1. Anomaly and Outlier Detection
AI-driven statistical and machine learning models can quickly identify unusual patterns in financial transactions, flagging anomalies that may indicate errors or potential fraud for further investigation.
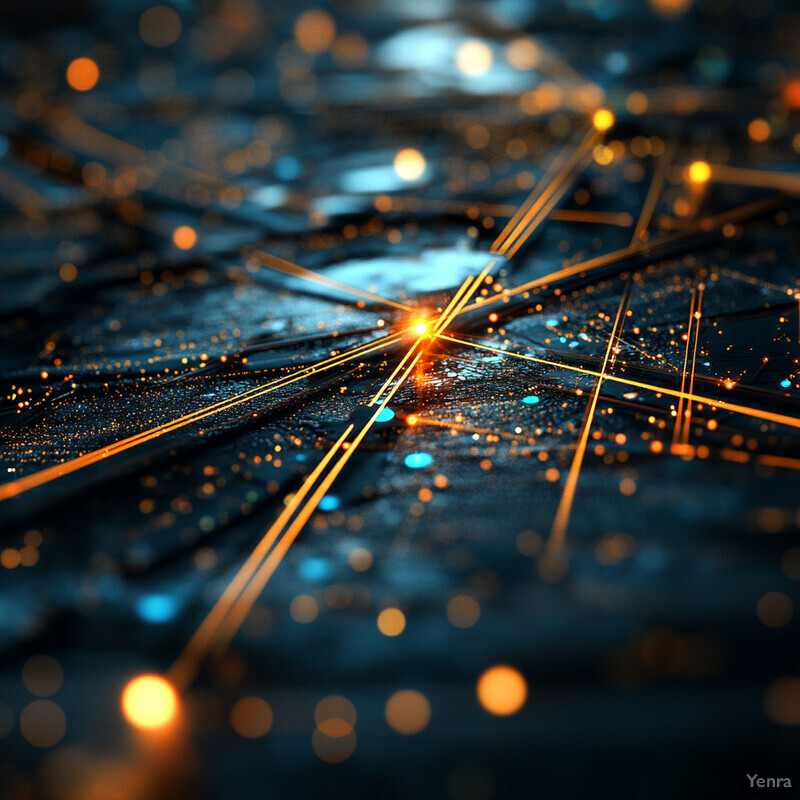
AI-enhanced anomaly detection utilizes advanced statistical modeling, machine learning, and pattern recognition techniques to isolate unusual activities in financial datasets. Traditionally, auditors had to rely on heuristic rules and random sampling to detect irregularities, which were time-consuming and prone to human oversight. By continuously scanning massive volumes of transactions, AI can identify subtle patterns that deviate from historical norms or established benchmarks. For instance, it can highlight sudden spikes in a certain expense category, unusual changes in customer payment patterns, or suspicious journal entries that occur at odd hours. This capability empowers auditors to rapidly pinpoint potential red flags, reduce false negatives, and increase the likelihood of catching errors and fraud early in the process.
2. Real-Time Transaction Monitoring
AI systems can continuously process financial data streams and raise alerts in near real-time, rather than relying solely on periodic manual checks, improving the speed and effectiveness of auditing.
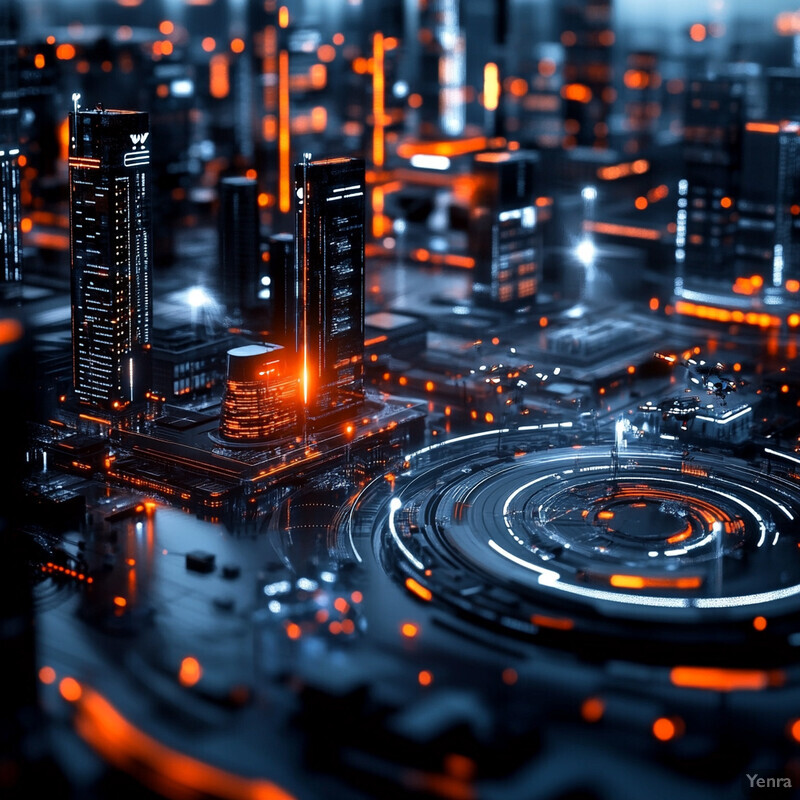
Instead of periodic or batch-based auditing, AI systems can evaluate and flag transactions as they occur. This real-time capability allows auditors and compliance teams to intervene swiftly when issues arise, rather than retroactively investigating anomalies long after the fact. Through continuous data feeds and streaming analytics, these AI systems can apply complex business rules and predictive models on the fly. The result is immediate alerts for suspicious activities—such as abnormally high invoice amounts or unauthorized vendor payments—enabling auditors to address potential problems proactively. By integrating seamlessly with enterprise resource planning (ERP) systems and financial software, real-time monitoring reduces the time between detection and corrective action, bolstering the overall robustness of the financial control environment.
3. Automated Classification of Expenses and Revenues
Natural Language Processing (NLP) and machine learning can categorize and tag financial entries automatically, reducing the manual effort associated with classifying expenditures or revenues and lowering the risk of human error.
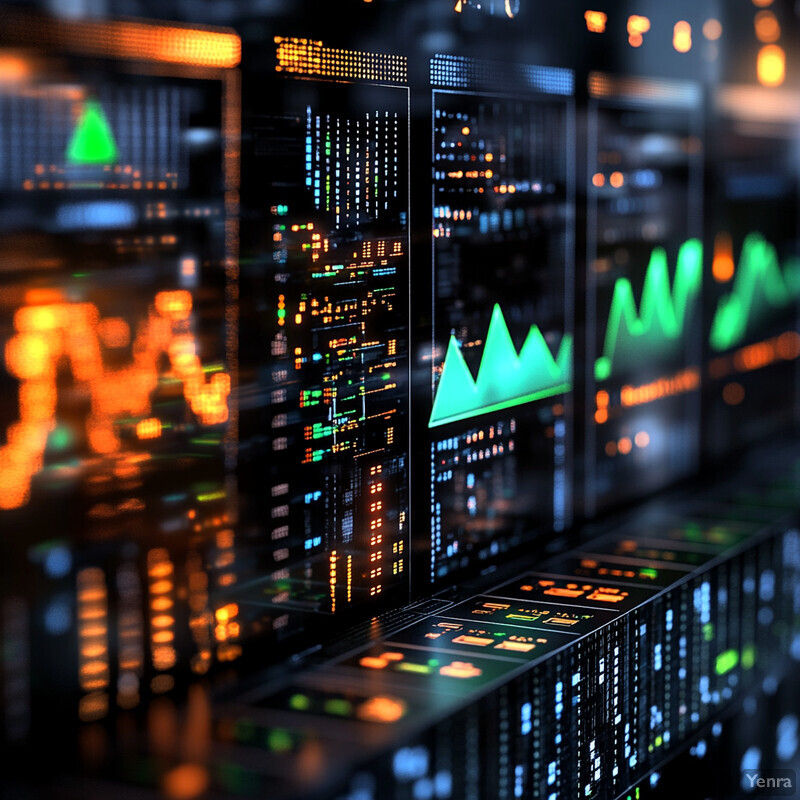
Classifying financial transactions can be a painstaking endeavor when done manually, as it often involves going through vast amounts of supporting documents and following complex classification rules. AI-driven Natural Language Processing (NLP) models and machine learning classifiers can rapidly and accurately categorize expenses, revenues, and other financial entries. For example, they can read invoice descriptions, match vendor categories, and apply consistent categorization logic across multiple departments and regions. By automating this labor-intensive process, auditors can rely on a more uniform classification of financial data, reducing the risk of human error and freeing them to focus on higher-level analytical tasks, such as investigating unusual variance in expense patterns.
4. Process Mining and Workflow Optimization
AI-driven process mining tools can examine transaction logs to map out financial workflows, identify inefficiencies, and suggest improvements in internal controls, thereby streamlining the audit process.
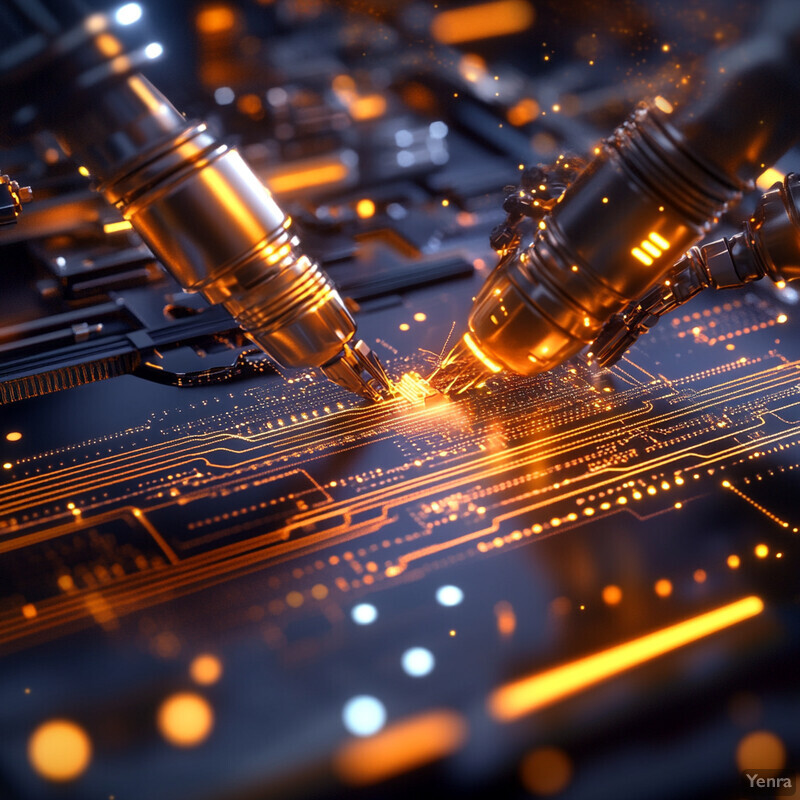
AI-powered process mining tools analyze system logs and transactional data to reconstruct the actual flow of financial operations, from invoice receipt to payment approval. They highlight inefficiencies, bottlenecks, and deviations from standard processes. For instance, if a particular approval step is consistently bypassed or if payments to certain vendors are unusually delayed, the AI system will bring these process irregularities to the auditor’s attention. These insights enable auditors to refine internal controls, streamline workflows, and ensure better compliance with established financial policies. Over time, continuous process mining can lead to more transparent, efficient, and auditable financial operations, reducing errors and potential fraud opportunities.
5. Predictive Analytics for Risk Assessment
Advanced AI models can forecast potential future financial risks—such as liquidity issues, credit defaults, or volatility—enabling auditors to proactively address concerns before they escalate.
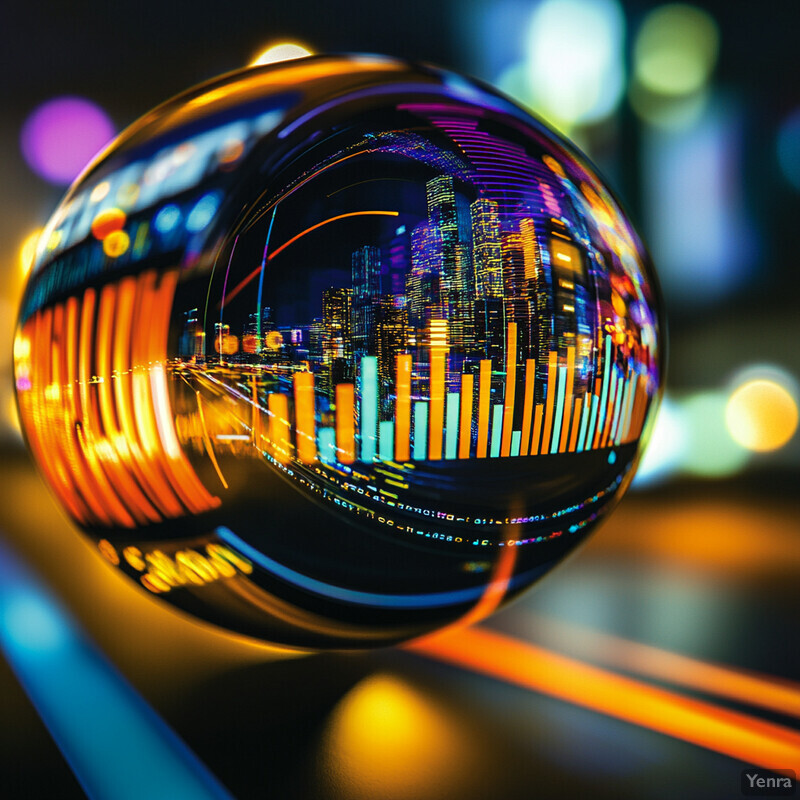
Auditors increasingly need to anticipate future risks, not just evaluate past performance. AI-driven predictive analytics can forecast potential financial pitfalls by analyzing historical data patterns, external market indicators, macroeconomic trends, and even sentiment from news sources or social media. For example, machine learning models can predict the likelihood of credit defaults, liquidity crunches, or spikes in commodity prices that could affect the company’s bottom line. Armed with these predictive insights, auditors can prioritize areas of high risk, make proactive recommendations to management, and design targeted audit procedures that address emerging vulnerabilities before they materialize into significant financial losses.
6. Fraud Detection and Prevention
AI’s pattern recognition capabilities help detect subtle indicators of fraud—such as ghost vendors, collusion, or rapidly shifting account balances—at a scale and speed far beyond manual auditing methods.
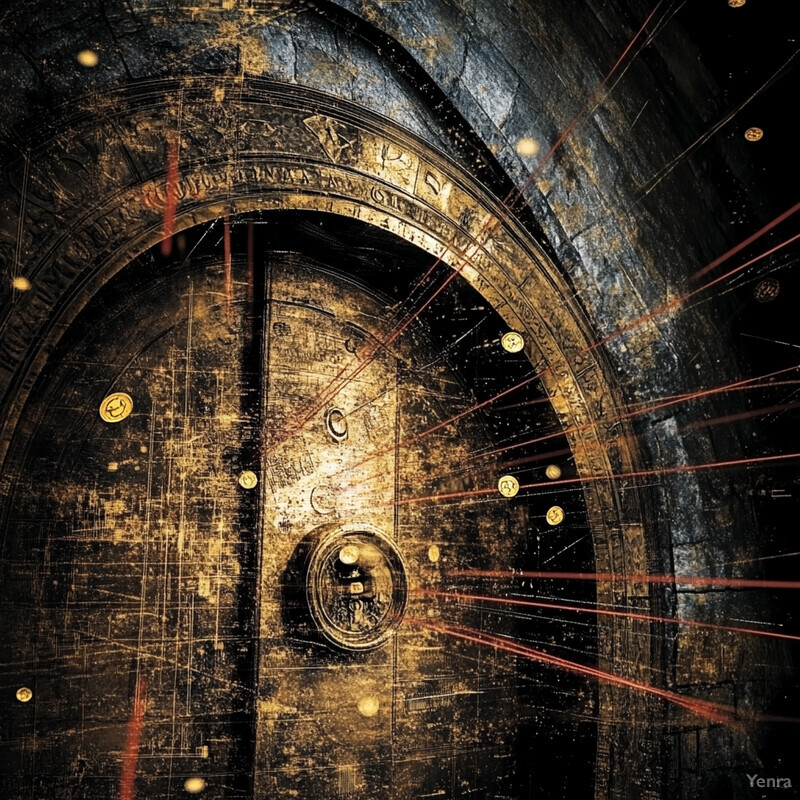
Fraud can be subtle, evolving quickly to evade traditional controls. Advanced AI models can detect hidden relationships between entities, such as vendors and employees, or identify patterns that are commonly associated with fraudulent activities, like splitting large invoices into multiple smaller ones to stay under certain approval thresholds. By integrating various data sources and employing unsupervised learning techniques, AI tools can uncover sophisticated fraud schemes that might otherwise slip through conventional audits. This heightened ability to identify wrongdoing not only protects an organization’s finances and reputation but also strengthens the overall integrity of the internal control environment.
7. Automated Reconciliations
Intelligent automation tools can match financial records across multiple systems, reconciling bank statements, invoices, and general ledgers with minimal human intervention, increasing accuracy and efficiency.
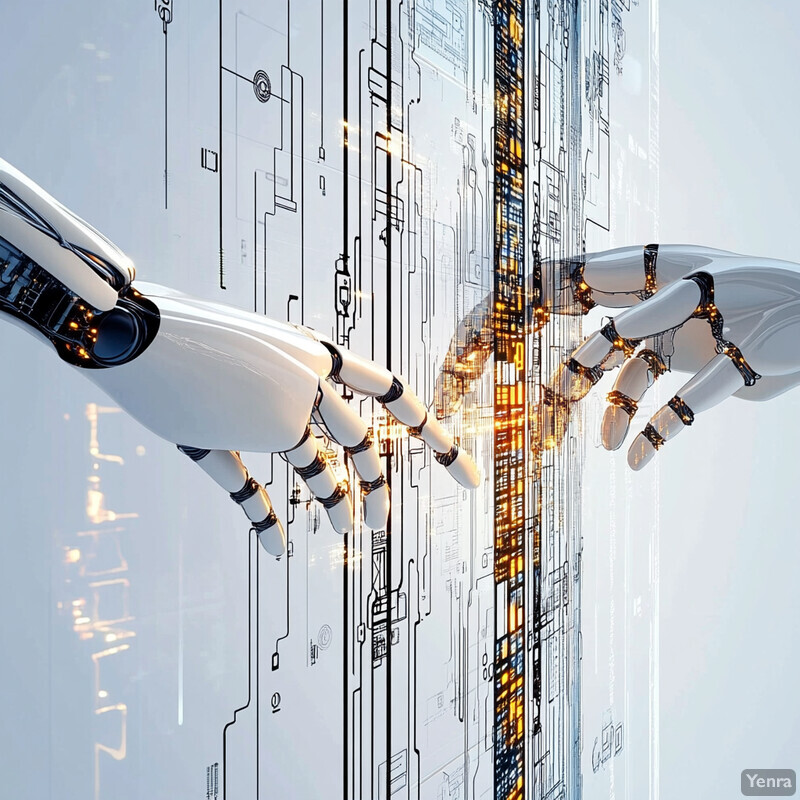
Reconciliation is a critical yet time-consuming activity for auditors, involving the matching of data from different sources—such as bank statements, general ledgers, and subsidiary systems—to ensure that recorded balances align. AI-powered reconciliation tools utilize pattern matching, fuzzy logic, and machine learning to automatically match entries, detect discrepancies, and pinpoint the reasons behind mismatches. This approach significantly reduces the number of manual corrections auditors must make and speeds up the month-end close process. Ultimately, automated reconciliations lead to faster, more accurate financial reporting and free up auditors to focus on more complex analytical reviews.
8. Continuous Assurance and Auditing
AI allows for continuous auditing practices, where financial data is monitored and evaluated continuously, rather than waiting for monthly or quarterly reporting cycles, improving timeliness of insights.
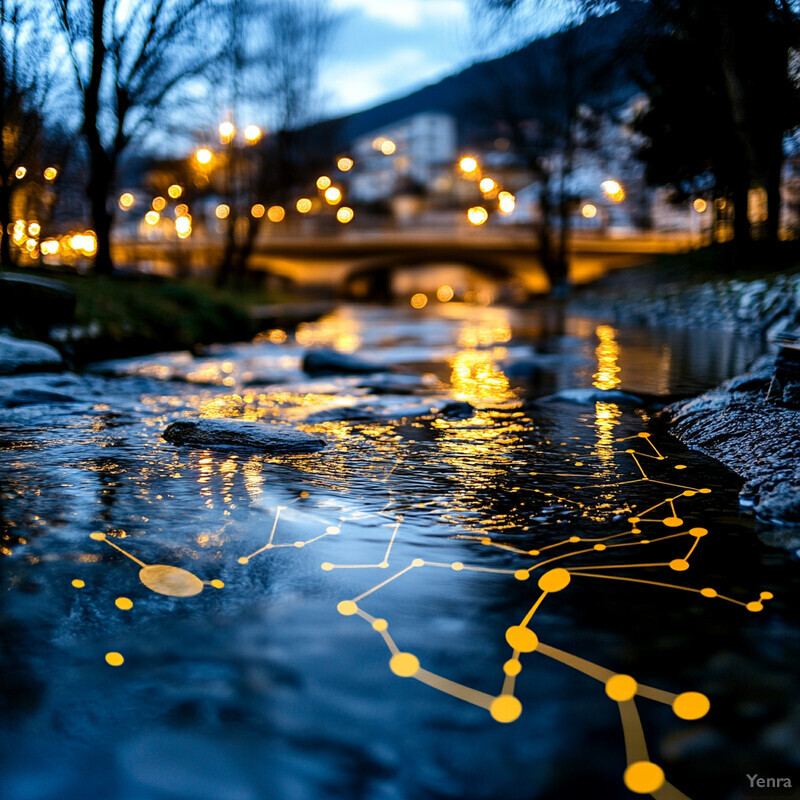
Traditional audits often occur on a periodic basis—monthly, quarterly, or annually—leaving extended periods during which errors or fraud can accumulate undetected. By leveraging AI, organizations can shift to a continuous auditing model, where financial data streams are analyzed as they are generated. This model provides ongoing assurance, enabling auditors to detect issues promptly and respond in near real-time. Continuous auditing also leads to better risk management and increased transparency. Instead of reviewing large datasets after a delay, auditors can keep a finger on the pulse of the organization’s financial health, ensuring that stakeholders are always informed of current conditions.
9. Natural Language Processing for Document Review
NLP can interpret large volumes of contracts, invoices, and legal documents, extracting key financial and contractual terms. This reduces the time auditors spend reading and allows for more thorough, large-scale document analysis.
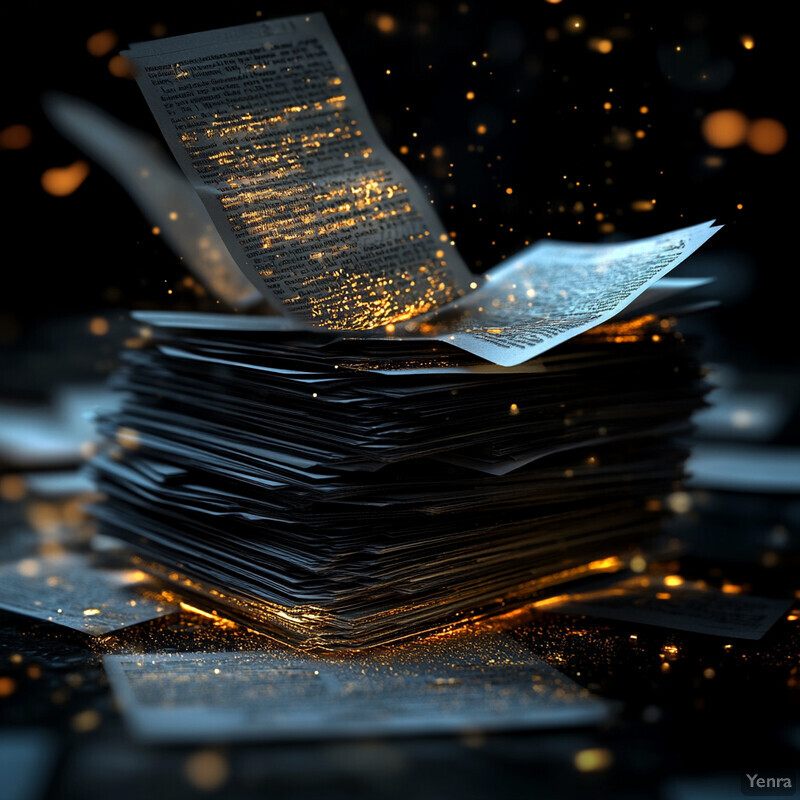
Large volumes of contracts, purchase orders, and invoices often need careful examination to ensure compliance with accounting standards and contractual obligations. NLP-powered AI solutions can extract relevant financial information, key terms, and risk indicators from these documents at scale. For instance, they can identify payment terms in supplier contracts or locate clauses that conflict with organizational policies. By surfacing these insights quickly, auditors can perform more thorough and comprehensive reviews of documentation without being overwhelmed by reading every line. This increased efficiency ensures more reliable compliance checks and supports informed decision-making.
10. AI-Enhanced Sampling Techniques
Instead of manual or random sampling, AI can intelligently select statistically significant samples and higher-risk items to audit, maximizing audit quality while minimizing workload.
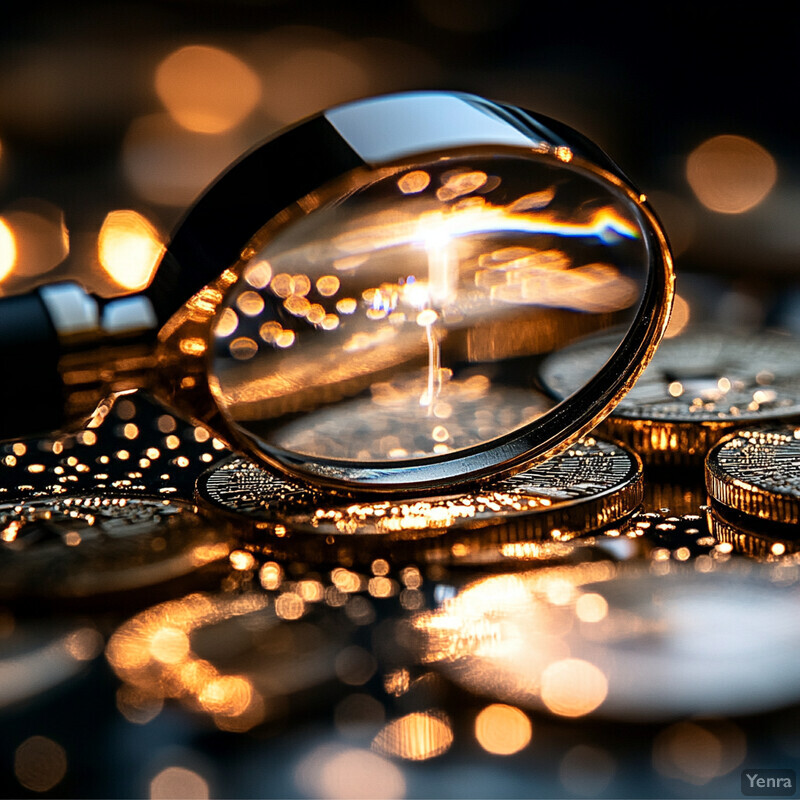
Sampling is a core audit technique but selecting the right samples to verify can be challenging. AI uses statistical modeling and machine learning to determine which transactions or accounts represent higher risks, focusing auditor attention where it matters most. Instead of random or judgment-based sampling, auditors can rely on data-driven intelligence to select transactions that are statistically more likely to contain errors or irregularities. This targeted approach improves audit quality by increasing the probability of detecting misstatements and reduces the time spent checking low-risk items that are unlikely to yield any findings.
11. Sentiment Analysis and Compliance Audits
By analyzing communication records—emails, chat logs, and call transcripts—AI can detect sentiment shifts and potential regulatory compliance violations, giving auditors leads on where to focus deeper investigation.
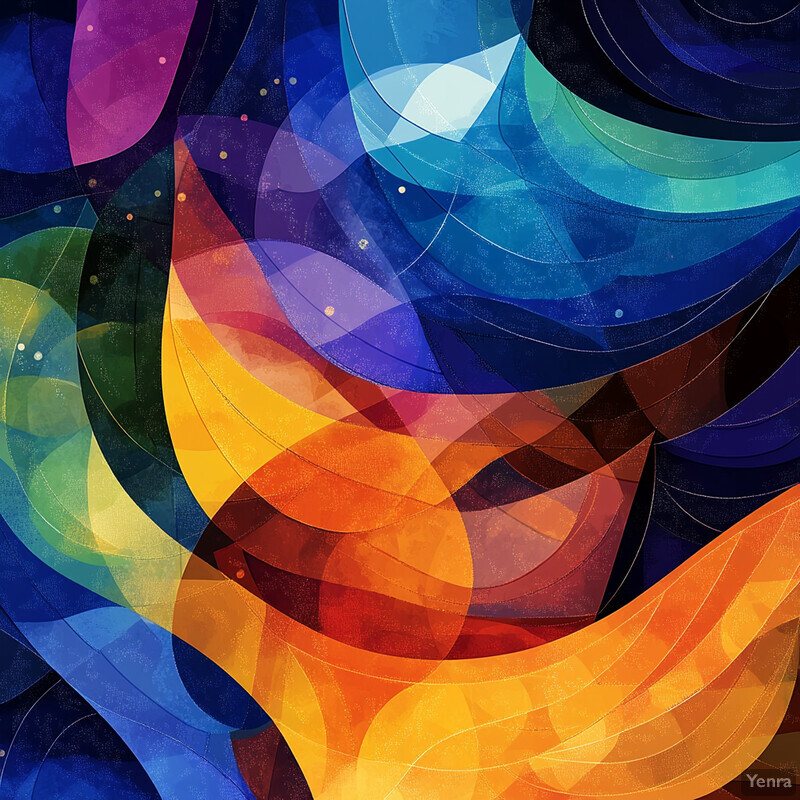
Beyond structured financial data, auditors can glean insights from unstructured communication records, such as emails, chat transcripts, and call logs. AI-driven sentiment analysis and text mining can identify patterns of potential misconduct, regulatory non-compliance, or internal control weaknesses. For instance, a sudden increase in negative sentiment in emails discussing financial reporting could signal underlying issues that need to be examined. By correlating these insights with financial data, auditors gain a more holistic understanding of the organization’s risk environment, enabling them to intervene earlier and more effectively.
12. Automated Regulatory Updates and Compliance Checks
AI-driven systems can track changes in financial regulations and standards globally, ensuring that auditing processes and checklists remain updated, thus reducing the risk of non-compliance.
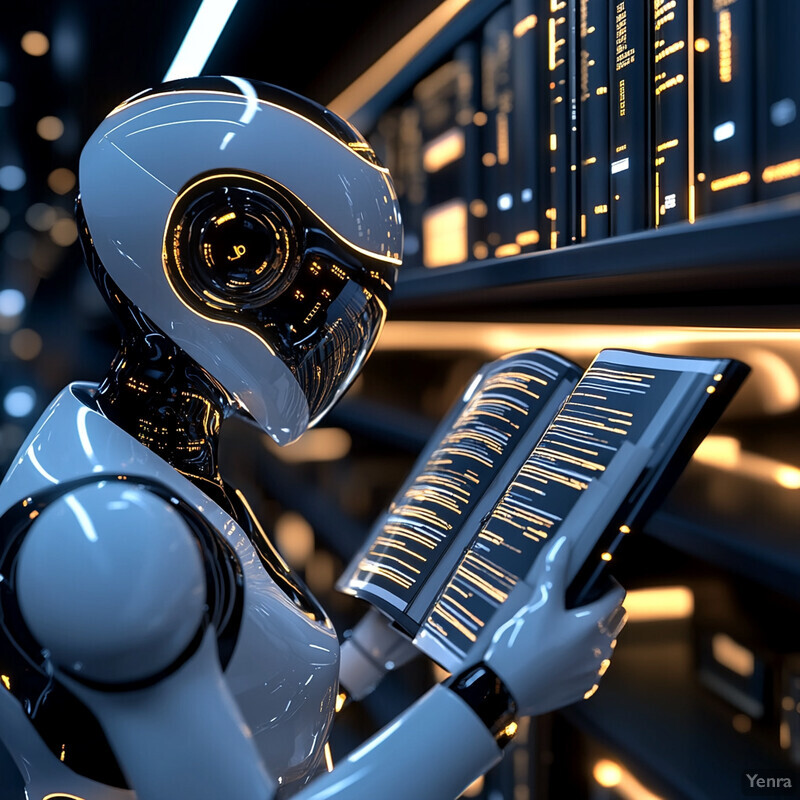
Financial regulations and accounting standards change frequently, varying across jurisdictions and industries. AI-powered compliance tools continuously monitor regulatory databases, professional guidelines, and news sources to stay informed of updates. They can automatically adjust audit checklists, control frameworks, and risk models accordingly. This proactive compliance approach reduces the risk of non-compliance and regulatory penalties. By integrating these updates into the auditing process, AI ensures that auditors are always working with the latest requirements, freeing them from the manual burden of tracking every regulatory development.
13. Scalable Data Integration
AI tools can integrate and analyze data from multiple, disparate systems—ERP, CRM, banking platforms—without extensive manual intervention, supporting a more holistic and integrated audit.
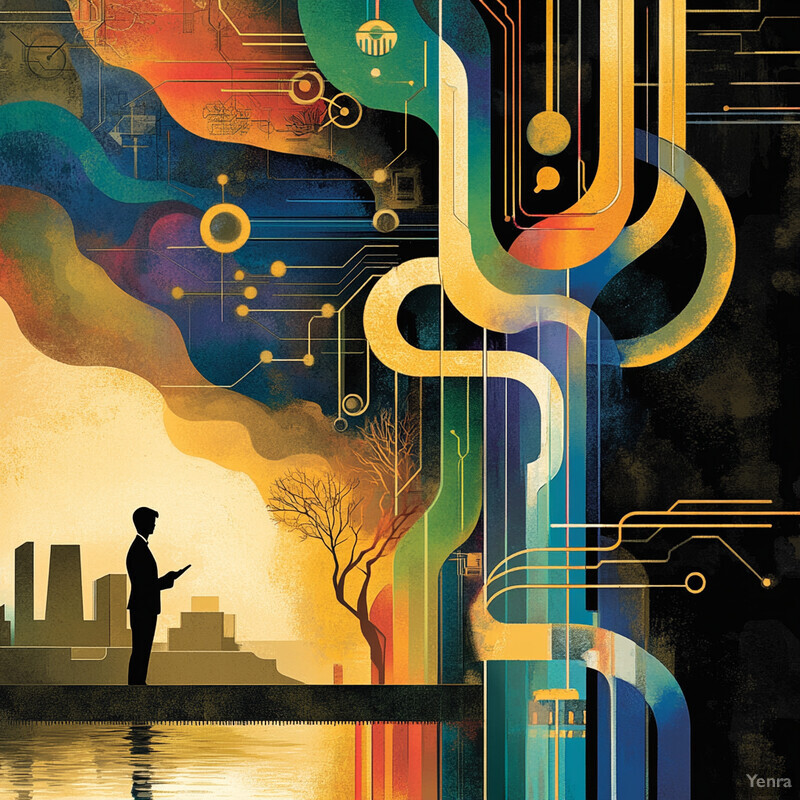
Modern organizations store financial information across multiple platforms—ERP systems, CRM solutions, inventory management tools, and external banking portals. Consolidating this information into a coherent audit dataset can be laborious. AI-driven integration tools leverage APIs, data mapping, and machine learning-based data normalization to unify these disparate sources automatically. This holistic view enables auditors to understand the entire financial landscape without manually stitching together reports. The ability to scale data integration ensures that even as a company grows or diversifies its operations, the auditing function remains efficient and comprehensive.
14. Robotic Process Automation (RPA) for Routine Tasks
Combining RPA with AI allows for the complete automation of repetitive tasks—such as invoice verification or ledger entry—freeing auditors to focus on higher-value, analytical work.
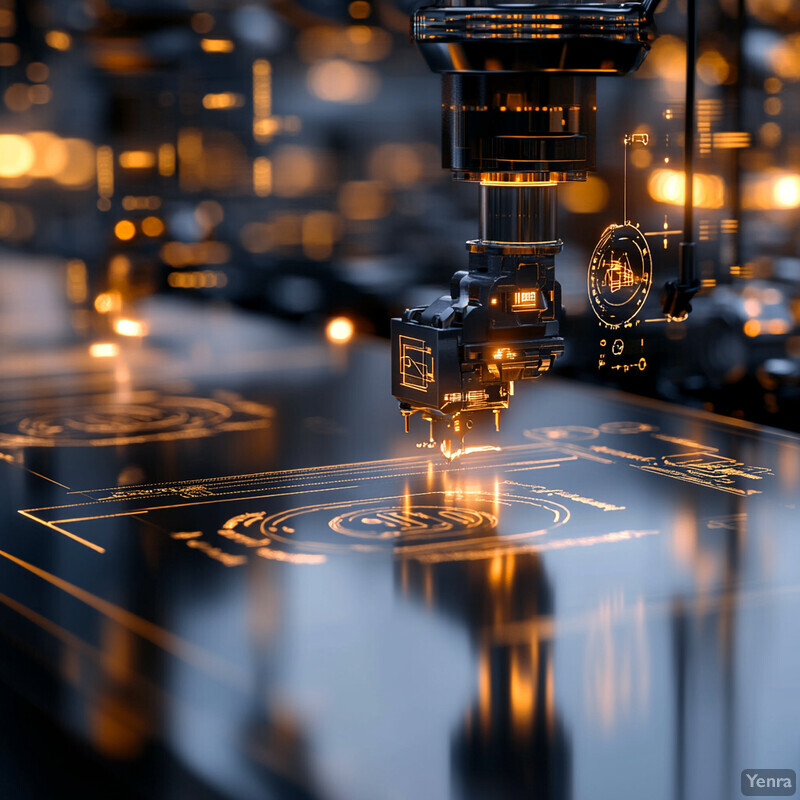
Many audit-related tasks are repetitive, such as verifying invoice details, updating spreadsheets, or checking vendor master files for consistency. By combining AI with RPA, these routine tasks can be fully automated. The AI component enhances RPA bots with cognitive abilities—such as understanding context and learning from past exceptions—so that even slightly varying tasks can be handled without human intervention. This automation relieves auditors of low-value clerical work, enabling them to devote their expertise to investigative analysis, strategic evaluations, and advisory activities that add greater value to the organization.
15. Enhanced Data Quality and Cleansing
AI-driven data quality tools can detect and correct inaccuracies, duplicate entries, and inconsistent formatting before the audit, ensuring higher-quality data and more reliable audit conclusions.
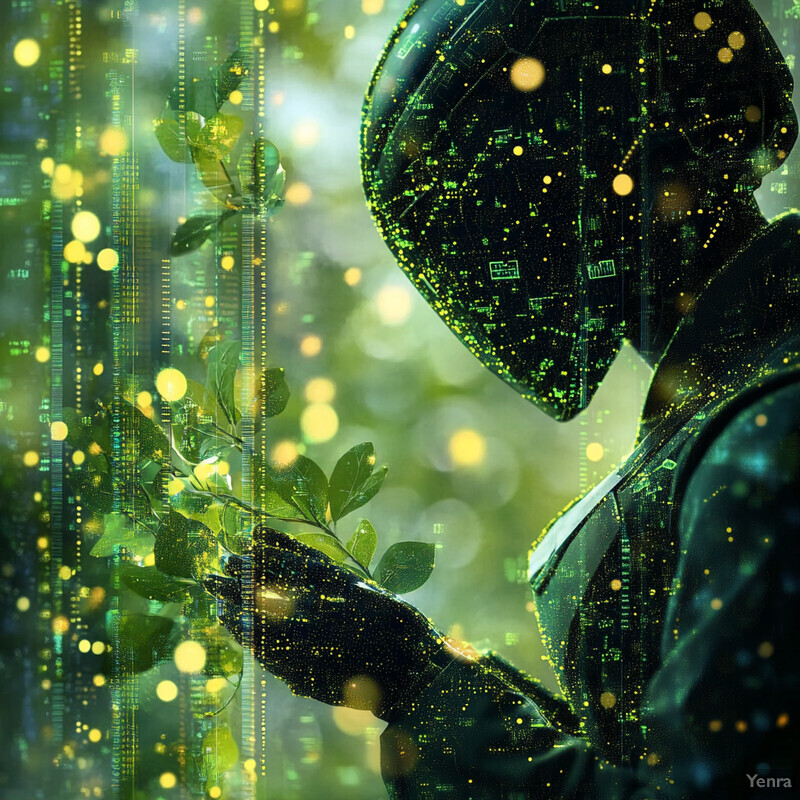
Poor data quality—duplicate entries, missing fields, and inconsistent coding—slows down audits and increases the risk of inaccuracies. AI-based data quality tools detect and correct these issues proactively. By applying statistical models and pattern recognition, these tools can identify duplicates, suggest consistent naming conventions, and infer missing values where appropriate. This preemptive data cleansing ensures that the audit team is working from a more accurate dataset, reducing the time spent on manual corrections and allowing for more reliable conclusions from subsequent analyses.
16. Intelligent Trend Analysis and Benchmarking
Machine learning models can compare a company’s financial data against industry benchmarks or historical performance, highlighting irregularities or areas that warrant closer examination.
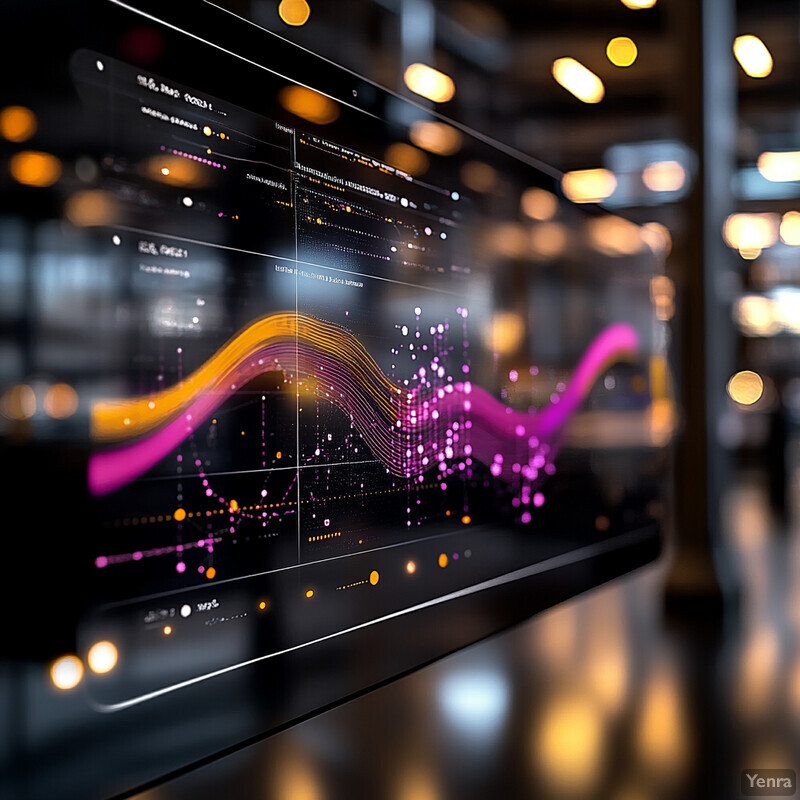
Benchmarking an organization’s financial metrics against historical performance or industry standards is crucial for identifying anomalies. AI excels at analyzing large historical datasets, spotting subtle trends, and understanding seasonality or cyclical patterns. It can also incorporate external data—such as industry averages or competitor performance metrics—to determine if the company’s financial indicators are in line with expectations. By quickly flagging unexpected deviations from norms, AI provides auditors with actionable leads on areas that warrant deeper investigation, helping maintain the integrity of financial reporting.
17. Scenario Modeling and Simulation
AI-powered simulation engines can test how different market conditions, policy changes, or operational decisions might impact a company’s financial statements, helping auditors assess risk more comprehensively.
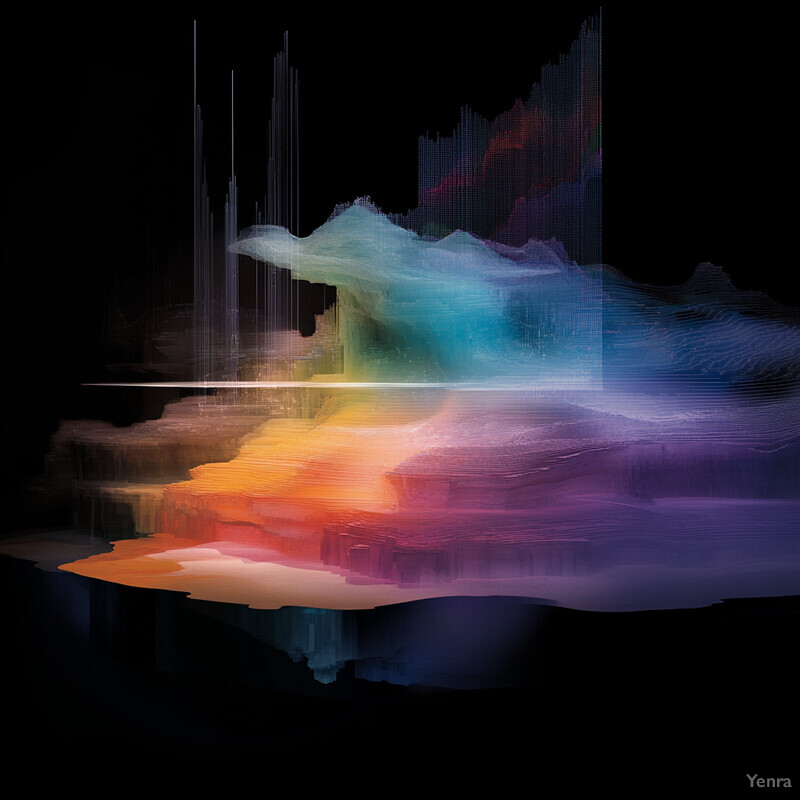
Financial auditors often consider various 'what-if' scenarios to understand potential impacts on the organization’s financial health. AI-powered modeling and simulation tools can rapidly test a range of conditions—such as interest rate changes, currency fluctuations, or regulatory shifts—and project their effects on cash flow, revenue, or asset valuations. These scenario analyses help auditors assess the robustness of the organization’s financial strategy and guide management toward preventative measures, ensuring that stakeholders are prepared for potential adverse conditions before they materialize.
18. Adaptive Machine Learning Models
AI models can learn from past audits, improving their accuracy and efficiency over time. As they process more cases, these systems become better at recognizing complex patterns and reducing false positives.
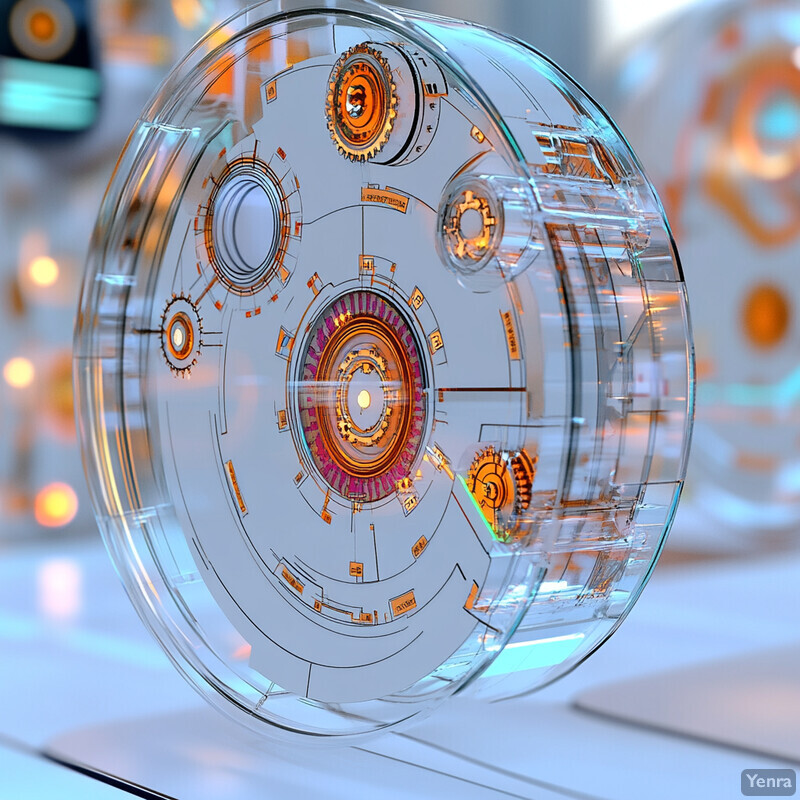
As auditors use AI tools more frequently, the algorithms behind these systems learn and improve. Each audit cycle provides fresh data and feedback, allowing machine learning models to refine their predictions, detection capabilities, and accuracy. Over time, these adaptive models become increasingly adept at recognizing evolving fraud tactics, identifying new types of compliance risks, and understanding the unique financial characteristics of the organization. This continuous improvement ensures that the AI solutions grow more effective and efficient with each use, providing a long-term strategic advantage for the audit function.
19. Explainable AI for Enhanced Transparency
Newer AI techniques aim to provide understandable rationales for automated judgments, helping auditors and regulators trust AI-driven insights by clearly showing the factors that led to an alert or finding.
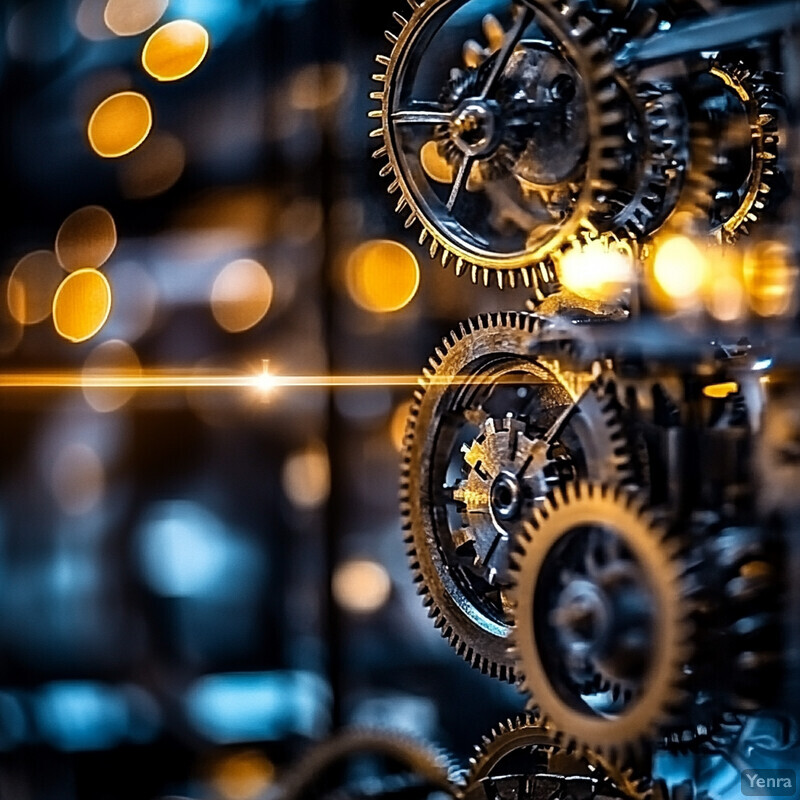
Trust in AI-driven insights is paramount. Explainable AI (XAI) methodologies help auditors understand how and why an AI model arrived at a particular conclusion or recommendation. Instead of treating the AI’s outputs as a 'black box,' auditors can view reasoning pathways, feature importance, and other explanations that elucidate the logic behind decisions. This transparency fosters trust, supports compliance with auditing standards that require justifications for findings, and reassures stakeholders that the AI tools are functioning fairly and responsibly. In turn, explainability strengthens the collaboration between auditors and intelligent systems.
20. Proactive Remediation Recommendations
Beyond detecting issues, advanced AI systems can offer recommendations for process improvements, changes in internal controls, or policy adjustments, helping organizations proactively strengthen their financial integrity and reduce future audit issues.
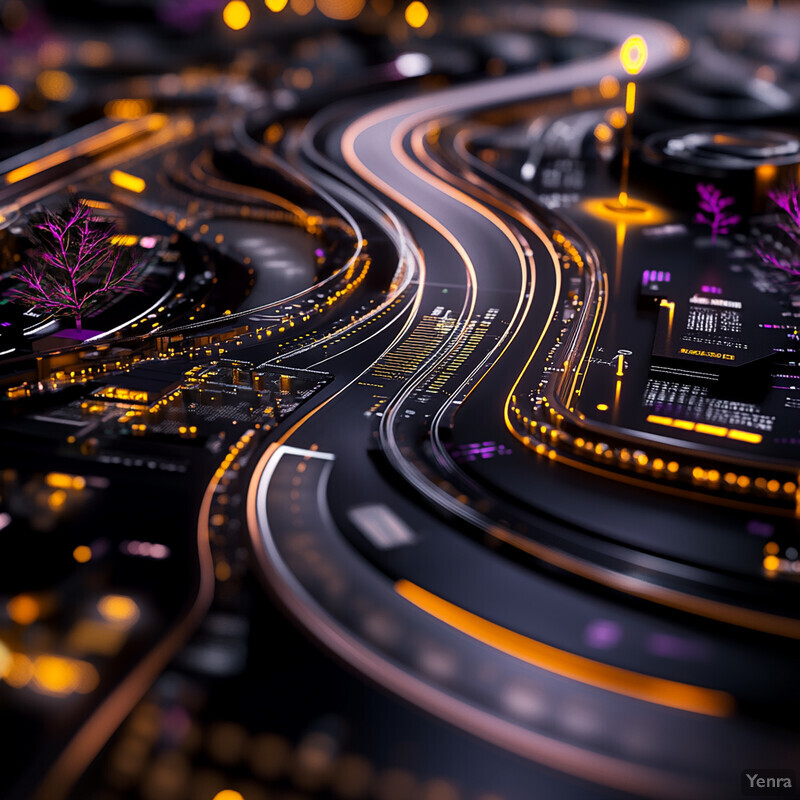
AI not only identifies problems but can also propose targeted solutions. By analyzing the root causes of anomalies—be they process inefficiencies, control weaknesses, or policy gaps—intelligent systems can suggest practical remediation steps. For example, they may recommend adding a new control approval step, adjusting vendor selection criteria, or updating internal policies. These proactive recommendations help organizations fortify their financial processes against future irregularities. Instead of merely reacting to issues after they arise, auditors and management can leverage AI insights to create a safer, more resilient financial environment that supports long-term organizational health.