1. Autonomous Drone-Based Inspections
AI algorithms enable drones to navigate inspection routes without human input, reducing time and risk.
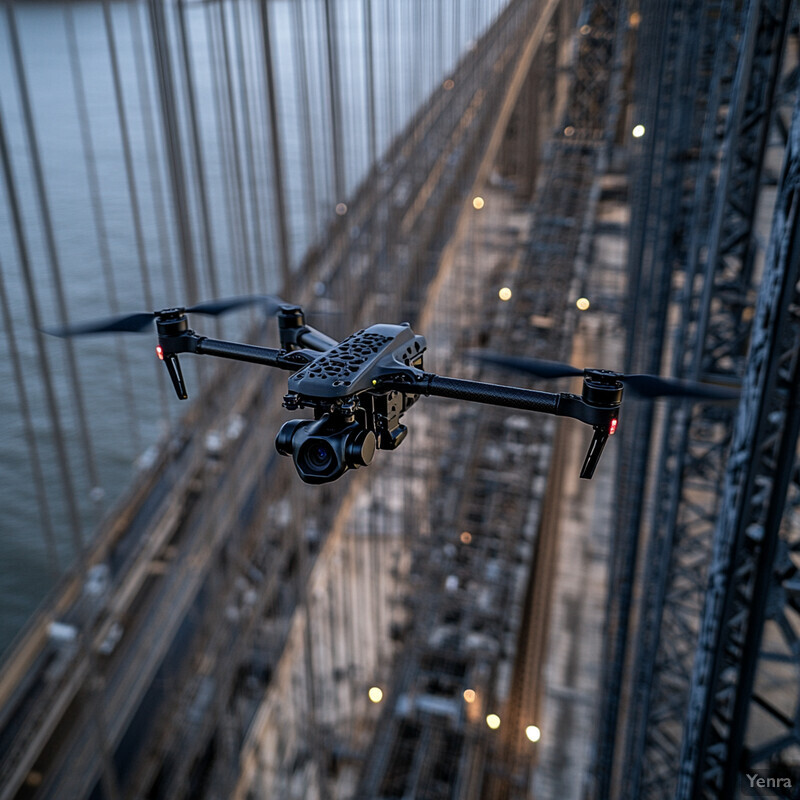
AI is revolutionizing how we use unmanned aerial vehicles (UAVs) for inspection by endowing them with autonomy. Rather than relying on a pilot’s direct control, AI algorithms plan and execute flight paths, handle obstacle avoidance, and ensure comprehensive coverage of targeted infrastructure. By doing so, human operators can shift their focus from controlling the drone’s every movement to analyzing the data it collects. This not only reduces the risk to human inspectors—especially in hazardous environments—but also significantly shortens inspection times. Over multiple missions, the drone’s AI can learn optimal flight patterns, refine its inspection strategy, and continually improve the efficiency and effectiveness of each subsequent mission.
2. Computer Vision for Defect Detection
Deep learning models analyze images and videos to identify subtle defects, ensuring more reliable and consistent inspections than manual methods.
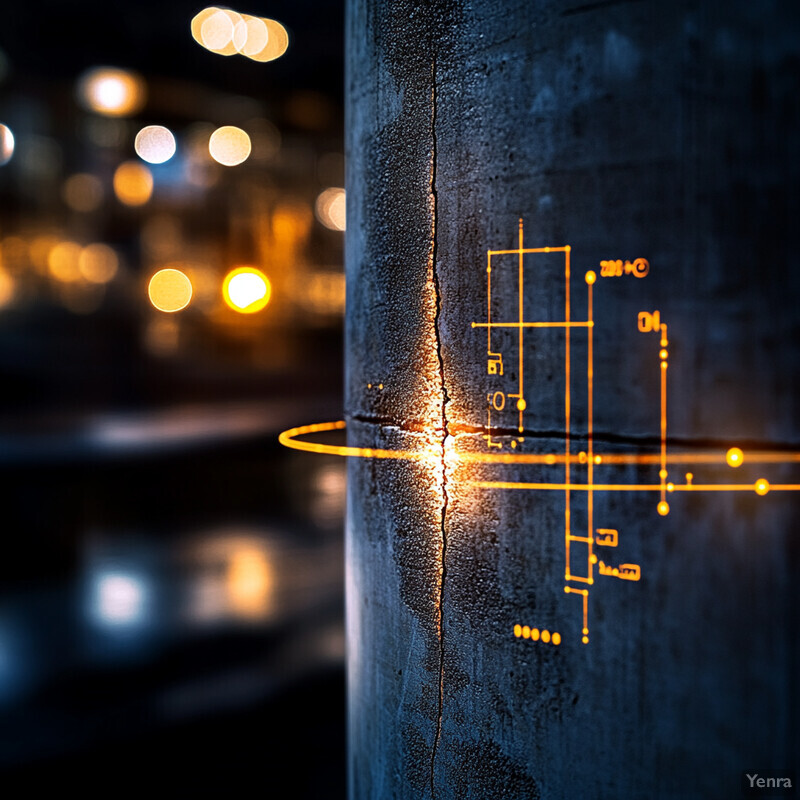
Deep learning models and advanced image recognition techniques allow AI systems to identify subtle defects in infrastructure—such as minute cracks in concrete or slight discolorations indicative of corrosion—at an early stage. High-resolution cameras feed streams of images into computer vision algorithms trained to detect even the tiniest anomalies. By eliminating the biases and inconsistencies inherent in human-based evaluations, computer vision ensures a uniform standard of inspection quality. This early detection capability can lead to proactive maintenance, preventing minor issues from escalating into costly and dangerous failures. Over time, as the AI is exposed to more examples, its accuracy and speed in identifying defects continues to improve.
3. Lidar and Radar Data Processing
AI interprets Lidar and radar data to create precise 3D models of infrastructure, helping visualize hidden internal damage.
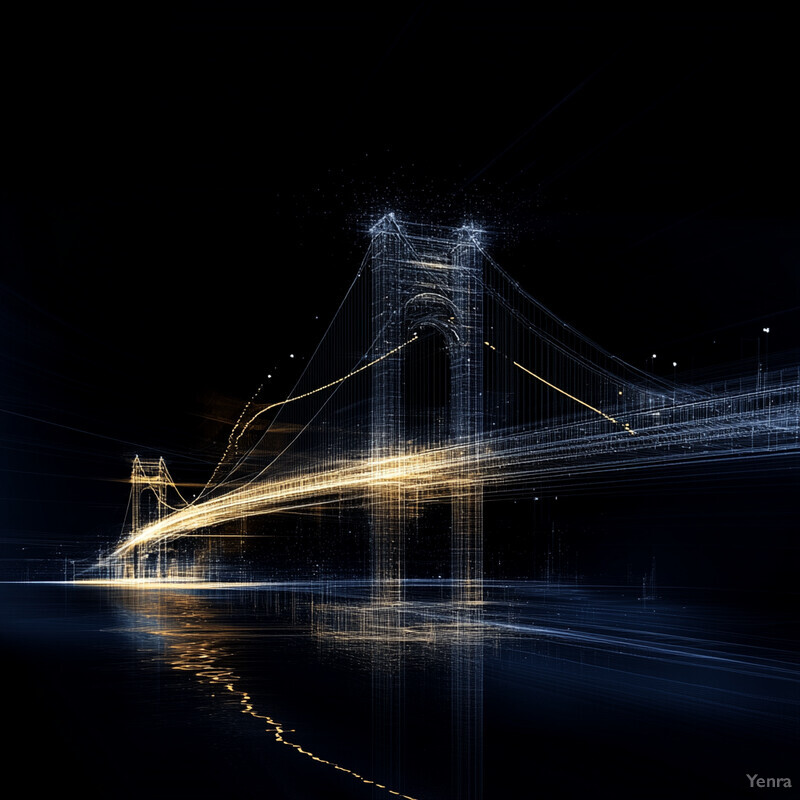
Infrastructure often demands three-dimensional analysis beyond what conventional imaging can provide. AI-driven processing of Lidar and radar-generated point clouds allows inspectors to create precise 3D models of structures such as bridges, tunnels, pipelines, and transmission towers. These models reveal hidden internal damage, thickness variations, and structural deformations that might not be visible in flat images. Because AI can process massive data sets quickly and discern patterns that humans may overlook, it can highlight subtle irregularities and dimensional shifts. This insight enables more informed decision-making, ensuring that maintenance interventions are timely, targeted, and cost-effective.
4. Predictive Maintenance Scheduling
By analyzing historical data, AI can forecast when infrastructure issues are likely to arise, enabling proactive repairs.
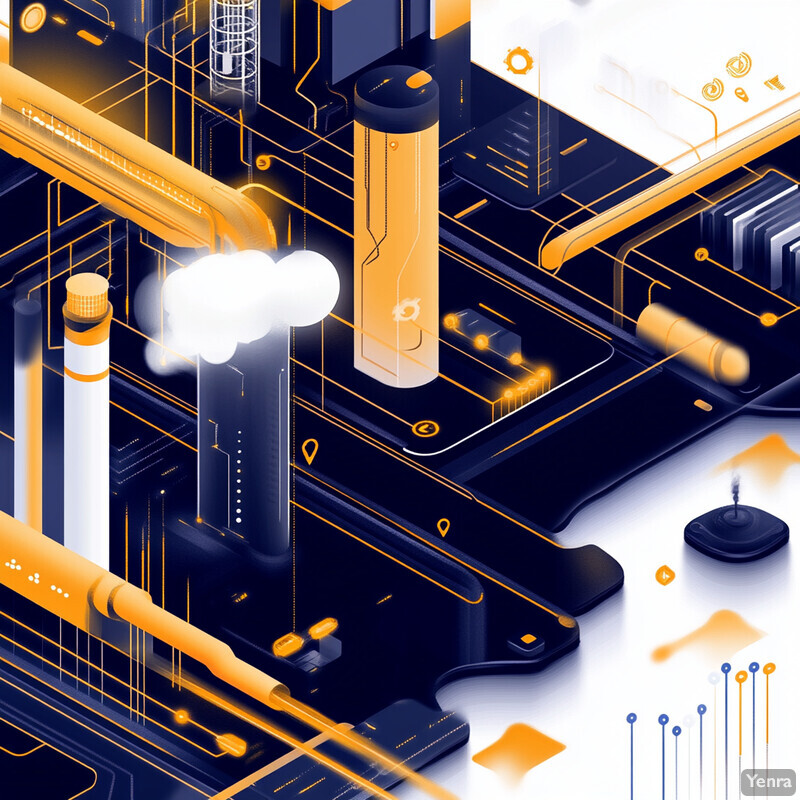
Historically, infrastructure maintenance has followed fixed intervals, regardless of the structure’s actual condition. AI transforms this approach by learning from historical data and current inspection results to forecast when and where problems are likely to arise next. For example, if an AI model knows that certain stress patterns or materials are correlated with rapid corrosion, it can predict when a specific component will likely need reinforcement. By anticipating future issues, asset managers can schedule repairs before problems manifest, minimizing downtime and potentially saving millions of dollars in emergency maintenance. This predictive approach also extends the lifespan of infrastructure and improves overall reliability.
5. Automated Route Planning
AI-based algorithms determine efficient inspection paths for drones or robots, saving time and energy.
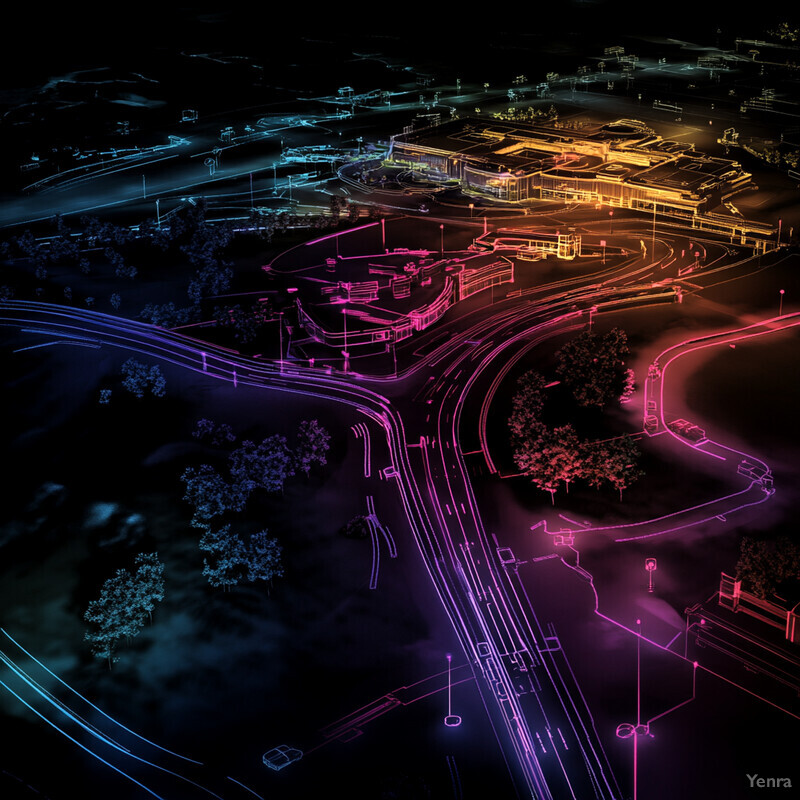
Planning an inspection route for drones, ground robots, or other inspection tools can be complex, especially for large-scale or geographically challenging infrastructure. AI algorithms consider factors such as battery life, sensor range, access constraints, and weather conditions to optimize the path and ensure comprehensive coverage. This reduces the likelihood of missed spots, shortens the total inspection time, and conserves energy resources. Over time, as the AI gains experience, it can refine these routes based on actual performance outcomes, continually improving efficiency. Consequently, human operators spend less time in planning mode and more time making strategic decisions based on the collected data.
6. Digital Twin Integration
AI-powered analytics synchronize real-world data with digital twins, enabling predictive simulations and better decision-making.
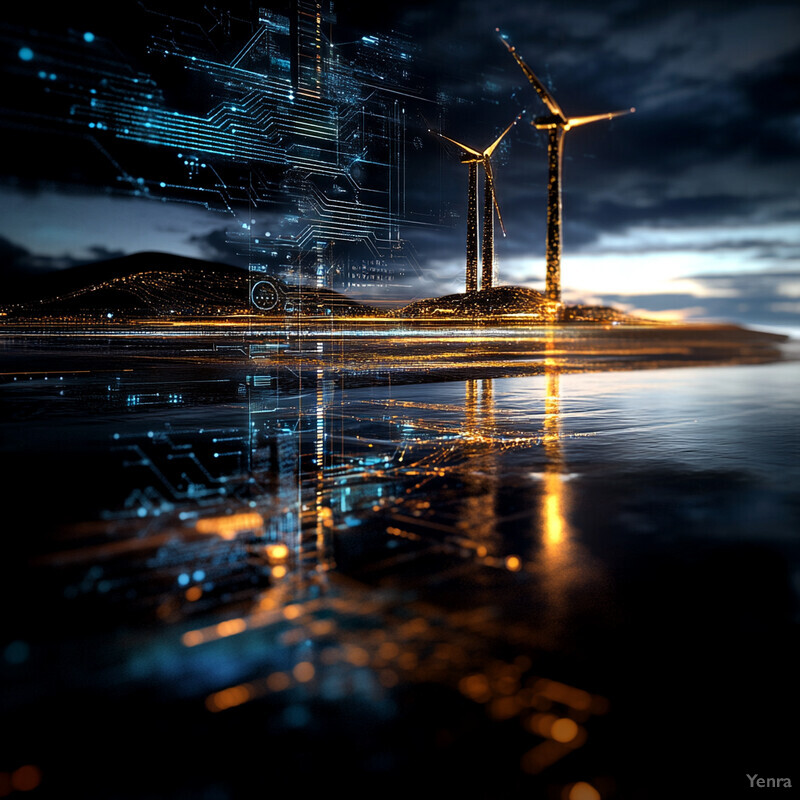
A digital twin is a virtual, data-driven replica of a physical asset. By integrating AI-driven inspection data with these digital twins, engineers and asset managers can simulate various “what-if” scenarios to anticipate how the infrastructure might evolve under different stresses or environmental conditions. The AI updates the digital twin continually with new inspection findings, making it a living, evolving model. This synergy enables predictive analytics, allowing teams to run simulations before making physical changes, thus reducing trial-and-error and improving overall decision-making. In practice, digital twins paired with AI can lead to more accurate investment planning, better risk management, and improved asset lifecycle management.
7. Sensor Fusion for Enhanced Insight
Machine learning merges data from multiple sensors, providing a more complete picture of infrastructure health.
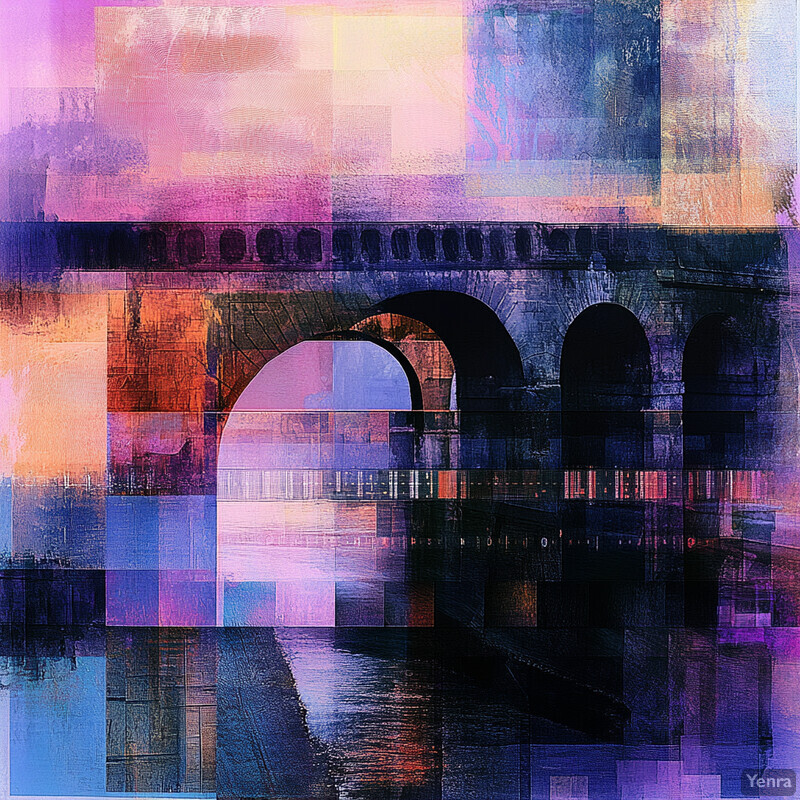
Modern inspection platforms gather data from a wide array of sensors: high-resolution RGB cameras, thermal imagers, ultrasonic sensors, vibration monitors, and more. AI excels at fusing these diverse data streams to create a richer, more holistic understanding of infrastructure health. For instance, by combining temperature anomalies from thermal imaging with structural data from ultrasonic sensors, the system may detect corrosion pockets invisible to any single data source. This multi-sensory approach allows for earlier fault detection and a more comprehensive assessment of infrastructure integrity. It also improves decision-making accuracy and reduces the risk of overlooking critical problems.
8. Real-Time Anomaly Alerts
Onboard AI systems flag potential issues immediately, allowing for prompt investigation and faster corrective action.
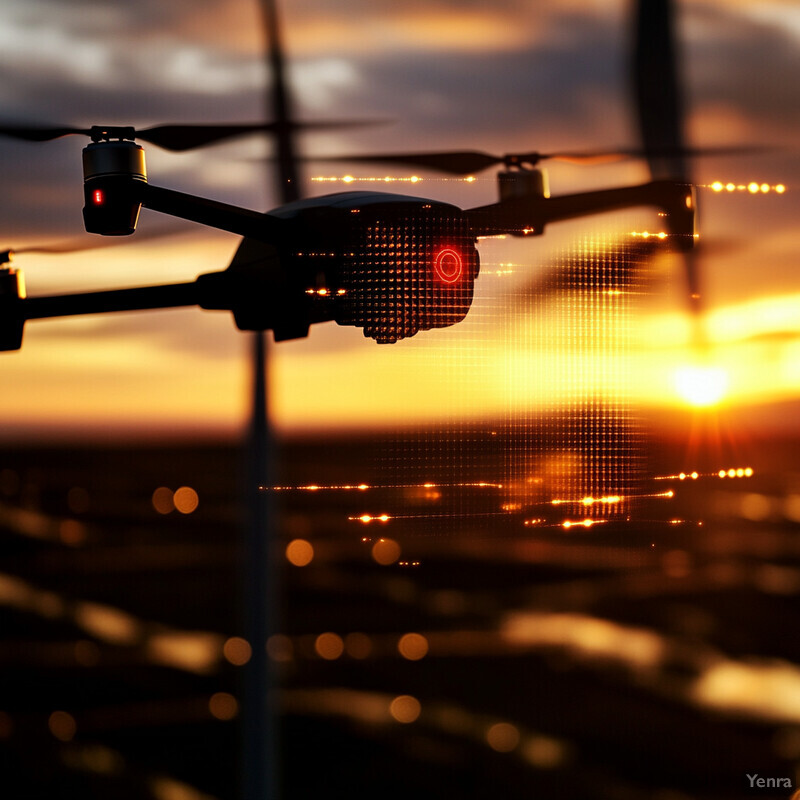
Real-time data processing enables AI-driven inspection systems to notify operators instantly when they detect potential issues. Instead of waiting for post-mission data analysis, inspectors can investigate flagged anomalies on the spot. For example, if a drone-mounted camera discovers a crack that meets predetermined risk criteria, the AI can send an alert during the flight. This immediate feedback loop streamlines the inspection process, allowing teams to obtain supplementary data or even begin repairs sooner. Such timely intelligence helps prevent minor damage from escalating, ensuring that the right interventions happen at the right time.
9. Progressive Damage Tracking
By comparing current inspections to past data, AI reveals slow, progressive degradation trends.
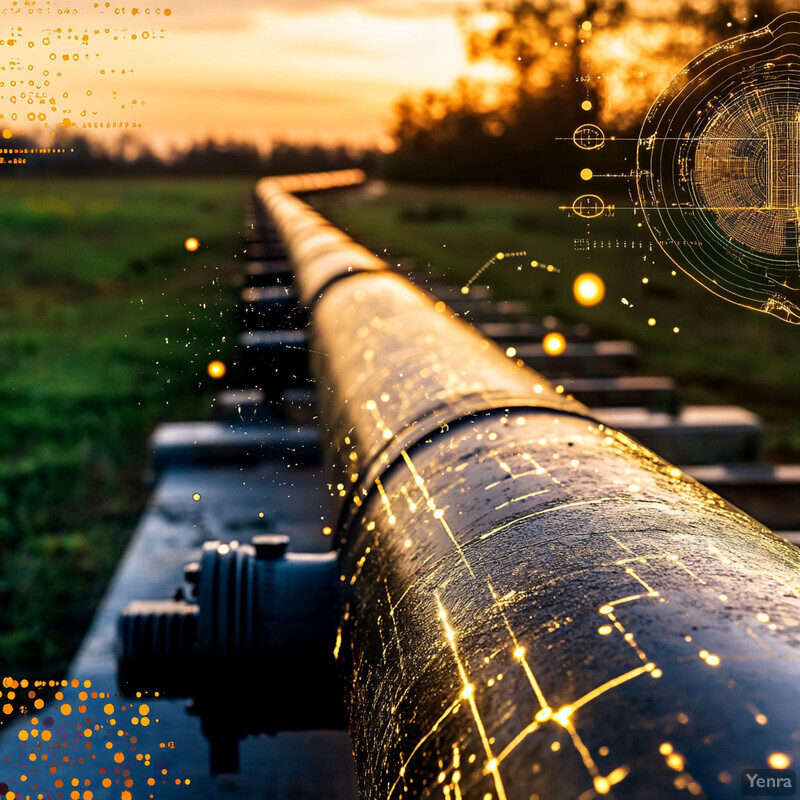
Infrastructure often degrades over long periods, and small defects can gradually worsen without obvious signs until it’s too late. AI-based analysis compares current inspection results to previous baselines, quantifying changes in defect size, corrosion rates, or material thickness. Instead of treating each inspection as an isolated event, the system understands infrastructure health as a dynamic, evolving condition. This long-term, data-driven view helps asset owners identify trends, prioritize maintenance schedules, and potentially discover previously unknown degradation mechanisms. Ultimately, progressive damage tracking leads to more informed planning and better allocation of resources.
10. Risk Prioritization Models
AI models rank defects by severity and likelihood of failure, focusing resources on critical issues first.
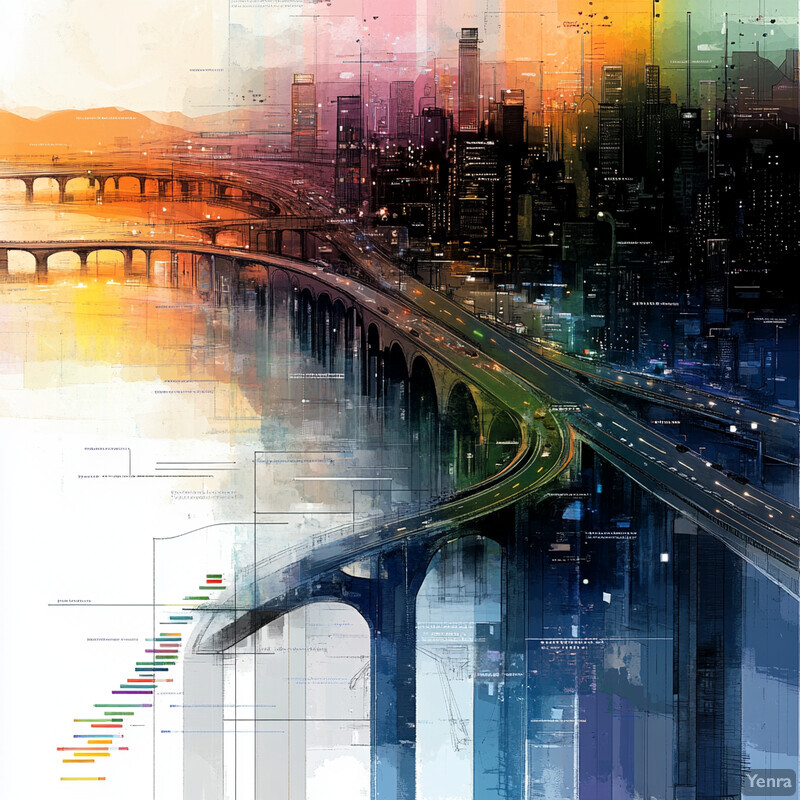
Not all defects carry the same level of risk. Some may be cosmetic, while others could signal an impending catastrophic failure. AI-driven risk models evaluate the severity and likelihood of various defects and conditions, considering factors like load stresses, historical failure modes, and environmental conditions. By ranking risks, the AI helps managers focus their attention and budgets on the most critical infrastructure components first. This prioritization ensures that limited maintenance resources are used effectively, improving safety, reliability, and cost-effectiveness. Instead of a “one-size-fits-all” approach, owners can implement a targeted strategy that aligns with the actual risk profile of their assets.
11. Natural Language Processing for Reporting
NLP systems convert raw inspection data into clear, standardized reports, streamlining documentation.
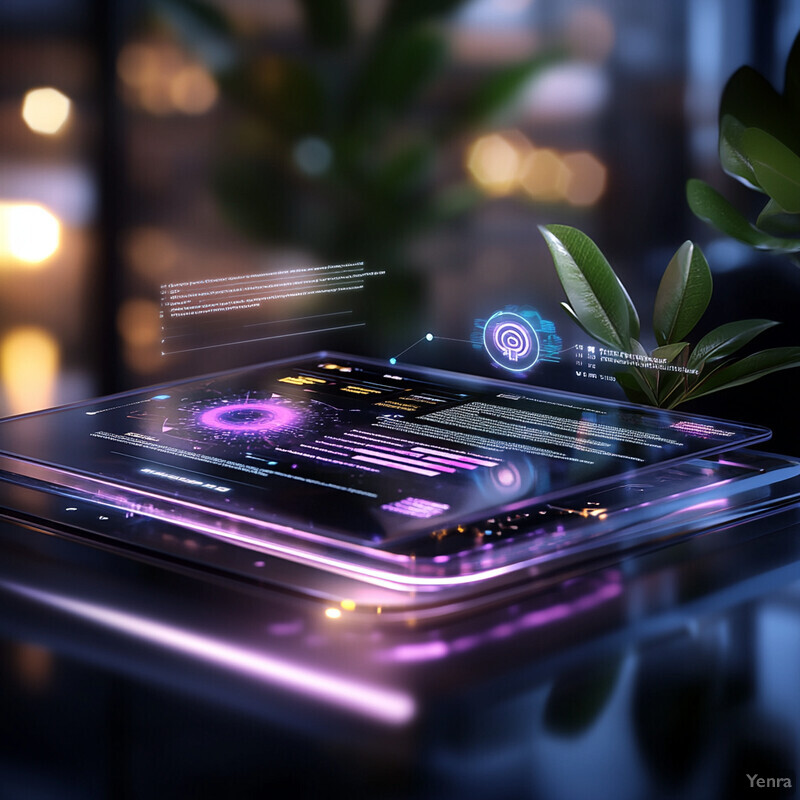
The wealth of data gathered by AI-driven inspections can be overwhelming without a clear format for communication. By employing NLP techniques, AI systems can transform raw sensor readings and image analysis results into coherent, standardized reports. This helps technicians, engineers, and regulatory bodies quickly understand the state of the infrastructure without having to sift through complex data sets. These AI-generated summaries ensure consistent documentation, easy compliance checks, and reduced administrative overhead. The simplified reporting also makes it easier to share findings with stakeholders, leading to faster decision-making and improved accountability.
12. Automated Classification of Infrastructure Types
AI identifies infrastructure categories instantly, tailoring inspection protocols to each asset type.
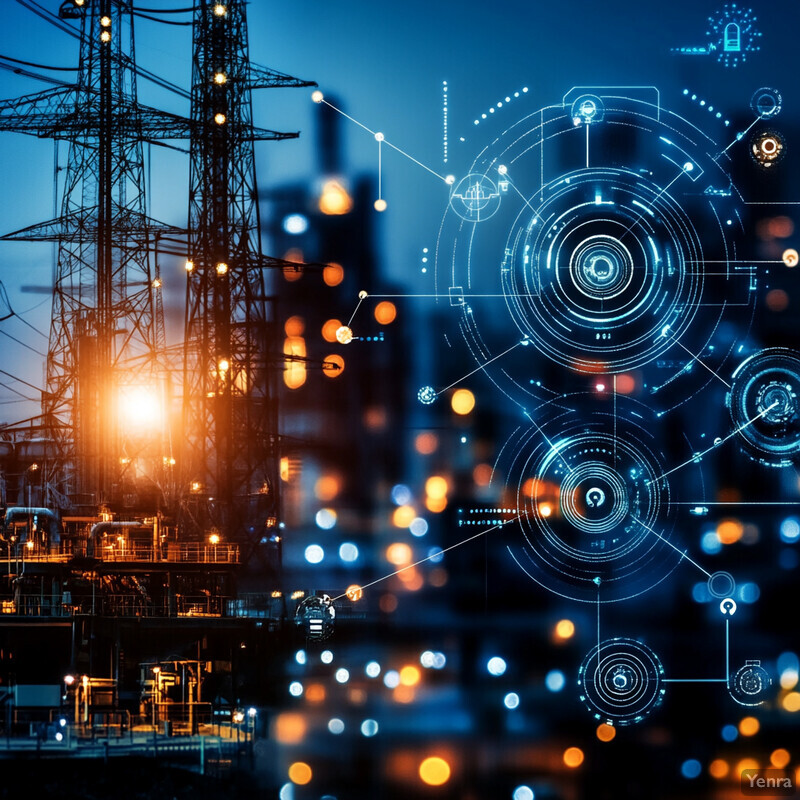
Infrastructure assets vary widely—from bridges and dams to wind turbines and pipelines—each demanding a unique inspection strategy. AI classification algorithms can automatically identify the type of structure being inspected and adjust the inspection parameters and analysis methods accordingly. This adaptation can be especially helpful when dealing with large or mixed infrastructure portfolios. Instead of manually configuring each inspection, the system tailors its approach, ensuring that the right sensors, analysis models, and evaluation criteria are applied. As a result, inspections become more accurate, efficient, and scalable.
13. Energy Efficiency and Resource Management
By optimizing routes and sensor use, AI reduces energy consumption, manpower, and costs.
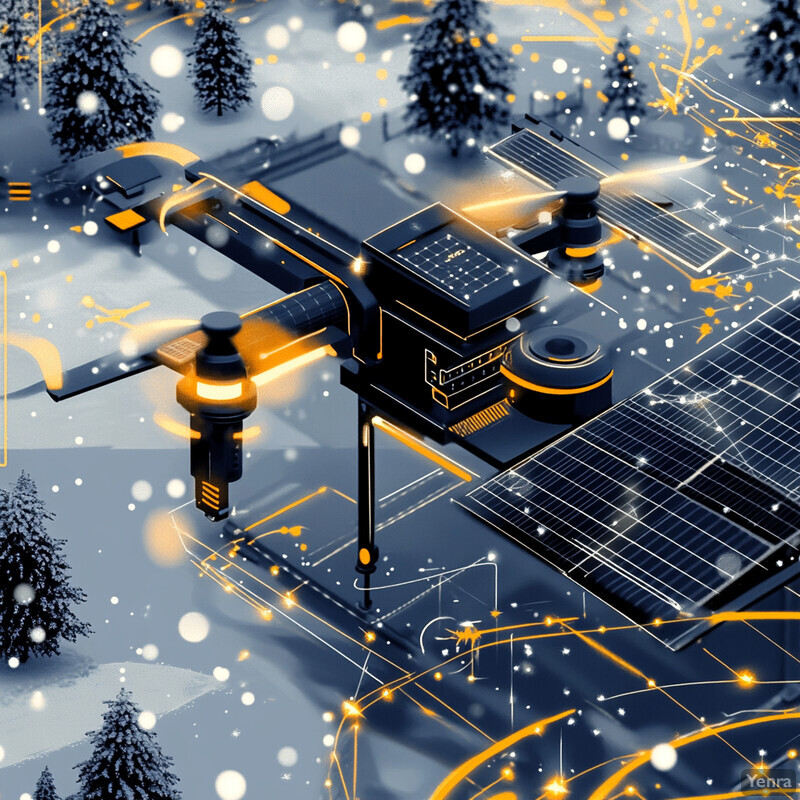
Operating drones, robots, and other inspection equipment consumes energy and manpower. AI helps optimize these resources by planning routes, balancing sensor usage, and predicting exactly when and where to deploy certain tools. Reduced flight times, fewer unnecessary inspection passes, and effective use of onboard sensors all translate to lower operational costs and environmental impact. Over many inspections, these incremental gains add up to significant savings, allowing organizations to re-invest in more advanced technologies, expand their inspection programs, or enhance worker training—all while reducing their carbon footprint and environmental impact.
14. Enhanced Safety Through Remote Monitoring
Autonomous inspections keep human workers at safe distances, minimizing exposure to hazardous conditions.
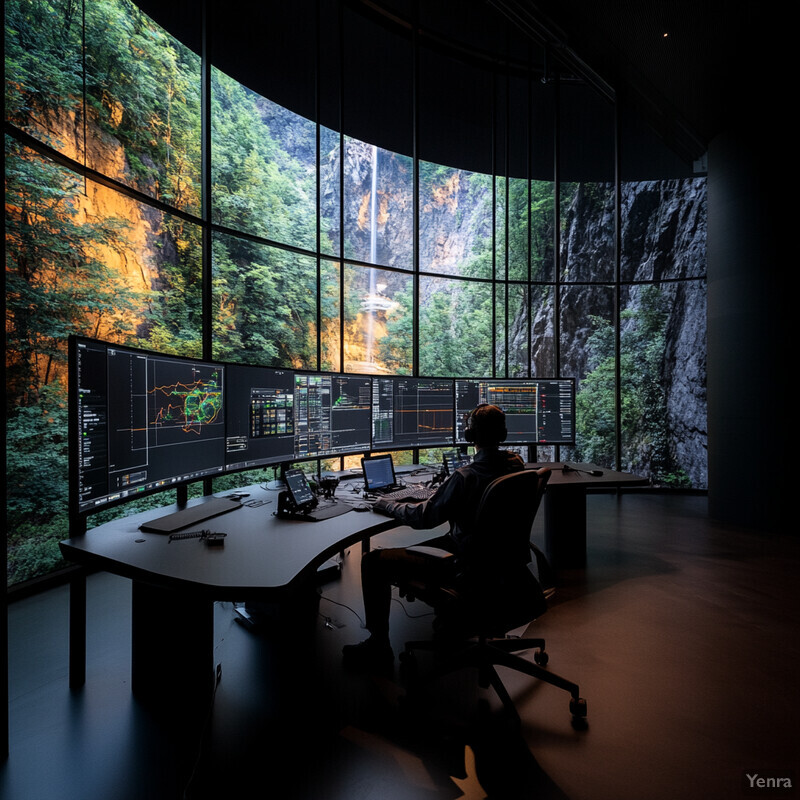
Performing manual inspections often involves sending workers to high places, confined spaces, or hazardous environments. AI-driven autonomous inspections let human experts stay at safe distances, monitoring data streams and controlling equipment remotely. By minimizing direct human interaction with potentially dangerous areas, the risk of falls, exposure to toxic substances, or structural failures is dramatically reduced. Safety improvements not only protect workers but also lower insurance costs, reduce compliance challenges, and prevent interruptions caused by workplace accidents. In the long run, enhanced safety measures contribute to more sustainable and ethical inspection practices.
15. Augmented Reality Overlays
AR technology powered by AI highlights defects on-site, guiding inspectors with visual cues.
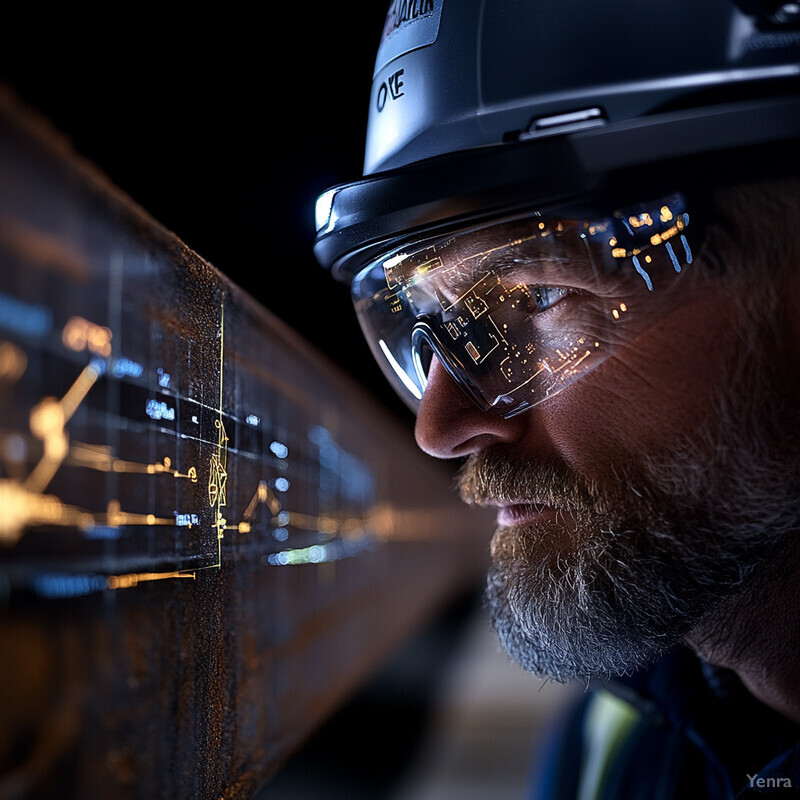
When inspectors do need to be on-site, AI can support them through augmented reality (AR) interfaces. AR headsets or handheld devices can overlay data from the AI analysis onto the real-world view of the structure. For example, an inspector looking at a bridge column might see highlighted areas indicating internal cracks or corrosion identified by previous drone inspections. This direct merging of digital insights with physical reality helps technicians pinpoint issues faster, reduces guesswork, and improves the accuracy of on-the-spot decisions. AR guided by AI-driven analytics thus speeds up the inspection process and enhances the quality of field assessments.
16. Learning from Collective Industry Data
Federated learning allows AI models to improve by pooling insights from multiple infrastructure owners.
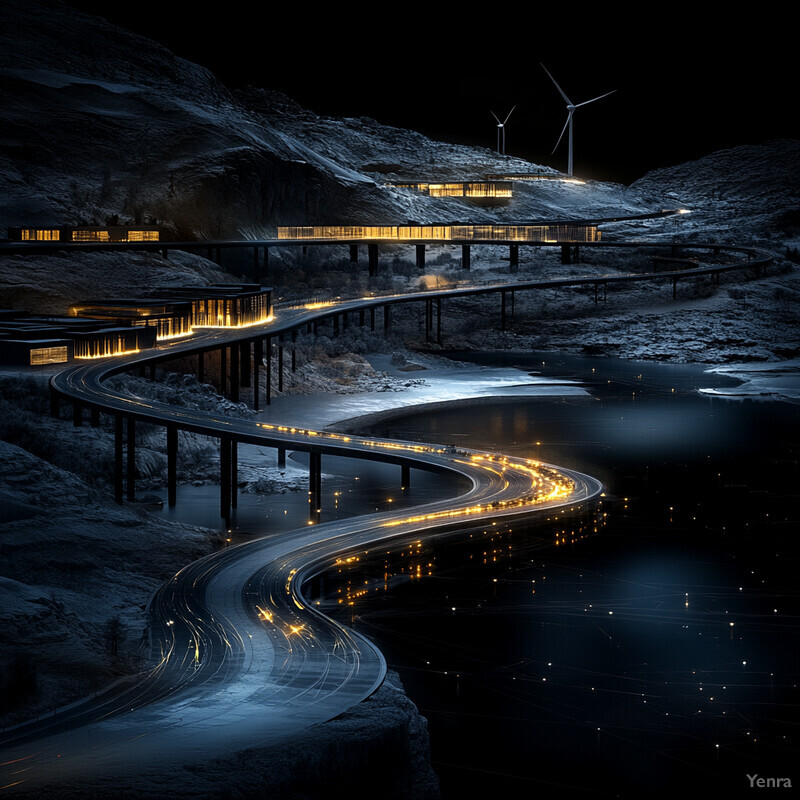
Large-scale infrastructure inspections generate immense amounts of data. Federated learning and collaborative AI models enable organizations to pool insights without sharing sensitive details. By collectively training models on distributed datasets, the AI systems benefit from a broader spectrum of scenarios, defect types, and environmental conditions. As more companies adopt AI-driven inspections, the industry as a whole accelerates its learning curve. Models become more robust, accurate, and adaptive, ultimately raising inspection standards, reducing costs, and improving outcomes for all stakeholders involved in infrastructure management.
17. Adaptive Algorithms for Diverse Conditions
AI adjusts inspection parameters on the fly to maintain quality, even under changing weather or lighting.
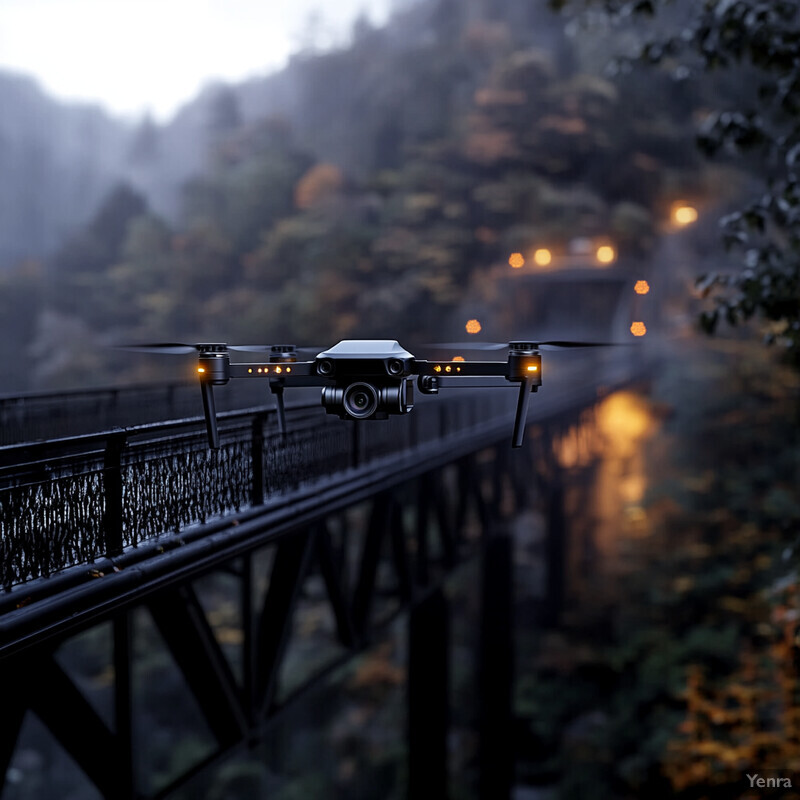
Infrastructure inspections occur under varying weather patterns, lighting conditions, and environmental constraints. AI algorithms can adapt in real-time, adjusting camera settings, sensor thresholds, and even flight paths to maintain consistent data quality. For example, if visibility declines due to fog, the system can rely more heavily on thermal or Lidar data. This adaptability ensures that inspections remain reliable regardless of unpredictable conditions, producing trustworthy analyses every time. As a result, maintenance teams gain more consistent and actionable insights, even when working in challenging environments.
18. Global Scalability and Standardization
AI-driven methods provide a consistent framework for inspections worldwide, ensuring uniform quality and reliability.
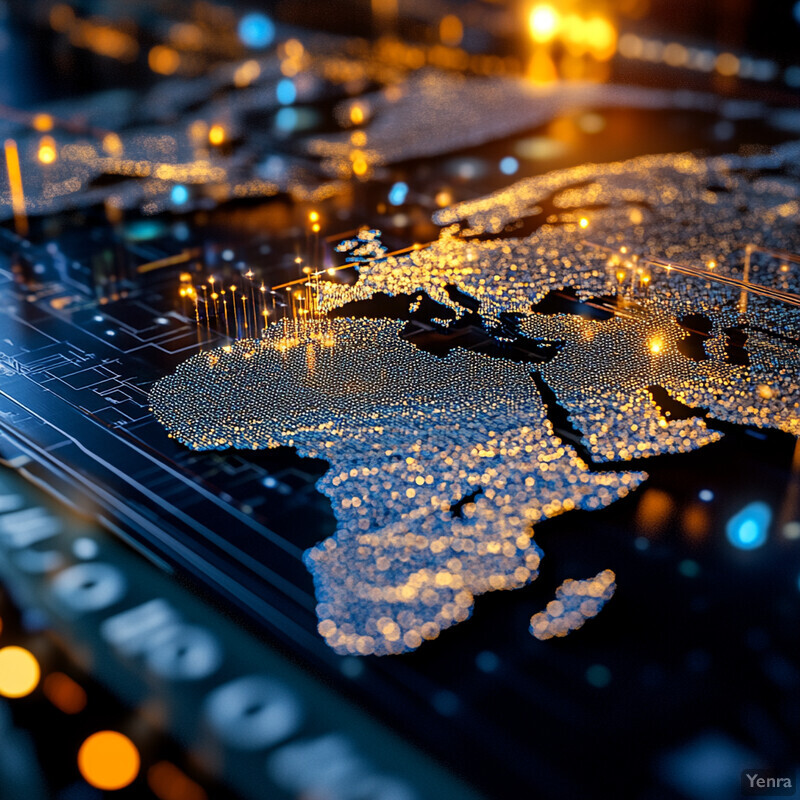
Infrastructure inspection is a universal need, transcending national borders and industry sectors. AI-driven techniques provide a standardized, scalable approach that can be applied globally, enabling uniform quality control and reliability. With consistent analysis models and best practices embedded in the AI, organizations can deploy the same high standards across multiple sites and continents. This global scalability simplifies expansion, acquisition, and integration processes, allowing multinational companies to maintain coherent infrastructure health strategies worldwide and fostering international collaboration on infrastructure safety and maintenance.
19. Reduced Downtime and Disruption
Efficient, accurate AI inspections minimize the need for shutdowns, keeping infrastructure operational and reducing losses.
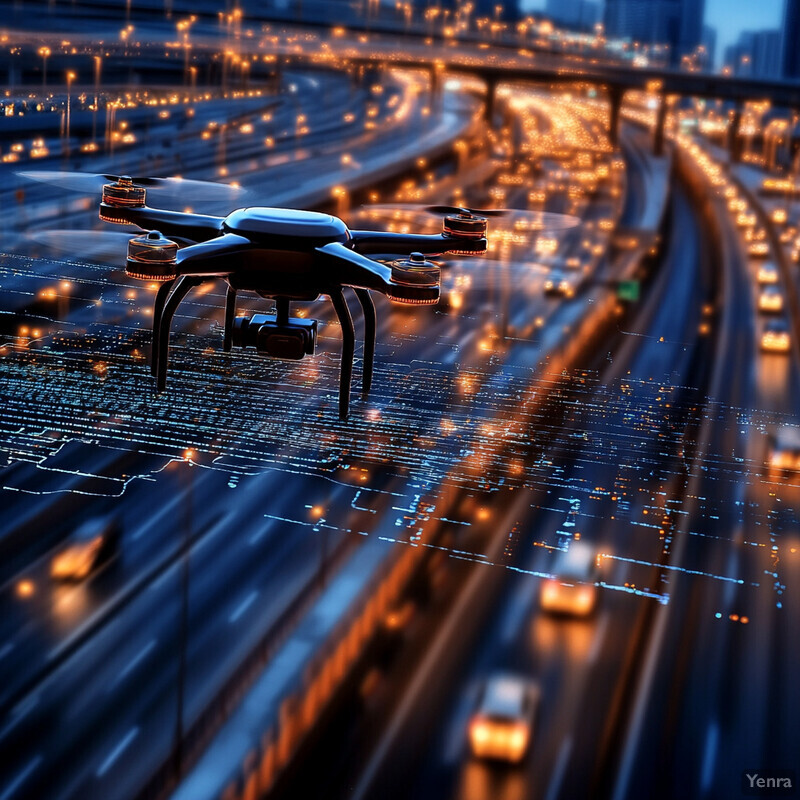
Traditionally, infrastructure inspections often required partial shutdowns, detours, or other operational disruptions. AI-driven inspection tools can function with minimal intervention, performing their tasks efficiently and often alongside normal operations. By detecting issues early and accurately, maintenance can be planned more strategically, avoiding emergency work and long periods of downtime. The improved reliability of inspections translates to more stable operation of critical assets, less inconvenience to the public, and lower economic losses. Over time, this enhanced operational continuity contributes significantly to both cost savings and public satisfaction.
20. Compliance with Regulatory Standards
AI instantly checks conditions against regulations, aiding in prompt correction and simplified audits.
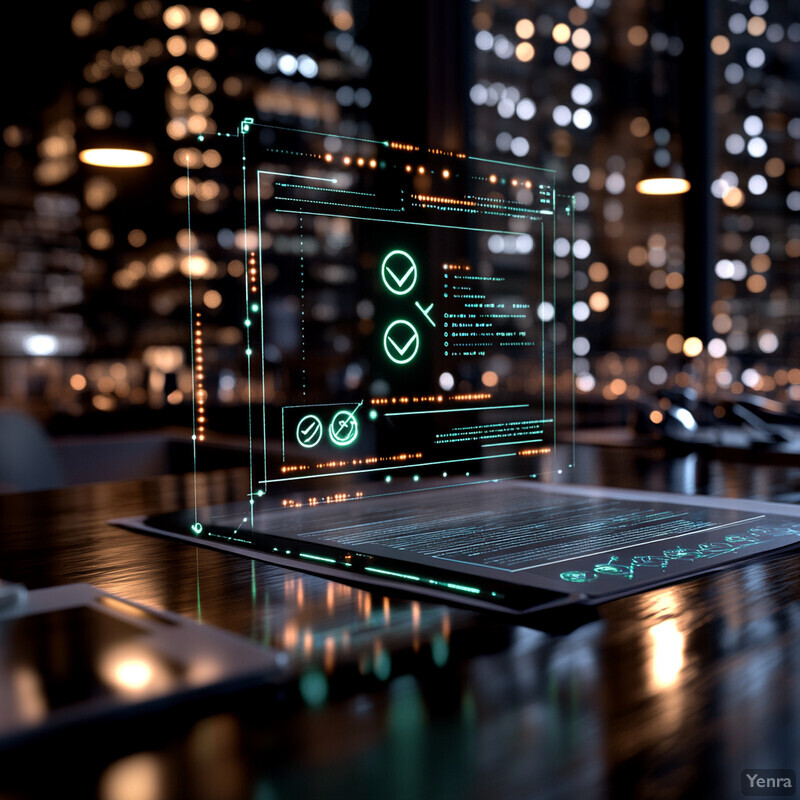
Many infrastructure assets are subject to strict regulatory requirements regarding safety, maintenance, and documentation. AI can automatically compare inspection findings against relevant regulations and guidelines. When the AI detects non-compliance, it can flag these issues immediately, reducing delays in meeting standards. Furthermore, having AI-generated reports and historical data on hand simplifies audits, as inspectors and regulators can easily verify conditions. This ensures that asset owners maintain accountability, remain transparent, and meet the legal obligations that govern infrastructure safety and performance, ultimately benefiting communities and stakeholders alike.