1. Personalized Product Recommendations
Advanced recommendation engines use AI to analyze past purchase history, browsing habits, and user preferences to deliver highly relevant product suggestions tailored to individual tastes.
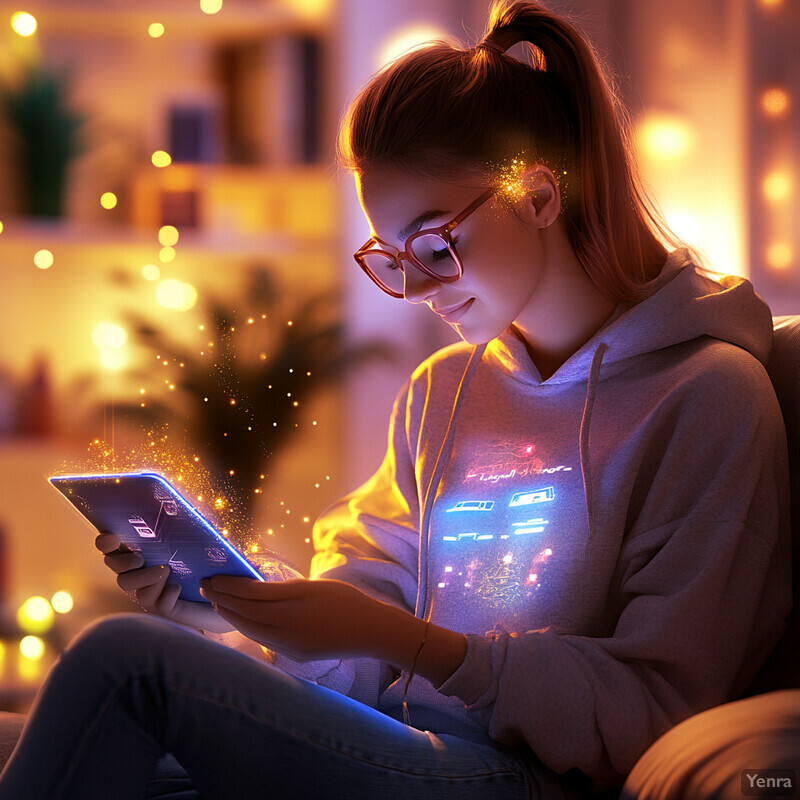
AI-driven recommendation engines use a wealth of data—from past purchases and browsing history to item ratings and wishlists—to generate highly individualized product suggestions. By identifying patterns in consumer behavior and understanding subtle preferences, these systems can curate selections that align with a shopper’s tastes, style, and price range. This level of personalization not only boosts customer satisfaction and engagement but also increases conversion rates, as users are more likely to buy items that resonate with their unique interests. Over time, these algorithms refine their recommendations further by learning from each interaction, ensuring that the shopping experience remains fresh, relevant, and enticing.
2. Natural Language Understanding for Queries
AI-powered natural language processing enables users to communicate with shopping assistants in everyday language rather than through rigid keywords, allowing more intuitive and conversational searches.
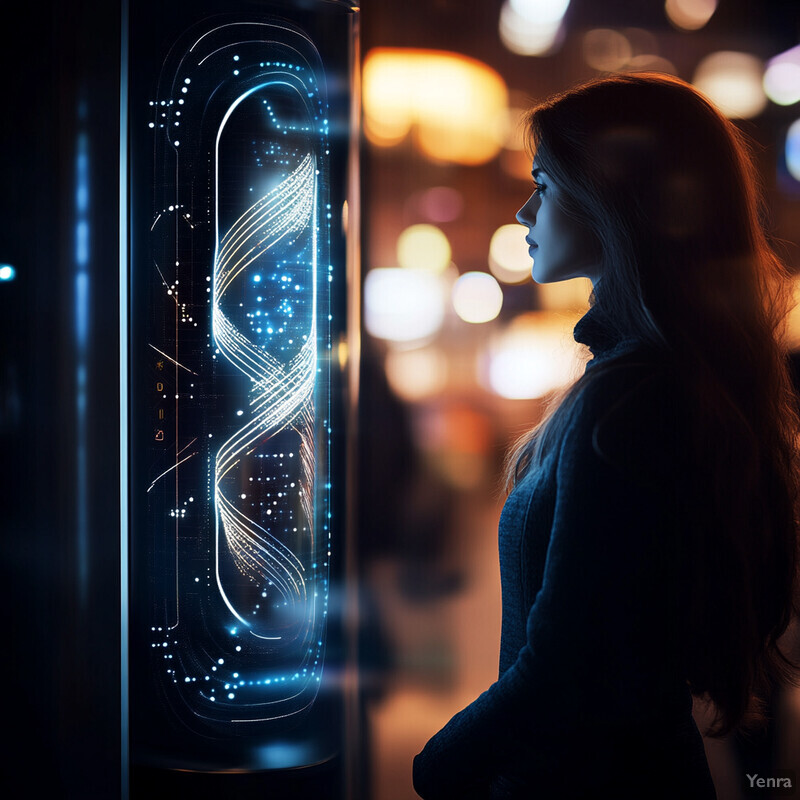
Traditional search functions require users to input carefully selected keywords. In contrast, AI-powered language models enable customers to communicate with personal shopping assistants in conversational, everyday language. These systems parse complex sentences, understand intent, and respond accurately to ambiguous queries, making the process feel more like speaking to a knowledgeable human assistant than wrestling with a search bar. As a result, shoppers can ask questions such as “I need a dress suitable for a summer wedding,” and the assistant will return highly relevant options. This human-like interaction reduces friction in the shopping experience and encourages more frequent use of the platform.
3. Context-Aware Suggestions
Intelligent assistants consider context—such as current location, time of day, seasonal trends, or upcoming events—to provide recommendations that are timely and situationally appropriate.
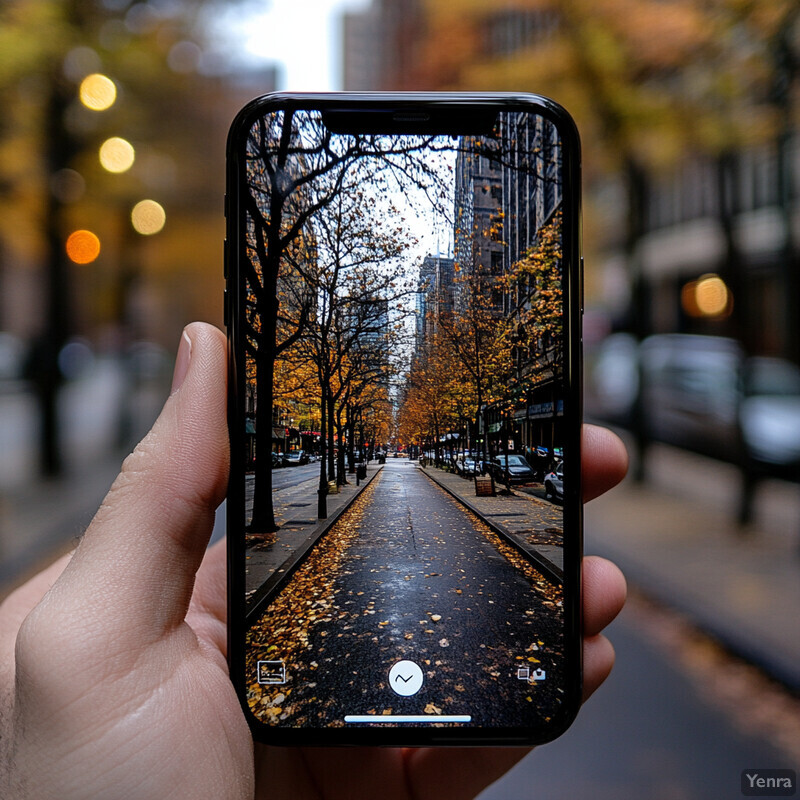
Advanced AI models take into account a user’s broader context to deliver recommendations that feel timely and situationally fitting. Factors like seasonal trends, upcoming holidays, local climate, and even the user’s recent browsing topics can all influence suggestions. For example, if it’s early December, the AI may prioritize winter coats, holiday décor, or gift sets. If the user is browsing from a coastal location, swimwear and beach accessories may rise in relevance. By weaving contextual signals into product discovery, the shopping assistant continuously adapts its suggestions to the changing circumstances of the consumer’s life, making the guidance feel personal and well-informed.
4. Image and Visual Search
Using computer vision, shoppers can upload photos of desired items and have the AI assistant identify and suggest similar or complementary products, streamlining the discovery process.
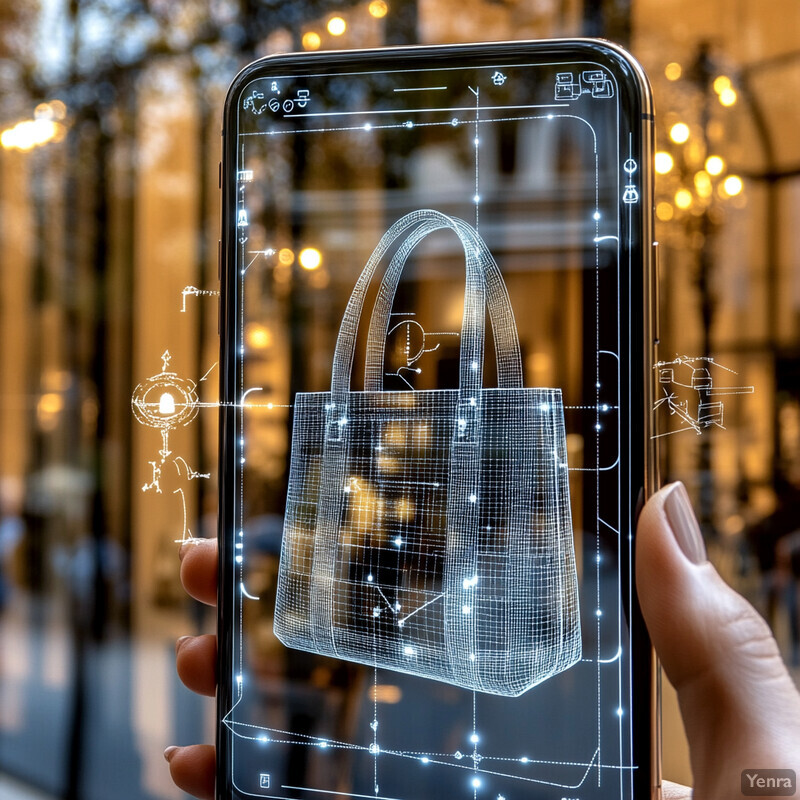
AI-driven computer vision empowers personal shopping assistants to identify products from images, turning photos into queries. Instead of typing a description, a user can upload a picture of a chair they admire, and the system will suggest similar styles, colors, or materials. This eliminates guesswork and the need to know specific product names or terms, thereby simplifying the discovery process. Additionally, visual search can help users find complementary items that match the aesthetics of something they already love. This capability not only streamlines the customer’s journey but also opens up new avenues for inspiration, creativity, and product exploration.
5. Voice-Enabled Interactions
Integration with voice assistants (e.g., Alexa, Google Assistant, Siri) and specialized voice-based interfaces allow users to request products, ask for reviews, or complete purchases hands-free and on-the-go.
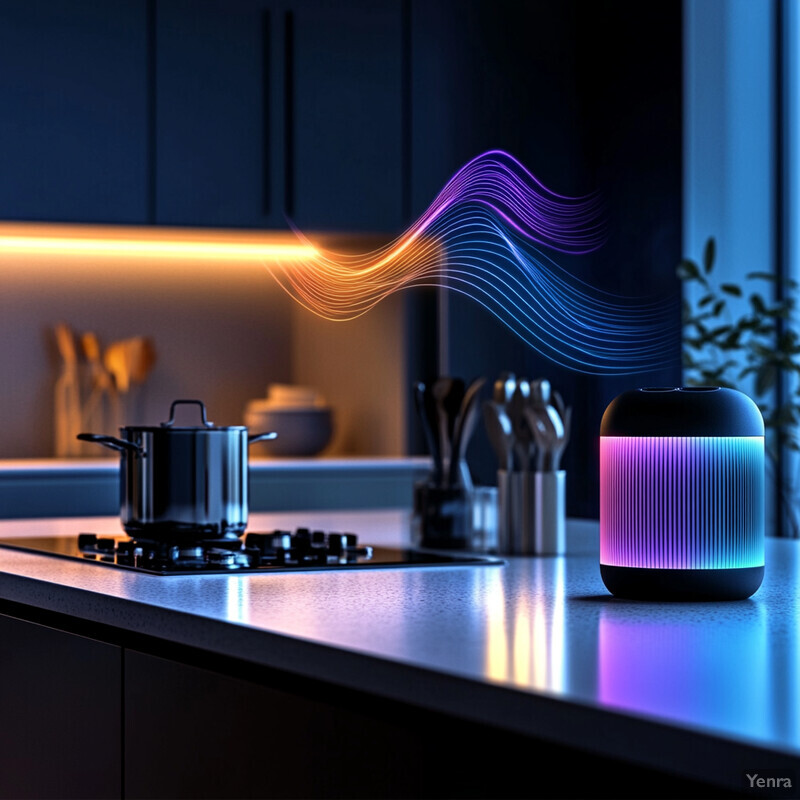
By integrating with popular voice assistants or employing dedicated speech-recognition technologies, AI-driven shopping assistants let customers interact hands-free. Users can request recommendations, add items to their cart, ask for shipping details, or reorder favorite products simply by speaking. This convenience is especially valuable for on-the-go scenarios, busy multitaskers, or those with limited ability to use a keyboard or touchscreen. Voice interfaces also foster more natural conversations, strengthening the sense that the assistant is a helpful companion rather than a static tool. As speech recognition accuracy improves, voice-enabled shopping will become increasingly fluid, intuitive, and user-friendly.
6. Predictive Inventory Tracking
AI algorithms predict stock availability, alert customers before products run out, and suggest suitable alternatives if an item is sold out—improving the reliability of the shopping experience.
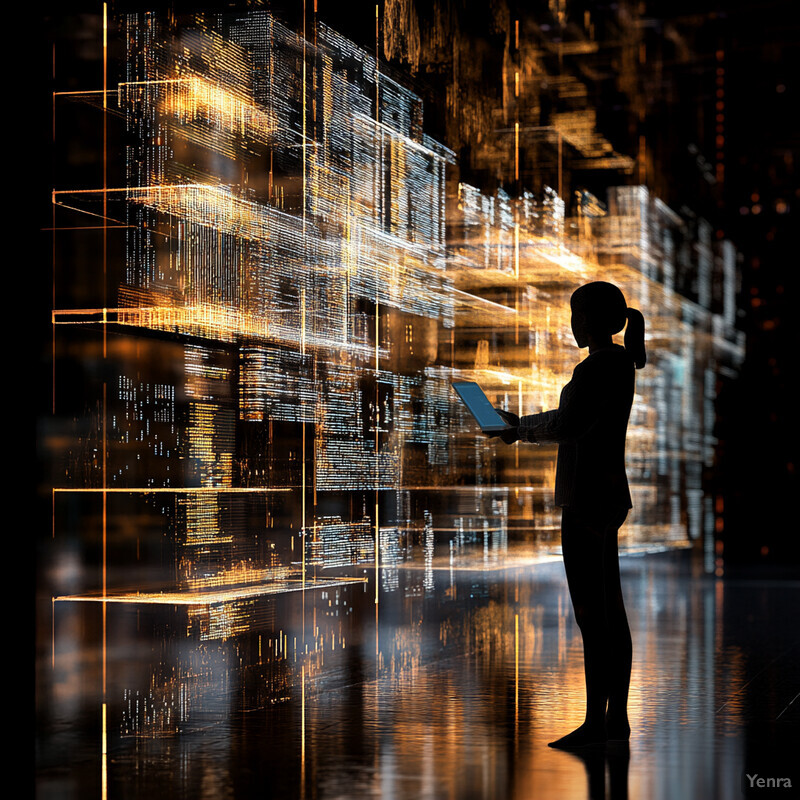
AI can analyze historical sales data, seasonal demand patterns, and real-time market signals to predict when certain products will go out of stock. By alerting customers early, the assistant prevents disappointment and can offer viable substitutes before they run out. This proactive inventory management fosters trust and reliability, as users learn that the assistant can guide them toward in-stock items rather than leading them into dead ends. Moreover, such intelligence helps retailers optimize their procurement and supply chains, ensuring that hot-selling items are restocked promptly and underperforming products are phased out efficiently.
7. Dynamic Pricing Optimization
Machine learning models monitor market data, competition, and demand patterns to adjust prices in real-time, ensuring customers receive competitive offers while maximizing retailer profitability.
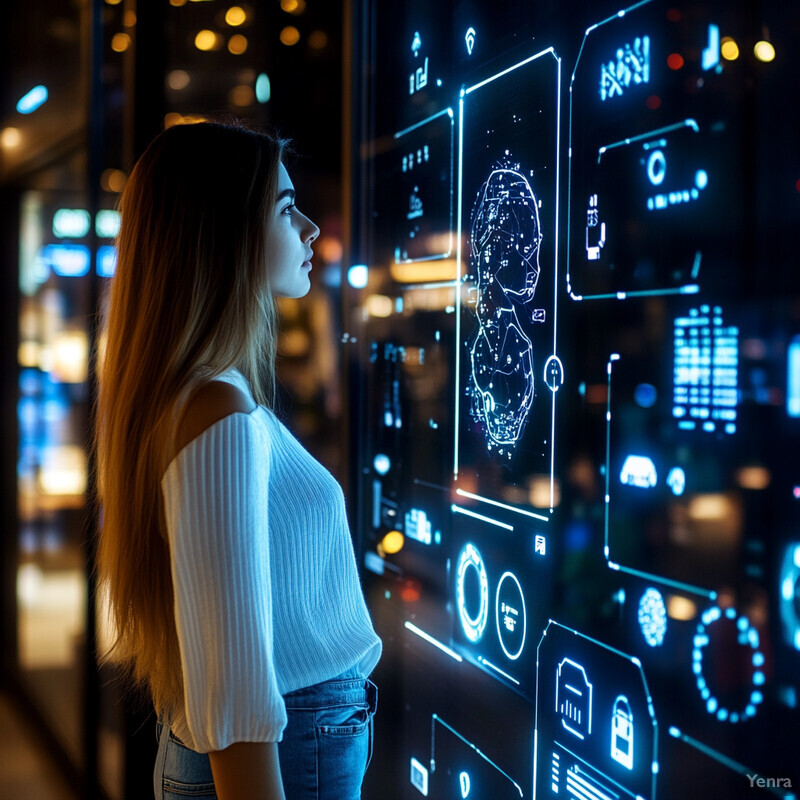
Intelligent pricing algorithms continuously monitor market data, demand fluctuations, and competitive pricing to adjust product costs in real-time. For shoppers, this can mean discovering frequent deals and personalized discounts. For retailers, it ensures pricing remains appealing yet profitable. Over time, the system learns from buyer responses—whether a slight discount boosts sales or a premium markup deters purchases—and refines its approach. This dynamic interplay of supply, demand, and consumer psychology results in a more agile and responsive marketplace, offering each shopper a fair and relevant price point that aligns with their perceived value.
8. Hyper-Localized Suggestions
AI can leverage geolocation data to provide region-specific recommendations, such as items popular in the customer’s city or clothing suggestions tailored to local climate conditions.
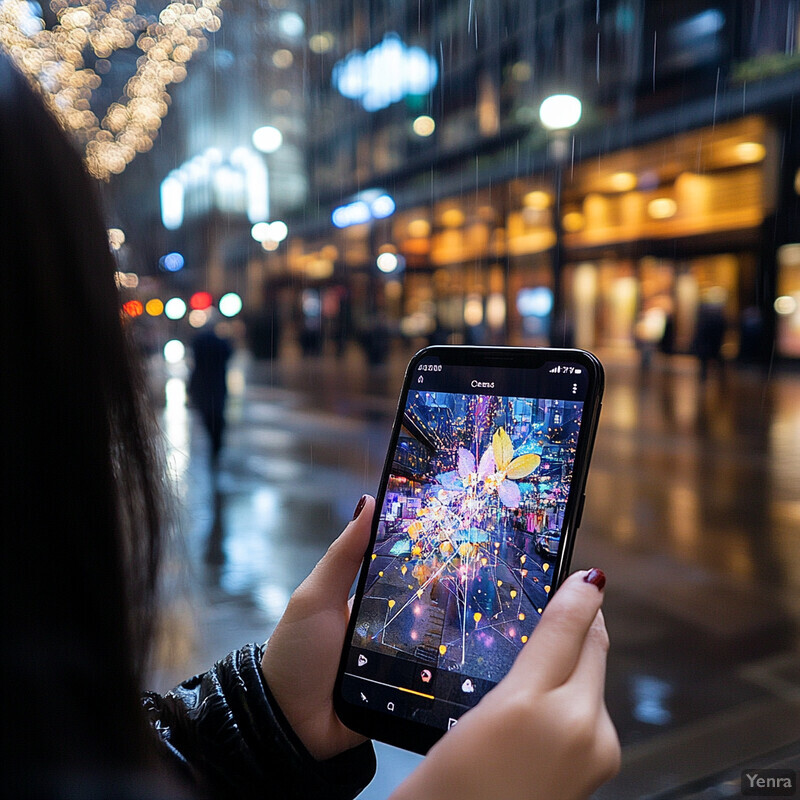
AI-enhanced assistants leverage location data to provide recommendations that match local conditions. For instance, if a customer lives in a rainy region, the assistant might frequently suggest waterproof jackets or umbrellas. In metropolitan areas, it could highlight items popular among urban trendsetters, or if the user is traveling, it might recommend region-specific souvenirs or necessities. This localized approach ensures that the products are not only personally relevant but also geographically and culturally appropriate, thereby creating a richer, more meaningful connection between the user and the marketplace.
9. Behavioral Profiling for Better UX
By analyzing how users browse, linger on certain products, or interact with features, AI refines user profiles and predicts the products they’re most likely to be interested in.
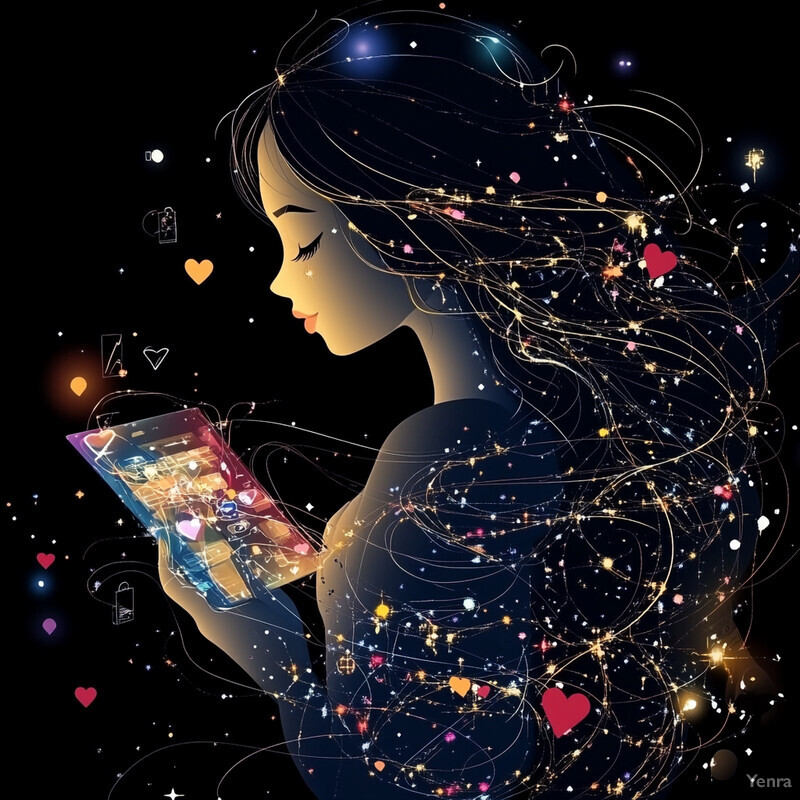
Beyond explicit data like purchases and search history, AI can analyze subtle user behaviors. Time spent viewing certain products, items that consistently appear in abandoned carts, or the types of filters a user applies can all feed into more nuanced profiles. From these patterns, AI infers deeper tastes and motivations, adjusting the user interface to highlight preferred categories, brands, or price ranges. As a result, each interaction with the platform feels increasingly aligned with the user’s natural shopping habits, reducing friction and time-to-purchase while enhancing overall satisfaction.
10. Automated Cross-Selling and Upselling
AI models identify products that naturally complement a chosen item and present them at the right moments, increasing overall value and making the shopping journey smoother.
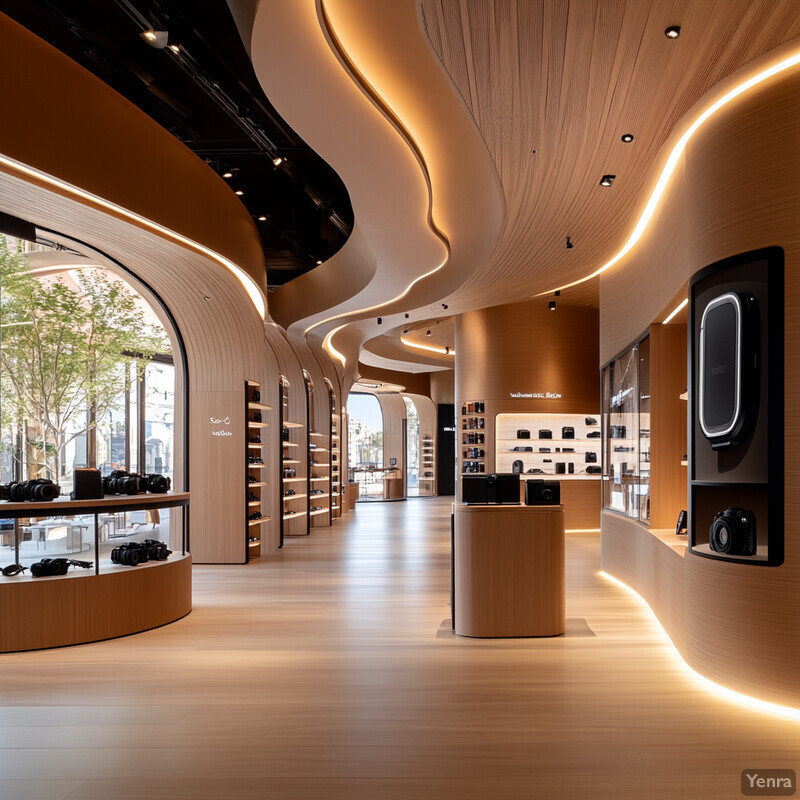
By analyzing product relationships and purchase histories, AI can identify logical complements and upgrades for items currently being viewed or bought. For instance, a customer looking at a digital camera might receive suggestions for memory cards, lenses, and protective cases. Similarly, someone considering a mid-tier laptop could be offered a more powerful model at a modest price difference. This intelligent merchandising strategy feels organic and helpful rather than pushy, as it stems from a precise understanding of the user’s interests and needs. Through well-timed, relevant prompts, the assistant increases the value of each transaction and simplifies the buyer’s research process.
11. Emotion Recognition and Sentiment Analysis
Sentiment analysis tools gauge consumer reactions from reviews or social media to refine recommendations and create more emotionally resonant shopping suggestions.
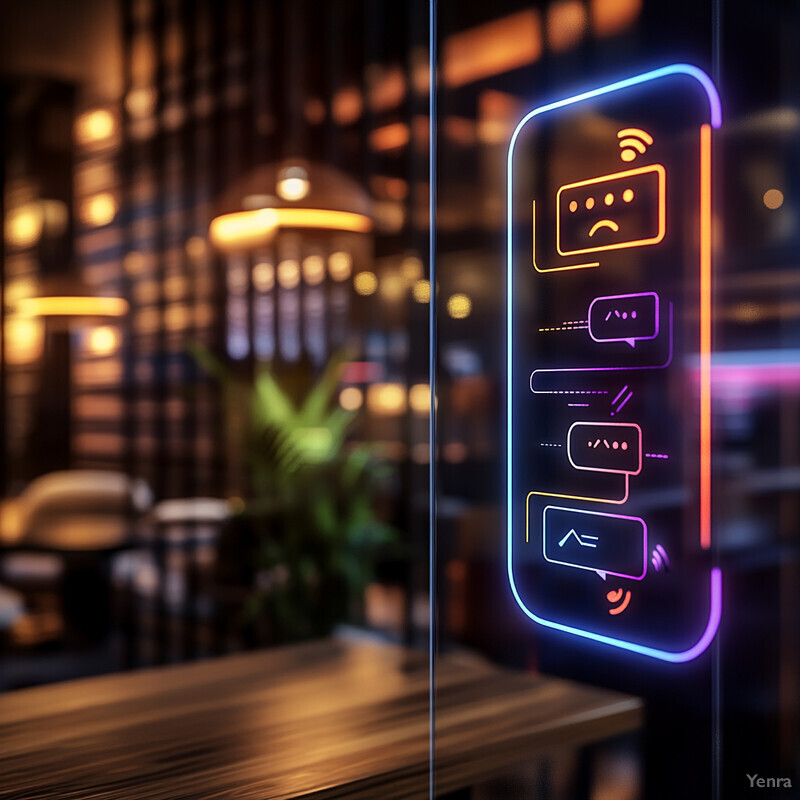
AI can parse social media chatter, user reviews, and customer feedback to gauge how people feel about specific products or trends. By understanding the underlying emotional responses—excitement, skepticism, satisfaction, or disappointment—the assistant refines its recommendations accordingly. Products associated with positive sentiment can be prominently featured, while those receiving negative feedback can be de-emphasized or highlighted with cautionary information. Over time, integrating emotional intelligence into the shopping experience helps build trust, guiding users toward items more likely to delight them and away from potential regrets.
12. Virtual Try-On and Augmented Reality Integration
AR technologies let customers visualize how items would look on them, boosting confidence and reducing returns.
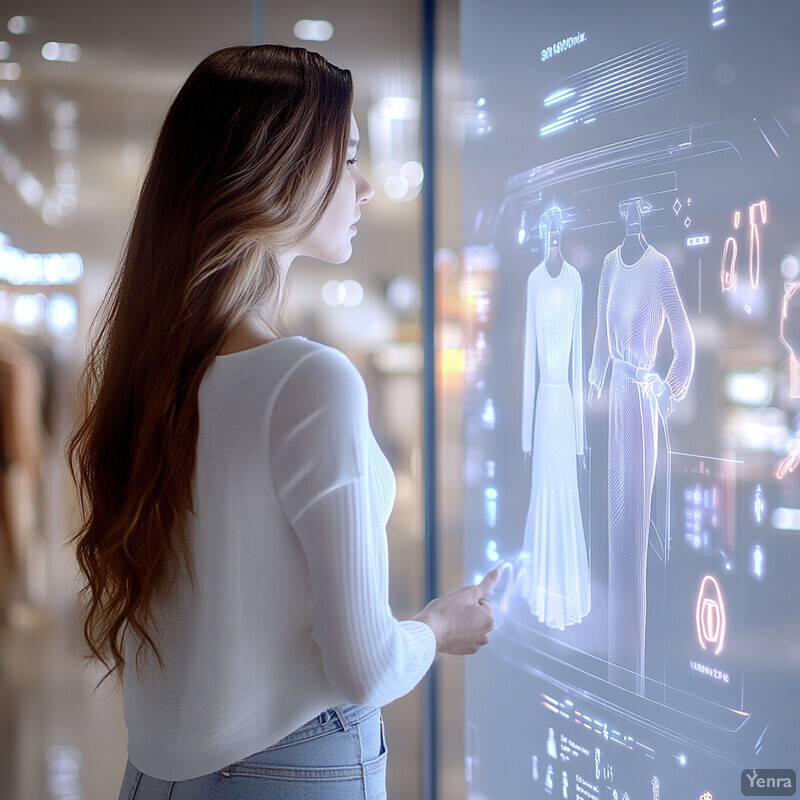
With AR tools, shoppers can visualize how clothing, accessories, or cosmetics will look on their own bodies. AI assists by accurately simulating product fit, color matching, and even texture—eliminating uncertainty and enhancing confidence in online purchases. This immersive, playful experience reduces returns and dissatisfaction, as customers can “try before they buy.” By blending the digital and physical realms, the assistant becomes a personal stylist that empowers the user to experiment freely. Over time, these simulations become more realistic and detailed, reshaping online shopping into a more tangible, sensory experience.
13. Real-Time Trend Analysis
AI scans social media, forecasts, and industry reports to predict hot trends, offering shoppers timely and popular product assortments.
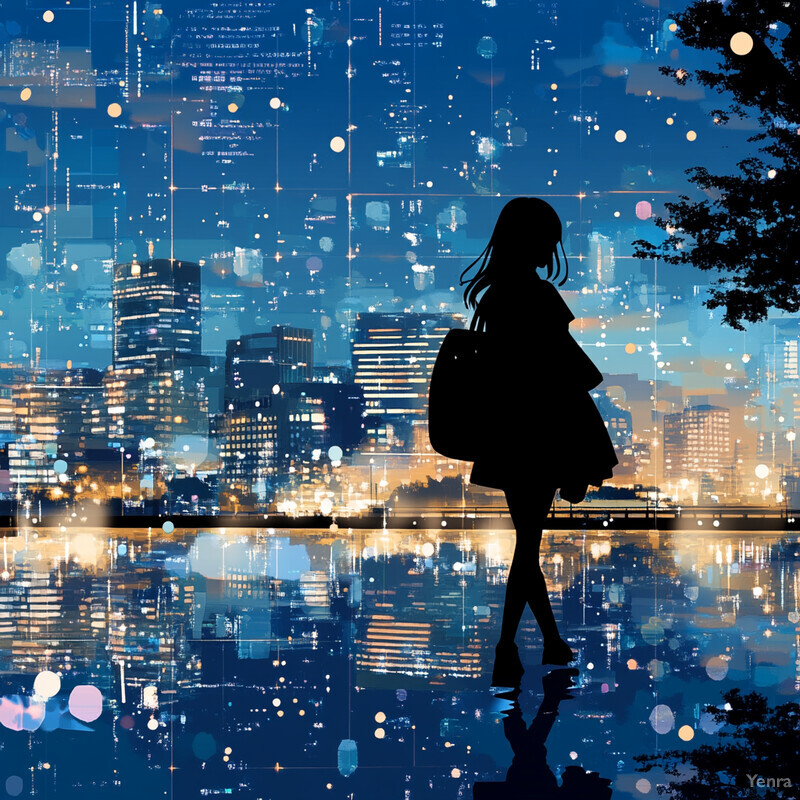
The fashion and retail landscapes evolve rapidly, influenced by global events, pop culture, and seasonal shifts. AI constantly scans social media, news outlets, and industry reports to identify emerging styles or bestsellers. The assistant uses these insights to keep product recommendations fresh and relevant, ensuring that customers are always aware of the latest releases or upcoming must-haves. By anticipating what’s next and aligning suggestions with current public interest, these systems help users stay ahead of the curve, providing an ever-evolving, trend-savvy shopping experience.
14. Intelligent Chatbots for Immediate Assistance
Sophisticated chatbots handle inquiries and provide round-the-clock support, making the shopping experience more personal and responsive.
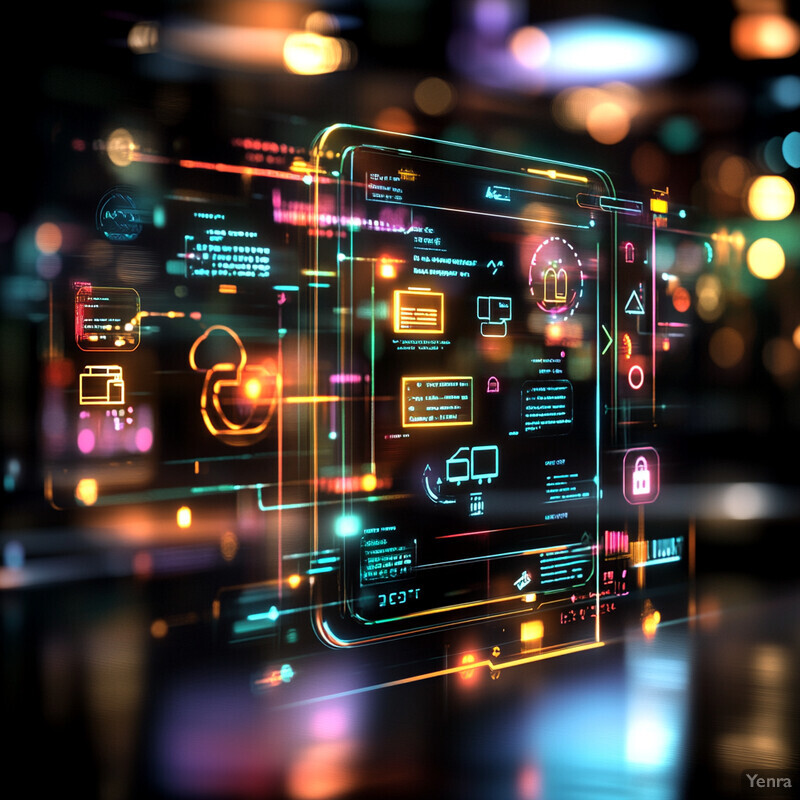
Conversational AI chatbots provide round-the-clock customer support, answering questions about shipping, returns, product availability, and technical issues. Beyond basic FAQs, they can guide shoppers through complex decision-making processes—offering styling advice, comparing product features, or suggesting gift ideas. These bots learn from each interaction, improving the quality and relevance of their support over time. Instead of navigating tedious help menus, users interact with a responsive, knowledgeable assistant that streamlines problem-solving and adds a human touch to digital retail.
15. Lifecycle-Based Customer Journey Mapping
AI tracks the entire customer journey, providing consistent recommendations and support at each stage.
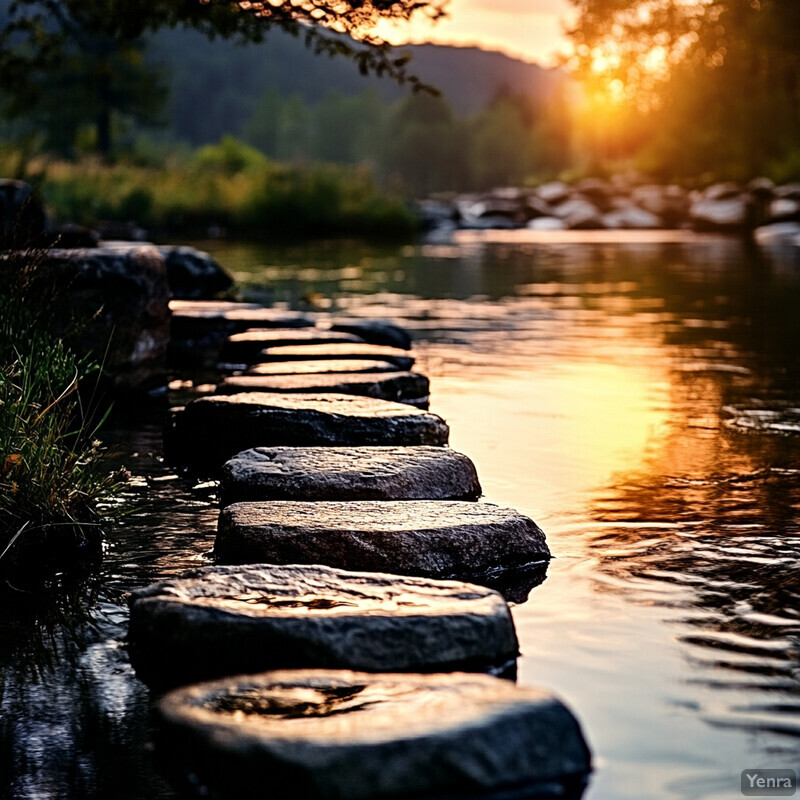
AI examines each step of the customer journey, from initial product discovery and consideration to purchase and post-purchase engagement. By understanding where customers drop off, what motivates a final purchase, and how brand loyalty evolves, the assistant provides proactive guidance at every stage. It might suggest a first-time buyer discount, remind a regular customer about their loyalty points, or recommend a warranty extension after several months of use. This holistic approach ensures that the shopping experience isn’t just about individual transactions but about fostering a long-term relationship with the consumer.
16. Automated Content Generation and Curation
Machine learning creates fresh product descriptions, style guides, and lookbooks, reducing manual effort and keeping content up-to-date.
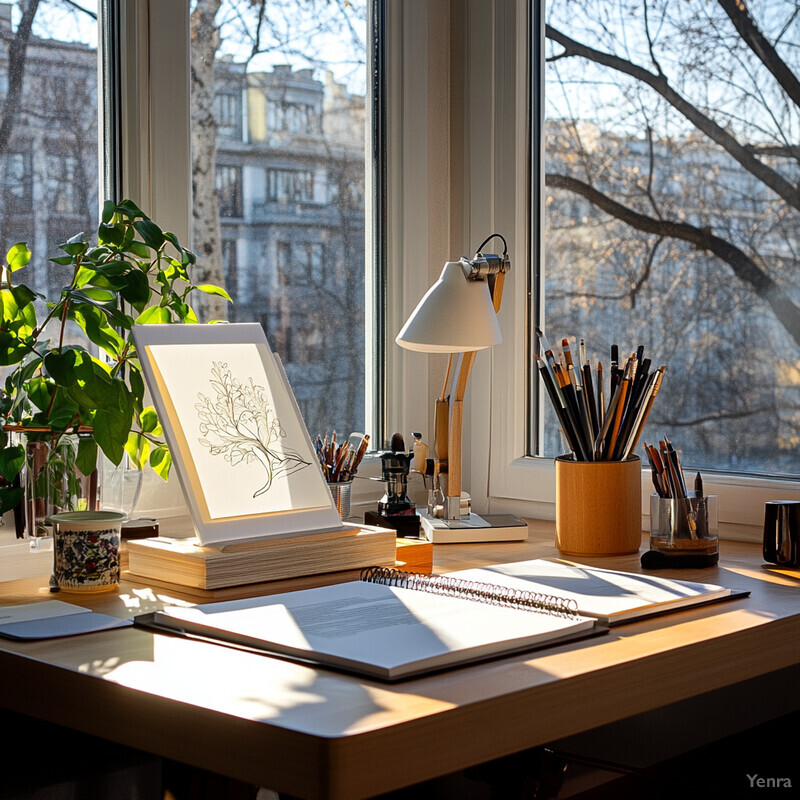
Machine learning models can automatically produce product descriptions, styling tips, blog articles, and lookbooks that align with each user’s interests. By carefully selecting relevant language and imagery, these AI systems ensure that every piece of content feels personalized and up-to-date. This automation not only cuts down on manual work for retailers but also keeps the shopping environment dynamic and informative. Over time, shoppers gain access to a wealth of curated insights and inspirations, encouraging them to engage more deeply with the brand and explore new product categories.
17. Enhanced Fraud Detection and Security
AI identifies unusual purchase patterns and suspicious behavior, creating a safer shopping environment.
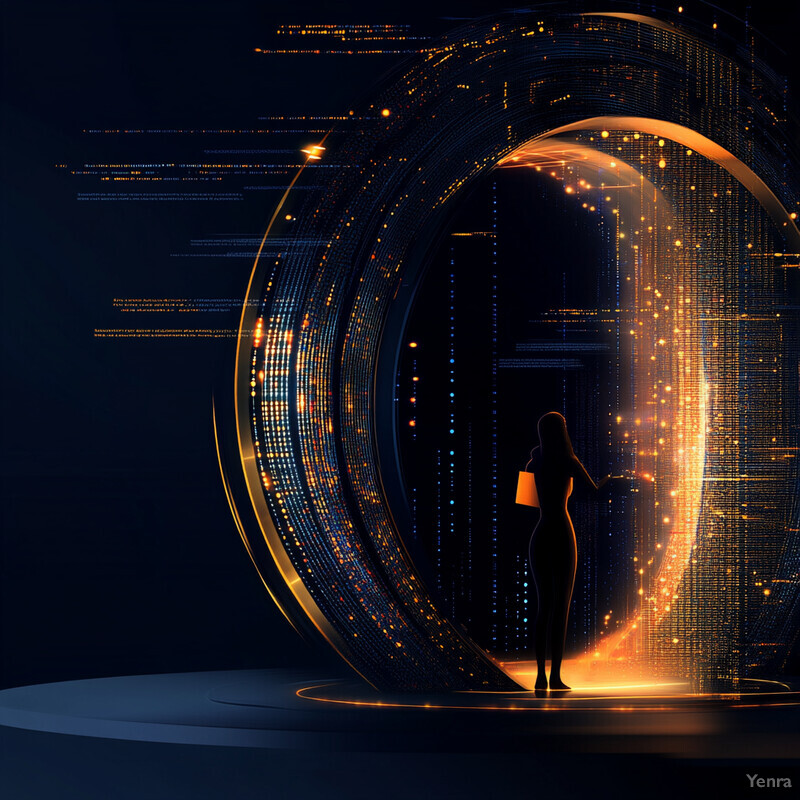
AI excels at recognizing anomalies in buying patterns, which helps detect fraudulent transactions quickly. For example, sudden bulk purchases from an unfamiliar location or the repeated use of declined credit cards can trigger automated investigations. As a result, genuine shoppers benefit from a safer environment, while bad actors find it harder to exploit vulnerabilities. This security advantage reassures customers that their personal and financial information is protected, fostering trust and confidence in the platform.
18. Adaptive Interfaces and Accessibility
AI-driven personalization adjusts interfaces for user comfort, enhancing accessibility for all shoppers.
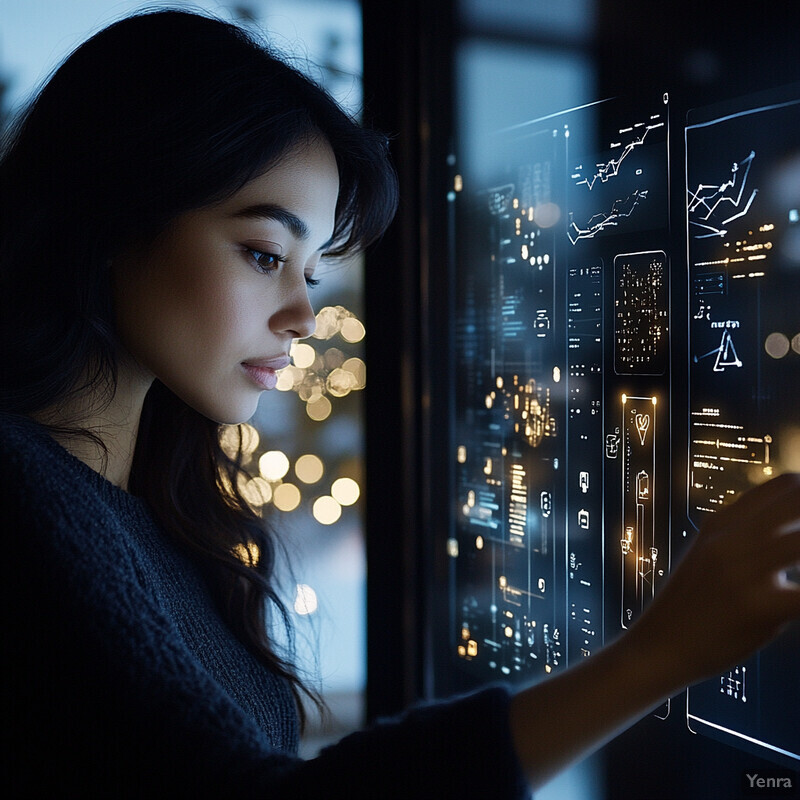
Personal shopping assistants can adjust their interfaces dynamically based on user preferences or needs. AI might detect that a user spends more time on their mobile phone and rearrange the layout for better touch navigation. Similarly, for users with visual impairments, it can enlarge text and incorporate voice feedback. These adaptive design features make online shopping more inclusive, ensuring that everyone can find what they need comfortably. As the system learns from engagement patterns, it continues to refine the user interface, guaranteeing a consistently accessible and friction-free experience.
19. Optimized Search and Navigation
AI refines on-site search results, understanding user intent to help shoppers find what they need quickly and easily.
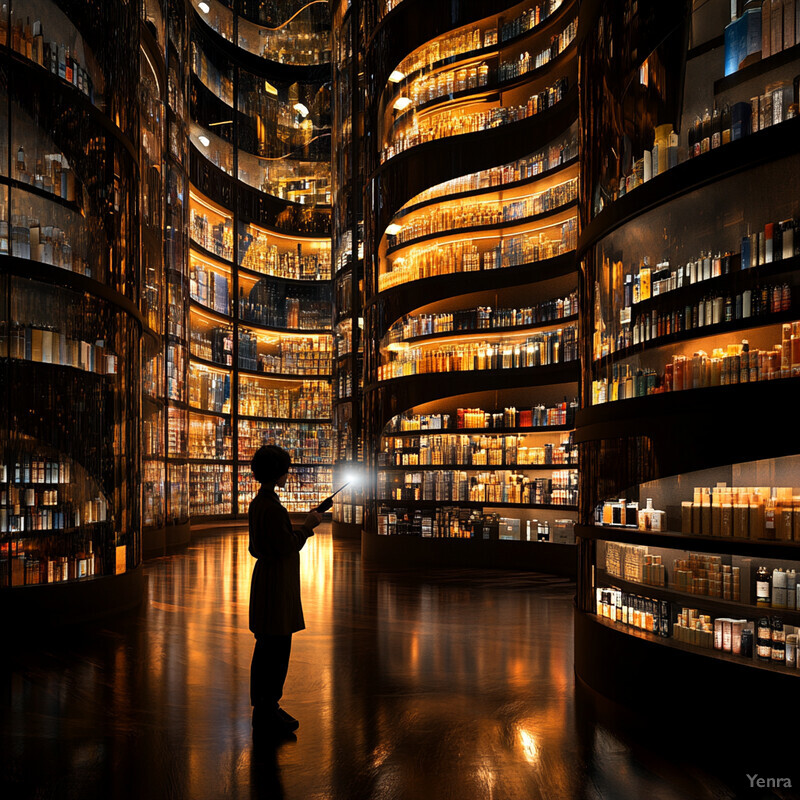
Traditional filters and sorting options can feel clunky, but AI improves search and navigation by understanding user intent and tailoring results more precisely. It can highlight relevant categories, predict keywords, and correct spelling errors automatically. Furthermore, the assistant can learn from previous search queries, offering shortcuts to frequently visited sections or preferred brands. By making it effortless to sift through thousands of products, AI-driven navigation ensures that users quickly find what they’re looking for—cutting down on frustration and encouraging exploration.
20. Continuous Learning and Improvement
AI systems learn from user feedback, purchases, and trends, continuously refining recommendations and interfaces over time.
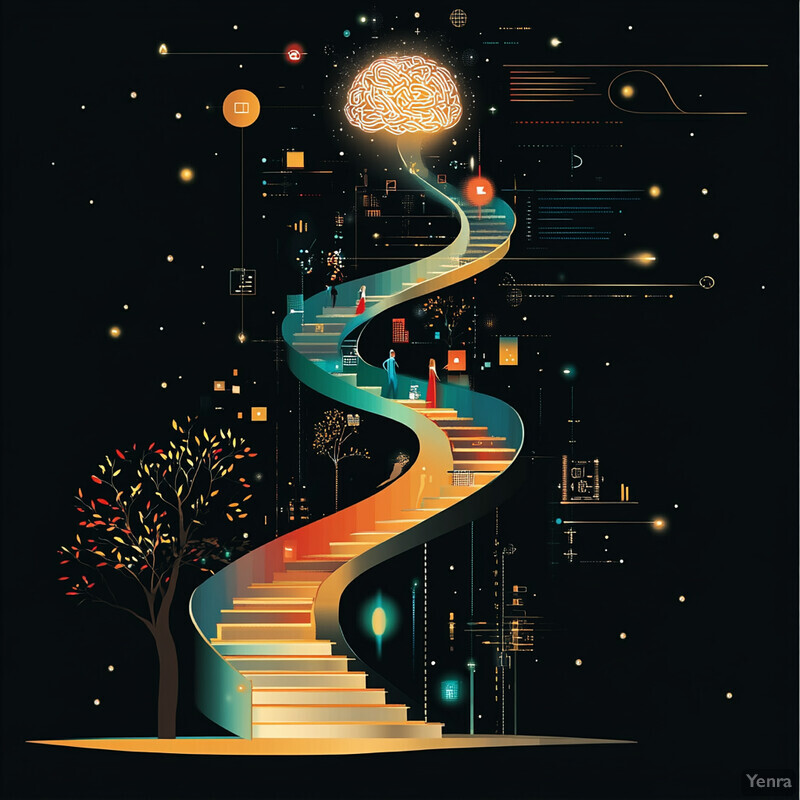
Central to all these capabilities is the AI’s ability to learn iteratively. With each user interaction, preference update, market trend, and piece of feedback, the system refines its algorithms. Over time, it becomes increasingly adept at understanding user needs, anticipating challenges, and delivering precisely what shoppers seek. This virtuous cycle of learning ensures that the personal shopping assistant never grows stale or outdated, always evolving alongside shifts in consumer behavior and industry developments. Ultimately, this continuous improvement cements the assistant’s value as a reliable and ever-advancing tool for modern consumers.