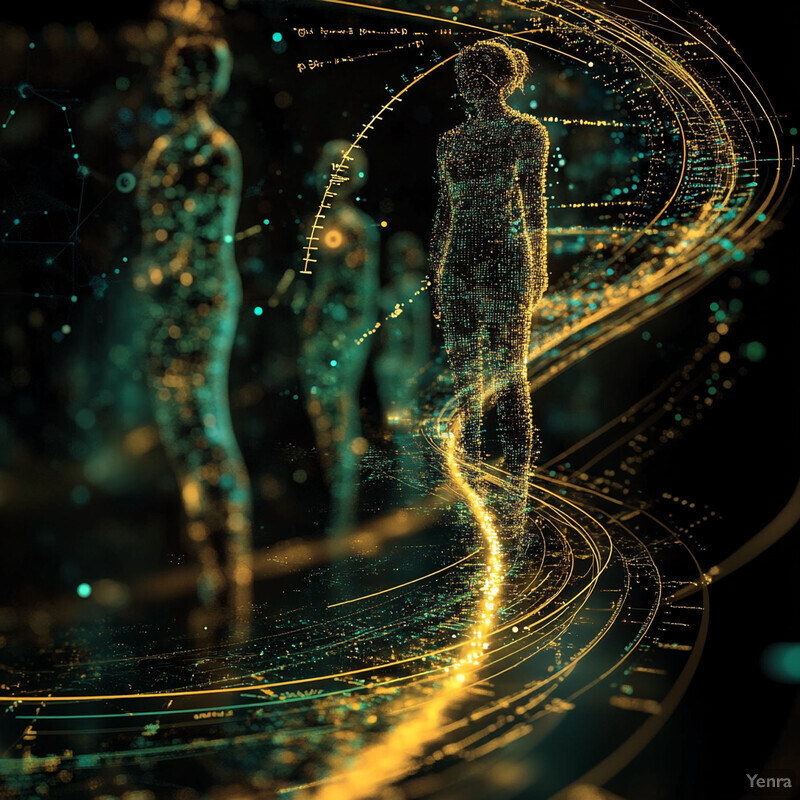
Clinical trials demand substantial investment and time. Poor biomarker selection can lead to inconclusive results, missed opportunities, or failed trials. AI helps mitigate these risks by using predictive modeling to highlight biomarkers that are most likely to yield meaningful stratification and reliable endpoints. By analyzing patient data, disease characteristics, and treatment responses, machine learning algorithms can rank biomarkers based on their clinical relevance. With a more focused set of biomarkers, researchers can design more effective trials, enroll the right patient cohorts, and ultimately increase the likelihood of a successful path to regulatory approval and clinical adoption.
8. Unbiased Pattern Recognition
Unlike human analysis, AI approaches do not rely on preexisting hypotheses, enabling the unbiased discovery of novel biomarkers that might not fit established disease models.
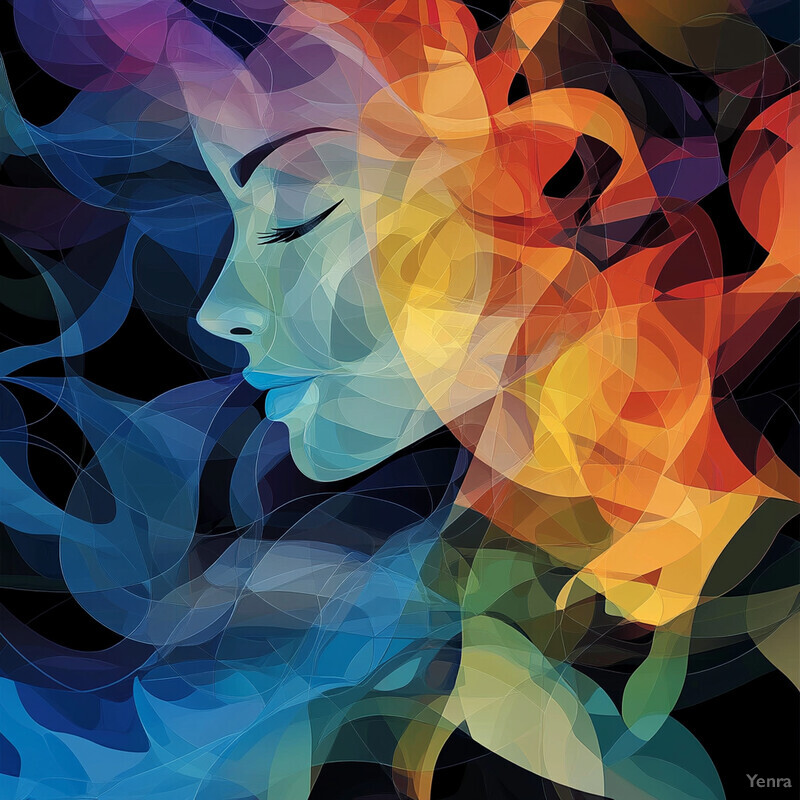
Human-driven research often depends on preconceived notions and established hypotheses. AI, however, excels at recognizing patterns in a data-agnostic manner. By applying unsupervised learning algorithms such as clustering or dimensionality reduction, AI can uncover hidden relationships and subgroups within patient cohorts without relying on prior hypotheses. This unbiased approach frequently leads to the discovery of novel biomarkers that defy traditional disease models and assumptions. By challenging conventional wisdom, AI-driven unbiased pattern recognition can open new avenues for understanding diseases and identifying previously overlooked molecular indicators that could guide novel diagnostics or therapies.
9. Real-time Analysis of Wearable Device Data
AI can continuously analyze data from wearable sensors to identify early changes in physiology, serving as dynamic biomarkers for disease onset or treatment response.
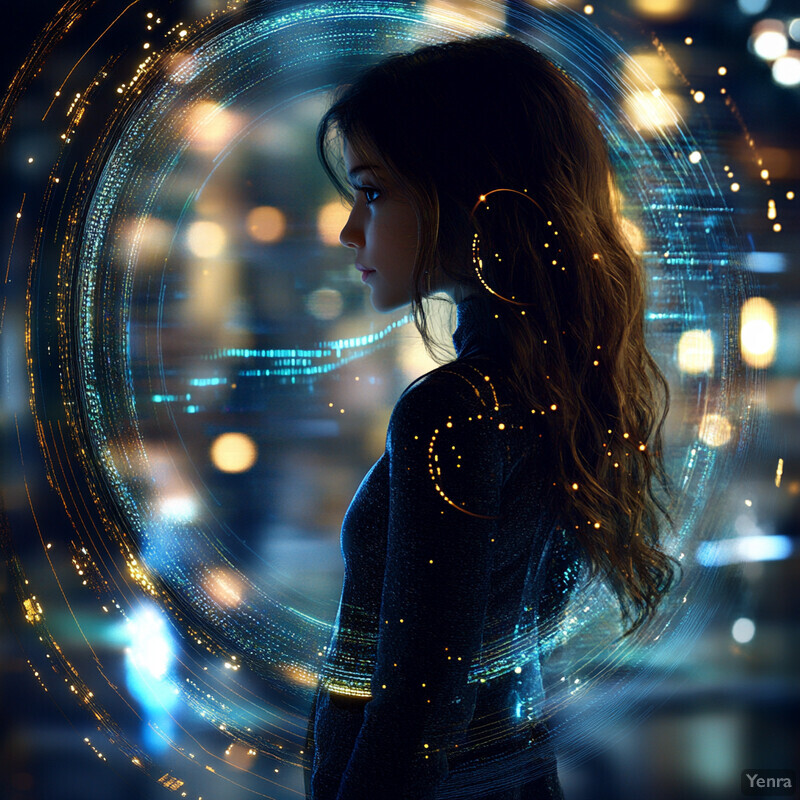
Advances in wearable technology have enabled continuous, real-time monitoring of physiological parameters—heart rate, glucose levels, sleep patterns, or activity counts—collected passively from patients. AI can process these massive streams of data to identify early shifts in health that serve as dynamic biomarkers. For instance, subtle changes in heart rate variability might signal impending cardiac issues, while alterations in movement patterns could indicate neurodegenerative disease progression. This real-time biomarker discovery offers the potential for earlier interventions and more personalized healthcare management. As more patients adopt wearable devices, the role of AI in discovering clinically actionable biomarkers from these data streams will only increase.
10. Population-Level Biomarker Identification
AI can parse data from large patient cohorts, identifying population-level biomarkers that remain stable and predictive across diverse demographics and disease stages.
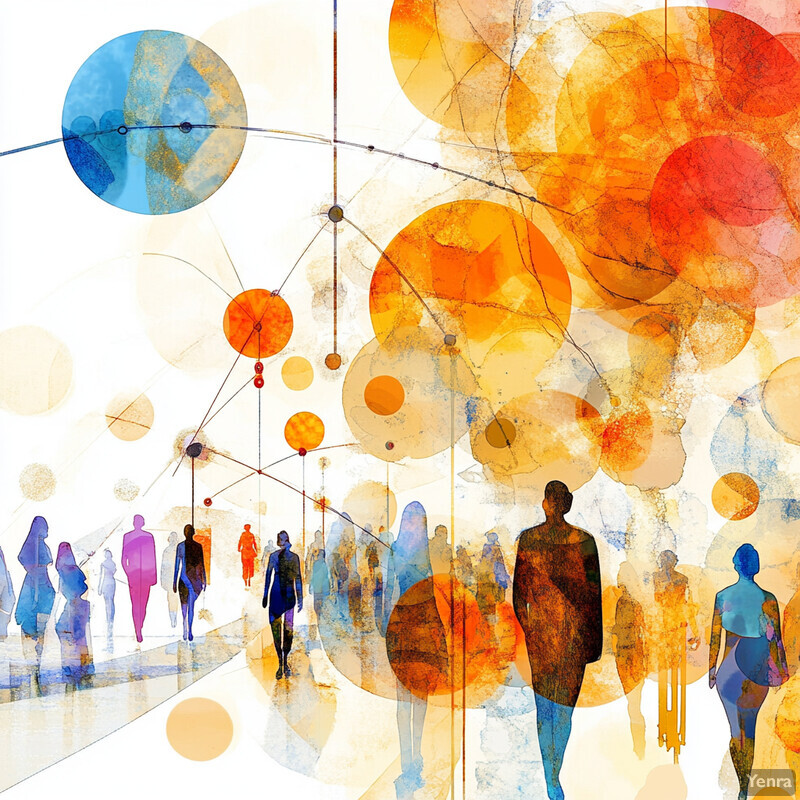
Diseases manifest differently across ages, ethnicities, and environmental contexts, and no single biomarker may hold universal significance. AI excels at conducting large-scale population-level analyses, integrating diverse patient cohorts drawn from electronic health records, biobanks, and international consortia. By detecting patterns that are consistent across broad populations, AI can highlight robust biomarkers that retain their predictive value in real-world clinical settings. This approach ensures that biomarkers are not only scientifically sound but also generalizable, improving their utility in guiding healthcare decisions across different demographics, healthcare systems, and geographic regions.
11. Rare Disease Biomarker Discovery
Machine learning tools can highlight subtle differences in patients with rare conditions, aiding the discovery of biomarkers that would be difficult to find with limited patient samples.
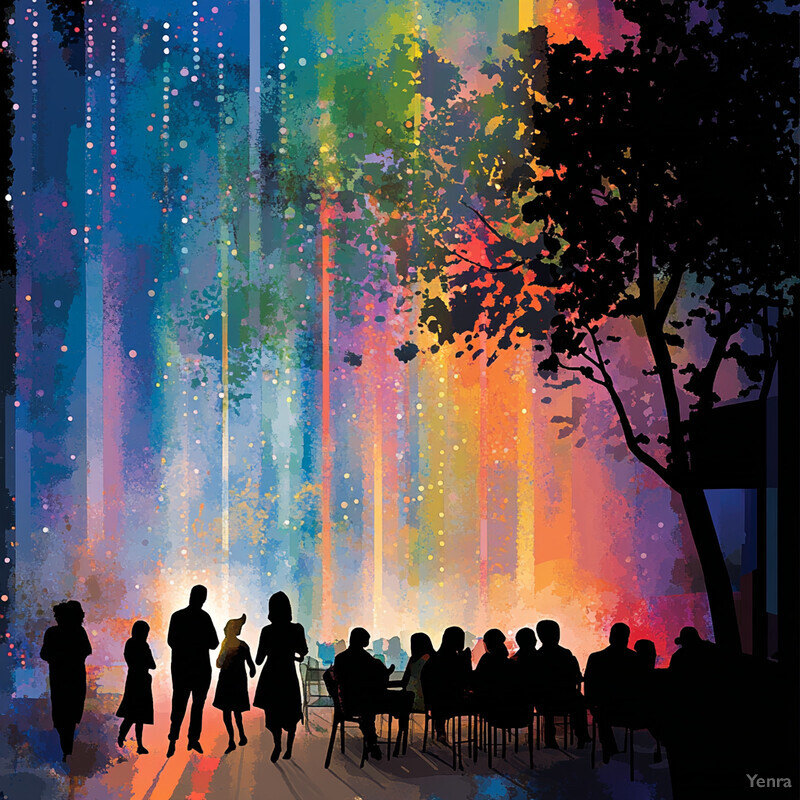
Rare diseases pose a unique challenge for biomarker discovery due to limited patient samples and heterogeneous presentations. Traditional statistical approaches often fail to detect meaningful patterns under these constraints. AI algorithms, however, can learn from sparse and noisy data, applying transfer learning and advanced feature selection techniques to pinpoint subtle biomarkers indicative of these rare conditions. By shining a light on these elusive signatures, AI empowers clinicians and researchers to diagnose rare diseases earlier, improve patient stratification for research studies, and tailor treatments that significantly enhance patient outcomes in a previously underserved population.
12. Predictive Early Intervention Markers
By identifying biomarkers that appear long before clinical symptoms manifest, AI can guide clinicians to intervene earlier, improving patient outcomes and prevention strategies.
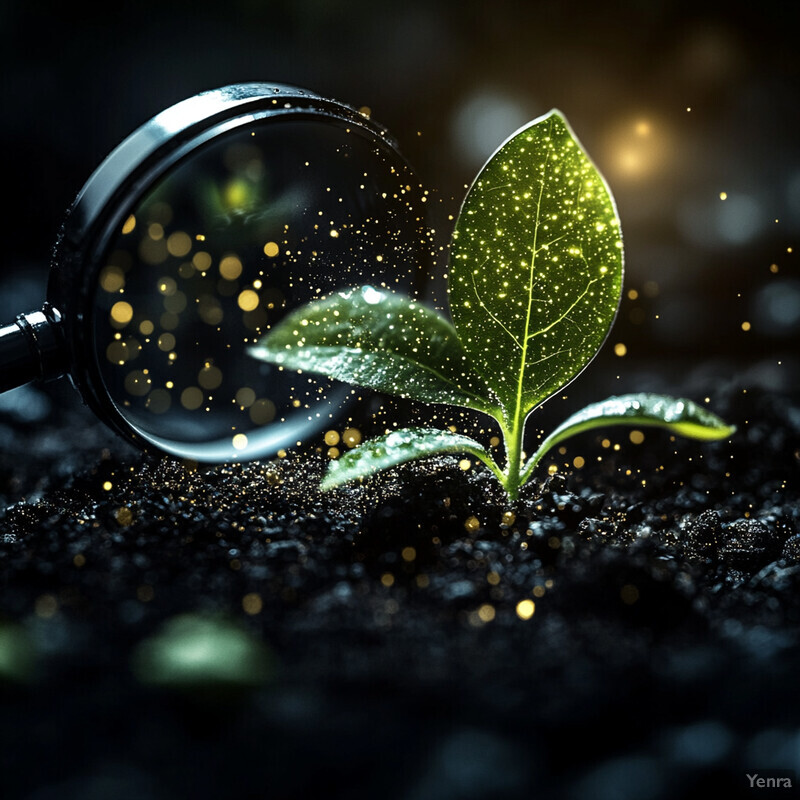
One of the ultimate goals in healthcare is to detect disease long before it causes irreparable harm. AI plays a crucial role by discovering biomarkers that present at the earliest stages of a condition, well before traditional diagnostic criteria are met. For instance, machine learning models can identify gene expression patterns or protein shifts that foreshadow disease onset. With these early intervention biomarkers, clinicians can proactively manage patients at high risk, potentially halting or delaying disease progression. This approach has profound implications for public health, allowing for timely prevention strategies, improved quality of life, and reduced long-term healthcare costs.
13. Robust Stratification of Disease Subtypes
AI-driven clustering and classification methods can reveal disease subtypes, each associated with distinct biomarker profiles, informing personalized medicine approaches.
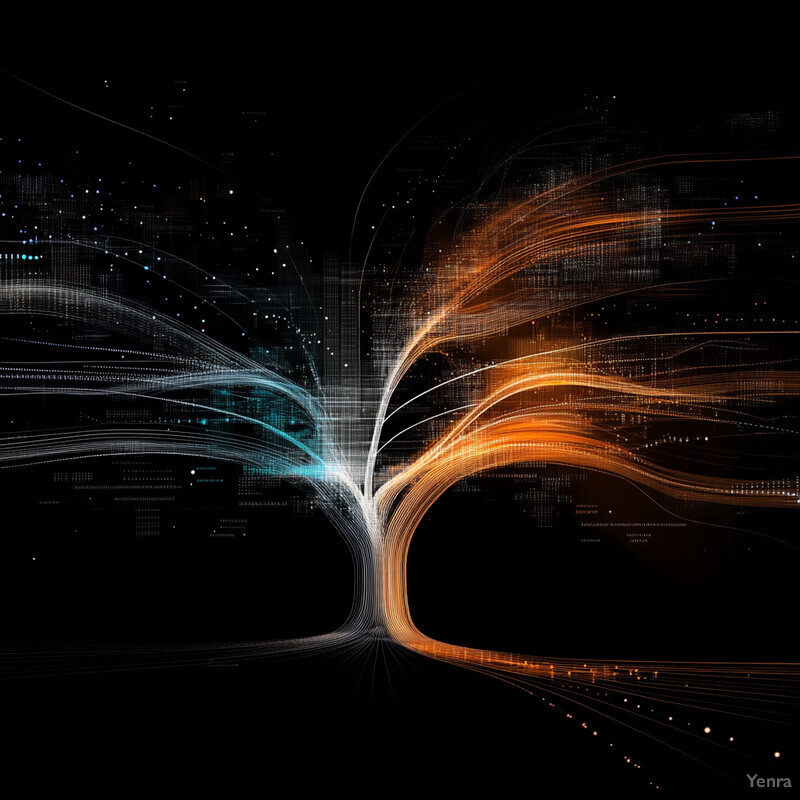
Many diseases are not monolithic but rather consist of multiple subtypes with distinct underlying biology and clinical courses. AI-driven clustering and classification tools can sort patients into meaningful subgroups based on molecular or clinical biomarkers. By uncovering these subtypes, clinicians gain a more accurate understanding of prognosis, identify differential responses to treatments, and personalize therapies accordingly. For researchers, this stratification guides biomarker discovery by focusing on the molecular signatures unique to each subtype. Over time, refining the molecular taxonomy of diseases paves the way for more targeted diagnostic tests and more effective, personalized interventions.
14. Reduction of False Positives and Negatives
Techniques like ensemble learning and regularization help refine biomarker sets, minimizing the risk of selecting non-specific or noisy indicators.
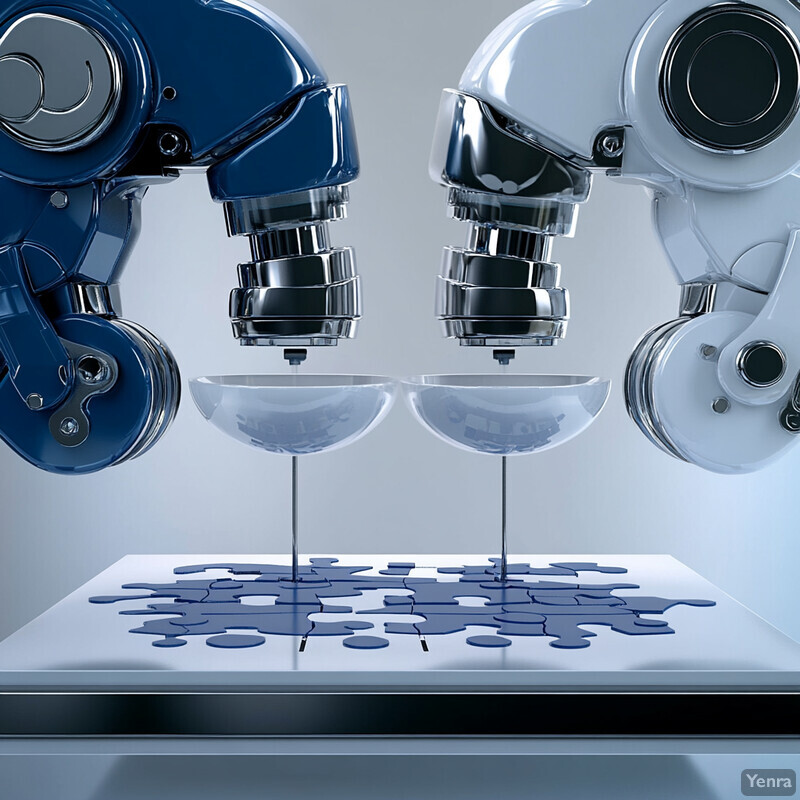
Biomarker discovery is fraught with the risk of choosing indicators that are either too nonspecific (false positives) or miss crucial disease signals (false negatives). AI mitigates these issues by employing robust statistical methods, ensemble learning, and cross-validation strategies. These approaches help ensure that the selected biomarkers are both sensitive and specific, yielding a high level of diagnostic or prognostic accuracy. By continuously refining models against new data, AI-driven tools can maintain and improve performance over time. This rigorous approach to validation boosts clinical confidence and encourages the successful translation of biomarker discoveries into routine practice.
15. Identification of Response Biomarkers for Therapies
AI can correlate specific biomarker patterns with patient responses to certain drugs, guiding the development of targeted therapies and companion diagnostics.
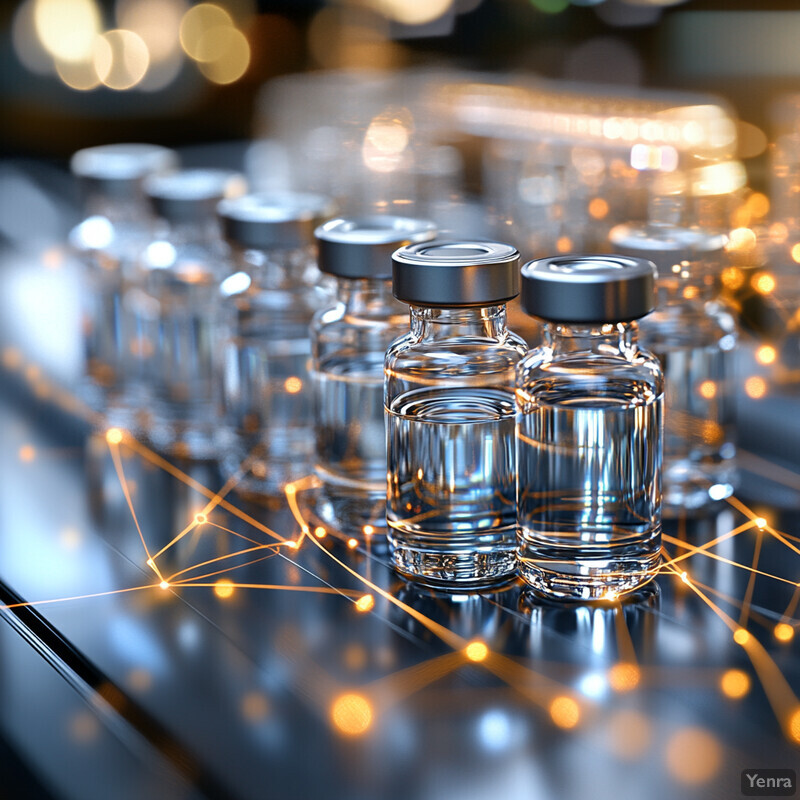
Not all patients respond equally to a given treatment. AI can help identify biomarkers that predict which patients will benefit from specific therapies and which may suffer adverse effects or fail to improve. By correlating molecular signatures, clinical parameters, and treatment outcomes, machine learning models reveal predictive biomarkers of therapeutic response. Such insights guide personalized treatment plans, reduce trial-and-error prescribing, and increase the success rate of targeted therapies. As a result, patients receive safer and more effective treatments, while healthcare systems save resources by avoiding ineffective interventions.
16. Epigenetic Biomarker Discovery
By analyzing methylation patterns and histone modifications with machine learning, researchers can identify epigenetic biomarkers that may serve as early disease indicators or therapeutic targets.
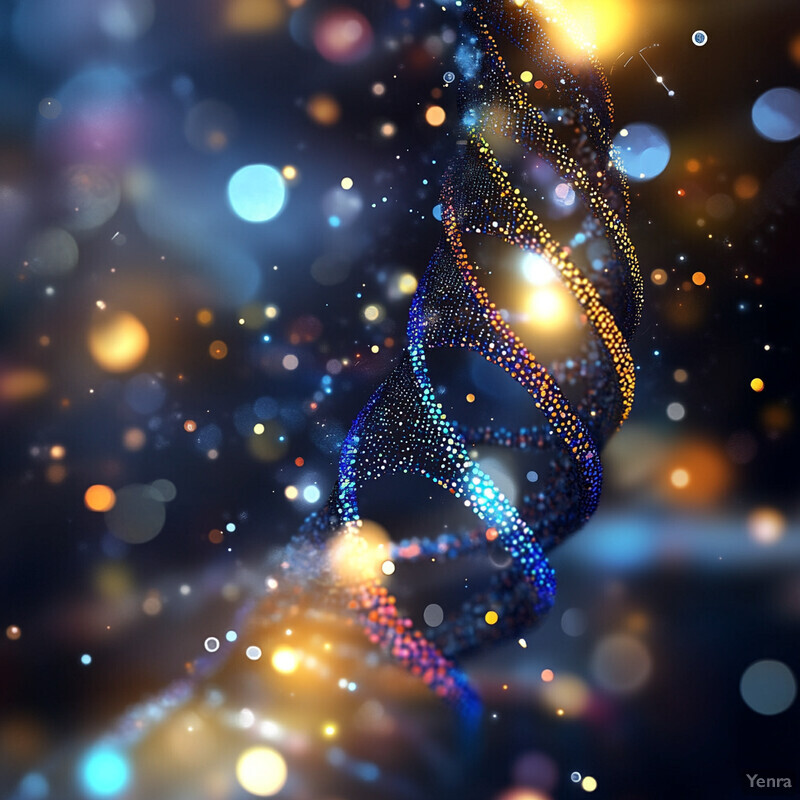
Epigenetic changes, such as DNA methylation patterns and histone modifications, play a pivotal role in disease initiation and progression. However, the complexity and subtlety of these changes make them difficult to interpret using conventional methods. AI algorithms can process these intricate datasets and highlight patterns associated with disease states or therapeutic responses. By discovering epigenetic biomarkers, researchers gain insights into the regulatory mechanisms underlying pathology. These biomarkers can lead to earlier diagnoses, refined prognostics, and even new targets for therapies that modulate epigenetic states. This dimension of biomarker discovery thus enhances our understanding of disease at a regulatory level.
17. Natural Language Processing for Literature Mining
NLP models can sift through scientific publications and clinical notes to identify candidate biomarkers supported by existing evidence, accelerating the research process.
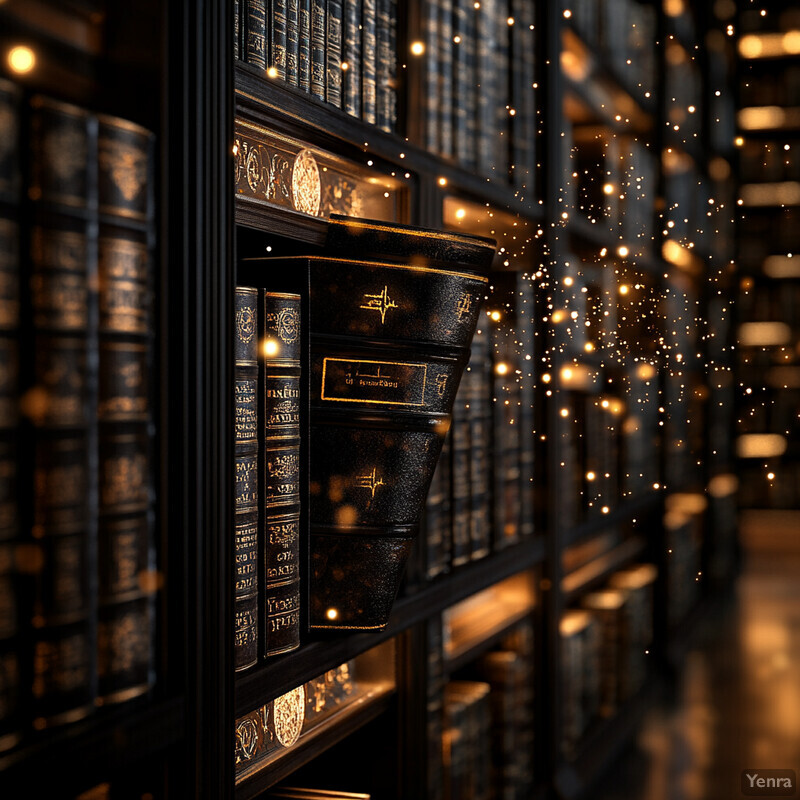
The volume of biomedical literature is growing exponentially, making it difficult for researchers to stay current or systematically identify all the evidence supporting certain biomarkers. NLP (Natural Language Processing) models, powered by AI, can quickly parse and summarize scientific publications, patents, clinical trial reports, and electronic health records. They can identify mentions of candidate biomarkers, synthesize existing knowledge, and highlight emerging trends. By using NLP to mine the literature, researchers ensure that no valuable piece of evidence is overlooked. This accelerates the initial phases of biomarker discovery and fosters a more robust and evidence-driven approach to research.
18. Automated Quality Control in Data Collection
AI systems can detect and correct data anomalies or batch effects, ensuring cleaner data sets and more reliable biomarker discovery.
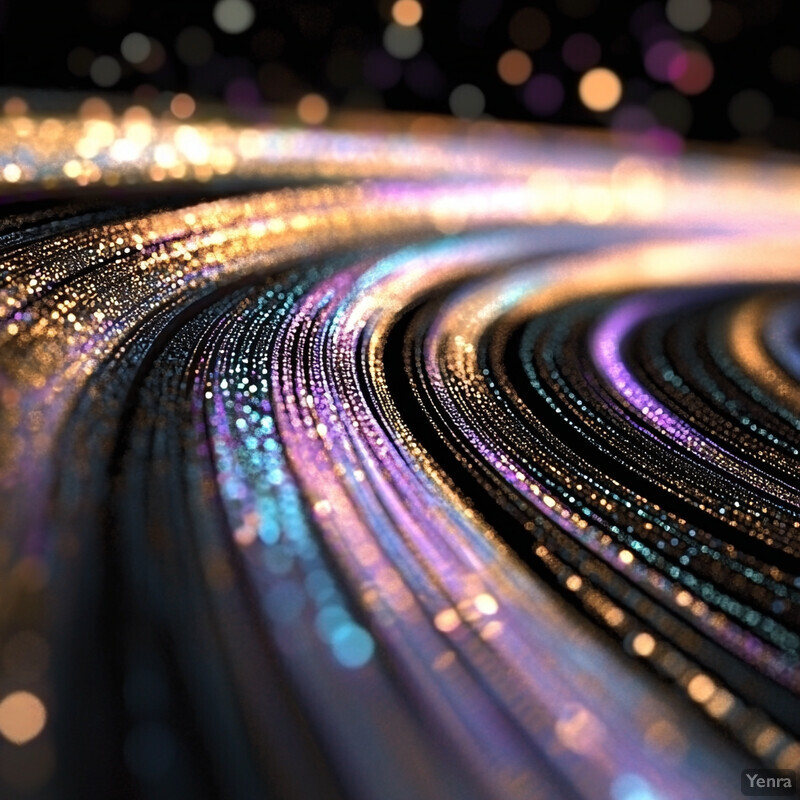
AI does not only shine during data analysis, but it also improves data quality upfront. Machine learning models can detect anomalies, inconsistencies, and outliers in experimental or clinical datasets. Automated quality control ensures that noisy data do not mislead downstream analyses, thereby increasing the likelihood that discovered biomarkers are truly meaningful rather than artifacts of poor data. For example, AI might flag inconsistencies in sample labeling or detect batch effects in genomic data. By enhancing data integrity, these automated checks contribute to more reliable and reproducible research outcomes, ultimately strengthening the validity of identified biomarkers.
19. Longitudinal Data Analysis
AI can track changes in biomarker levels over time within individual patients, helping distinguish between transient variations and stable disease-associated patterns.
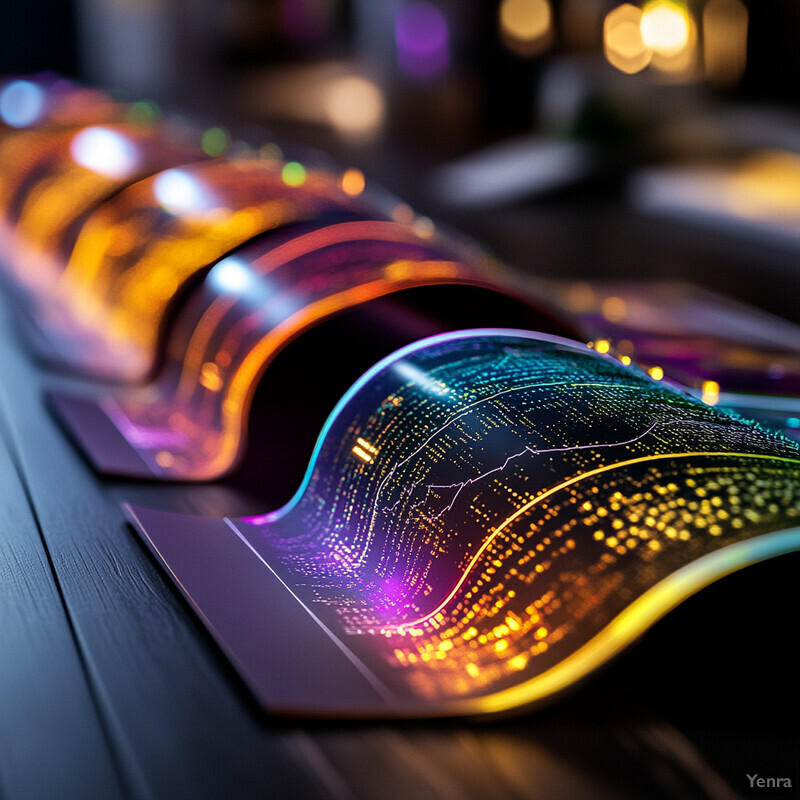
Diseases progress over time, and static snapshots rarely tell the full story. AI-driven longitudinal analysis can track changes in molecular biomarkers across various time points in patient cohorts. By detecting how these biomarkers evolve, machine learning models distinguish transient fluctuations from stable disease-associated patterns. Such insights are crucial for understanding disease trajectories, determining optimal timing for intervention, and refining prognostic models. Longitudinal biomarker profiles also help researchers assess treatment efficacy over the patient’s journey, enabling dynamic adjustments to therapeutic strategies that improve patient outcomes.
20. Personalized Biomarker Panels
Machine learning can tailor biomarker panels to individuals based on their unique genetic, environmental, and lifestyle factors, paving the way for highly personalized diagnostics and treatments.
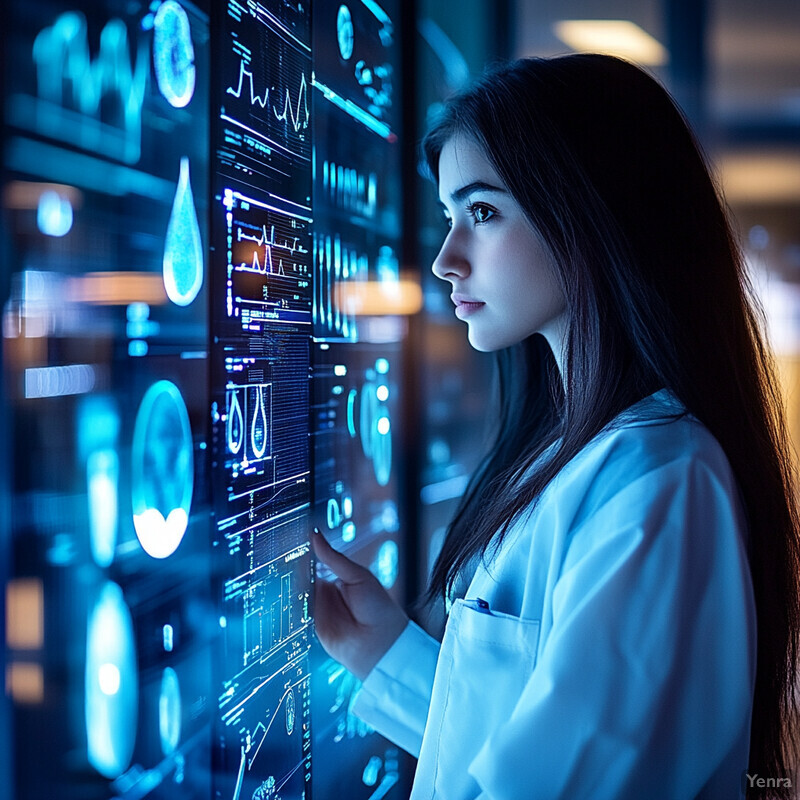
Every patient is unique, influenced by a combination of genetic background, lifestyle, and environmental factors. AI’s ability to learn from diverse and large datasets makes it possible to tailor biomarker panels to individual patients. Rather than relying on a one-size-fits-all test, machine learning models can select the biomarkers most relevant to a specific patient’s condition and risk factors. This personalization increases diagnostic accuracy, ensures that preventive measures are truly preventive, and guides treatments that align best with the patient’s unique profile. The result is a more individualized, data-driven approach to healthcare, one that holds great promise for improved patient satisfaction, better clinical outcomes, and more efficient use of healthcare resources.