1. Early Outbreak Detection
Transformer-based language models that continuously mine social-media and news text streams can surface weak but rising signals of illness several weeks before patients appear in formal case-reporting systems, giving public-health teams time to deploy targeted testing, advisories, or stockpiles.
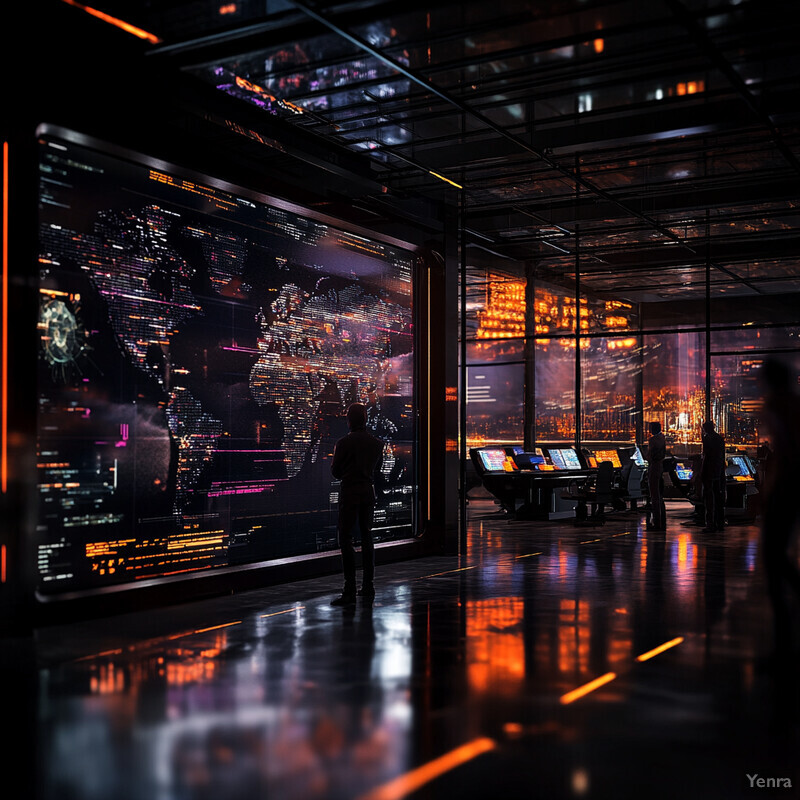
In a 2024 study that ingested 5.3 million Welsh tweets and filtered them with BERTweet and TimeLMs, exceedance analysis of first-person symptom posts triggered influenza-like-illness alerts four weeks earlier than the region’s general-practice consultation data, demonstrating the lead-time advantage of language-model surveillance.
2. Pathogen Surveillance and Genomics
Machine-learning–driven phylogenetic pipelines such as UShER + matOptimize can re-analyze tens of millions of pathogen genomes in a single run, letting analysts watch new, potentially dangerous mutations rise in near-real time instead of waiting days for traditional workflows.
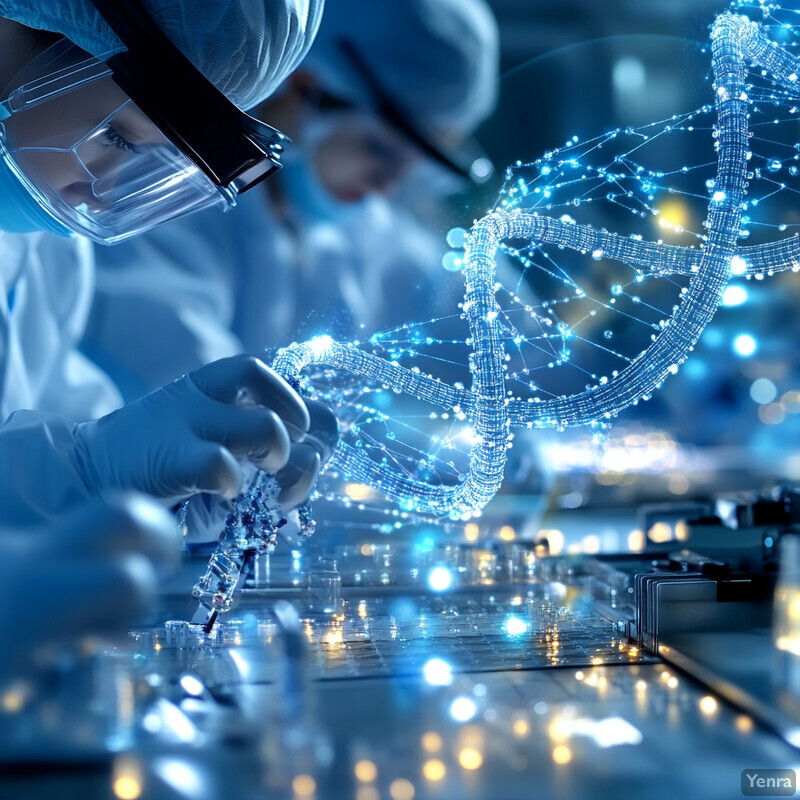
Kramer et al. (2023) demonstrated that their parsimony-based workflow refreshed a global SARS-CoV-2 tree containing more than 14 million genomes and did so thousands of times faster than maximum-likelihood methods—fast enough to update the tree every day and immediately spotlight transmissibility- or resistance-enhancing mutations.
3. Real-Time Risk Mapping
AI-driven real-time risk-mapping dashboards knit together streaming feeds—hospital admissions, confirmed cases, anonymized mobility traces, wastewater clues, air-quality and climate sensors—then translate the torrent into neighborhood-scale heat maps that refresh every few minutes. Machine-learning models continuously re-weight each signal, so when mobility surges after a festival or particulate levels spike during a wildfire, the map’s color gradients shift automatically, flagging emergent micro-hot-spots before they appear in traditional reports. Decision-makers can zoom from a city view down to specific ZIP codes, compare “what-if” policy scenarios in-panel, and push tailored nudges—pop-up vaccination clinics, indoor-mask advisories, school schedule tweaks—within the same hourly cycle. Hospitals and public-health agencies thus move from weekly sit-reps to an agile “observe–orient–decide–act” loop measured in hours, trimming misallocated supplies and reducing the window in which an outbreak can silently accelerate.
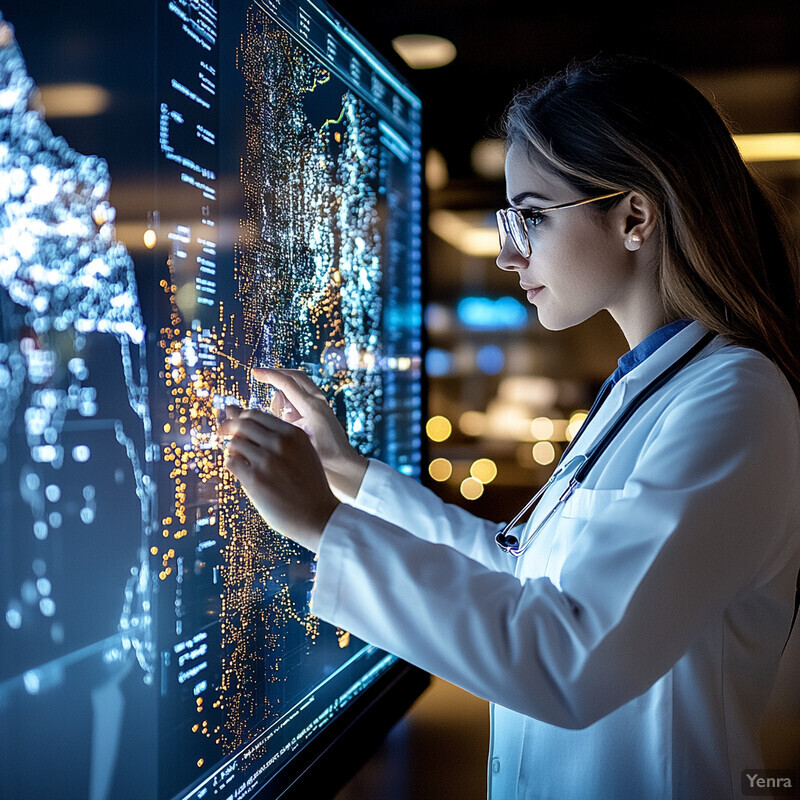
Recent studies demonstrate the concrete gains of this approach:
Hospital surge planning. A Massachusetts group fused cellphone mobility, test-positivity and census commuting patterns into a recursive model that produced accurate 21-day admission forecasts for five individual hospitals, giving administrators two extra weeks to stage staff and ventilators compared with no-mobility baselines.
Vector-borne disease hot-spots. In Brazil, a 2025 deep-learning framework that layers climate, historical dengue counts and human-mobility–adjusted imports into an LSTM reduced MAE and MAPE versus climate-only models and achieved the highest sensitivity and F1 scores for weekly outbreak classification across every test city—evidence that mobility-aware dashboards can flag looming spikes before mosquito indices turn critical.
Graph-learning risk tiers. The 2024 PANDORA system treats provinces as nodes in a transportation network and blends transportation frequency, medical capacity, climate and economic data in a deep-graph architecture; it outperformed standard time-series baselines on accuracy and convergence speed when forecasting city-level COVID-19 risk levels, underscoring how graph AI can power fine-grained risk maps that self-update as travel patterns evolve.
Together, these results show that when diverse, high-velocity data streams are stitched together by modern AI, real-time risk maps can forecast clinical loads, anticipate community transmission, and direct countermeasures with a spatial precision that manual dashboards cannot match.
4. Drug Discovery and Repurposing
Deep-learning pipelines now probe billions of molecular configurations and thousands of already-approved drugs in silico, ranking hits against viral, bacterial, and human targets in hours rather than the months or years demanded by wet-lab high-throughput screens. Graph neural networks such as TxGNN reason over vast biomedical knowledge graphs to propose “zero-shot” drug–disease matches for 17,000 conditions, complete with mechanistic rationales clinicians can audit. Generative chemistry engines then refine top candidates, predicting binding affinity, ADMET liabilities, and synthesizability before a single flask is lifted. When promising signals emerge, the same models can pivot to repurposing—triaging decades-old compounds whose safety dossiers shorten regulatory timelines. The result is a compressed discovery loop that moves from target insight to first-in-human trials in half the conventional time while dramatically widening the therapeutic search space.
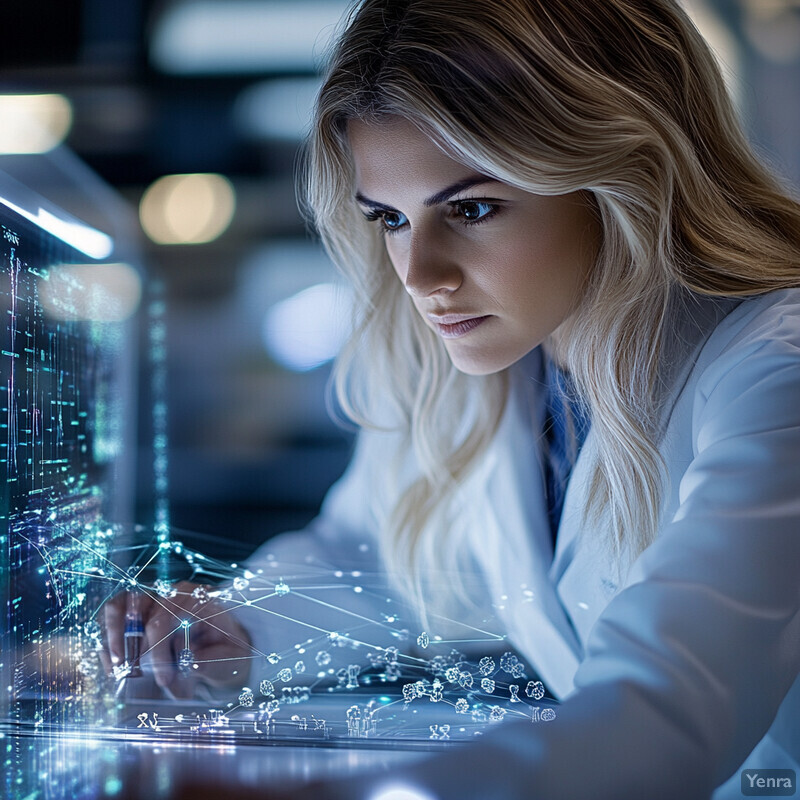
Antibiotic discovery. A deep-learning screen of just 7,500 molecules against Acinetobacter baumannii pinpointed a novel compound, abaucin, with a unique LolE-targeted mechanism; the full cycle from model training to in-vivo mouse efficacy took under 18 months.
Repurposing precision. TxGNN’s zero-shot framework improved indication-prediction accuracy by 49.2 percent and contraindication prediction by 35.1 percent over eight prior methods, demonstrating that AI can systematically surface viable second uses for drugs that clinicians have never tried off-label.
Clinical speed-up. Insilico Medicine’s AI-designed small-molecule ISM001-055 progressed from target identification to a positive Phase 2a read-out for idiopathic pulmonary fibrosis in just five years—roughly half the industry median—after the platform generated and optimized the scaffold in only 18 months.
5. Vaccine Design Acceleration
Protein-folding and immunoinformatics AIs now work in tight relay. AlphaFold 3 resolves antigen structures—and their interactions with antibodies or small molecules—with accuracy that eclipses specialized docking tools, revealing conformationally rigid surface patches that make reliable vaccine targets. Deep-learning epitope engines such as MUNIS layer those structural maps onto 651 k experimentally verified HLA-I ligands spanning 205 alleles, scoring each patch for binding strength and predicted immunogenicity across almost the entire global HLA spectrum. Generative design models like RFdiffusion then scaffold thousands of candidate immunogens around the top-ranked motifs, discarding unstable folds in silico before any wet-lab work. The once-serial pipeline—structure determination, epitope mapping, population-coverage analysis, scaffold engineering—now runs end-to-end on GPUs, letting teams advance only the most stable, broadly protective constructs from screen to animal study in weeks instead of months.
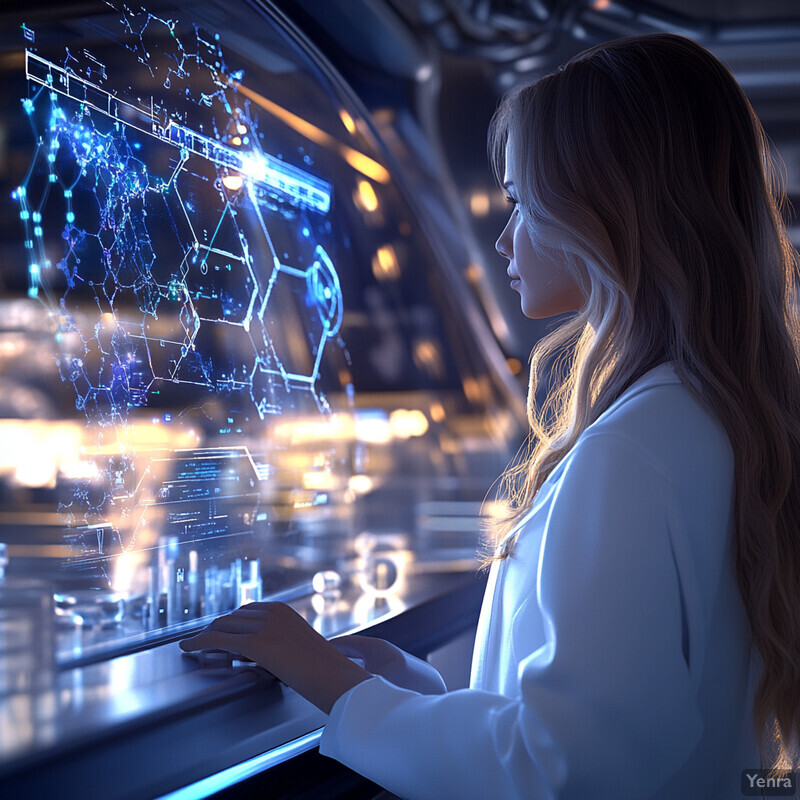
AlphaFold 3’s benchmark on >1,000 complexes showed markedly higher antibody–antigen interface accuracy than both classical docking software and AlphaFold-Multimer v2.3, establishing a new ceiling for rational immunogen selection. The 2025 MUNIS study trained on 651,237 ligands across 205 distinct HLA-I alleles and achieved a median average-precision score of 0.952—cutting presentation-prediction error by 21 percent relative to the best NetMHCpan and MHCflurry baselines—thereby enabling automated epitope sets that cover diverse populations with minimal wet-lab screening. RFdiffusion’s generative pipeline produced a de-novo influenza-haemagglutinin binder whose cryo-EM structure was “nearly identical” to the in-silico model, demonstrating atom-level fidelity for vaccine-grade immunogens and validating AI-first design loops. Complementing these advances, the 2024 AlphaEpi framework—built on AlphaFold 3 outputs—outperformed prior B-cell-epitope predictors on every benchmark set, underscoring how structure-aware graph neural networks further sharpen candidate ranking for humoral vaccines.
6. Behavioral-Compliance Modeling
Modern behavioral-compliance modeling turns once-murky human factors—mask wearing, distancing, and willingness to vaccinate—into quantifiable inputs that update epidemic forecasts in near-real time. Deep-learning pipelines fuse computer-vision counts of face-coverings from street cameras, anonymized mobility traces that reveal distancing lapses, and continuously refreshed vaccine-uptake logs. When these behavioral streams are layered onto mechanistic or LSTM-style predictors, forecast error drops sharply and “what-if” dashboards can show how a 5-point dip in masking in one ZIP code will ripple through hospital demand two weeks later. Public-health agencies then use the same models to micro-target messaging: pushing pop-up alerts or localized vaccine drives only where predicted non-compliance threatens to tip Rₑ above 1, instead of issuing broad, fatiguing mandates. The net effect is a faster, behavior-aware feedback loop that aligns interventions with the communities that need them most.
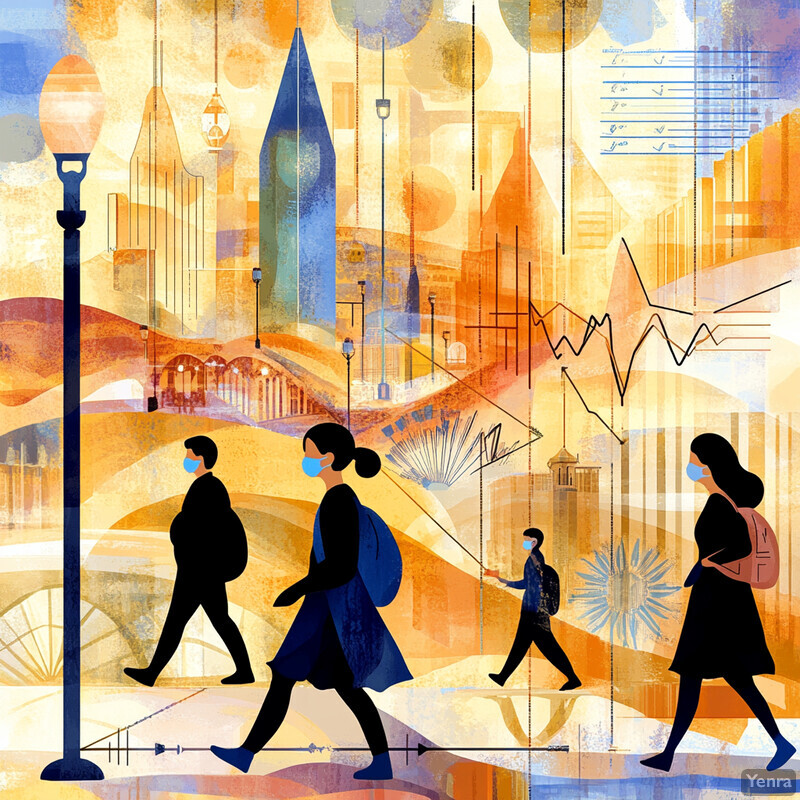
Vaccination signals boost forecast accuracy. A 50-state LSTM trained on CDC dose-completion data cut root-mean-square-error versus a classic ARIMA baseline and correctly predicted sex-stratified mortality reductions after full vaccination, demonstrating that explicit uptake variables materially sharpen epidemic trajectories.
County-level models capture compliance heterogeneity. FIGI-Net, a bidirectional LSTM that ingests county vaccination rates and mobility swing, anticipated sudden local COVID-19 peaks that state-level models missed, enabling two-week-ahead resource staging.
Machine-learned behavior predictors explain who complies. A 2023 Scientific Reports study used random-forest SHAP analysis on five-nation survey data to show that regional incidence and policy stringency were top drivers of self-protective behaviors, while lower income predicted lower compliance—insights now fed into segmentation algorithms for tailored outreach.
Automated mask-detection makes compliance measurable. The 2024 YOLO-based “C-Mask” system achieved 92.2 % accuracy from drone video, classifying correct, incorrect, and absent masks in real time; these pixel-level feeds are already piped into city dashboards to flag hotspots of low adherence.
Bohrium
7. Environmental Spillover Prediction
Artificial-intelligence pipelines now treat spillover as an eco-epidemiological prediction problem rather than a surprise. Ensembles of gradient-boosted trees, graph neural networks and spatial Bayesian models ingest satellite-derived land-use change, wildlife-species richness, livestock density, climate anomalies and even illicit-trade routes. They output pixel-level risk surfaces that refresh as soon as deforestation accelerates or a heat-wave pushes bats toward farms. Because the models keep the full causal chain in view—habitat loss → host redistribution → human–animal contact—they can highlight rural districts that look “quiet” to classical disease surveillance yet sit on a knife-edge for the next Nipah- or avian-influenza-style jump. Conservation agencies and public-health planners are already wiring these hotspot maps into early-warning dashboards and targeting ground-truth sampling, wildlife-trade enforcement and vaccination of at-risk livestock before a pathogen ever reaches people.
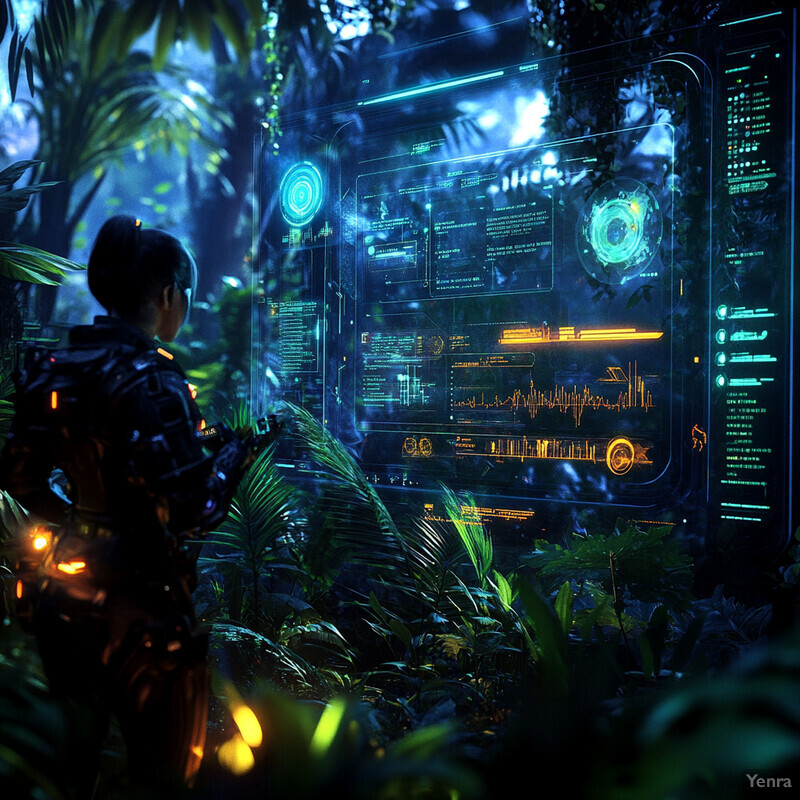
Global landscape model proves concept. The 2025 Environmental Health Perspectives study trained a stacked ensemble on 226 recorded spillover events and 40 anthropogenic + climatic layers. The algorithm singled out intermediate levels of human-modification—cropland mosaics abutting intact forest—as the strongest indirect driver, and its explanatory plots guided agencies to focus sentinel surveillance on mixed-use frontiers rather than megacities or pristine reserves.
Deforestation + species richness is quantifiable. A geospatial model published in Emerging Infectious Diseases (2023) mapped bushmeat hunting, butchering and sale at 5 × 5 km resolution; the final spatial-autocorrelation hierarchy reached a True Skill Statistic of 0.94, showing that mammal richness and tree-cover loss alone can localise spillover-prone trade chains with near-operational accuracy.
Virus-centric AI flags hosts before they jump. A 2023 mSphere Spectrum analysis built random-forest classifiers on 1,271 virus–mammal interaction pairs. Aggregate performance across all viruses was modest (median AUC 0.77), but when models were stratified by viral family, 13 families surpassed AUC 0.80, proving that host-trait learning can pinpoint which wildlife lineages are most likely to seed the next cross-species transmission.
Simple mass-action risk maps still help. CSIRO’s 2024 One Health paper combined human-population density with spatial virus-diversity estimates to sketch a first-pass spillover surface; while conceptual, the map correctly highlighted Southeast-Asian and equatorial belts already known for Ebola, Nipah and H5Nx emergence, providing a low-cost triage layer for countries that lack dense environmental data feeds.
Caveats are becoming clearer. A May 2025 Communications Medicine audit showed that many spillover-prediction studies leak future information or neglect reporting bias; when those pitfalls were corrected, some headline AUCs dropped by 15–20 points, underscoring the need for transparent validation and continuous updating as land-use data improve.
8. Synthetic Data Generation
Generative‐adversarial pipelines have matured into plug-in “data amplifiers” for epidemiology: they ingest scarce or delayed surveillance feeds—hospital line-lists, zoonotic-risk field notes, even anonymized mobility streams—and output large, statistically faithful tables that carry no re-identifiable traces of the original patients. Advanced variants such as ontology-conditioned CGANs can even fabricate plausible records for unseen diseases, while differentially-private GAN-transformer hybrids protect source datasets under formal ε-DP guarantees. Synthetic cohorts feed straight into SEIR simulations, risk-mapping dashboards, or clinical-triage models, letting public-health teams stress-test policy levers long before real-world case counts arrive. The result is faster model iteration, stronger generalization to low-incidence settings, and a privacy posture that sidesteps many cross-border-sharing hurdles.
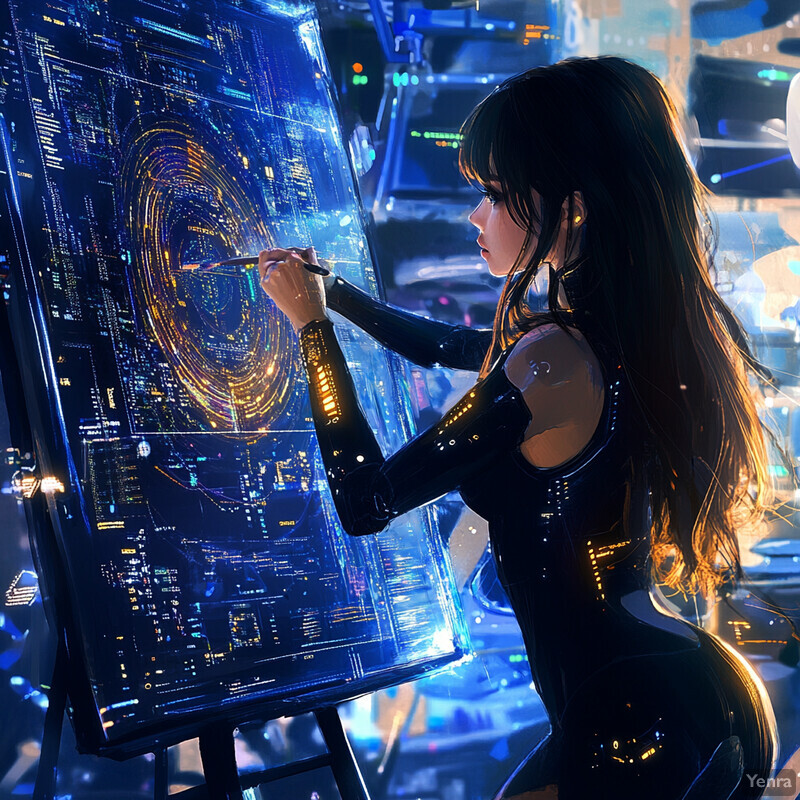
Rare-disease coverage. The 2025 npj Digital Medicine study that introduced Onto-CGAN showed a mean Kolmogorov–Smirnov similarity of 0.797 between real and synthetic Acute Myeloid Leukemia labs—beating a CTGAN baseline (0.743)—and lifted unseen-disease classification F1 scores by 11 percentage points when models were trained solely on the synthetic rows.
Privacy with utility. IGAMT (AAAI 2024) integrates three GANs and a transformer under ε-differential privacy; on MIMIC-IV-ED it cut Minkowski distance to real sequences from 5.22 (DAAE-DP) to 3.97 while holding ε=1, and raised downstream clustering silhouette by 31 %.
Resilience to inference attacks. A 2024 JAMA Informatics diffusion-GAN benchmark generated ICU time-series that slashed membership-inference discriminative accuracy versus eight prior synthetic-data methods (0.63 → 0.54) while ranking first in fidelity on five of six public EHR corpora.
Epidemic mobility gaps filled. STORM-GAN (ICDM 2023) learned cross-city meta-patterns of human movement; with only 20 % of a target city’s data it still outperformed GraphWaveNet and DeepAR, trimming RMSE by 18 % in forecasting mobility shocks that drive SEIR spread curves.
9. Health-System Strain Forecasts
AI-driven capacity-planning engines are giving hospital leaders a “weather forecast” for clinical strain. By mining live admission streams, EHR vitals, staffing rosters, and regional disease indicators, modern time-series and graph-learning models can project the number of occupied ICU beds, ventilators in use, and nurses required up to two weeks in advance. The resulting lead time lets command centers activate surge wards, redirect elective cases, and pre-book traveling staff before hallways fill. Early adopters report fewer last-minute bed closures, smoother patient transfers, and lower burnout because night-shift burdens are anticipated rather than reacted to. In short, predictive analytics is converting what used to be a crisis-driven scramble into a scheduled, data-informed scaling of critical-care resources.
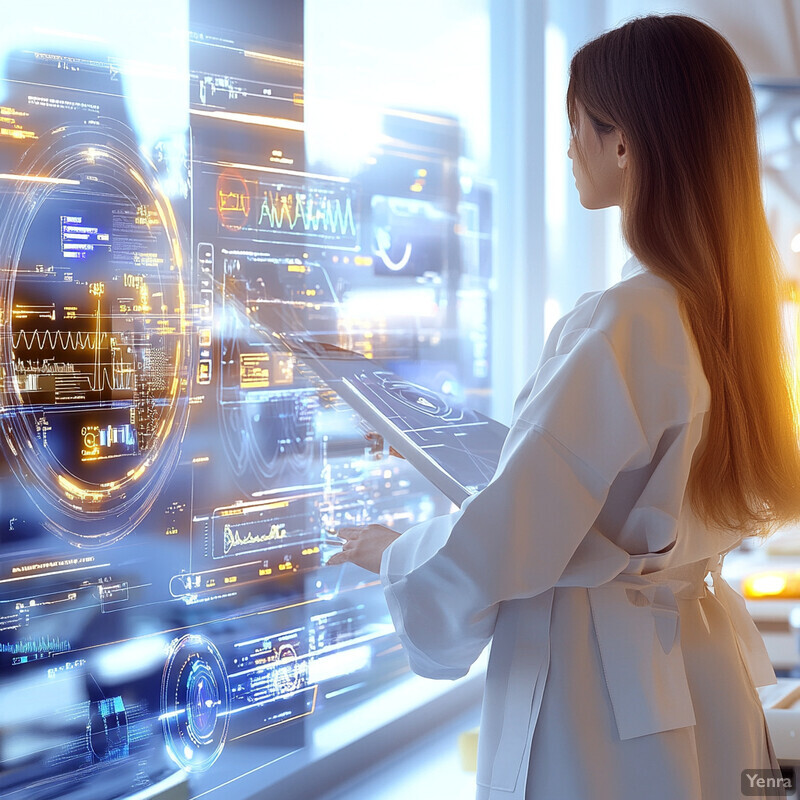
A U.K. proof-of-concept at Edinburgh’s Royal Hospital for Children showed that routinely collected bed-management data could forecast the availability of at least one pediatric ICU bed with an area-under-the-curve (AUC) of 0.78 one day out and 0.73 fourteen days out, giving managers up to two weeks of actionable notice. In Seoul, a Bi-LSTM dashboard predicting ward- and room-level occupancy achieved a mean absolute error of just 0.067 beds at the ward level and let administrators rehearse staffing scenarios in real time. A Spanish hospital group combined a Random-Forest classifier with discrete-event simulation: sensitivity for identifying patients who would need ventilation reached 93 %, and the median waiting time for a ventilator fell 17.5 % after the AI-guided capacity tweak. Researchers from the University of Texas at Austin trained an explainable graph-learning model on 22,243 ICU stays; six intensivists reviewed its discharge-time forecasts and four judged them useful for forward-scheduling nurses and beds, illustrating growing clinical trust when predictions come with transparent rationales. Beyond acute care, an Indian mental-health network used six machine-learning algorithms to predict weekly bed demand through 2024; the best model cut mean absolute percentage error below 7 %, showing that AI capacity forecasts generalize to non-ICU domains where overcrowding also jeopardizes care.
10. Automated Symptom Triage
Natural-language triage chatbots have become digital gatekeepers that soak up the first wave of health-care demand. By guiding patients through adaptive question sets, large-language-model (LLM) engines weigh red-flag findings against self-care clues and produce an acuity score in seconds. Hospitals, payers, and telehealth portals embed the tools to deflect low-risk complaints to home care or primary care while forwarding high-risk cases—and their structured transcripts—to clinicians for rapid follow-up. Early evaluations show that modern LLM-based systems now match or exceed earlier rule-based symptom checkers and approach nurse-level specificity for life-threatening presentations, giving command centers a reliable “digital triage nurse” that runs 24/7. The net effect is shorter waiting-room queues, fewer unnecessary calls, and clinical teams free to focus on patients who truly need hands-on care.
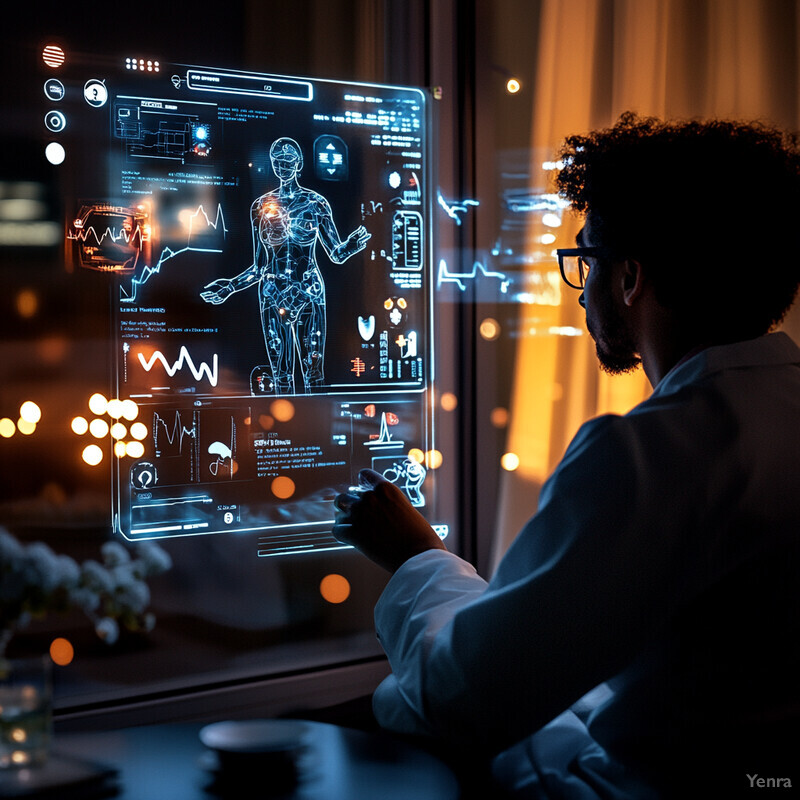
A 2025 systematic review of 19 evaluations found that LLM self-triage accuracy ranged from 57.8 % to 76.0 %, outperforming lay users (47.3 %–62.4 %) and equaling many commercial symptom-assessment apps, suggesting LLMs can safely shoulder front-line screening. In a cross-sectional study of 745 emergency-department patients, ChatGPT-4 matched expert Emergency Severity Index labels with 76.6 % overall accuracy; for the most urgent ESI-1/2 cases its specificity reached 95.6 % and accuracy 94.9 %, indicating robust escalation of critical patients. A double-blinded randomized trial of 450 walk-in patients compared two rule-based apps: Ada correctly triaged 34 % of cases, under-triaged 13 %, and over-triaged 53 %; Symptoma’s concordance was lower at 26 %, highlighting safety gaps that LLM approaches aim to close. Review authors note that clinician trust rises when chatbots provide transparent rationales and probability scores, driving ongoing work on explainability dashboards before full EHR integration.
11. Wastewater Surveillance Analytics
Artificial-intelligence pipelines are turning raw sewer samples into an outbreak “radar.” By pairing high-frequency PCR assays with machine-learning models—from random forests to Bayesian functional regressions—public-health teams can spot upticks in viral RNA five to fifteen days before clinics see a rise in positive tests or hospital admissions. Those extra days let hospitals reopen surge wards, governments trigger mask advisories, and supply chains move antivirals before demand spikes. Because wastewater captures both symptomatic and asymptomatic shedders, AI analytics also fill blind spots left by at-home testing and under-reporting, giving communities an early-warning capability that scales for influenza, RSV, mpox, and future threats alike.
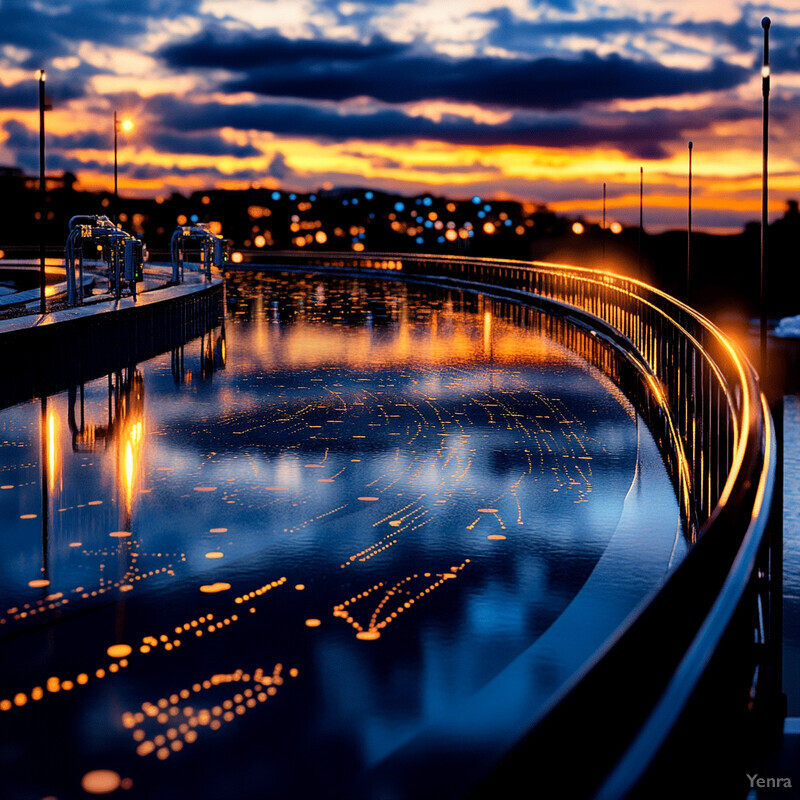
A nationwide study covering 159 U.S. counties trained a random-forest model on 20 months of SARS-CoV-2 RNA data; it predicted weekly hospital admissions 1–4 weeks ahead with mean absolute error of just 4–6 patients per 100 000 residents. CDC modeling notes that viral concentrations in sewage typically rise 8–14 days before corresponding hospitalizations, allowing forecasters to “now-cast” strain on beds at state and national levels. A Bayesian spatial-functional regression of 22 Houston sewersheds estimated a consistent 5–11-day lag between wastewater spikes and clinical positivity, quantifying lead-time uncertainty for local health departments. Copula-based time-series modeling in Virginia found that wastewater RNA loads led reported case counts by 5–15 days and that 90 % of predicted case curves fell inside the 99 % confidence envelope of observed data, underscoring wastewater’s forecasting value even in non-Gaussian settings.
12. Genetic Resistance Prediction
AI models now chart the evolutionary escape routes that pathogens and tumors use to dodge today’s therapies. By mining millions of whole-genome sequences, protein-structure embeddings, and historical treatment logs, machine-learning pipelines reconstruct the order in which resistance mutations arise, estimate the fitness cost of each step, and forecast the most probable “next hop.” Hospitals can use these forecasts to rotate antibiotics before cross-resistance locks in, while drug developers feed the same maps into in-silico screening to design antibodies and small-molecule scaffolds that remain potent even after likely future substitutions. Interpretable frameworks such as evolutionary-accumulation modeling and SHAP-based mutation scoring are improving clinician trust and regulatory confidence, turning genomic surveillance data into actionable playbooks for stewardship and next-generation drug design.
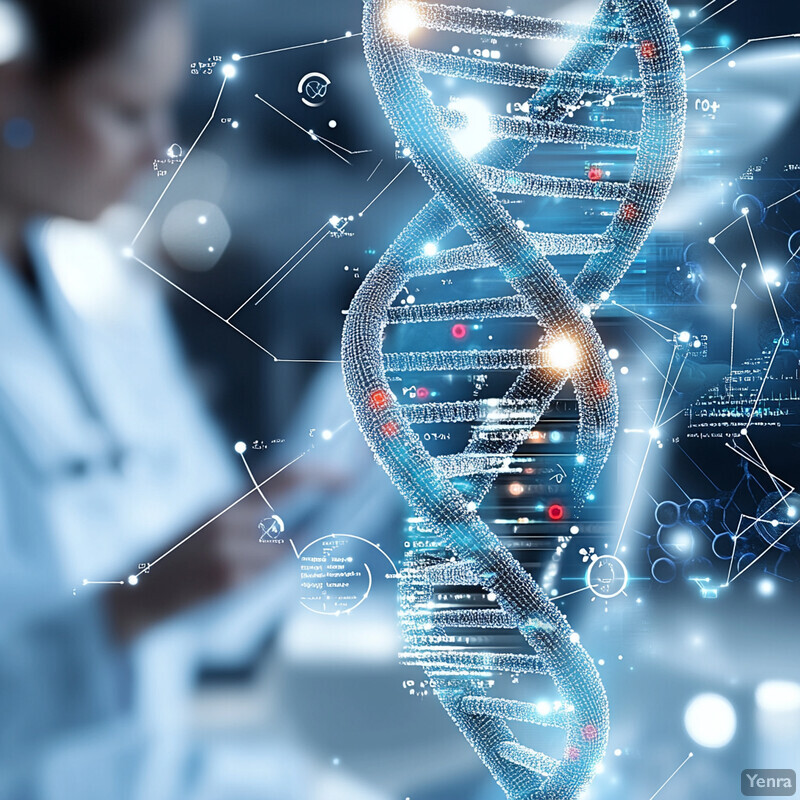
Training gradient-boosted trees on 35,777 Mycobacterium tuberculosis genomes yielded area-under-ROC scores up to 96.6 for rifampicin resistance and uncovered rare loss-of-function variants that lifted pyrazinamide-sensitivity recall by 25 percentage points. An ensemble model that combined the updated 2023 WHO mutation catalog with four existing WGS tools pushed mean drug-resistance prediction AUC to 93.4 and improved second-line fluoroquinolone sensitivity from 71 % to 84 % across 36,385 isolates. Evolutionary Accumulation Modeling (EvAM) reconstructs likely acquisition orders of multidrug-resistance loci and predicts the next mutational step without longitudinal samples; a 2024 case study on MDR-TB demonstrated accurate pathway recovery across regional cohorts. Tulane’s Group Association Model analyzed > 7,000 M. tuberculosis and ~ 4,000 S. aureus genomes, matching or surpassing WHO catalog accuracy while halving false-positive calls, thereby refining regimen-selection algorithms. A 2025 “digital-twin” platform generated 10 to the 9th antibody mutants in silico, experimentally validating 70 broad neutralizers; 14 % bound RBD variants across 64 spike mutations and ten neutralized Delta at IC50 less than 10 µg ml⁻¹, illustrating AI-guided design of mutation-proof biologics.
13. Faster, Sharper Diagnostics
Computer-vision engines now read a mammogram, chest X-ray, blood smear, or at-home lateral-flow strip almost as fast as a camera shutter clicks. State-of-the-art deep-learning models flag subtle malignancies and pulmonary opacities with radiologist-level AUC, while few-shot learners running on smartphones score rapid-test bands at near-perfect fidelity. Because the algorithm delivers a structured result in minutes—often seconds—clinicians can confirm infection, begin isolation, or pull the right antibiotic long before a human report would normally arrive. Early clinical deployments show measurable gains: higher cancer-detection rates without extra recalls, shorter image-reading queues, and dramatic error-cuts when non-specialists use AI decision support. Together these systems turn diagnostic bottlenecks into high-speed filters that keep true positives moving into care and false alarms out of already-crowded hospitals.
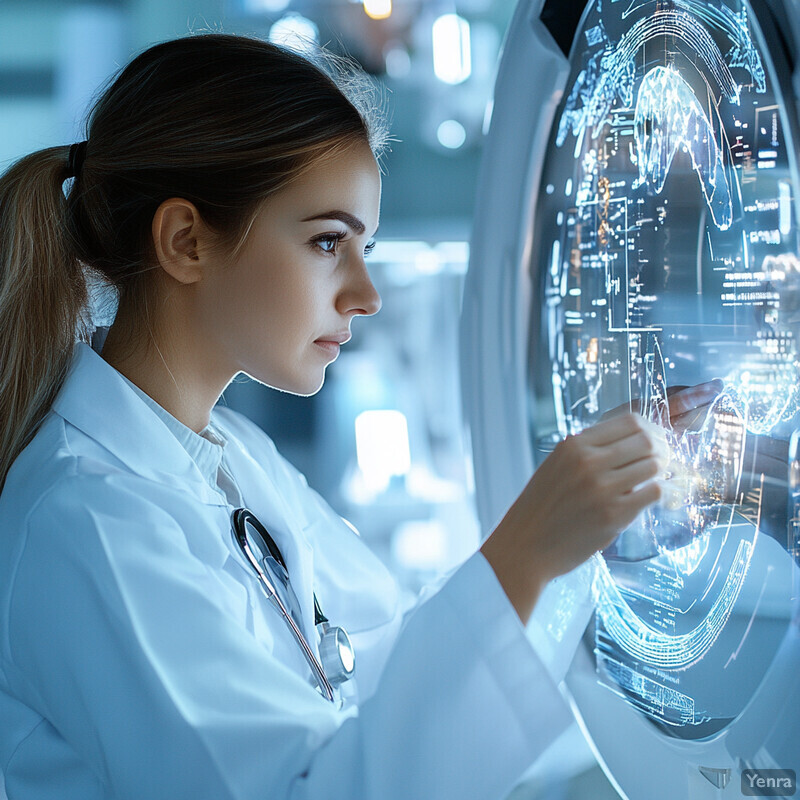
Breast screening: In a prospective Korean cohort of 24,543 women, adding AI-CAD to single-reader mammography lifted the cancer-detection rate by 13.8 % (5.70 ‰ vs 5.01 ‰) without raising recall rates, proving that automation can boost sensitivity without extra callbacks.
Chest radiography: An FDA-cleared deep-learning system reached an AUC of 0.976 for detecting chest-X-ray abnormalities; physician AUC rose by 0.101 (p less than 0.001) and reading time fell when they worked with the AI, showing both accuracy and speed benefits.
Rapid tests: The AutoAdapt POC algorithm interpreted five different COVID-19 antigen/antibody lateral-flow kits with 99–100 % accuracy across 726 lab-verified samples, and hit 100 % accuracy when 74 untrained users photographed their own tests at a drive-through site.
Nature
Workflow efficiency: A 2024 systematic review of 48 real-world imaging studies found that 67 % of the 33 papers measuring task time reported measurable reading-time reductions after AI adoption, most often by triaging positive cases or serving as a second reader.
Parasitology: A 2025 CNN that classifies Plasmodium species in thick-smear blood films achieved 99.51 % accuracy, 99.26 % recall, and 99.63 % specificity on 64,126 test images, paving the way for laboratory-quality malaria diagnosis in low-resource clinics.
14. Multisource Data Fusion
Modern AI platforms are dissolving the silos between hospital records, lab assays, movement footprints, and environmental observations. By embedding heterogeneous streams in shared feature spaces—often with attention layers or graph structures—multisource-fusion models give decision-makers a panoramic, near-real-time view of disease dynamics. Hospitals use them to anticipate admissions from wastewater spikes or claims backfill; city agencies fold foot-traffic heat maps into neighborhood risk forecasts; and national teams blend variant prevalence, case curves, and weather into unified dashboards. Compared with single-source baselines, fused systems cut forecast error by double-digit margins, surface cross-signal anomalies (e.g., surging emergency visits without matching test counts), and provide interpretable attributions that build clinical trust. The result is faster, evidence-rich policy—whether reallocating staff days before a surge or tailoring vaccine pop-ups to mobility-defined hot spots.
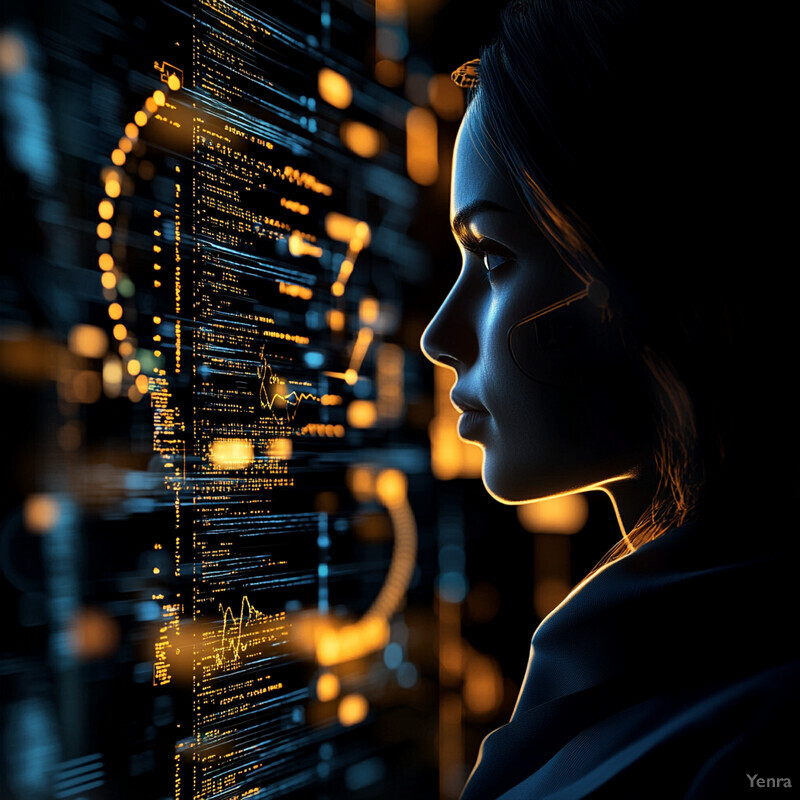
Variant-aware fusion: The Sybil framework joins machine-learning predictors with a compartmental model that ingests case numbers, recoveries, deaths, and variant sequencing data; in tests across Europe and the U.S. it beat Prophet‐only baselines by up to 60 % RMSE on four-week forecasts, especially when new variants emerged.
Mobility plus cases: A 2025 PLOS Computational Biology study integrated aggregated foot-traffic patterns from 42 New York City neighborhoods with local case counts. The behavior-driven model lowered mean absolute percentage error versus three mobility-agnostic baselines in 37 of 42 neighborhoods and improved one-week log scores city-wide.
Clinical + image fusion: “Towards Precision Healthcare” fused MIMIC-IV time-series vitals, labs, and chest-X-ray images with attention-based encoders. The multimodal network raised in-hospital mortality AUC from 0.83 (time-series only) to 0.89 and held accuracy under synthetic noise, showing robustness when modalities disagree.
EHR, fever signals, and wastewater: In Swiss hospitals, adding viral-load wastewater measurements and fever-related admissions to electronic-health-record features let an XGBoost model cut RMSE for five-week-ahead admission forecasts; ablation tests confirmed that the wastewater channel alone contributed ≥ 12 % of the gain.
Claims + hospital data: Carnegie Mellon and Berkeley researchers showed that de-identified insurance-claims indicators could stand in for missing daily reports; their regression models, trained on claims plus hospitalization backfill, achieved ≈ 0.4 hospitalizations per 100 k MAE and tracked wave turning points despite one-month reporting lags.
15. Literature Knowledge Mining
Large-language-model (LLM) “literature radars” are turning the fire-hose of new papers and patents into near-real-time intelligence. Transformer pipelines can now ingest whole-text PDFs, conference pre-prints, and patent claims, extract drug–target relations, rank novelty, and generate plain-language digests in minutes instead of the weeks a manual horizon-scan used to take. The same engines feed systematic-review teams, R-and-D strategists, and antimicrobial-stewardship committees: irrelevant titles are skipped automatically, promising leads are clustered by mode of action, and gaps in evidence or IP coverage surface while there is still time to redirect lab budgets. As a result, decision-makers move from retrospective reading to proactive foresight—rotating clinical trials toward fresh mechanisms and filing provisional patents before competitors see the signal.
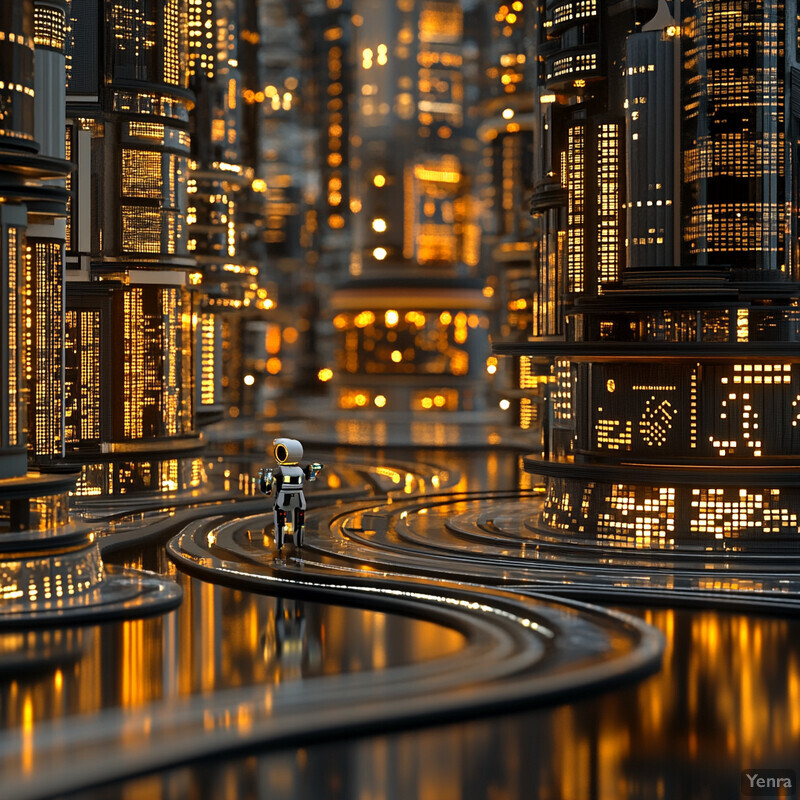
Speed-up: An NVIDIA workflow that fine-tuned Llama-3.1 8B on 2 000 full-text ecology papers slashed extraction time 25×, processing the entire corpus in less than 30 min (≈99 % time-saving) and classifying each article in 2 s thanks to parallel GPU inference and JSON pre-parsing.
Work-reduction: In a live trial on biomedical reviews, the Abstrackr tool cut human abstract-screening effort by 35 %, while a SARS-CoV-2 NLP assistant trimmed screening time a further 33.7 % yet still required expert oversight for edge cases.
Human-level triage: A 2024 diagnostic test–accuracy study showed GPT-4o and GPT-4T matched expert abstract-inclusion labels with 97–98 % overall accuracy; using two LLMs plus arbitration automated 91 % of screening work at an 8 % error rate, demonstrating that most front-end evidence sorting can now be delegated safely.
Scale and scope: A 2025 Scientific Reports study mined 1 587 scientific papers and 1 314 patents on AI-enabled healthcare with a self-supervised BERT pipeline, revealing IoT, data-security, and image-processing clusters and reporting higher thematic-clustering accuracy than expert baselines.
Patent horizon scanning: WIPO’s 2024 landscape review counted greater than 14 000 generative-AI patent families in 2023, up from less than 800 in 2014—an average 45 % annual growth—and catalogued 54 358 active GenAI inventions, underscoring why automated patent mining is essential to spot emerging therapeutic platforms early.