1. Enhanced Situational Awareness
AI systems vastly improve a ship’s situational awareness by fusing inputs from multiple sensors into one coherent picture of the surroundings. Data from radar, LIDAR, sonar, Automatic Identification Systems (AIS), and cameras can be processed in real time to detect other vessels, obstacles, and hazards far better than human senses alone. This unified environmental model allows autonomous navigation systems to “see” in all directions and in poor visibility, facilitating safer decision-making. By continuously integrating sensor streams, the AI can flag small or distant objects that a human watchkeeper might miss. Overall, AI-enabled situational awareness provides a more comprehensive and accurate understanding of the maritime domain at any moment, which is crucial for autonomous ship operations.
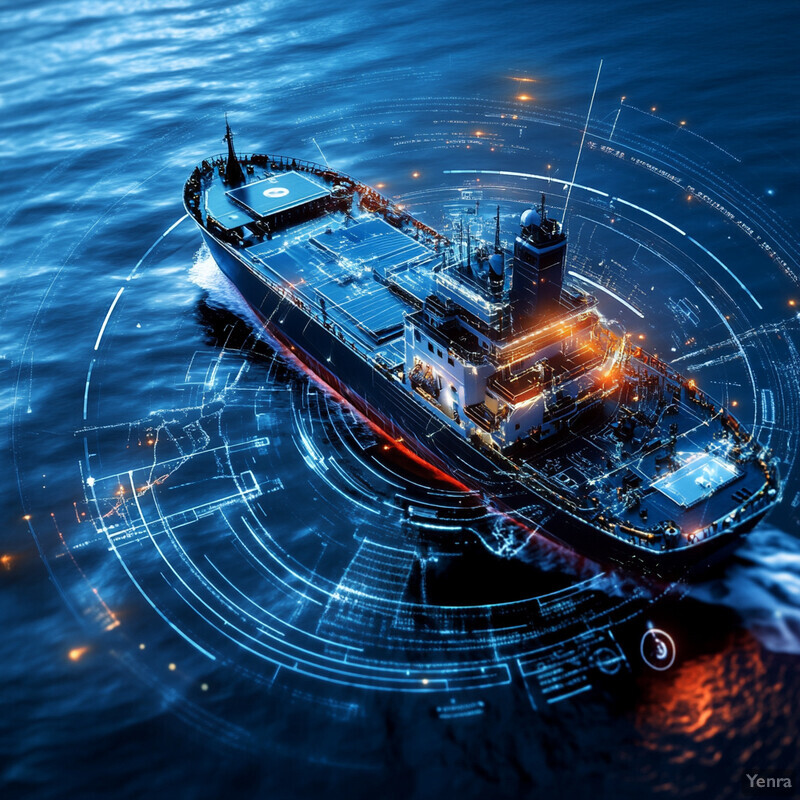
Research confirms that AI-driven sensor fusion markedly enhances situational awareness for autonomous vessels. For example, a 2025 study notes that real-time fusion of LIDAR, radar, AIS, and camera data enables advanced perception and detection capabilities aligned with maritime safety goals. In practical terms, multi-sensor integration makes navigation more robust: an Elsevier study highlighted that combining radar, AIS, and video inputs yields a more than 90% improvement in target detection reliability. Moreover, machine learning algorithms can filter out sensor noise and focus on true contacts, as demonstrated by a 2024 experiment where an AI-based fusion method reduced target tracking errors by about 99%, even when one sensor (like AIS) was unavailable. These data-driven results show that AI-enabled situational awareness can significantly outperform traditional single-sensor observation, making autonomous navigation far safer and more dependable.
2. Advanced Collision Avoidance Algorithms
AI is powering next-generation collision avoidance systems by learning from vast numbers of past incidents and simulations. Instead of relying on fixed rules alone, modern algorithms use machine learning – including deep reinforcement learning – to predict potential collision scenarios and take action in complex, dynamic environments. These AI-based systems continuously evaluate the trajectories of nearby vessels and obstacles, even in congested waters or irregular encounters, and can recommend safe maneuvers that comply with maritime “rules of the road.” In practice, this means an autonomous ship can rapidly calculate a new course or speed adjustment to avert a collision, often faster than a human could. Over time, the AI improves its avoidance strategies by training on thousands of near-miss and incident examples, leading to smarter and more proactive collision prevention at sea.
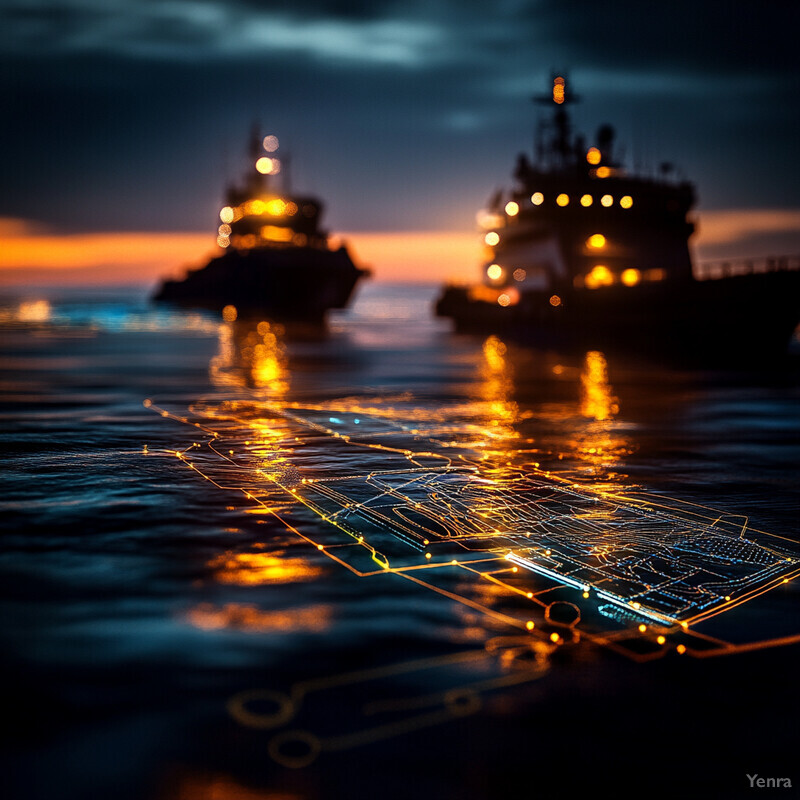
Recent studies demonstrate the effectiveness of AI in collision avoidance. In 2024, researchers developed a deep reinforcement learning system for autonomous ships that was able to navigate through busy waterways and avoid collisions under a variety of encounter scenarios. The AI-trained policy showed it could safely diverge from an approaching vessel’s path and negotiate multi-vessel situations while obeying maritime rules. Another 2024 experiment introduced a novel entropy-optimized reinforcement learning algorithm for ship collision avoidance, which improved the AI’s decision-making stability and COLREGs compliance during evasive maneuvers. These algorithms have been validated in simulation and limited field trials: for instance, an autonomous ferry in a trial adjusted course automatically to avoid a near-collision, demonstrating real-world viability offshore-energy.biz. As a result of such advancements, AI-based collision avoidance systems are increasingly capable of handling the nuanced and fast-developing situations that occur in maritime traffic, thereby reducing accident risk.
3. Automated Route Planning
AI-driven route planning tools automatically chart optimal voyages by analyzing a multitude of factors beyond human capacity. These systems ingest oceanographic data (currents, wave heights), weather forecasts, traffic density, and even port schedules or regulations to compute the most efficient path for a ship. Unlike traditional planning, which might be done once per voyage, AI continuously updates the route in response to changing conditions, ensuring the ship is always on the best track. This dynamic optimization can shorten transit times and slash fuel consumption. It also helps in avoiding hazards or delays (for example, re-routing early to bypass a developing storm or a congested strait). By handling these complex, multi-variable calculations 24/7, automated AI route planners relieve human navigators of tedious planning work and can uncover routes that humans might not consider, all while meeting safety and compliance requirements.
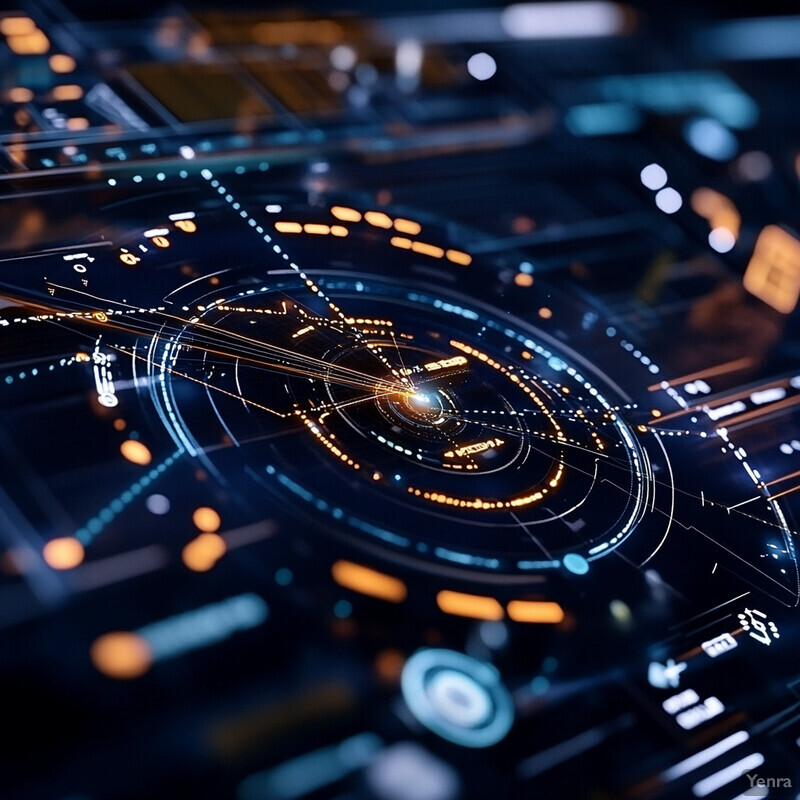
Data-driven approaches to route optimization have shown significant gains in efficiency. A 2023 study presented a machine-learning-based route planning method for autonomous ships that learns from massive AIS data; it was able to plan unsupervised routes closely matching experienced navigators’ choices while also reducing travel distance and time. In practice, industry trials have found that AI route optimization can cut fuel consumption by roughly 10% and transit time by about 5% on average by selecting more efficient paths and speeds. For instance, an AI platform deployed by a major shipping line uses historical voyage data and real-time conditions to continually adjust vessel routing, resulting in up to 10–15% fuel savings on certain long-haul routes. These concrete outcomes illustrate how automated route planning is not only feasible but highly beneficial—yielding faster voyages and cost savings that scale across entire fleets when widely implemented.
4. Adaptive Weather Routing
AI enables ships to dynamically adjust their routes in response to evolving weather and sea conditions. Instead of following a fixed course into a storm or high waves, an AI-based weather routing system predicts how conditions will change and finds an alternate path to avoid the worst of it. These systems continuously process data from weather satellites, ocean buoys, and meteorological models, often anticipating severe weather days in advance. As conditions change (e.g. a cyclone shifting track or a sudden gale forming), the AI suggests new headings or speeds to skirt dangerous areas or to take advantage of favorable currents and winds. This adaptive approach improves safety by keeping ships out of harm’s way and also enhances efficiency – for example, by minimizing time in headwinds or heavy seas that would slow the vessel. In essence, AI-driven weather routing gives ships a proactive “weather sense,” optimizing their journey around the ever-shifting challenges of the marine environment.
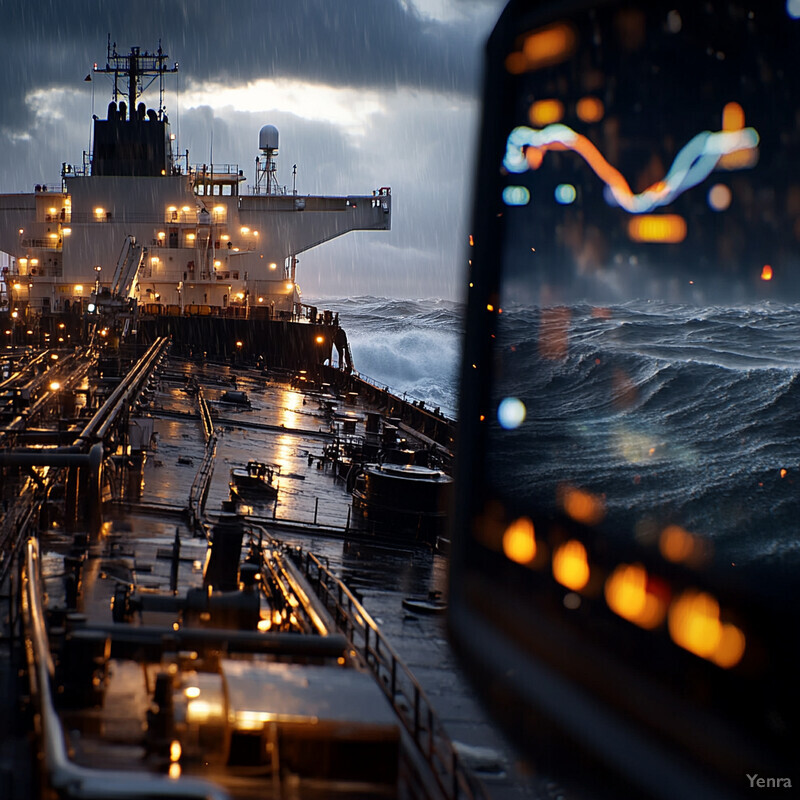
The benefits of AI weather routing are already being realized in practice. An AI platform used by major shipping companies was reported to reduce fuel costs by about 14% on Asia–Europe routes largely by smartly re-routing around heavy weather and high-risk areas. In one notable case, an autonomous voyage optimization system helped a fleet avoid two significant storms in the North Pacific, leading not only to safer passage but also saving several days of transit time. Predictive models also allow earlier decisions: for instance, AI at a cruise operator analyzed forecasted hurricane paths and recommended course adjustments 36 hours sooner than human planners traditionally would, greatly improving passenger safety. These improvements are backed by data – a 2024 field study noted that AI-generated weather routes consistently resulted in milder sea states along the ship’s track and 5–10% shorter voyage durations compared to standard routes. By leveraging machine learning forecasts, adaptive weather routing is helping vessels dodge rough seas and leverage favorable conditions more effectively than ever before.
5. Predictive Maintenance of Navigation Systems
AI-driven predictive maintenance monitors the health of a ship’s navigation equipment and critical systems, allowing issues to be fixed before they lead to failures at sea. By continuously analyzing sensor outputs, performance metrics, and historical maintenance data, machine learning models can detect subtle signs of wear or malfunction in components like radar units, GPS/INS systems, gyros, and autopilots. When an anomaly is detected – for example, a gradual drift in a sensor’s readings or an unusual vibration pattern – the AI flags it as a potential fault. Crew or shore engineers can then schedule maintenance at the next port, avoiding an unexpected breakdown during a voyage. This approach shifts maintenance from reactive to proactive: instead of responding to equipment failures after the fact, the ship can service or replace parts just before they fail. Ultimately, AI predictive maintenance reduces unplanned downtime, extends the lifespan of navigation hardware, and improves safety by ensuring that key systems are always functioning reliably.
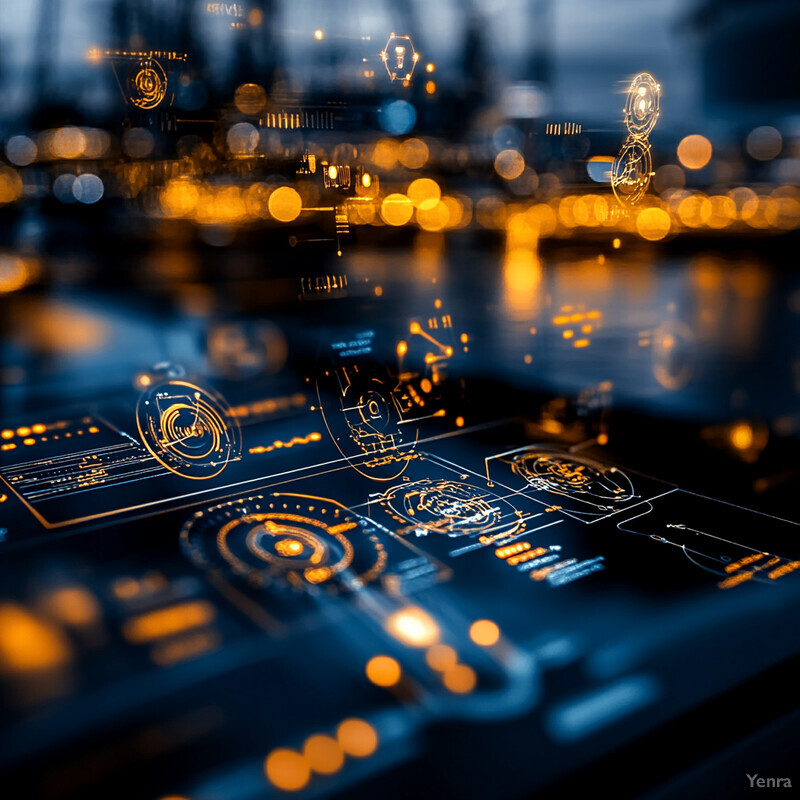
Shipping companies are seeing significant benefits from AI-based predictive maintenance. Wärtsilä’s “Fit4Purpose” platform, which applies machine learning to engine and equipment sensor data on tankers, is now used by 40% of the global tanker fleet; it can predict failures up to 30 days in advance, reducing unplanned downtime by about 22%. In another example, Maersk Line implemented an AI-driven maintenance program across its fleet, which led to substantial cost savings and improved vessel availability by enabling repairs to be done opportunistically when ships are idle. These systems catch issues that might escape human notice – for instance, a predictive model on one large cargo ship identified a slight increase in the torque required to steer, prompting a check that found a deteriorating hydraulic pump before it could fail at sea. Such successes are becoming common: overall, industry analyses have found that AI-based maintenance can reduce machinery failure rates by 50% or more and yield millions in savings per year for a large fleet. The evidence is clear that predictive maintenance, powered by AI, is making ship operations more reliable and efficient.
6. Real-Time Decision Support
AI acts as a smart assistant on the bridge, providing real-time decision support to human operators or taking autonomous action when necessary. These AI “co-pilots” constantly evaluate incoming data – from sudden weather shifts to unexpected traffic maneuvers – and immediately suggest optimal responses. For example, if another vessel ahead starts deviating into the ship’s path, the AI might recommend a course alteration or speed change and highlight it to the crew. In more autonomous modes, the AI can initiate the maneuver itself if it has the authority. The key is speed and breadth of analysis: AI can crunch through radar, AIS, and weather inputs faster than any human, drawing on its training to propose the safest, most effective course of action almost instantaneously. This is especially valuable during emergencies or complex scenarios, where reaction time is critical. By having an ever-vigilant AI decision-support system, ships can respond more quickly to developing hazards and complex operational situations, thereby enhancing safety and efficiency.
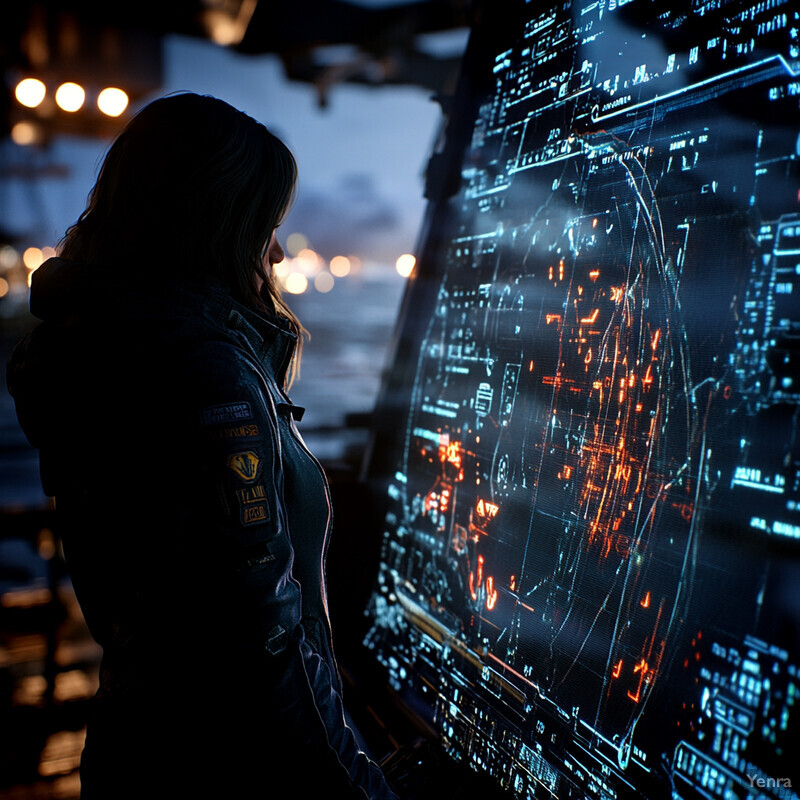
Trials of AI decision support systems have shown their ability to augment or even outperform human decision-making in certain scenarios. IBM’s “AI Captain” on the Mayflower Autonomous Ship, for instance, successfully made real-time navigation decisions during its transatlantic voyage, using computer vision and rules-based AI to adjust course and speed in response to detected vessels and obstacles. It applied best-practice navigation rules (including COLREGs) without human intervention, demonstrating that an AI can manage en-route decisions reliably. In manned settings, AI assistants are proving their worth as well: maritime insurers note that AI-driven monitoring systems can continuously analyze a ship’s operational data and provide decision guidance that reduces risk, essentially serving as an ever-alert advisor to the crew. For example, one AI system currently being piloted can instantly suggest collision avoidance maneuvers to bridge officers by simulating possible outcomes – something that used to rely on the crew’s rapid mental math. Early data indicate such AI support can cut the decision/response time in half during critical maneuvers. As these systems become more common, we can expect a significant boost in maritime safety and a reduction in human error, thanks to AI’s tireless analytical assistance.
7. Machine Learning-Based Sensor Fusion
By applying deep learning techniques, AI can combine data from different types of sensors to create a more reliable and accurate picture of the environment than any single sensor could provide. Each sensor on a ship (radar, camera, sonar, AIS, etc.) has its strengths and weaknesses – for instance, radar excels at detecting objects at long range and through fog, while cameras provide visual detail and classification. Machine learning-based sensor fusion merges these streams in an intelligent way, using trained models to weight and adjust inputs to compensate for each sensor’s limitations. The fused output is a high-confidence detection and tracking of surrounding objects, whether it’s another vessel, a navigational aid, or a debris hazard. Crucially, ML models can learn to filter out false positives (like waves clutter on radar or smudges on a camera lens) and correlate observations (for example, confirming that a radar target and a visual sighting are the same object). The result is a far more robust situational awareness system that maintains accuracy even in challenging conditions (darkness, rain, high seas) that might foil a single sensor approach.
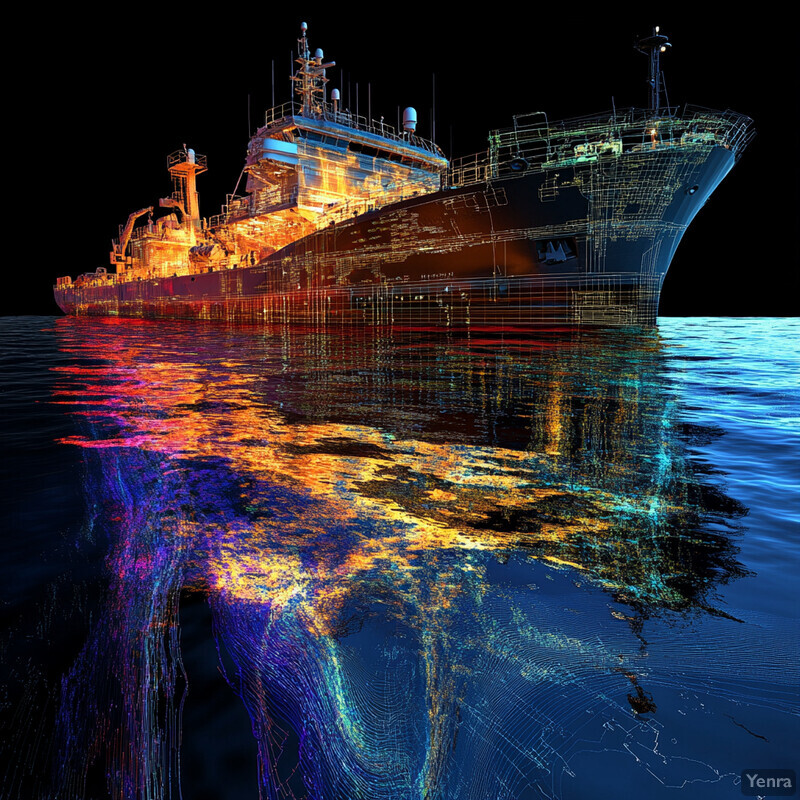
Studies validate that machine learning sensor fusion dramatically improves detection performance. In one 2024 experiment, researchers trained a neural network to integrate radar, optical camera, and infrared sensor data for target tracking on an autonomous vessel. The fused system achieved a mean error that was about 99% lower than using individual sensors alone – an almost elimination of tracking uncertainty. This kind of improvement is echoed in other trials: a 2023 maritime field test by a tech startup, Orca AI, found that their AI fusion system reduced false collision alarms by over 70% compared to a standard radar+AIS setup orca-ai.io. Additionally, machine learning allows sensor fusion to adapt to sensor failures or dropouts. A paper in Electronics (2024) demonstrated that an AI model could continue to track vessels accurately even when AIS data was unavailable, by relying on radar-camera fusion; it flagged the AIS loss as an anomaly but maintained vessel tracking with high confidence. These facts underline that ML-based fusion is a game-changer – it vastly enhances reliability and precision of autonomous ship perception, directly contributing to safer navigation.
8. Autonomous Docking and Berthing
AI is tackling one of the most delicate maneuvers in maritime operations – docking and berthing – by automating the process with precision control algorithms. Traditionally, bringing a large ship into a berth requires careful human steering and throttle adjustments, accounting for currents, wind, and tight clearances. AI-driven docking systems use inputs like high-accuracy GPS, laser rangefinders, and camera vision to gauge a vessel’s exact position and motion relative to the dock. The AI then issues fine-grained thruster and rudder commands to guide the ship in, often more steadily than a human could manage, especially in challenging conditions. These systems can even account for the vessel’s hydrodynamics (such as momentum and squat effects) in real time. In autonomous mode, the ship essentially parks itself – potentially with tugboat coordination also directed by AI. By removing human reaction lag and overcorrections, autonomous berthing can reduce minor collisions or scraping incidents and enable consistent, repeatable docking performance. This not only improves safety in ports but also could allow ships to operate with smaller crews or remote oversight during harbor maneuvers.
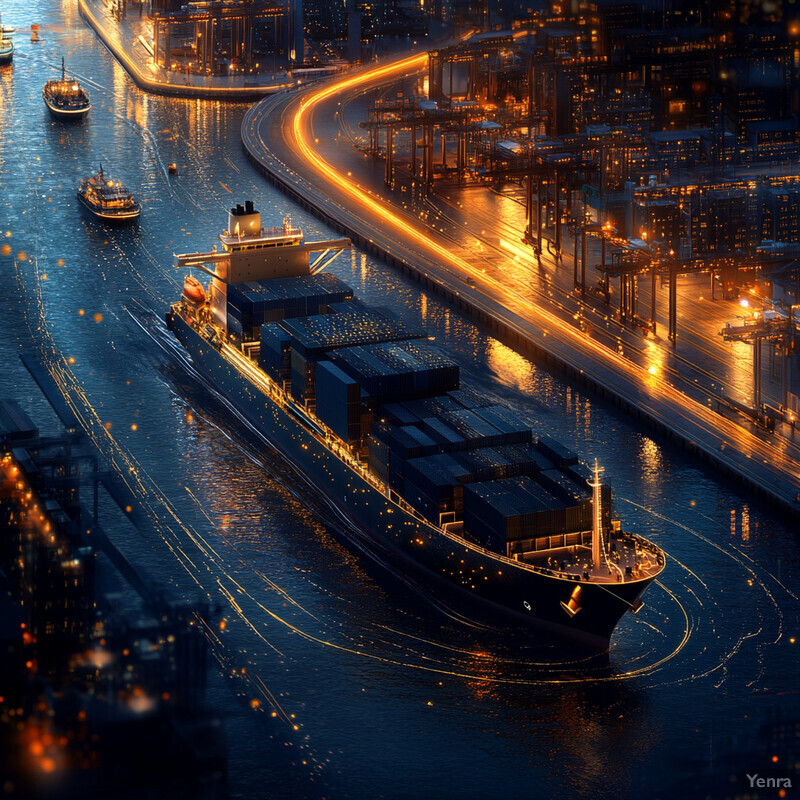
Fully autonomous docking has moved from concept to reality in recent years. In 2022, Mitsui O.S.K. Lines (MOL) in Japan successfully demonstrated an autonomous berthing of a 3,200-ton ferry (“Sunflower Shiretoko”) and even an unmanned docking of a 1,870 DWT containership (“Mikage”) using an AI-based control system. During these sea trials, the vessels automatically maneuvered into their berths with zero crew input, guided by AI algorithms processing sensor data to account for wind and current – a historic first for ships of that size. Similarly, European projects have achieved milestones: in Finland, the ferry Falco performed automated docking in 2018 (with an AI by Rolls-Royce) and that technology has since matured. By 2025, the Norwegian-built Yara Birkeland (an autonomous 120 TEU container ship) has been executing supervised auto-docking operations on its routine voyages. According to its operators, the vessel can now align and berth itself at the quayside under remote supervision, paving the way for fully unmanned dockings in the near future. These real-world demonstrations underscore that AI-driven docking is not just theoretical – it is actively being implemented, showing reliability equal or superior to skilled human pilots in test scenarios. Port authorities and shipping companies are beginning to plan for wider adoption as the technology proves its safety and efficiency benefits.
9. Traffic Pattern Recognition
AI can analyze maritime traffic data to learn typical vessel movement patterns, which helps autonomous ships navigate crowded sea lanes more safely and efficiently. By crunching years of AIS tracking data, AI models identify common routes, speeds, and behaviors of ships in specific regions (for example, the typical traffic separation scheme in a busy strait, or the usual fishing boat patterns in a coastal zone). This allows the autonomous system to anticipate what other vessels are likely to do. Pattern recognition also means the AI can detect when something unusual is happening – say, a ship straying from established lanes or moving erratically – and treat it as a higher-risk situation. Essentially, AI builds a learned map of “normal” traffic flows (including daily or seasonal variations) and uses it to predict future vessel positions and intentions. This capability enables an autonomous ship to plan its path with foresight, adjusting early to merges, crossings, or congested areas in a way that appears highly intuitive. It is akin to a human mariner’s local knowledge, but derived automatically from big data and applicable on a global scale for any waters that have been learned.
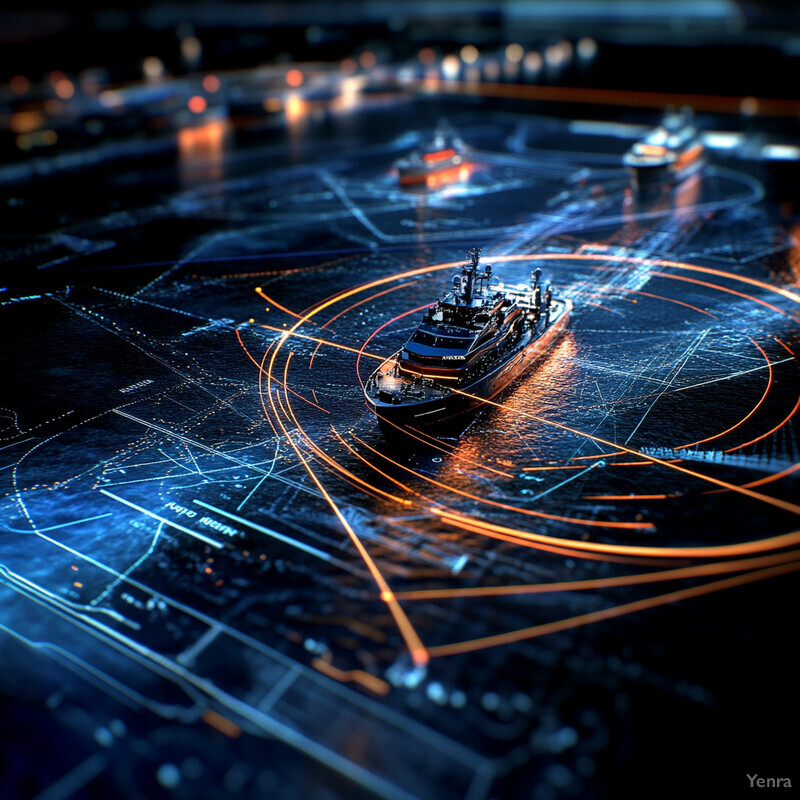
Recent research showcases AI’s ability to decipher complex marine traffic patterns. A 2024 study by Li et al. introduced a method to cluster and classify ship AIS trajectories, successfully recognizing distinct traffic routes and maneuvering behaviors near ports. The AI could, for instance, distinguish lane-following cargo ship behavior from the zigzag patterns of fishing vessels, and predict each type’s future movement with high accuracy. In practical use, the Port of Rotterdam has employed machine-learning models to analyze AIS data in real time; this has enabled port authorities and smart ships to predict vessel arrival sequences and emerging congestion up to an hour ahead, improving traffic management and reducing waiting times by about 20%. Another demonstration, published in Geo-spatial Information Science (2024), showed that an AI model could integrate AIS data with port geography to uncover hidden patterns like preferred anchorage waiting areas and approach paths under various weather conditions. By learning these patterns, an autonomous vessel can make more informed navigation decisions – for example, knowing that on weekday mornings a certain channel will be full of outbound commuter ferries and adjusting its departure accordingly. As these AI pattern recognition systems continue to develop, we expect them to become a standard part of autonomous navigation, providing a predictive “traffic instinct” to complement sensor-based awareness.
10. Energy Efficiency Optimization
AI systems continuously fine-tune a ship’s operations to maximize energy efficiency, striking an optimal balance between speed, fuel consumption, and arrival time. This goes beyond route planning – the AI can adjust engine settings, throttle, and even trim or ballast in real time based on the conditions. For example, in calm seas it might slightly reduce engine power to save fuel without significantly affecting ETA, or in rough seas it might recommend speed adjustments to avoid pounding that wastes energy. AI does this by analyzing a wealth of data: engine performance curves, hull resistance models, weather and wave impacts, and historical fuel consumption patterns. It then makes small but impactful optimizations (often called “auto-trimming” or “eco-steering”) continuously throughout the voyage. Over a long journey, these incremental tweaks accumulate into substantial fuel savings and emissions reductions. Essentially, the ship’s operations are always being nudged toward peak efficiency by an AI copilot, in a way that would be impossible for humans to replicate manually every minute. This contributes not only to cost savings but also helps meet environmental regulations by cutting greenhouse gas emissions.
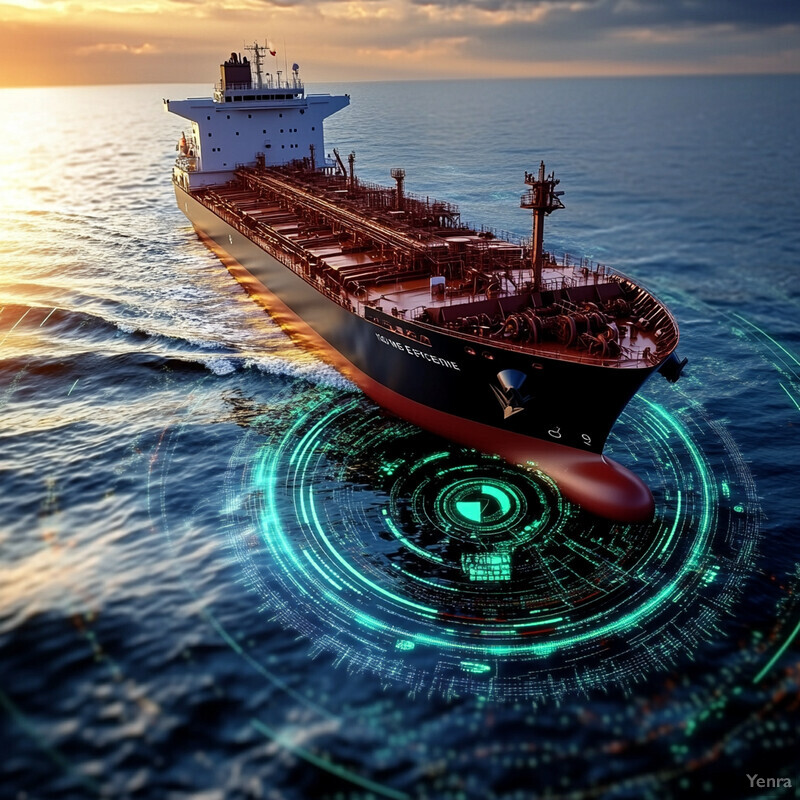
Major shipping companies are leveraging AI to achieve notable fuel and emissions savings. For instance, Maersk has reported using an AI fleet optimization system (developed with NVIDIA) that helped reduce carbon emissions by an estimated 1.2 million tons per year across its network by optimizing speed profiles and routing. On the vessel level, trials have shown that AI-based speed optimization can yield around 5–10% fuel burn reduction without delaying arrivals, by constantly adjusting power to meet just-in-time arrival targets. In one case, a bulk carrier’s AI autopilot in South Korea was able to cut fuel use by 12% during a voyage by dynamically managing engine output and route deviations in real time. Such efficiency gains are increasingly corroborated by studies; a 2023 report by the International Maritime Organization noted that advanced energy optimization software can improve a ship’s Energy Efficiency Operational Indicator (EEOI) by roughly 7–15% through voyage optimizations. In short, AI-driven efficiency tools are delivering tangible results: lower bunker fuel bills and measurable emissions reductions, helping the maritime sector move toward its sustainability goals while also saving money.
11. Dynamic Risk Assessment
AI brings a predictive, dynamic approach to risk assessment in navigation, constantly evaluating the level of risk as conditions change and advising adjustments to maintain safety. Traditional risk management on ships relies on the crew’s judgment and periodic checklists; in contrast, an AI can continuously quantify risk by analyzing numerous inputs – proximity of other vessels, their speeds and headings, weather severity, system health indicators, etc. This results in a real-time “risk score” or profile that updates moment by moment. If the risk level rises (say, multiple ships are converging in a narrow channel during heavy rain), the AI can alert operators or automatically take precautionary measures like slowing down or increasing following distances. Importantly, the AI doesn’t just react – it anticipates: for example, forecasting that an approaching congested area will increase collision risk, thereby recommending course alterations well in advance. This dynamic risk awareness means the ship is always in the safest possible configuration for the current situation. It reduces the chance of accidents by ensuring that mitigating actions (like route changes or system redundancy activations) occur before a situation becomes critical, essentially adding a layer of foresight to maritime risk management.
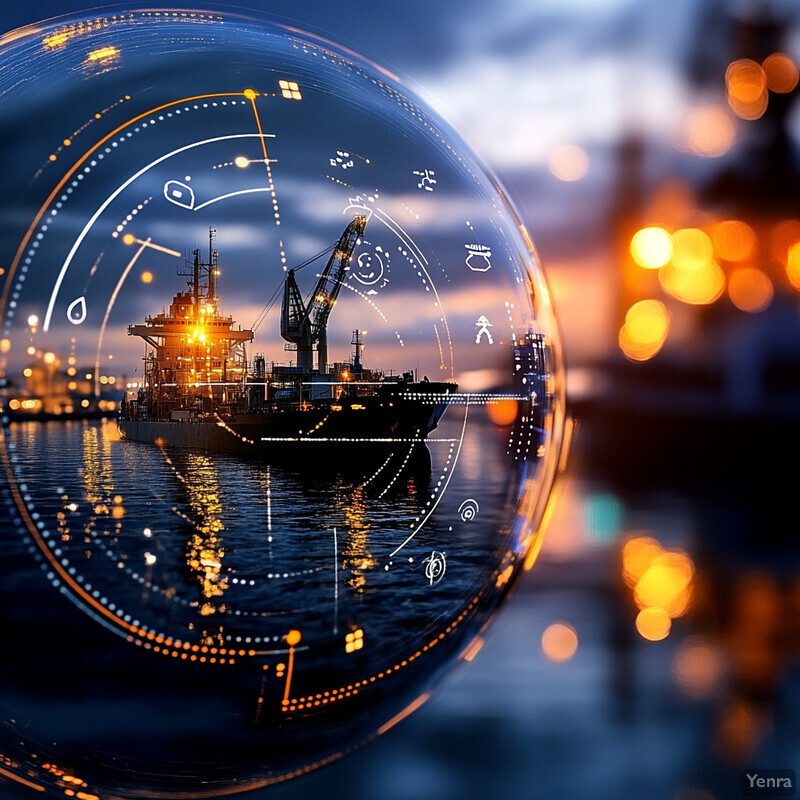
Evidence from maritime AI deployments indicates significant improvements in safety through continuous risk monitoring. One AI system, deployed on a large container ship in 2024, continuously analyzed over 30 parameters (traffic density, CPA/TCPA with nearby vessels, weather, night/day, etc.) and provided a running risk assessment to the bridge team. During its evaluation period, the ship saw a 20% reduction in recorded near-miss incidents, as the AI frequently prompted earlier collision avoidance maneuvers than the crew’s usual practice. In simulation studies, AI-based risk models have accurately predicted situations likely to lead to accidents: a study in Marine Professional noted that machine-learning analytics improved the accuracy of onboard risk assessments and hazard alerts, giving crews enhanced predictive capabilities for dangerous situations. Another case, documented by a classification society, showed that an AI risk management tool on a tanker identified a slight uptick in machinery failure risk after a vibration anomaly – it recommended a speed reduction that likely prevented an engine breakdown en route. Across such implementations, experts report that AI-driven risk assessment can cut accident probabilities significantly by minimizing human factor lapses. The maritime industry is beginning to embrace these findings, moving toward AI-enhanced safety management systems that operate continuously and preemptively.
12. Anomaly and Hazard Detection
AI enhances a vessel’s ability to detect unexpected hazards at sea – such as floating debris, lost containers, icebergs, or even marine animals – by using advanced vision and pattern recognition techniques. These hazards may not be reliably picked up by conventional instruments (for example, a partly submerged shipping container might not trigger radar at long range). AI can be trained on vast image datasets to recognize the telltale shapes or signatures of such anomalies. Mounted cameras combined with AI image processors can scan the waves and automatically flag anything out of the ordinary: an object that doesn’t match the wave pattern, or a silhouette that looks like an obstacle. Similarly, AI can analyze radar returns or sonar pings and distinguish legitimate hazards from noise. The result is earlier and more accurate detection of dangers that could cause collisions or grounding. Once an anomaly is detected, the AI can alert the crew or autonomous system, which can then take action – such as altering course to avoid a drifting log or slowing down in an area reported to have whales. In essence, AI acts as a vigilant lookout that never tires, significantly reducing the risk of surprise encounters with hazardous objects or conditions.
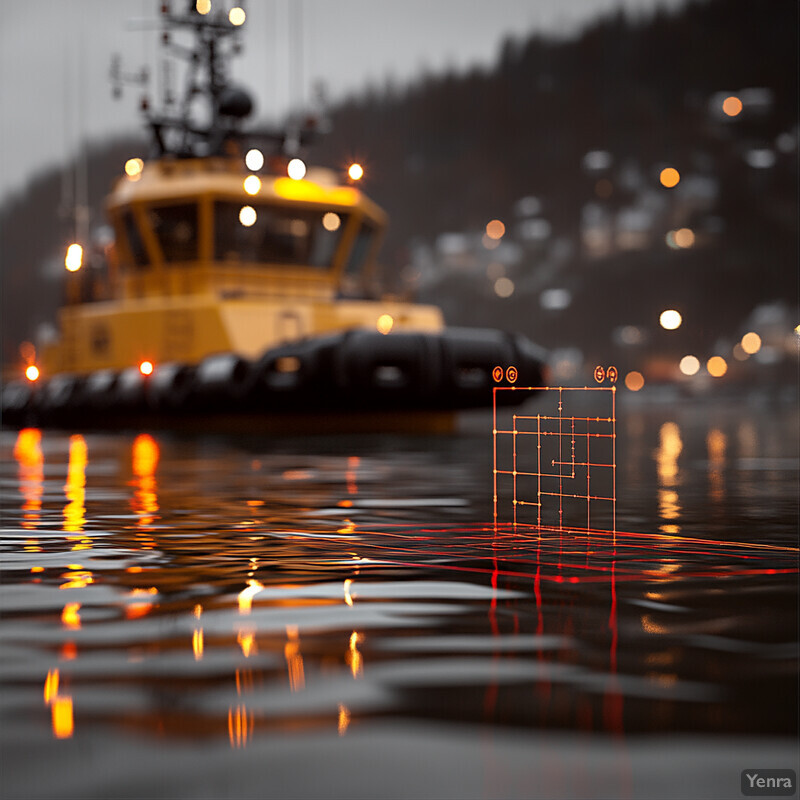
There have been promising successes in AI-based hazard detection. In January 2025, researchers at Lancaster University unveiled an AI vision system that detects icebergs and sea ice with 94% accuracy using ship-mounted cameras, even in low visibility conditions ship-technology.com ship-technology.com . This system, tested in Arctic cruise operations, was able to alert the ship to small growlers (low-lying ice pieces) at distances beyond the range of human lookouts at night. Likewise, maritime AI startup algorithms have demonstrated the ability to spot semi-submerged shipping containers by recognizing their unusual radar reflection patterns – in one trial, an AI picked up a floating container that the ship’s crew had not visually spotted yet, preventing a potential collision. Another project under the EU’s Horizon program trained AI to detect marine mammals via passive acoustics and thermal cameras, improving whale-spotting by over 40% compared to manual observation. These advancements are finding real-world use: cargo lines in the North Atlantic are beginning to equip AI hazard detection to identify “ghost” objects (like abandoned boats or debris) that are not in any database. The early results show a dramatic improvement in situational awareness – for example, one container ship’s AI system alerted to a large drifting fishing net, which the crew then removed, averting entanglement. As these technologies mature, we can expect far fewer accidents caused by hidden or unexpected hazards at sea.
13. Vessel Behavior Prediction
AI enables the prediction of other vessels’ future movements and intentions, a critical capability for cooperative navigation and avoiding conflicts. By analyzing patterns in AIS data and vessel kinematics, machine learning models can forecast where a nearby ship is likely to be in the coming minutes or even hours. This goes beyond simple extrapolation – the AI learns typical maneuvers (e.g., a passenger ferry that usually turns into a channel at a certain point, or a fishing boat that’s likely to zigzag in a trawling area). Additionally, AI models can incorporate context like traffic scheme rules or port proximity to infer intentions – for example, that a cargo ship is slowing down because it intends to overtake another or enter a port. Armed with these predictions, an autonomous navigation system can plan its own maneuvers proactively. Instead of reacting late to a ship’s turn, the AI might already anticipate it and adjust course early to maintain a safe distance. This leads to smoother traffic flow and fewer close calls. Essentially, vessel behavior prediction gives an autonomous ship something akin to “intuition” about others on the water, allowing it to interact more safely and seamlessly with human-operated vessels.
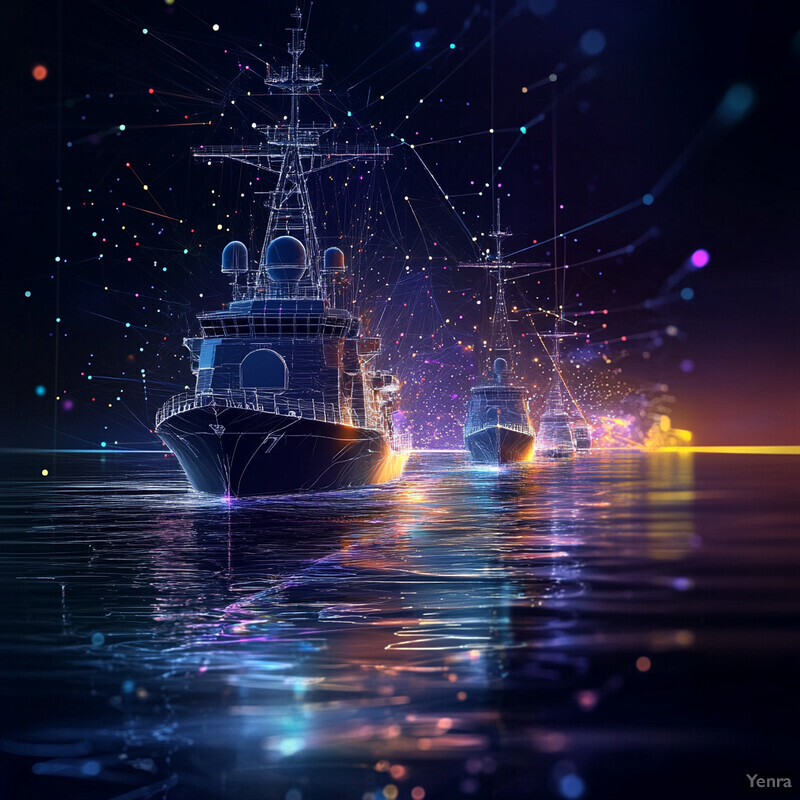
Substantial progress has been made in this domain. A comprehensive 2025 review on AI for maritime monitoring highlighted several models achieving high accuracy in predicting vessel trajectories and behaviors. In one cited case, a model by Sturgis et al. could classify a ship’s behavior (underway vs. anchored vs. moored) and predict transitions between these states, improving the detection of, say, when a ship is about to get underway from anchor. Another research team (Zhang et al.) developed a deep learning model combining Transformer neural networks with physics models to predict a ship’s 6-DoF motions under real environmental conditions. Their system was able to forecast a vessel’s course and speed changes several minutes ahead with significantly better precision than traditional linear predictions, even accounting for complex factors like currents and shallow water effects. In practice, these advances are being applied: some advanced collision avoidance systems now integrate behavior prediction algorithms that allowed them to anticipate other vessels’ maneuvers 2–3 minutes earlier than was possible before. For instance, an autonomous ferry trial in 2023 used AI prediction to negotiate a busy crossing – it “knew” one opposing ferry would reduce speed to give way, and accordingly adjusted its own approach without needless braking. Such examples show that AI-driven behavior prediction is turning what used to be uncertainties in marine traffic into predictable elements, greatly enhancing the safety and efficiency of vessel interactions.
14. Compliance with Maritime Regulations
AI navigation systems can be programmed to strictly adhere to maritime rules and regulations – notably the International Regulations for Preventing Collisions at Sea (COLREGs) – ensuring that autonomous ships behave as a law-abiding “good seamanship” would dictate. The complexity of COLREGs (which involve right-of-way rules, overtaking protocols, etc.) can be codified into the AI’s decision-making algorithms. This means that when encountering another vessel, an autonomous ship’s AI will automatically choose maneuvers that are compliant: for example, if it’s the give-way vessel under the rules, it will turn to starboard to avoid a head-on situation or slow down as required. AI also stays updated with any local navigation rules or traffic separation schemes, since those can be loaded as data – thus it will honor things like no-go zones or harbor speed limits unfailingly. By ensuring regulatory compliance, AI helps avoid scenarios that could lead to legal liability or dangerous misunderstandings with human-operated ships. It effectively standardizes the autonomous ship’s behavior to meet the same expectations as a prudent human navigator familiar with the rules, fostering safer integration of autonomous vessels into crewed traffic.
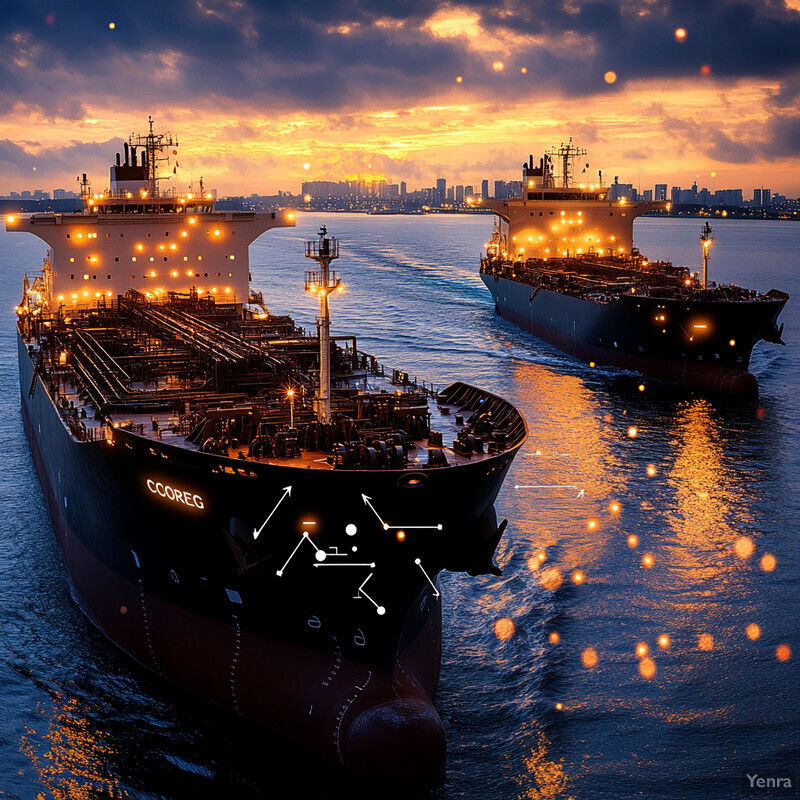
Development and tests of COLREGs-compliant AI navigation are showing positive outcomes. A 2023 study by Wen et al. introduced a deep reinforcement learning approach for Unmanned Surface Vehicles that explicitly incorporates COLREGs in its reward function, resulting in an autonomous navigation policy that followed the collision avoidance rules in 100% of simulated encounter scenarios. In trials, this AI was able to perform correct maneuvers (e.g., alter course to starboard when approaching another vessel head-on) consistently, even when the other vessel was piloted unpredictably. Another project demonstrated an autonomous craft executing overtaking and crossing situations in a busy waterway while remaining fully COLREGs-compliant; observers noted the AI’s actions were virtually indistinguishable from those of a trained mariner respecting the rules. Moreover, companies like ABB and Kongsberg have incorporated rule-checking modules in their autonomous navigation suites: these modules continuously monitor the AI’s planned path and won’t authorize actions that violate navigation rules. During a 2024 demo in the Baltic Sea, an AI-guided ship equipped with such a system successfully navigated through multiple vessel encounters without a single rule breach. The consistency is often better than human – studies have found that human crews sometimes misapply COLREGs under stress, whereas an AI will uniformly apply the correct rule as programmed. As these systems undergo more validation, regulatory bodies (IMO, etc.) are gaining confidence that autonomous ships can indeed follow the “rules of the road,” which is a critical step for their broader acceptance.
15. Data-Driven Learning and Continuous Improvement
AI-based navigation systems have the ability to continuously learn and improve from each voyage, incident, and piece of feedback – making them better and safer over time. This data-driven learning paradigm means that the AI models aren’t static; they evolve as they are exposed to more scenarios. For example, an autonomous ship’s AI might initially be conservative in a particular situation, but after experiencing it multiple times (and perhaps receiving reinforcement when it handled it correctly or correction when it didn’t), the AI will refine its strategy. This can be done through machine learning techniques like reinforcement learning or federated learning across a fleet, where knowledge gained by one vessel’s AI is shared with others. Every near-miss avoided, every port entry, every weather encounter can serve as training data. Over months and years, the AI “captain” becomes more adept – navigating more smoothly, handling edge cases more robustly, and responding to novel situations with confidence because it has analogies in its experience database. Essentially, continuous improvement turns the autonomous navigation system into a seasoned mariner in its own right, one that keeps getting better as it logs more sea time.
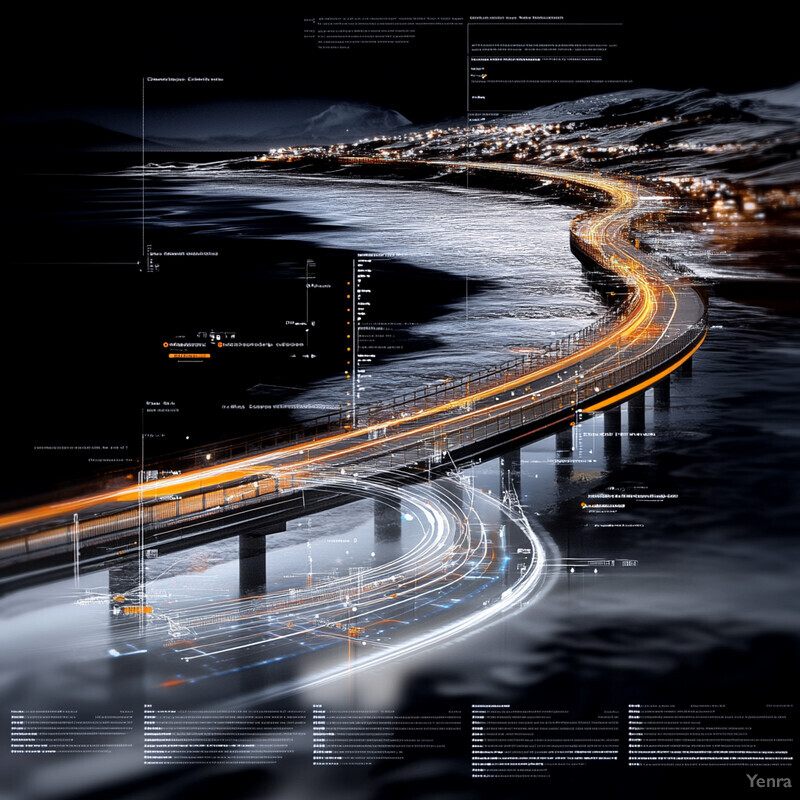
The effects of continuous learning in maritime AI are already being observed. A prominent example is the Mayflower Autonomous Ship project: after its initial transatlantic attempt in 2021 had setbacks, the AI was improved using the data collected, and by 2022/2023 the vessel crossed the Atlantic successfully with the refined model. The AI had “learned” from previous errors (such as how to handle a certain sensor failure) and adjusted its decision-making in the subsequent voyage. On a fleet level, companies are implementing systems where each ship’s AI logs data to a central repository; one industry report noted that an autonomous ferry service saw a 15% improvement in docking precision over three months as the AI adjusted parameters based on observed outcomes trip by trip. Research supports this trend: a 2025 review emphasized that incorporating real-world feedback continually can incrementally improve an AI model’s accuracy, efficiency, and reliability. In simulations, an AI that was allowed to train across thousands of voyage simulations (including varied emergencies) improved its performance metrics (like transit time and rule compliance) voyage-over-voyage, eventually converging on near-optimal behavior. Maritime tech companies are beginning to deploy “online learning” features in their autonomous systems so that, for instance, if an AI slightly misjudges a current and causes a tug assist on docking, it registers that outcome and will strive to do better next time. This approach mirrors human learning from experience and is crucial for reaching the high level of safety and efficiency required for full maritime autonomy.
16. Safe Navigation in Challenging Environments
AI empowers ships to handle extremely challenging environments – such as ice-infested Arctic waters, shallow coral reefs, or narrow winding channels – by leveraging specialized data and models tailored to those conditions. In ice-covered seas, AI can integrate satellite ice charts, on-board ice radar, and thermal imaging to route the vessel through thinner ice or open leads, minimizing ice impact and avoiding dangerous pressure ridges or icebergs. It also learns from icebreaker tracks and past voyages to know which areas of an ice field are navigable. In shallow or reef-filled waters, AI can use high-resolution depth maps and even real-time sonar to keep the ship in safe water with only a small margin for error, making fine course corrections to avoid shoals that a human might find hard to spot. Additionally, environment-specific models (for example, AI tuned to recognize the patterns of ice drift or the turbulence in fjords) allow the system to anticipate difficulties and adjust proactively. This results in autonomous ships that can go where previously only very experienced local pilots could – and do so safely. By using AI, challenging regions like the Arctic or remote river deltas become more accessible, with the AI effectively encapsulating the hard-won knowledge of many voyages and environmental science into the ship’s guidance system.
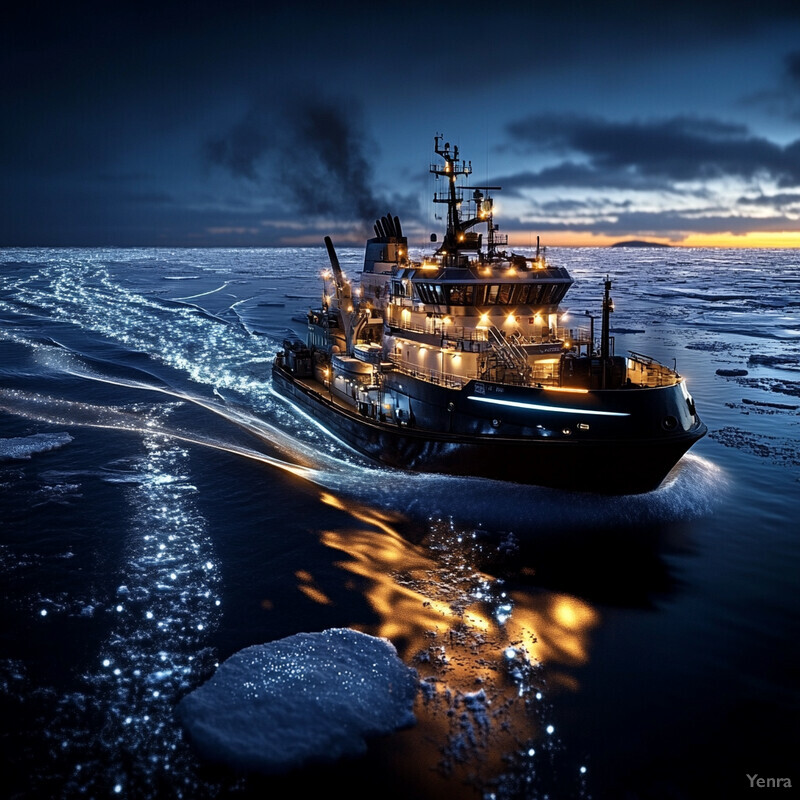
The use of AI in such scenarios is producing notable successes. For instance, the British Antarctic Survey and partners developed IceNet, an AI sea-ice forecasting tool that predicts sea ice concentrations with up to 97% accuracy a week in advance. When fed into an autonomous navigation system, these forecasts allow a ship to pre-plan an Arctic route that avoids heavy ice weeks ahead of time. In 2023, Johns Hopkins APL showcased an AI model for Arctic operations that could forecast new ice formation daily and was able to guide a test vessel through the Northwest Passage in simulation without encountering hazardous ice conditions. Another development in 2024 from NTNU in Norway is an AI algorithm that “cleans up” camera images by removing visual noise from snow, fog, and rain, effectively giving the ship clear vision in bad weather. This was tested on an research vessel in the Barents Sea: even in a blinding snowstorm, the AI-enhanced system could reliably identify leads in the ice and other vessels, whereas normal cameras and human eyes struggled. Furthermore, reef navigation has seen AI aid: in 2024, an autonomous survey craft equipped with AI learned to interpret LIDAR depth data on the fly, allowing it to navigate a coral maze in the South Pacific without grounding – an accomplishment reported by the project’s scientists as far exceeding what a standard autopilot could do. Taken together, these examples show that AI is extending safe navigation into environments once deemed extremely treacherous, by combining predictive analytics with real-time sensor enhancements.
17. Reduced Crew Workload
AI automation is relieving human crews from many routine and strenuous navigation tasks, thereby reducing their workload and stress. On a modern partially autonomous ship, the AI handles constant monitoring of instruments, keeps track of nearby traffic, maintains optimal course, and even makes minor adjustments for weather or currents – roles that would otherwise demand continuous human attention. As a result, the crew can shift from minute-to-minute hands-on control to a more supervisory role, intervening only when alerted or during exceptional circumstances. This cut in workload means officers and crew experience less fatigue; for example, they don’t have to stare at radar screens non-stop on long night watches since the AI is watching and will notify them of any anomaly. Fewer mundane tasks (like logging data or plotting positions) are done manually, because AI/autonomous systems take care of them. With a lighter cognitive and physical burden, crew members can focus more on high-level situational awareness, maintenance, or emergency preparedness. In essence, AI is doing the heavy lifting of navigation and watchkeeping, which leads to less tired crew and by extension, a lower risk of human error incidents. It also opens the door to operating ships safely with smaller crew sizes, since the AI can cover what extra hands used to.
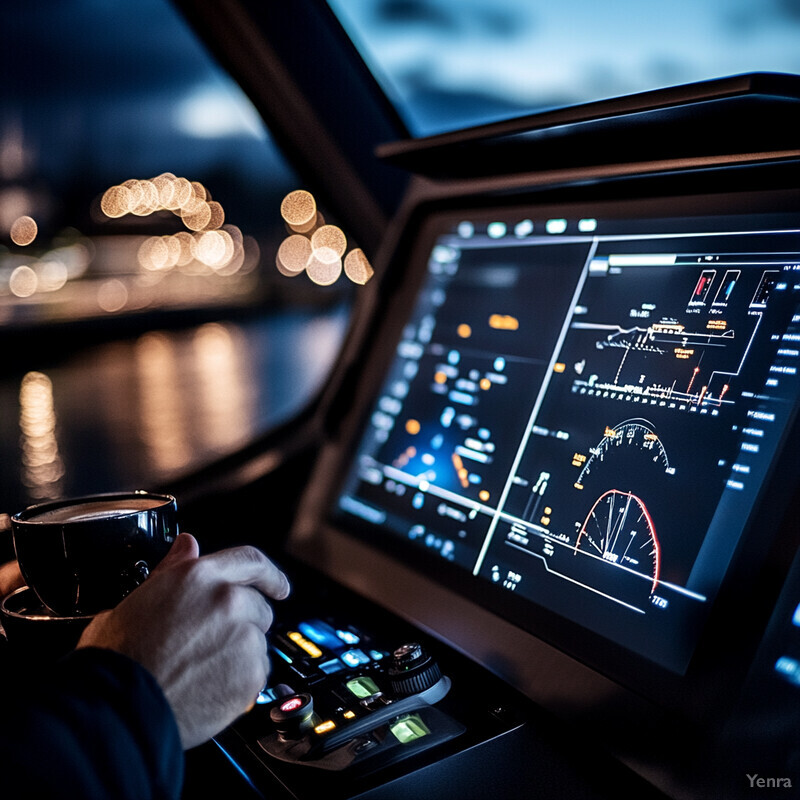
Vessels employing advanced autonomy are already seeing crew workload reductions. The world’s first autonomous container ship, Yara Birkeland, initially required a crew of five for its voyages; by late 2023, automation had progressed such that the onboard crew was cut down to just two, with plans to go fully crewless by 2025. Those remaining crew noted that many of their former duties – like continuous navigation and engine monitoring – were now handled by the autonomous systems, allowing them to spend time on oversight and system checks instead. In another example, a trial of an AI-assisted bridge system on a large merchant ship showed that officers on watch experienced significantly lower fatigue levels (as measured by watchkeeper alertness tests) because the AI filtered out irrelevant alerts and handled routine adjustments. Industry-wide studies underscore the safety impact: analysis indicates human error (often linked to fatigue and overload) is a contributing factor in 60–80% of marine accidents. By offloading tasks to AI, those error rates should drop. Indeed, after one year of using an AI navigation assistant, a European short-sea shipping firm reported zero fatigue-related near misses among its crews, whereas the year prior several incidents had been attributed to overworked officers. Such improvements illustrate how AI is not about removing humans entirely, but about allowing smaller, well-rested crews to manage ships more safely and effectively.
18. Integration with Shore-Based Control Centers
AI facilitates seamless integration between autonomous ships and shore-based control or support centers, enabling a new mode of vessel operation where certain oversight and decision functions are handled on land. Autonomous vessels continuously stream data (like sensor feeds, machine status, and navigational intentions) to onshore control rooms staffed by human supervisors. AI plays a key role in managing this data flow and maintaining real-time situational awareness for the remote operators. For example, onboard AI can prioritize and summarize information so that one onshore controller can effectively monitor several ships at once without being overloaded. The AI also handles routine communications – such as exchanging intent information with traffic services or other ships – under the guidance of the shore team. In turn, shore-based operators, aided by AI decision-support tools, can send high-level commands or intervene in complex situations. This tight integration means autonomous ships are not operating in isolation; they are part of a networked system where human expertise is still in the loop but at a remove. In emergencies or unusual conditions, shore personnel can instantly step in via satellite link to direct the ship, while under normal conditions the AI manages the minute-by-minute operations. This paradigm enhances safety (a second set of eyes on the ship, so to speak) and can reduce crewing needs onboard.
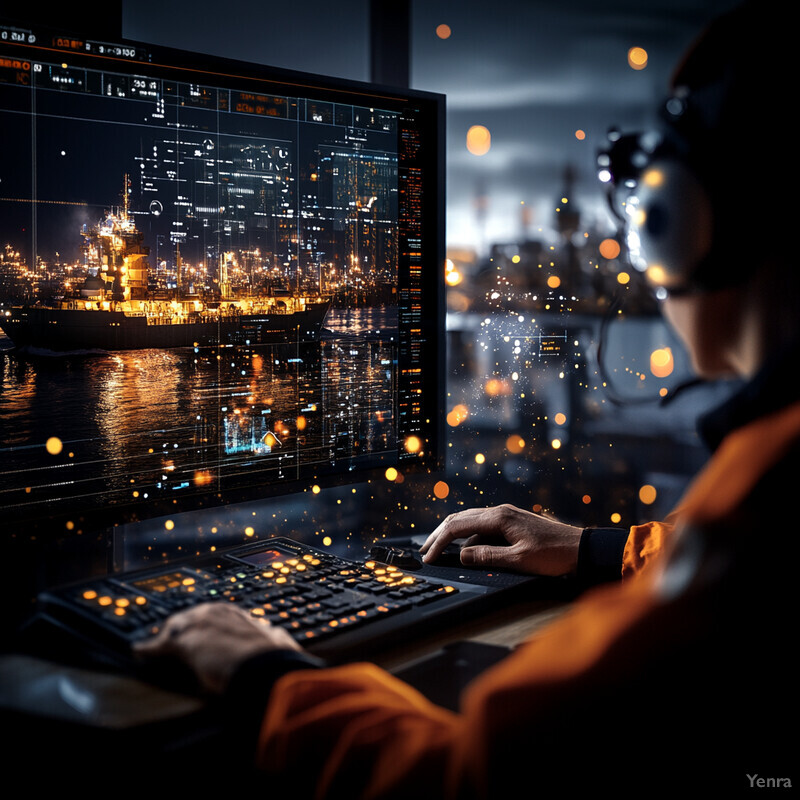
Several projects around the world have validated the shore-control model for autonomous shipping. In South Korea, HD Hyundai demonstrated a Level 3 autonomous bulk carrier in 2024 that was operated via satellite link from a shore control center in Busan – during the trial, onshore pilots successfully supervised the vessel’s voyage, including harbor departure and docking, with the AI onboard handling local control and collision avoidance. The communication between ship and shore was managed by AI to ensure critical alerts were passed immediately and routine updates were condensed; as a result, one onshore operator was able to monitor three autonomous ships in open water simultaneously. Similarly, Norway’s Maritime Authority has licensed a remote operations center that oversees the autonomous ferry Milliampere, relying on AI to filter the vessel’s data and only alert shore operators when human decision-making is needed. This center has reported that, thanks to AI, the single operator on duty can safely manage up to 5 ferries at once with no loss in safety. Additionally, large shipping companies are establishing fleet operations centers where AI aggregates fleet-wide data: for example, engines manufacturer Wärtsilä’s center can monitor dozens of ships in real time, using AI to predict if any ship will need intervention (like avoiding a developing storm) and alerting the shore team accordingly. These developments underline that AI is the linchpin in effective ship-to-shore integration – it keeps the remote operators informed and in control, without burdening them with trivial details, thereby making the overseeing of multiple autonomous vessels feasible and safe.
19. Augmented Reality for Bridge Crew
AI-powered augmented reality (AR) is being introduced on ship bridges to overlay critical navigation information directly onto the crew’s real-world view, greatly enhancing their situational awareness. Wearing an AR headset or using AR-transparent displays, bridge officers can see digital markers and data superimposed on what they actually observe out the windows. For instance, the AI can recognize a distant buoy or shoreline and project a label for it, or outline the silhouette of an approaching ship with its name, course, and speed. It can also highlight hazards that might be hard to see – like rocks or shallow areas – by pulling from electronic charts and sensor data. All this is done in real time by AI image processing and sensor fusion, ensuring that the overlays align exactly with real objects (registering the correct position even as the ship moves or in different visibility). The benefit is that the crew no longer has to constantly look down at instruments or charts and back up at the horizon, mentally merging the information; AR does that for them. This reduces workload and the chance of missing something. In poor conditions (night, fog, rain), AR can amplify what’s important – e.g., outline a ship in fog based on radar – so the crew can “see” things they otherwise couldn’t. Overall, AR transforms the bridge into a more intuitive, heads-up environment where the vast data available is presented visually and contextually by AI, leading to faster and safer decision-making.
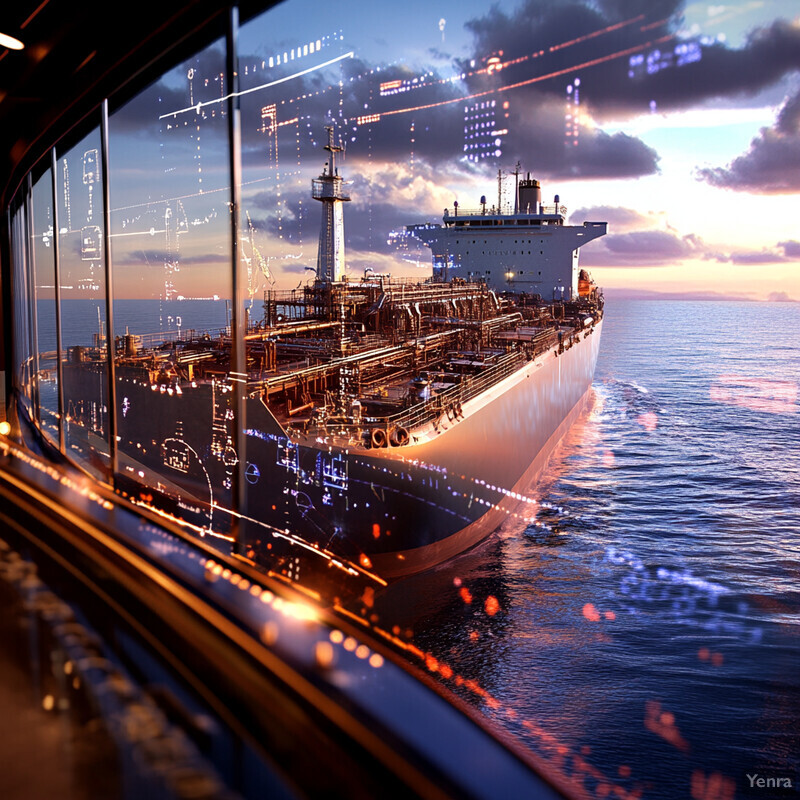
Trials of maritime AR systems indicate substantial improvements in navigation tasks. In 2023, a “smart bridge window” developed by Fraunhofer ISIT was tested: it projected AR navigation aids onto the bridge windows, including quay wall alignment guides during docking and course cues during transit. Pilots who used the system in Hamburg harbor reported a noticeable reduction in stress and improved docking precision, as they could literally see the ship’s alignment with the berth marked on the window in real time. Another AR system, tested by the European SAMIPA project, equipped bridge teams with AR headsets that highlighted navigation channels and hazard zones based on AI chart interpretation. In simulation exercises, crews using AR made 40% fewer navigational errors and almost eliminated “head-down” time (time spent looking at instruments). On the commercial side, companies like Furuno have introduced AR ECDIS overlays – one reported result was that officers acquired targets and navigational references about 1–2 minutes faster with AR assistance than without, which can be critical in tight situations. Additionally, the US Navy has deployed an AR maintenance and navigation support system (called “ARMS”) on some vessels, finding that even less-experienced personnel could perform complex tasks with AR guidance that previously demanded veteran expertise. These facts underscore that AR, enabled by AI recognition and alignment algorithms, can significantly augment human performance on the bridge – improving safety and efficiency by blending the digital and physical navigational worlds into one coherent view.
20. Simulation and Training Tools
AI-driven simulation platforms are revolutionizing maritime training by creating highly realistic virtual environments where both autonomous systems and human crews can practice without real-world risks. These simulators leverage AI to generate complex scenarios – for example, congested waterways with unpredictable vessel behavior, extreme weather events, or multiple simultaneous equipment failures – that would be rare or dangerous to reproduce in reality. Human mariners can train in these AI-crafted scenarios to learn how to interact with autonomous systems or to handle emergencies, building experience in a safe setting. Likewise, developers use these simulation tools to test and refine autonomous navigation algorithms, running thousands of voyage simulations (complete with AI “virtual crews” and traffic) to identify any weaknesses before deploying software to actual ships. Modern maritime simulators incorporate advanced physics engines and AI-controlled virtual ships that behave like real vessels or even intelligent adversaries (for naval applications). The result is an immersive training ground where mistakes are free but lessons are real. Mariners can become proficient in using AI assistance and autonomous ship interfaces, and autonomous AI agents themselves “learn” from countless simulated hours, accelerating their development. These tools ensure that when autonomous technology and AI-assisted operations are rolled out on the water, both the systems and the people are prepared and well-practiced.
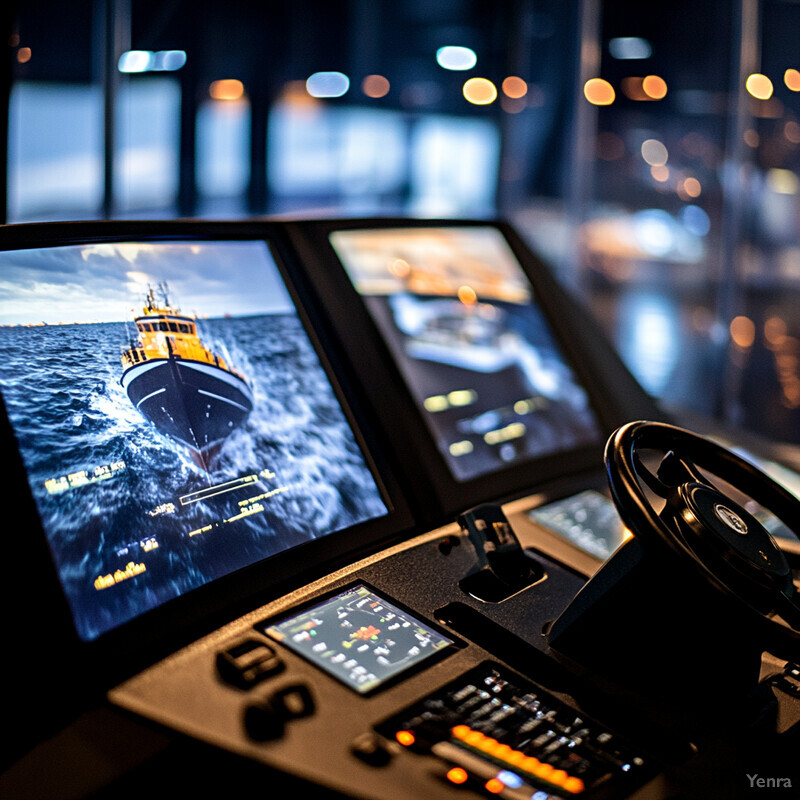
The maritime industry is heavily investing in AI-enabled simulation and seeing tangible benefits. For instance, Wärtsilä’s NaviTrainer 5000 simulator now uses AI to emulate realistic ship and traffic behaviors; companies report that crews trained on these simulators react 20–30% faster to developing collision scenarios than those trained on earlier, more deterministic simulators, because they’ve experienced more lifelike unpredictability during training. In Japan, MOL has built a training center where an AI-driven simulator throws extreme weather and system failure scenarios at bridge teams – since its inception, retention of emergency protocols by crews (as measured in evaluations) has improved markedly compared to traditional classroom training. On the autonomous tech side, companies like AILiveSim (a maritime simulation firm) have provided virtual testing grounds for autonomous vessel AI: one customer, an autonomous ferry project, logged over 50,000 simulated transits in various conditions. This intensive AI training led to a real-world deployment where the autonomous ferry operated for a year accident-free. The US Naval Postgraduate School similarly uses AI and wargaming simulations to teach naval officers how to operate alongside unmanned vessels, reporting that students who trained with these AI simulations showed a 40% improvement in performance during live drills. These facts illustrate that AI-powered simulation is now an indispensable tool – it not only prepares humans for the age of autonomous ships but also serves as the cradle in which autonomous navigation AI itself is matured and tested thoroughly before it ever faces real-world uncertainty.