1. Automated Feature Extraction from Rich Data Sources
AI enables rapid processing and extraction of nuanced behavioral indicators from large-scale, unstructured data (e.g., text from surveys, social media sentiment, transaction histories), which helps behavioral economists identify latent cognitive biases and decision-making patterns.
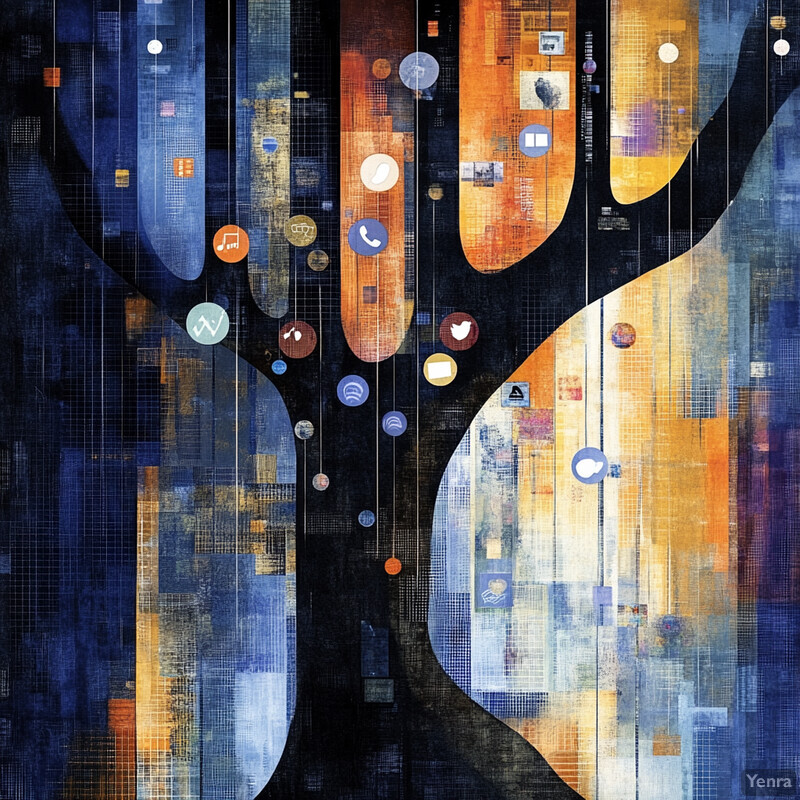
Artificial intelligence algorithms, especially those trained on vast amounts of unstructured data, have made it possible to identify subtle patterns in human behavior that would be difficult to detect using traditional methods. By applying natural language processing to social media posts, online reviews, and forum discussions, researchers can capture attitudinal shifts and emotional responses that influence economic decisions. Image and speech recognition technologies can also reveal cues related to trust, risk appetite, and decision uncertainty in real-time. This automated approach to extracting features not only streamlines the data gathering process but also presents a richer tapestry of behavioral indicators, enabling behavioral economists to model human decision-making in a more context-sensitive manner.
2. Advanced Predictive Modeling with Machine Learning
Modern machine learning algorithms can more accurately forecast economic behaviors—such as consumer purchasing patterns, investment decisions, or public policy reactions—by capturing complex, non-linear relationships that traditional econometric models may miss.
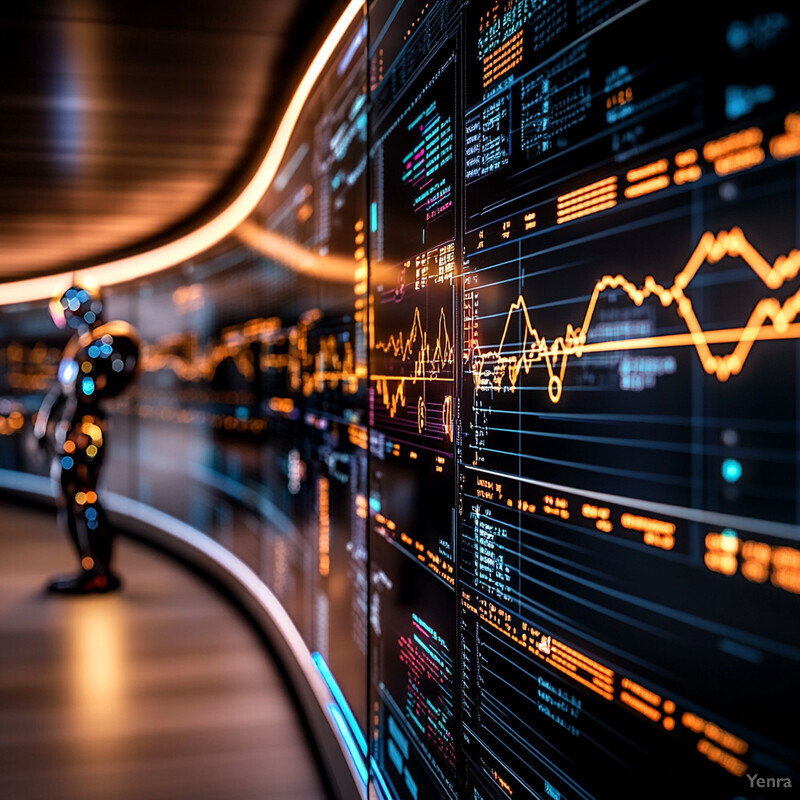
Behavioral economics traditionally relied on linear or simple non-linear models to forecast outcomes, often missing the complexity inherent in real-world decision-making. Machine learning models—ranging from gradient boosted trees to deep neural networks—enhance predictive accuracy by adapting to high-dimensional data and capturing intricate, non-linear relationships between variables. These models incorporate factors like temporal dynamics, evolving preferences, and feedback loops, resulting in predictions that more closely mimic observed outcomes. As a result, policymakers, firms, and researchers gain better foresight into how people will respond to changes in prices, incentives, and regulations, ultimately informing more effective decision-making strategies.
3. Uncovering Hidden Biases and Heuristics
Deep learning models can sift through massive datasets to detect subtle heuristics, framing effects, or temporal inconsistencies in decision-making that often remain hidden in classical models.
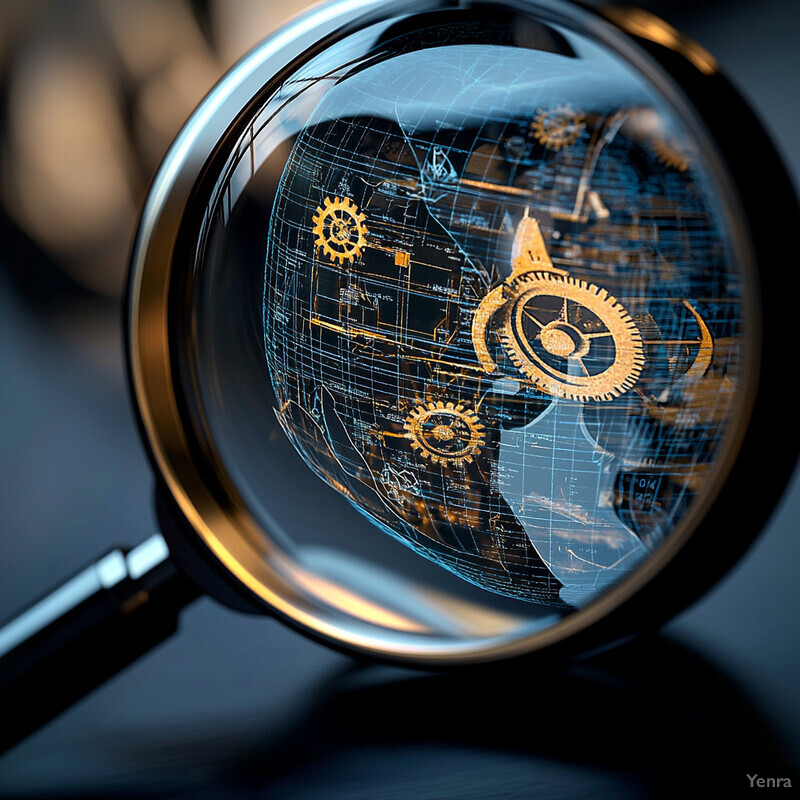
Conventional economic models often assume rational agents, but behavioral economics recognizes that individuals rely on mental shortcuts, rules of thumb, and systematic biases. AI excels at detecting these patterns by combing through diverse datasets—such as survey responses, transaction logs, and user interaction histories—with sophisticated algorithms that highlight subtle anomalies in behavior. For example, AI can reveal when people disproportionately overweight recent events, succumb to framing effects, or show strong present-bias in their choices. Discovering these hidden biases and heuristics is crucial for refining theories of bounded rationality, informing interventions designed to improve decision quality, and ultimately enhancing the realism and applicability of behavioral economic models.
4. Dynamic, Agent-Based Simulations with Reinforcement Learning
AI-driven agent-based models leverage reinforcement learning to simulate environments where virtual agents adapt their strategies over time, capturing learning processes, habit formation, and the influence of social norms on economic decisions.
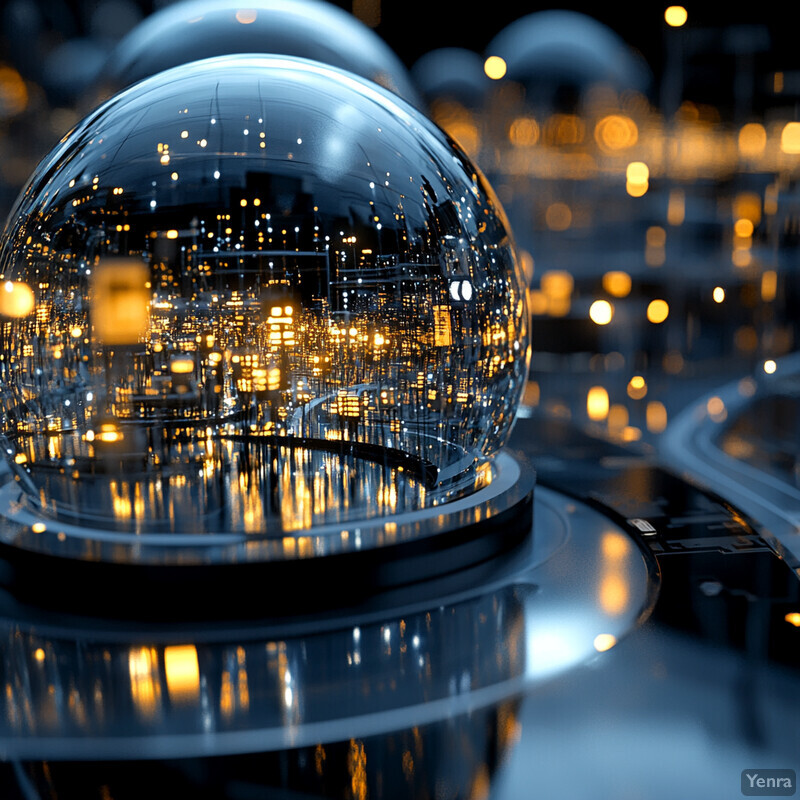
Agent-based modeling has long been a tool in behavioral economics to simulate how individual behaviors interact and evolve in complex environments. With AI-powered reinforcement learning, these virtual agents can learn from their experiences and update their strategies over time. Instead of static assumptions about agent preferences, beliefs, or heuristics, reinforcement learning agents adapt dynamically in response to changing economic conditions and the behaviors of other agents. This approach can model processes like habit formation, social norm emergence, and adaptive responses to regulatory changes, thereby shedding light on the rich tapestry of human decision-making within markets and institutions. It leads to simulations that not only mirror observed behavior more closely but also generate novel predictions and insights into how small changes in the environment might yield substantial shifts in collective outcomes.
5. Customizing Interventions and Nudges
With machine learning, policymakers and organizations can identify specific subgroups most susceptible to certain nudges or incentives, ensuring interventions are more targeted, efficient, and effective.
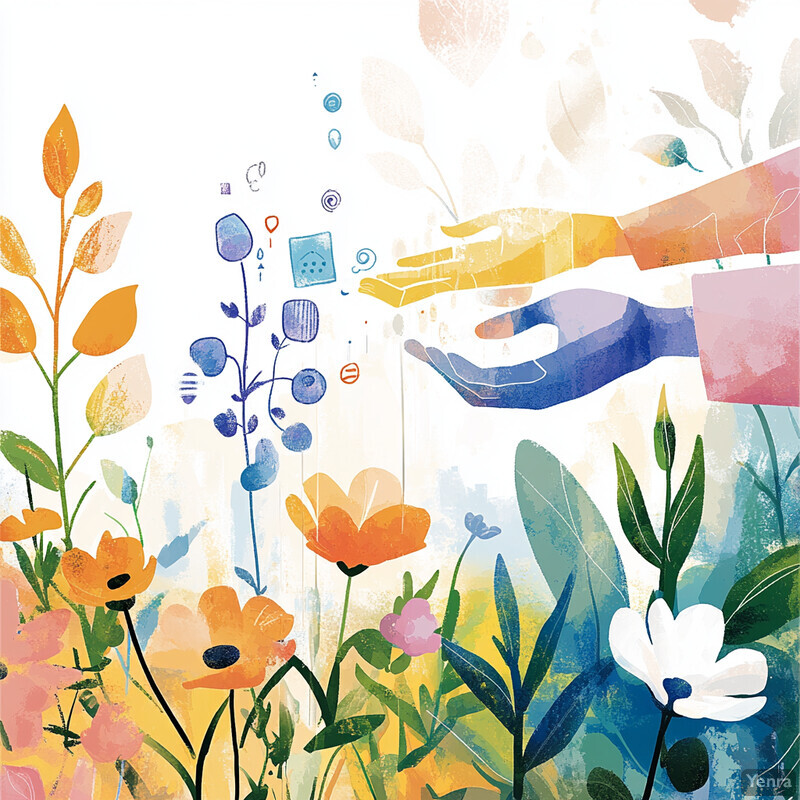
Behavioral economists have demonstrated that subtle cues or “nudges” can guide individuals toward better financial, health, or environmental decisions. AI can enhance the potency of these interventions by identifying which nudges work best for particular segments of the population. Machine learning models can cluster individuals based on their response to past interventions, sensitivity to risk, or susceptibility to social influence, enabling policymakers or service providers to design personalized nudges. This customization ensures interventions are neither too broad nor ineffective, thereby maximizing their impact. In this way, AI turns a one-size-fits-all approach into a finely tuned mechanism for shifting behavior in a more socially beneficial direction.
6. Integration of Behavioral and Traditional Economic Data
AI techniques facilitate the seamless merging of traditional economic indicators (e.g., prices, incomes) with behavioral metrics (e.g., attentional shifts, emotional sentiment), providing a more holistic view of decision-making contexts.
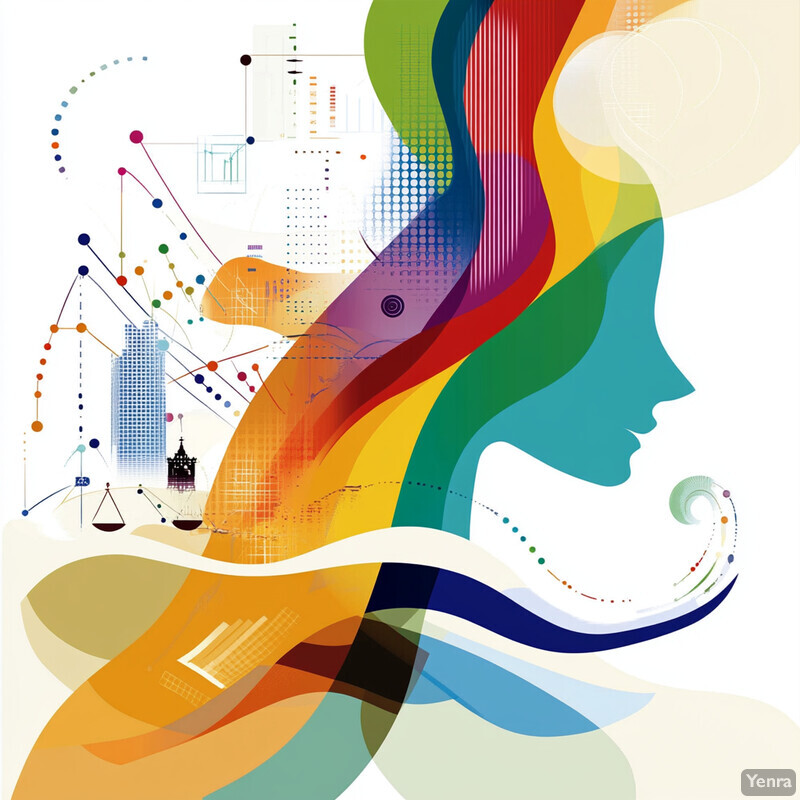
AI tools enable seamless integration of conventional economic indicators, such as GDP growth, inflation, and income distribution, with behavioral metrics like sentiment, attention, and time-inconsistent preferences. By using machine learning models that can handle multiple data modalities, researchers can combine macro-level trends with micro-level decision patterns. This integrated perspective fosters a more holistic understanding of economic phenomena, bridging the gap between theory and practice. It means that insights about social norms, cultural influences, and psychological triggers can be incorporated alongside standard economic variables, ultimately creating richer, more predictive, and more explanatory models of how people behave in real economic contexts.
7. Adaptive Experimental Design and Real-Time Feedback
In behavioral experiments, AI can optimize the design of studies on-the-fly, dynamically adjusting parameters, stimuli, or incentives to probe hypotheses more thoroughly and efficiently.
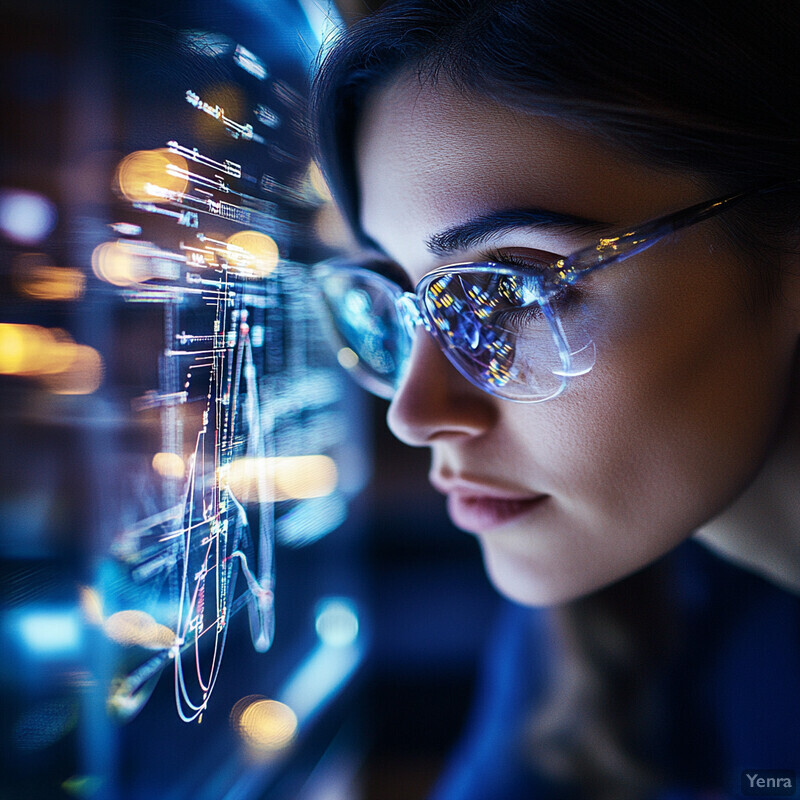
Experiments are central to behavioral economics, but static designs often fail to capture the dynamic complexity of decision-making. AI can optimize experiments on the fly, using reinforcement learning or Bayesian adaptive methods to adjust stimuli, questions, or incentive structures based on preliminary responses. For example, if early participants show reluctance to invest in a risky asset, the AI could alter subsequent conditions to probe how their risk aversion might change under different framing or incentive adjustments. By continually refining the experimental approach, researchers can hone in on more precise causal mechanisms, leading to sharper insights and more generalizable models. This reduces waste in data collection and increases the reliability of experimental findings.
8. Forecasting under Uncertainty and Ambiguity
Machine learning models that account for incomplete information, noisy signals, and risk preferences provide richer approximations of how individuals deal with uncertainty, enhancing models of bounded rationality.
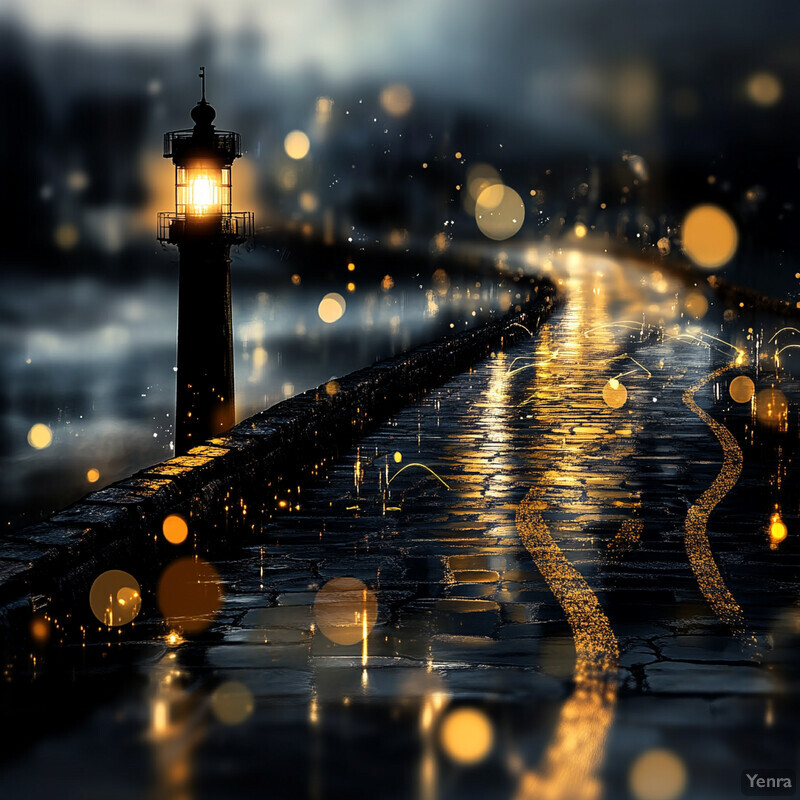
People often make decisions without complete information, facing both known and unknown risks. AI excels in modeling these scenarios by employing techniques that handle missing data, imprecise signals, or evolving distributions of outcomes. Bayesian machine learning and probabilistic programming can incorporate subjective beliefs, ambiguity aversion, and changing uncertainty levels into forecasting models. As a result, researchers and analysts can better predict how individuals respond to uncertainty—whether by seeking more information, relying on heuristics, or adjusting their risk tolerance. This improved forecasting feeds back into better policy design and market predictions, ensuring that economic models align more closely with the psychological realities of uncertainty.
9. Calibration and Estimation of Complex Behavioral Models
AI-assisted Bayesian methods and numerical optimization techniques automate the calibration of intricate behavioral models, reducing the time and complexity needed to estimate parameters consistent with observed data.
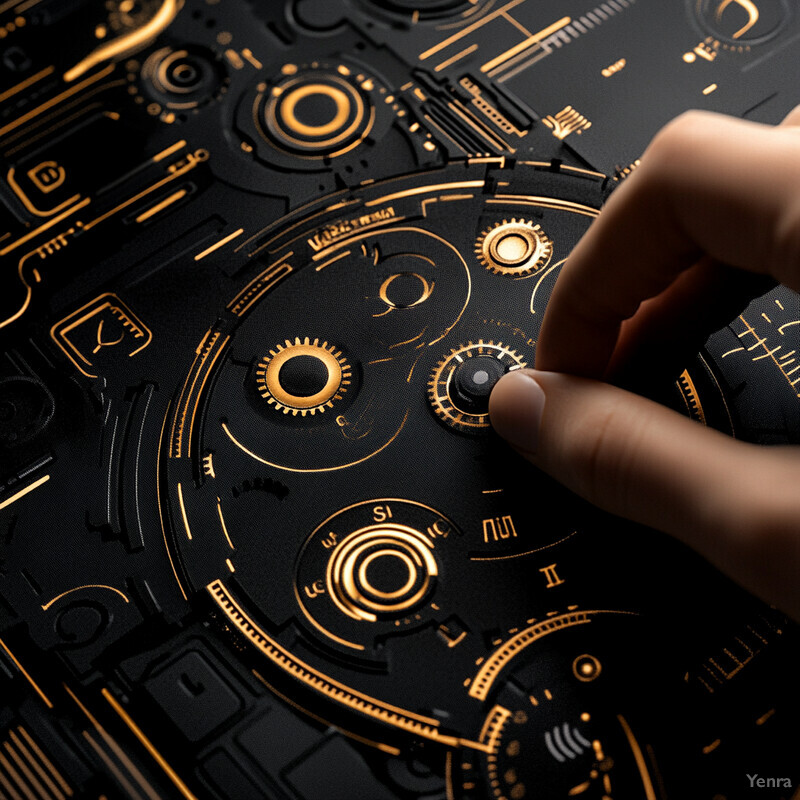
Behavioral economics has produced intricate theoretical models capturing various departures from rationality—such as reference dependence or probability weighting. However, calibrating these models to real-world data can be challenging and time-consuming. AI-based optimization techniques, including genetic algorithms and particle swarm optimization, help tune parameters automatically, ensuring that the model's predictions best fit observed behaviors. Moreover, AI-assisted Bayesian inference provides a structured way to update beliefs about parameter values as new evidence becomes available. This reduces the manual and computational burden on researchers and enhances the precision with which theoretical models map onto empirical realities.
10. Identification of Cultural and Contextual Variations
AI-driven clustering and classification methods isolate regional, cultural, or contextual differences in behavioral responses, improving model generalizability and highlighting local norms.
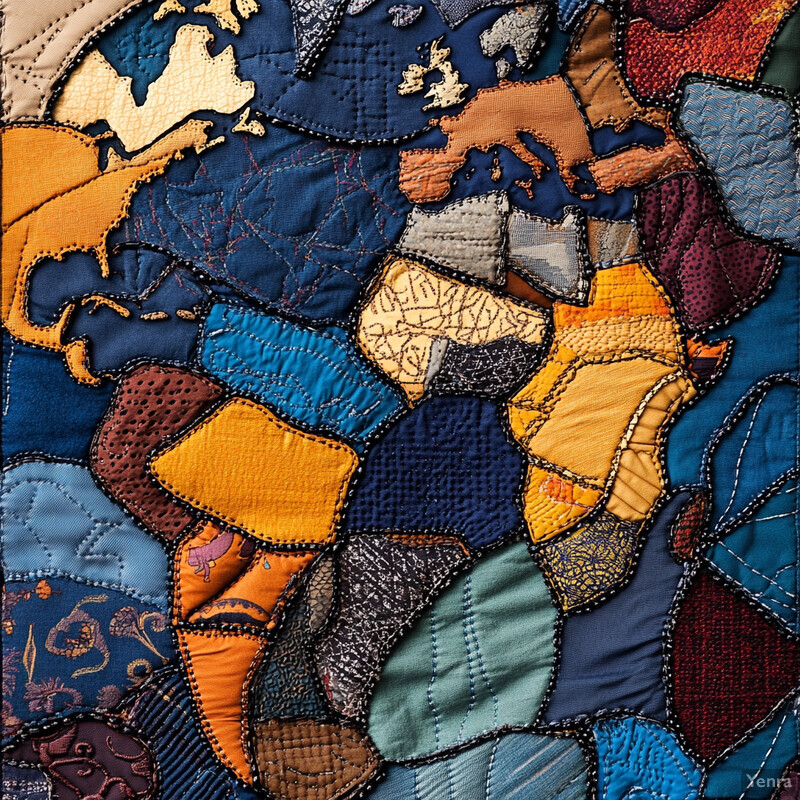
Decision-making is not monolithic; it varies widely across cultures, social contexts, and institutional frameworks. AI-driven clustering, classification, and factor analysis methods can segment data to reveal how certain biases or decision tendencies differ by region, socioeconomic group, or other contextual factors. This allows economists to understand why a particular policy might succeed in one cultural setting but fail in another. By highlighting these contextual nuances, AI provides actionable insights for tailoring policies, products, and interventions to local norms and values. Such context-specific models improve both explanatory power and the practical applicability of behavioral economics research.
11. Non-Linear Relationship Discovery
Beyond linear regression, deep neural networks capture complex, non-linear interactions between economic variables and psychological factors, refining understanding of phenomena like loss aversion and time-inconsistent preferences.
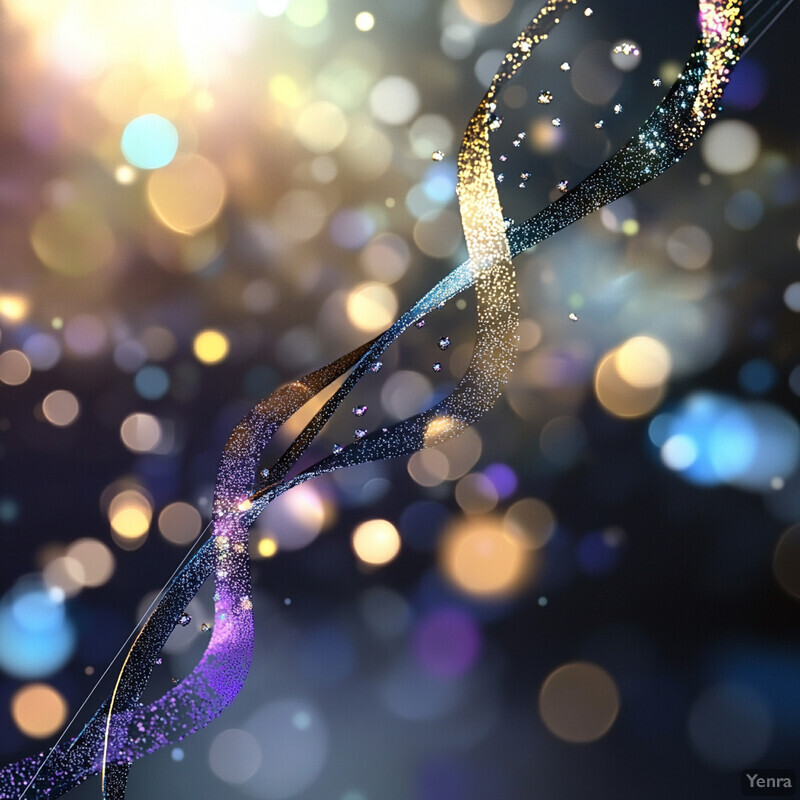
Traditional statistical models often assume linearity or simple functional forms. However, many behavioral relationships—such as the diminishing sensitivity seen in prospect theory or the complexity of time-inconsistent preferences—are non-linear. Deep neural networks and kernel-based methods can uncover these complex patterns without imposing strict functional assumptions. For example, AI might reveal how a small change in price perception triggers a disproportionately large shift in purchase behavior or how emotional states influence discounting rates in intricate ways. Identifying these non-linearities refines theoretical frameworks and ensures that policy recommendations better capture the nuanced reality of human decision-making.
12. Mapping Social Influence and Network Effects
AI techniques in network analysis measure how peer influence, social structures, and group dynamics shape individual decision-making, linking personal choices to broader social networks.
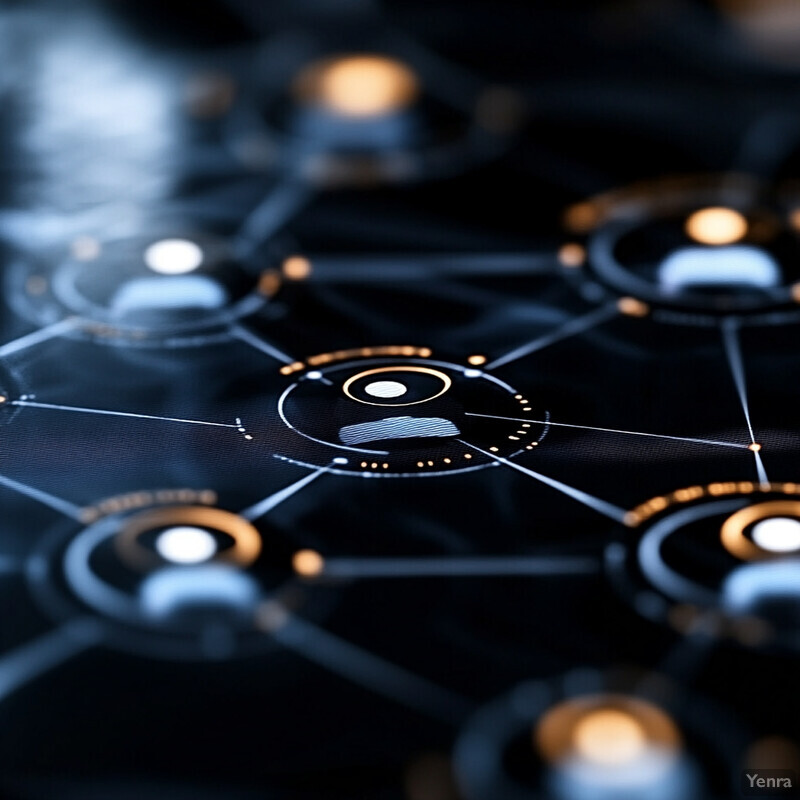
Human decisions are influenced heavily by social context, from peer pressure to market sentiment and the spread of trends. AI techniques for network analysis—such as graph embeddings, community detection, and influence maximization algorithms—enable economists to visualize and quantify how information, behaviors, and norms propagate through social networks. This not only explains group-level phenomena like panic buying or stock bubbles but also assists in designing interventions that leverage social proof or peer influence. Understanding these network dynamics allows for richer, more interconnected models that account for the social fabric underlying individual decisions.
13. Iterative Policy Simulation and Optimization
Policymakers can use AI-enabled simulations to repeatedly test various policy options under different behavioral assumptions, foreseeing unintended consequences and identifying interventions that maximize social welfare.
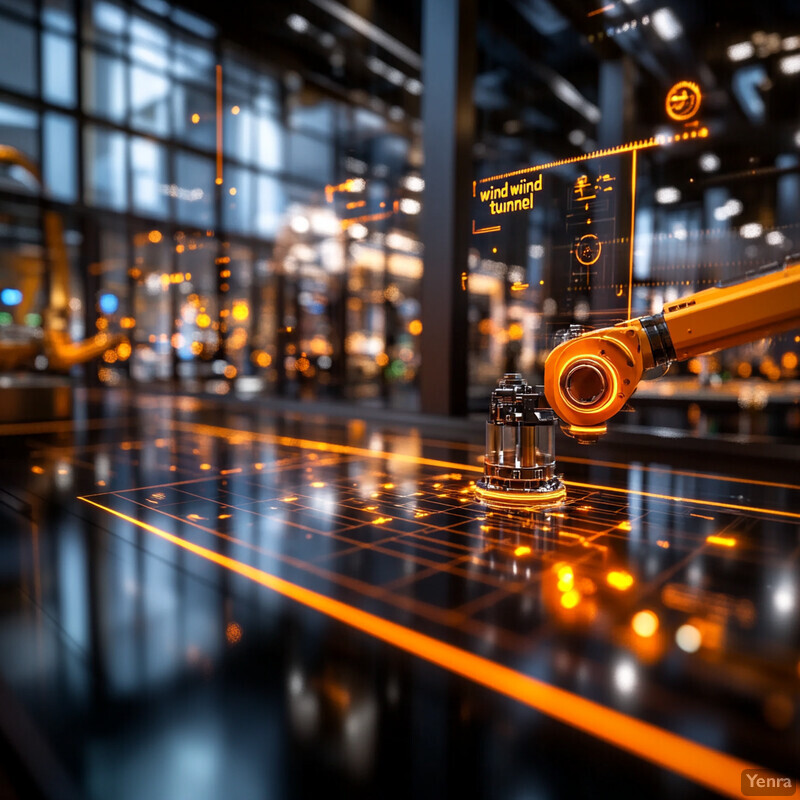
Policymakers can use AI-driven simulations to iteratively test policy options in a virtual environment before implementing them in the real world. By incorporating behavioral components, these simulations capture how people adapt to policy changes over time. For instance, an AI model can show how a tax incentive to promote greener choices interacts with existing preferences for convenience, and how these preferences may evolve after sustained exposure. Through iterative refinement, policies can be tuned to minimize unintended consequences, reduce social inequities, and achieve long-term goals. This approach leads to more robust, evidence-based policy-making guided by realistic behavioral assumptions.
14. Enhanced Personalization in Behavioral Finance
Financial service providers employ AI to detect individual risk profiles and behavioral tendencies, offering personalized advice and products aligned with real-world decision processes.
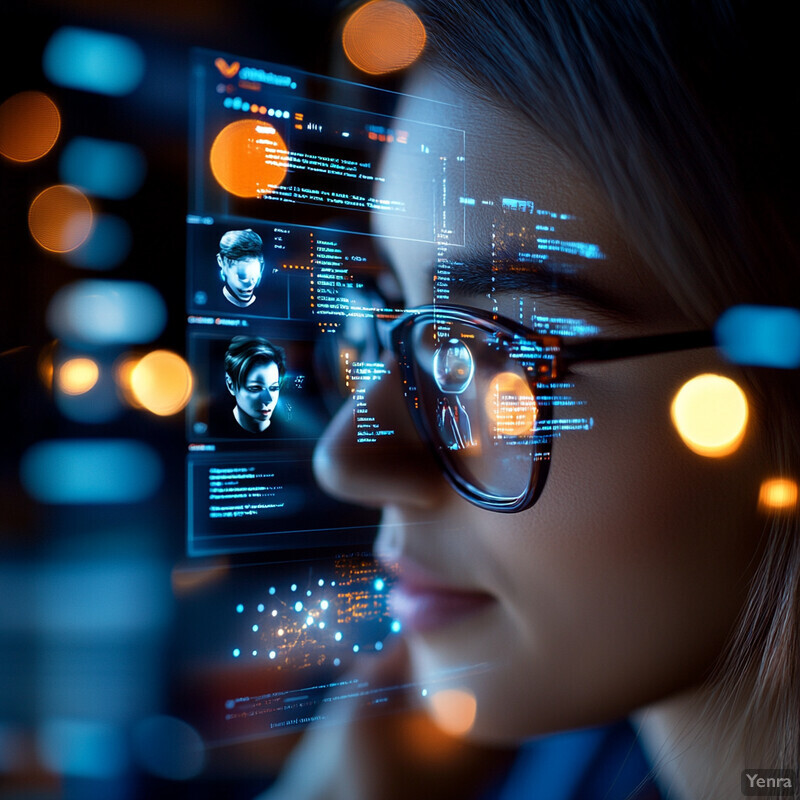
Individuals differ in their susceptibility to biases like loss aversion, mental accounting, or present bias. In behavioral finance, AI can analyze personal transaction histories, credit usage patterns, and stated preferences to model these individual differences precisely. This understanding helps financial advisors and institutions offer tailored advice—such as setting up automated savings plans for time-inconsistent savers or designing alerts for those prone to overconfident investment decisions. Personalization ensures that financial tools and services resonate with individual cognitive profiles, leading to better outcomes, greater trust, and a more stable financial system overall.
15. Incorporation of Neuroeconomic Data
Integrating brain imaging data, eye-tracking, or physiological measures into behavioral economics models, AI reveals how neurocognitive processes influence economic choices.
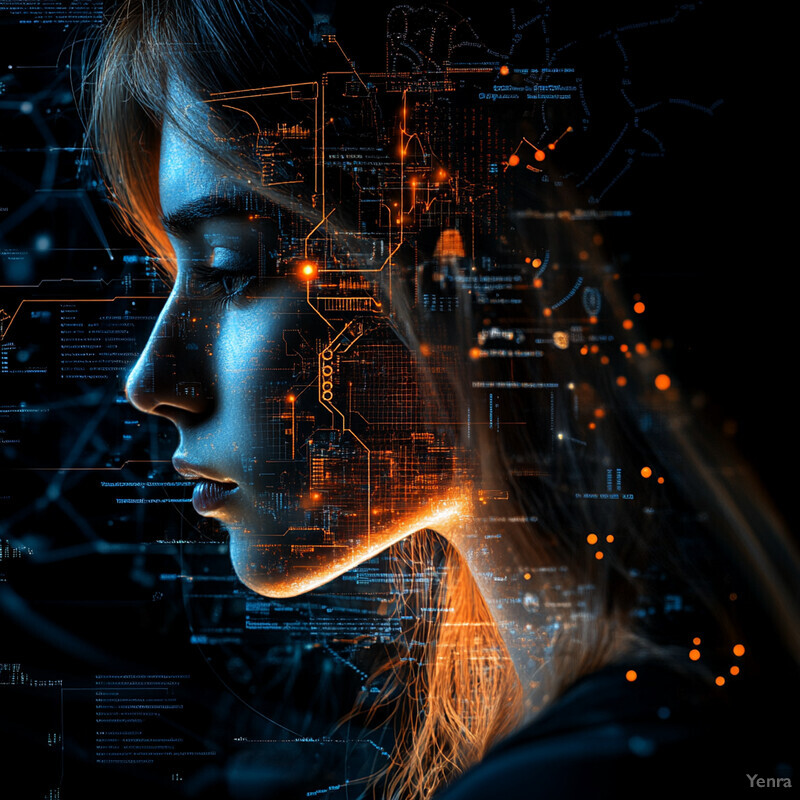
Neuroeconomics relies on physiological and neurological measurements—like fMRI scans, EEG readings, eye-tracking, and galvanic skin responses—to understand the biological underpinnings of decision-making. AI can process these high-dimensional, complex signals to uncover how neural activity correlates with specific economic choices or behavioral biases. Integrating these biological markers with traditional economic data deepens our understanding of the decision-making process at a fundamental level. By linking neural patterns to observed behaviors, researchers can validate existing theories or develop new ones that better explain why people deviate from rational norms, ultimately merging the fields of neuroscience, psychology, and economics into a unified framework.
16. Continuous Improvement Through Iterative Learning
As new data arrives, AI-driven models continuously refine their predictive power, ensuring behavioral economics models remain adaptively accurate and relevant.
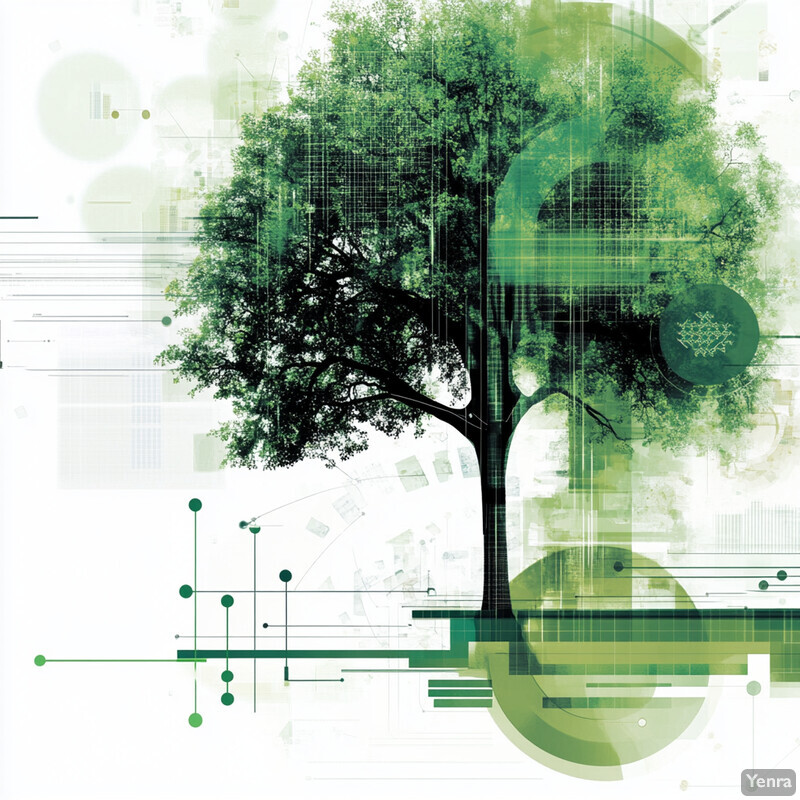
AI models are not static; they improve as new data is introduced, learning from fresh observations and changing conditions. When applied to behavioral economics, this means models stay up-to-date with evolving tastes, new market trends, or shifting social norms. For instance, consumer behavior following the introduction of a new technology can be continuously monitored and incorporated into predictive models. Over time, iterative learning refines theories, sharpens predictions, and ensures that policies and interventions remain effective in a changing world. This adaptability is a core strength of AI, ensuring that behavioral economic models do not stagnate but evolve alongside human behavior.
17. Scenario Analysis for Rare Events and Crises
AI models can explore multiple scenarios, including low-probability, high-impact events, helping understand how behavioral biases manifest under stress or uncertainty.
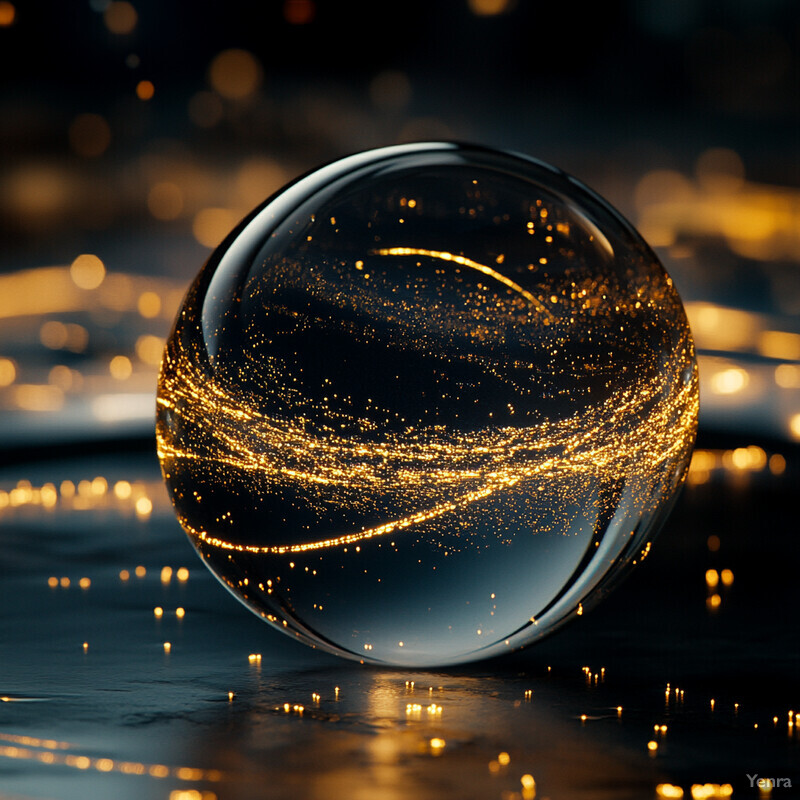
Economic crises, pandemics, or sudden regulatory changes often alter people’s behavior in unpredictable ways. AI models can generate scenario analyses that consider rare but impactful events, simulating how individuals and markets might respond to stressors. By blending behavioral assumptions—such as increased loss aversion in downturns or heightened herding tendencies during uncertainty—AI-driven scenarios can provide early warning indicators and stress-testing capabilities. Policymakers and businesses gain a toolkit to anticipate behavior in extreme contexts, improving resilience and strategic planning. This readiness can mitigate economic damage and guide supportive interventions during crises.
18. Bridging Micro- and Macro-Level Behaviors
Hierarchical AI models integrate individual-level behaviors with aggregate outcomes, deepening the understanding of how micro-level decision anomalies scale up to influence entire markets.
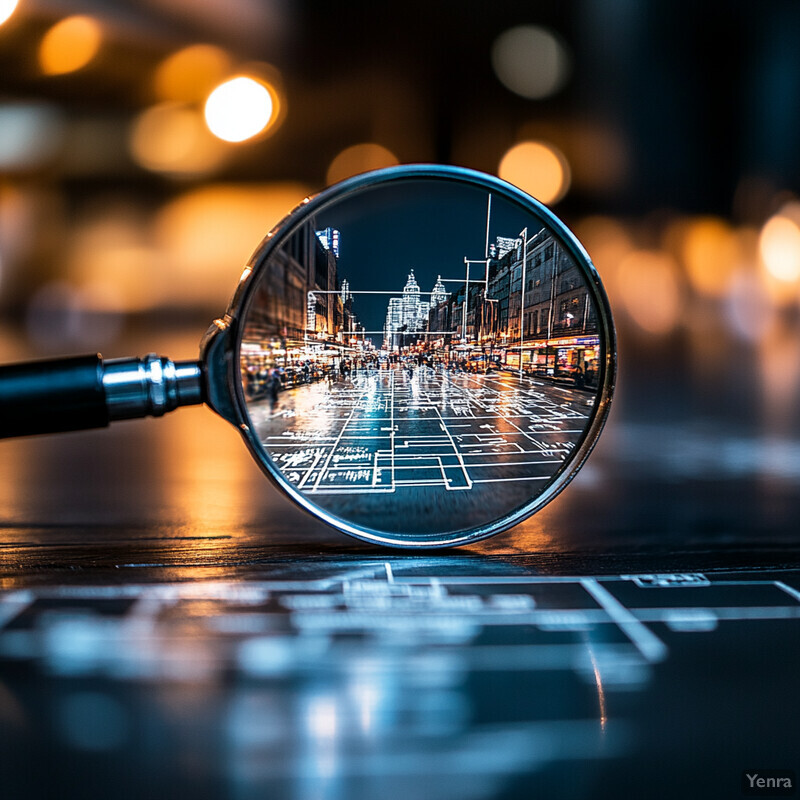
Behavioral economics often focuses on individual decision-making, while macroeconomics considers aggregate outcomes. AI can integrate these levels by modeling how micro-level behaviors scale up to influence market prices, unemployment rates, or overall economic growth. Hierarchical models can accommodate complex feedback loops: changes in aggregate indicators affect individual choices, which in turn shape macro-level conditions. By simulating the interplay between personal biases and systemic trends, researchers can identify leverage points for policy and understand how seemingly minor interventions at the micro level might yield significant macroeconomic effects.
19. Automatic Theory Generation and Hypothesis Testing
By identifying patterns in data not fully explained by existing theories, AI can propose novel hypotheses, leading to an evolving landscape of testable behavioral economic models.
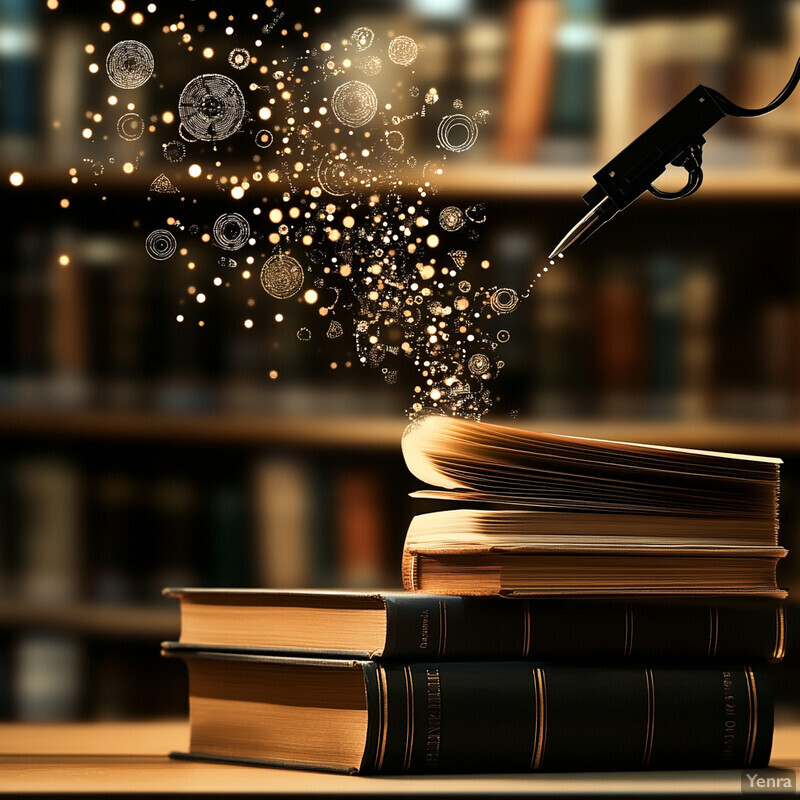
With the complexity of human behavior, it can be challenging to propose entirely new theories to explain observed patterns. AI can assist in this creative process by scanning large datasets and suggesting novel associations or mechanisms that have not yet been formally modeled. For example, AI might highlight that certain patterns in retirement savings mirror patterns in charitable donations, prompting theorists to investigate shared underlying cognitive principles. Once new hypotheses are generated, AI-based modeling and statistical tools can test them rapidly, refining theoretical frameworks at a pace previously unimaginable.
20. Exploration of Emergent Behaviors and Complex Dynamics
AI simulations reveal emergent behaviors and complex adaptive systems dynamics arising from interactions among agents with bounded rationality, uncovering patterns overlooked by traditional models.
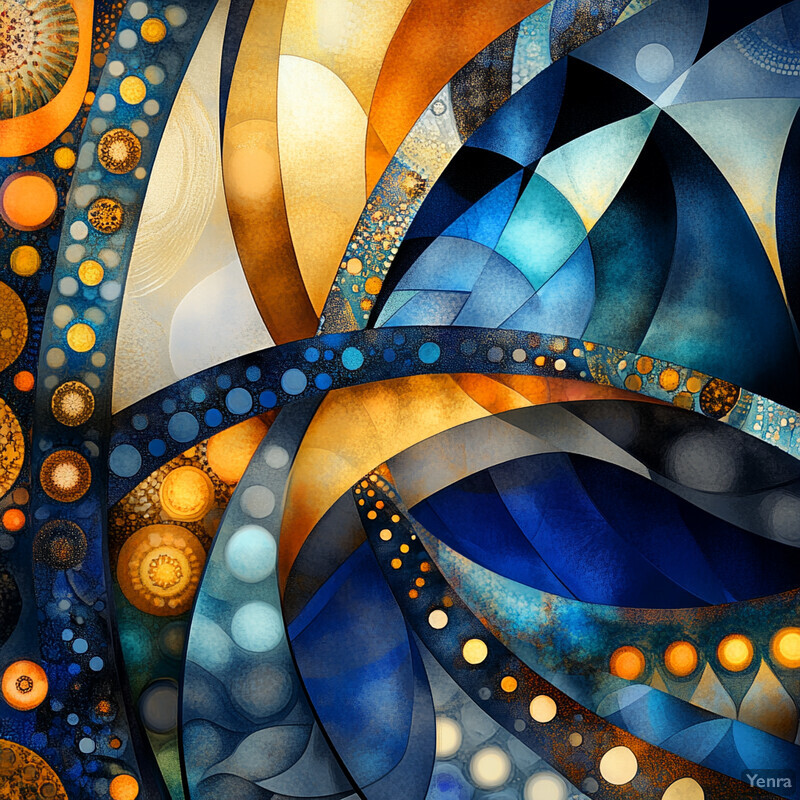
Some economic phenomena arise from the interplay of countless individual agents acting under imperfect information and evolving preferences. AI-driven simulations can expose emergent behaviors—such as self-reinforcing feedback loops, tipping points, or collective shifts in market sentiment—that were not clearly predictable from simple, static assumptions. By experimenting with different parameters and conditions, AI models reveal how small changes in incentives or information structures can produce large-scale effects. This exploration of complexity helps behavioral economics incorporate a richer understanding of dynamic systems, strengthening its capacity to explain and predict real-world economic outcomes.