1. Automated Movement Generation
AI-driven systems can generate novel sequences of dance movements that choreographers can use as a creative starting point.
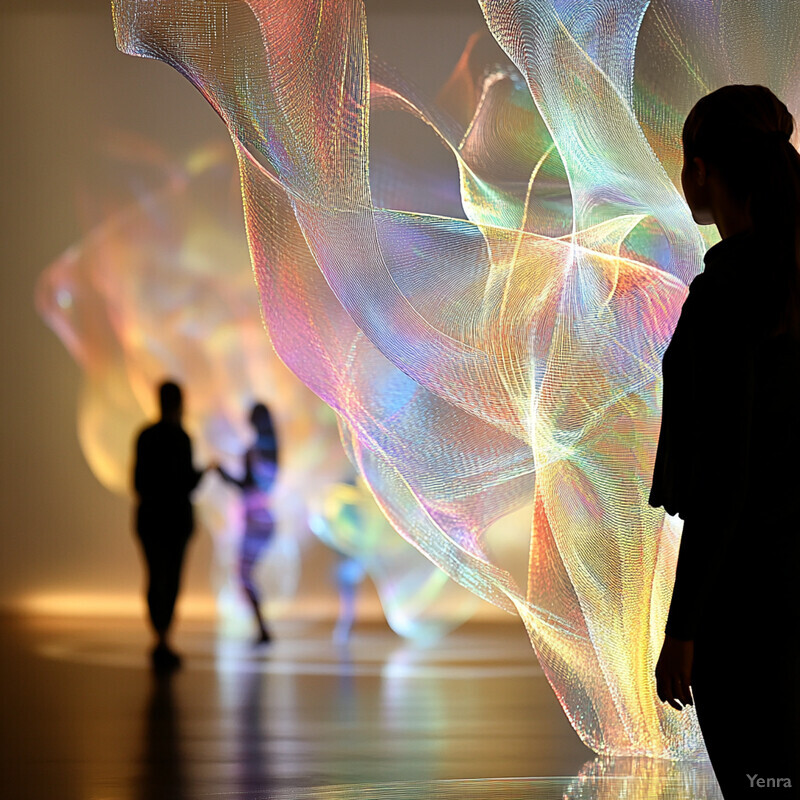
Through advanced generative models and reinforcement learning techniques, AI can produce original dance movements and sequences that serve as creative seeds for choreographers. Rather than starting from scratch, choreographers can input certain parameters—such as tempo, intended mood, spatial limitations, or the number of dancers—and receive suggested patterns of footwork, arm gestures, and transitions. These machine-generated sequences can range from straightforward steps that reflect established dance vocabularies to completely novel movements that spark new creative directions. Over time, as the AI system is trained on various styles and repertoires, its suggestions become increasingly refined, providing choreographers with an ever-expanding library of movement ideas.
2. Music-to-Movement Mapping
Advanced algorithms can analyze music for tempo, rhythm, and mood, then automatically propose dance steps or transitions aligned with the audio’s structure.
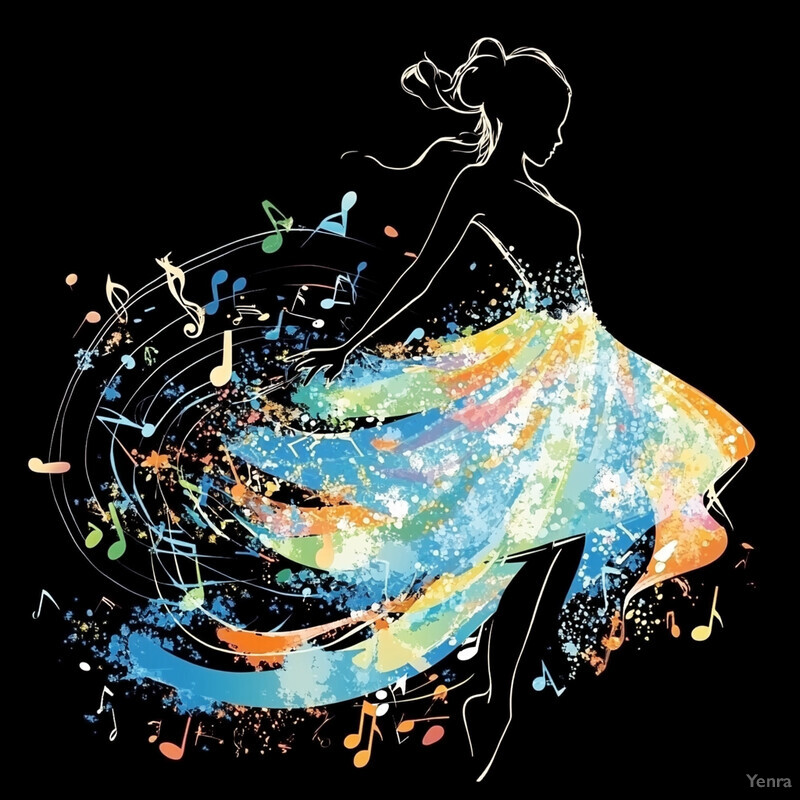
By analyzing a track’s rhythm, melody, tempo changes, and dynamic contrasts, AI can suggest corresponding dance movements that align closely with the music’s structure and feel. Choreographers can adjust parameters, such as intensity or complexity, and let the system automatically propose steps that match musical accents, phrasing, and thematic motifs. This alignment helps ensure that the resulting choreography maintains a strong, cohesive relationship between what is heard and what is seen. In addition, such mapping can be configured for different dance genres, so a classical ballet score and a hip-hop track each yield fitting and distinctive choreographic suggestions.
3. Style Adaptation
Machine learning models trained on various dance genres can suggest stylistic variations, allowing choreographers to seamlessly blend elements from multiple dance traditions.
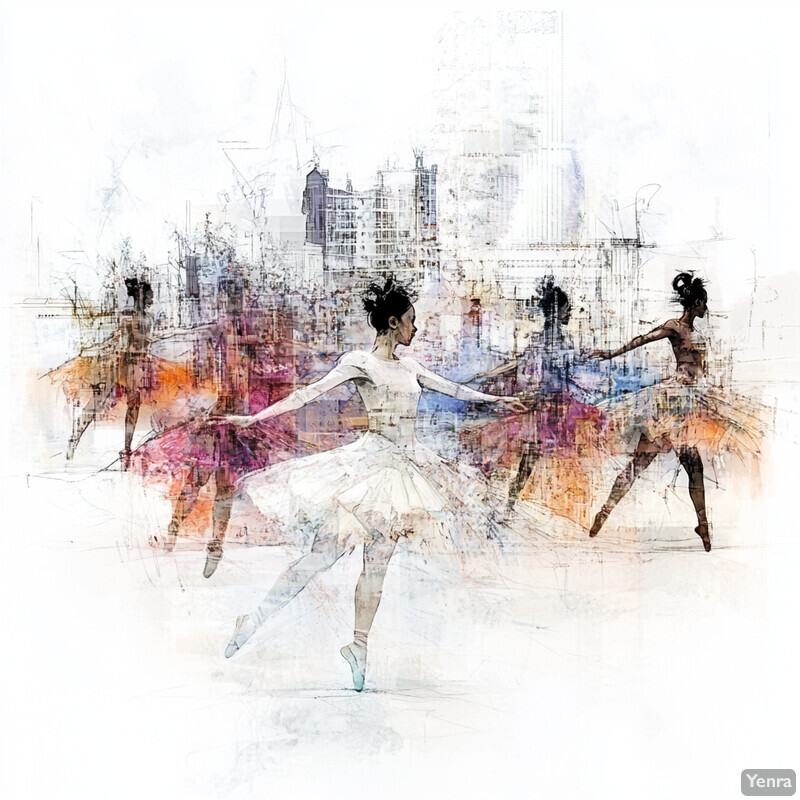
Machine learning models trained on massive repositories of dance videos and motion capture data can learn the signature elements of various dance styles—be it the fluid lines of contemporary ballet, the sharp isolations of hip-hop, or the graceful footwork of traditional folk dances. Once equipped with this knowledge, the AI can remix and integrate stylistic elements, allowing choreographers to fuse distinct genres and create hybrid forms. This style blending can inspire innovative choreography, broaden a dancer’s repertoire, and open up cultural dialogues between different dance traditions, all while giving the choreographer flexible control over how strongly one style influences another.
4. Personalized Difficulty Adjustment
AI can tailor choreography based on dancers’ skill levels, physical attributes, or training objectives, ensuring that sequences remain accessible yet challenging.
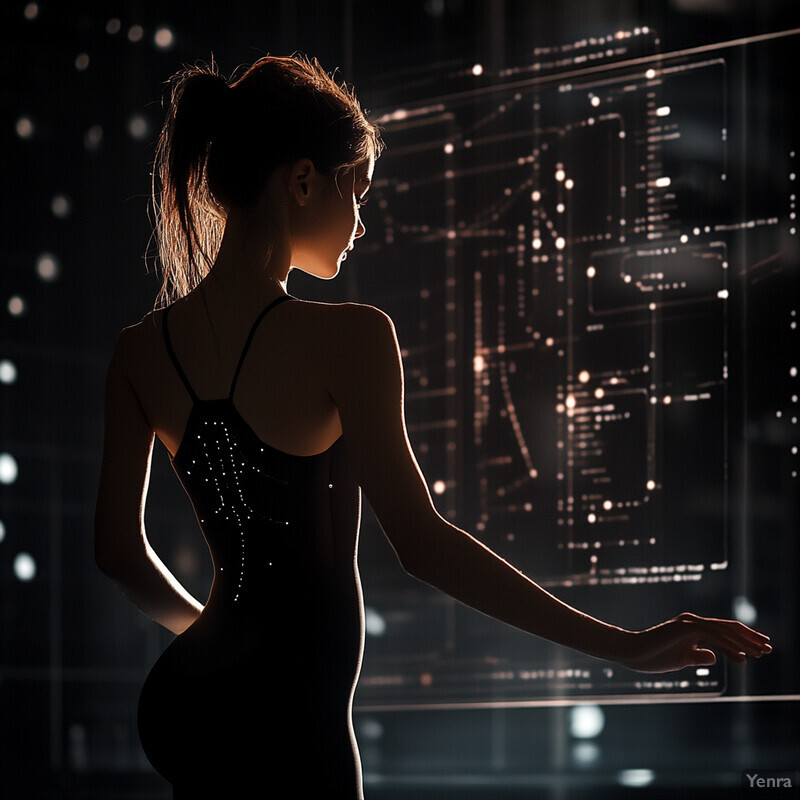
AI-driven systems can gauge dancers’ skill levels and physical attributes by analyzing their technique, strength, balance, and range of motion through sensors or video feedback. Using these insights, the system can adjust the complexity of proposed choreography so that it’s not too simple or too challenging. For instance, if a dancer struggles with certain lifts or turns, the AI can suggest safer and more achievable alternatives, enabling incremental skill-building over time. This tailored approach ensures that the learning curve is steady and encourages continuous improvement while minimizing frustration or injury risks.
5. Real-Time Feedback Through Computer Vision
By using pose estimation and motion tracking, AI tools can provide instant suggestions on posture, technique, and timing, helping dancers refine their craft as they rehearse.
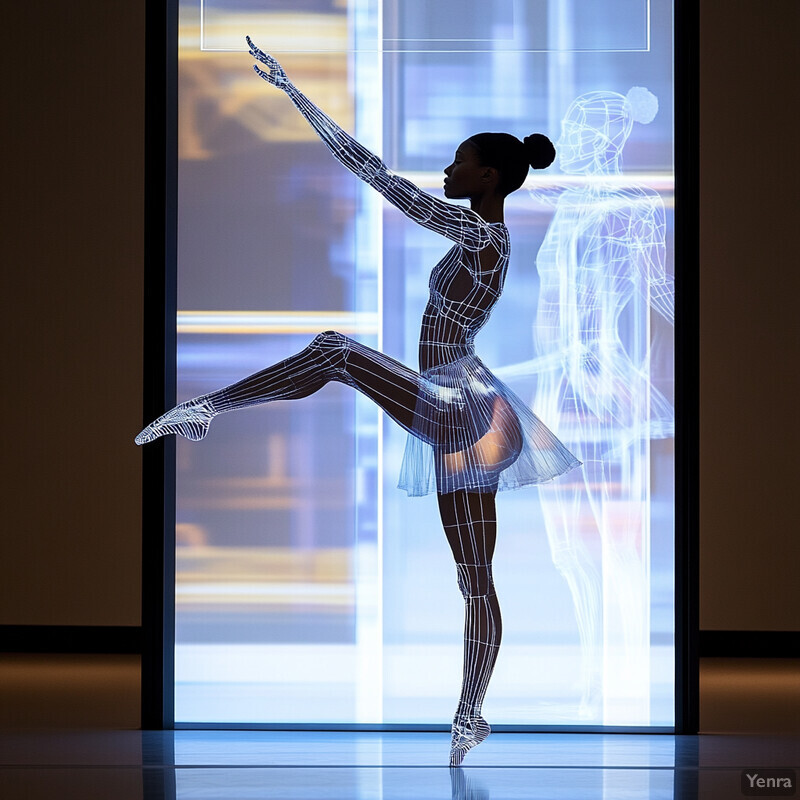
By employing pose estimation, motion tracking, and skeletal analysis algorithms, AI tools can provide immediate, data-driven feedback on dancers’ form and technique. As dancers rehearse, the system flags subtle misalignments, timing discrepancies, or off-balance moments. This feedback can be visually or verbally communicated in real-time, enabling dancers to make swift corrections. The result is more efficient rehearsals, as immediate insights replace after-the-fact notes, guiding dancers toward precise execution of each movement and facilitating rapid skill refinement.
6. Motion Pattern Analysis
Data-driven models can identify patterns in professional choreographies, uncovering common motifs, transitions, and structures that choreographers might use as inspiration.
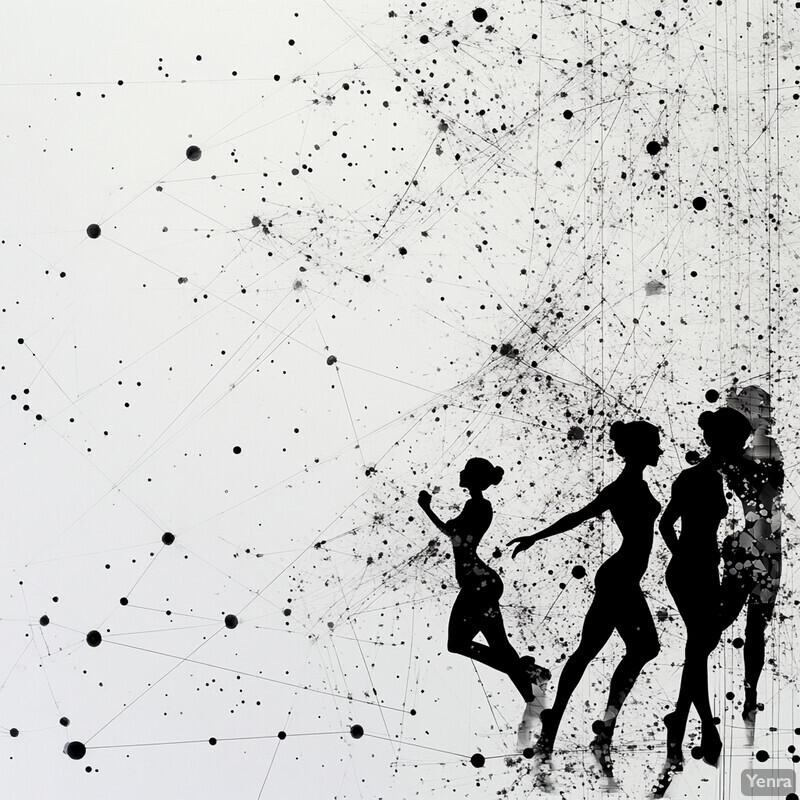
As AI sifts through extensive archives of professional dance recordings, it identifies recurring patterns, motifs, transitions, and compositional structures. These analytic capabilities help choreographers understand common building blocks of certain dance genres and spark ideas for new combinations. By comparing these patterns with the choreographer’s initial concepts, the AI can highlight potential pathways to develop a theme, intensify dramatic tension, or provide counterpoints within a routine. This process not only accelerates the creative process but also pushes choreographers toward more nuanced and informed artistic decisions.
7. Predictive Audience Engagement Analytics
AI can model how certain movements or sequences might resonate with an audience, guiding choreographers toward more compelling, emotionally resonant dance pieces.
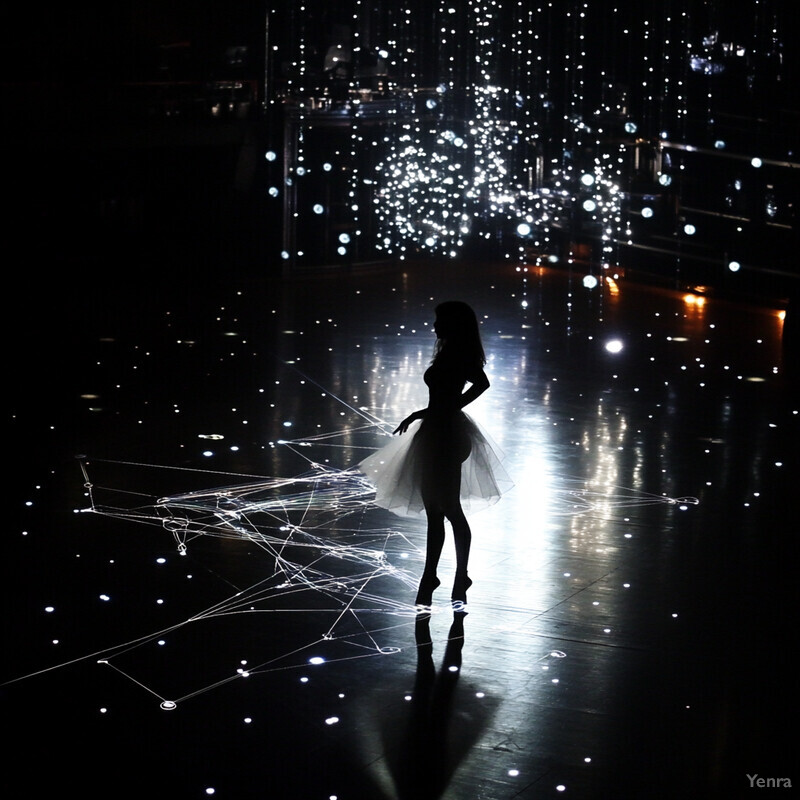
Using emotional recognition software, aesthetic metrics, and historical audience response data, AI can predict how viewers might react to certain movements, shapes, or narrative elements in a choreography. Before a piece is publicly performed, the system can estimate which sections might captivate spectators or which transitions might feel overly long. Choreographers can then refine their work to enhance audience engagement, ensure better pacing, and maintain the emotional arc. This data-driven approach transforms an otherwise subjective guessing game into a more informed and strategic creative process.
8. Rapid Prototyping in Virtual Spaces
Virtual and augmented reality platforms enabled by AI let choreographers experiment with spatial arrangements and formations without needing a physical studio or dancers on hand.
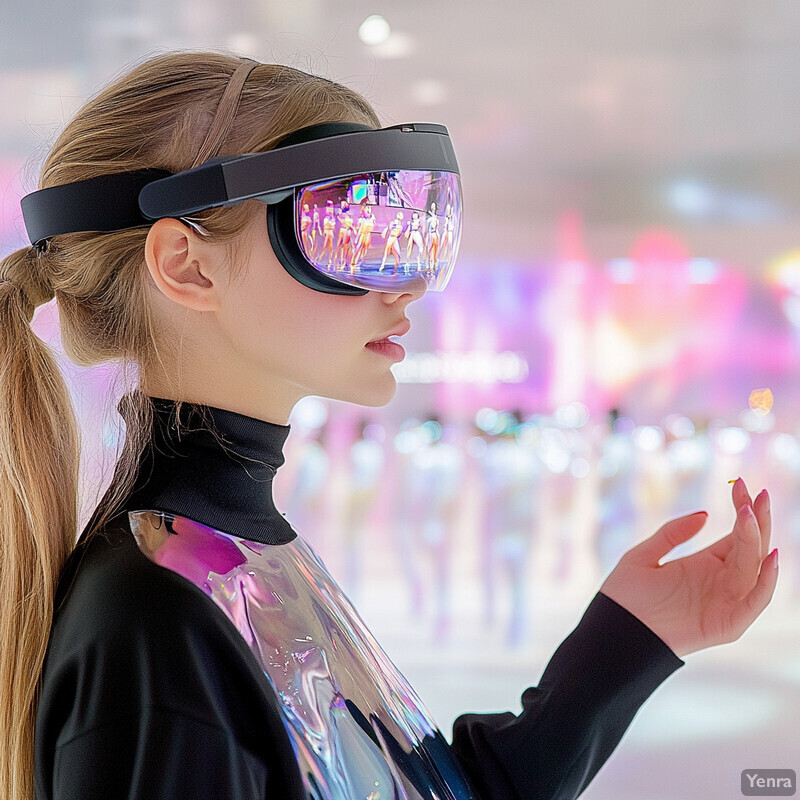
Virtual reality (VR) and augmented reality (AR) platforms, powered by AI, enable choreographers to experiment with spatial arrangements, dancer formations, and stage designs long before live rehearsals. By adjusting parameters in a virtual environment, creators can instantly preview how changes in spacing, lighting, or the number of performers impact the overall composition. These simulations allow testing different ideas without logistical constraints, reducing costs, and increasing efficiency. It’s like having an infinitely flexible rehearsal studio available at any time, empowering choreographers to find optimal staging solutions quickly.
9. Dynamic Sequencing Tools
AI can dynamically rearrange sequences in real-time as choreographers input constraints (like dancers’ formations or stage size), accelerating the iterative creative process.
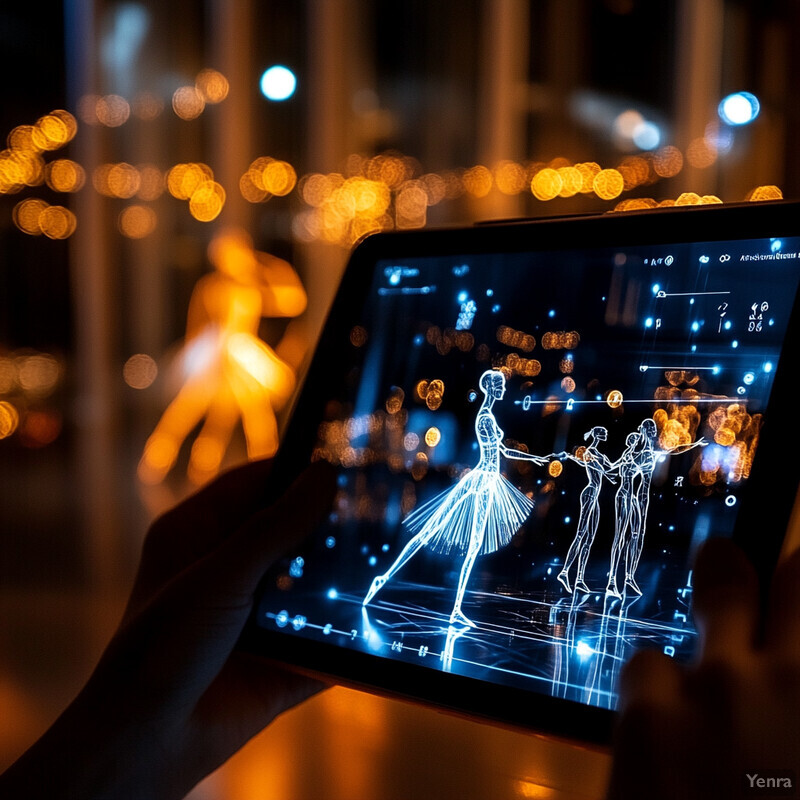
AI-driven tools can dynamically rearrange and recombine choreographic sequences based on constraints provided by the choreographer. If a director decides mid-process that certain dancers must enter from a different side of the stage or that a particular sequence must be shortened to fit time limits, the AI can automatically adjust transitions and formations. This responsiveness not only speeds up the iterative process of refining choreography but also helps maintain a coherent flow. Over time, these tools become “co-choreographers,” understanding the creator’s preferences and continuously adapting sequences on the fly.
10. Automated Dance Notation and Documentation
Machine learning can interpret and encode dance moves into standardized notation systems (like Labanotation) or metadata, making it easier to record and share choreographic knowledge.
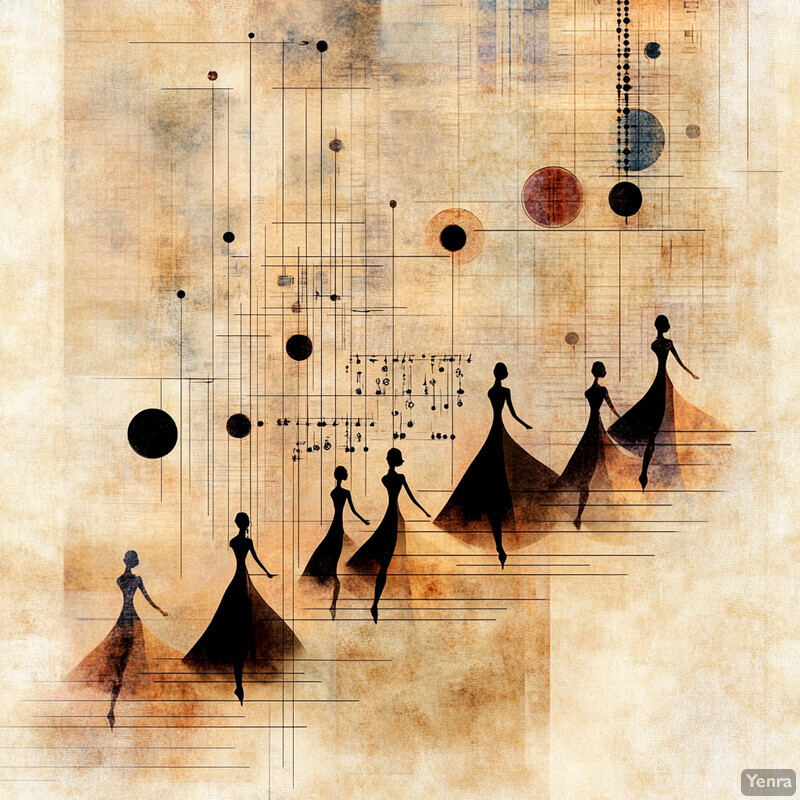
Translating choreographic ideas into a standardized form like Labanotation is traditionally time-consuming and specialized. AI can analyze video footage of dances and translate complex movements into structured data. This allows choreographers to document their work more efficiently, preserve it for posterity, and share it globally with dancers who may never have seen the original performance. In turn, other AI tools can interpret these notations and regenerate the choreography in different contexts, ensuring that valuable artistic knowledge does not fade with time.
11. Enhanced Rehearsal Management
Intelligent systems can schedule practice sessions, allocate specific parts of a routine to dancers needing more practice, and provide detailed rehearsal analytics.
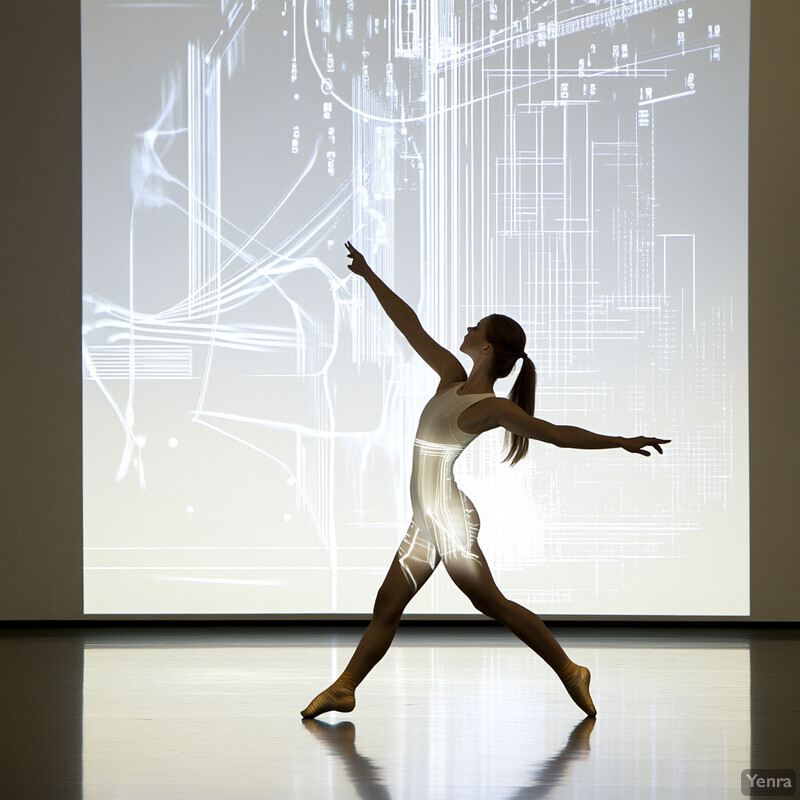
Intelligent scheduling systems can analyze dancers’ availability, rehearsal space constraints, and the complexity of different segments to propose optimized practice schedules. By monitoring performance metrics over time, AI can identify which dancers need additional practice on certain phrases, or when to introduce rest periods to avoid fatigue and injury. Automated reminders and rehearsal notes ensure that every session is used optimally, helping directors and stage managers keep the production process on track and maintain a harmonious working environment.
12. Physiological and Biomechanical Insights
Integrating wearable sensors with AI models can detect fatigue, range-of-motion issues, and injury risks, advising choreographers on safer and more efficient movements.
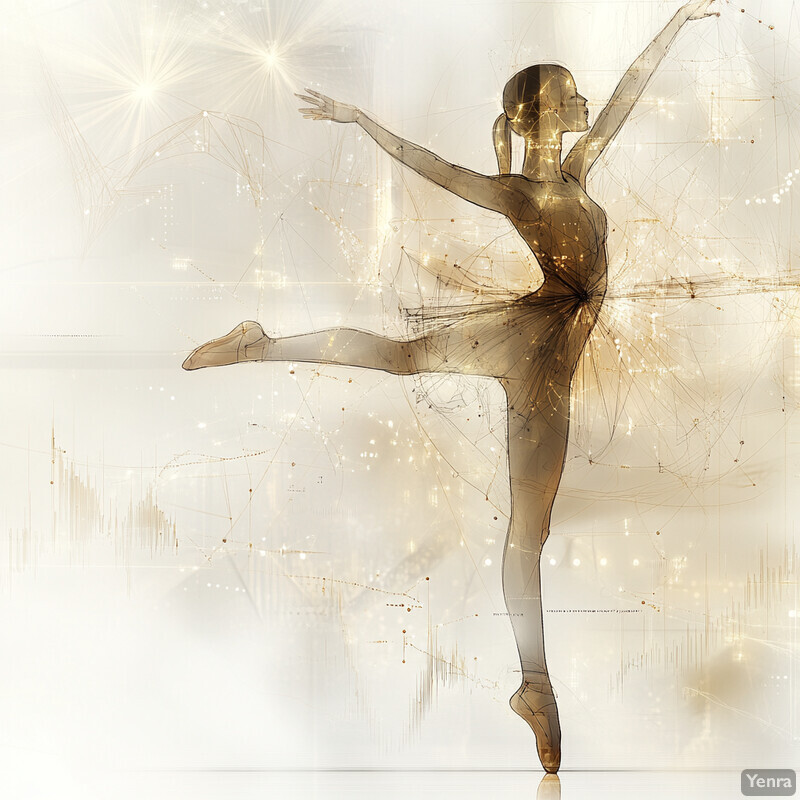
Integrating wearable sensors and motion-capture technology with AI analysis provides real-time assessments of dancers’ physical states. The AI can detect fatigue levels, asymmetries in movement, and unusual stress on joints or muscles. By alerting choreographers and dance coaches to these issues, early interventions can be made to prevent injuries and optimize training regimens. This data-driven approach to dancer health and biomechanics ensures a safer, more sustainable practice that supports both short-term performance quality and long-term career longevity.
13. Collaborative Co-Creation
Choreographers can interact with generative AI models as creative partners, exchanging ideas, testing variations, and refining sequences through iterative human–machine dialogue.
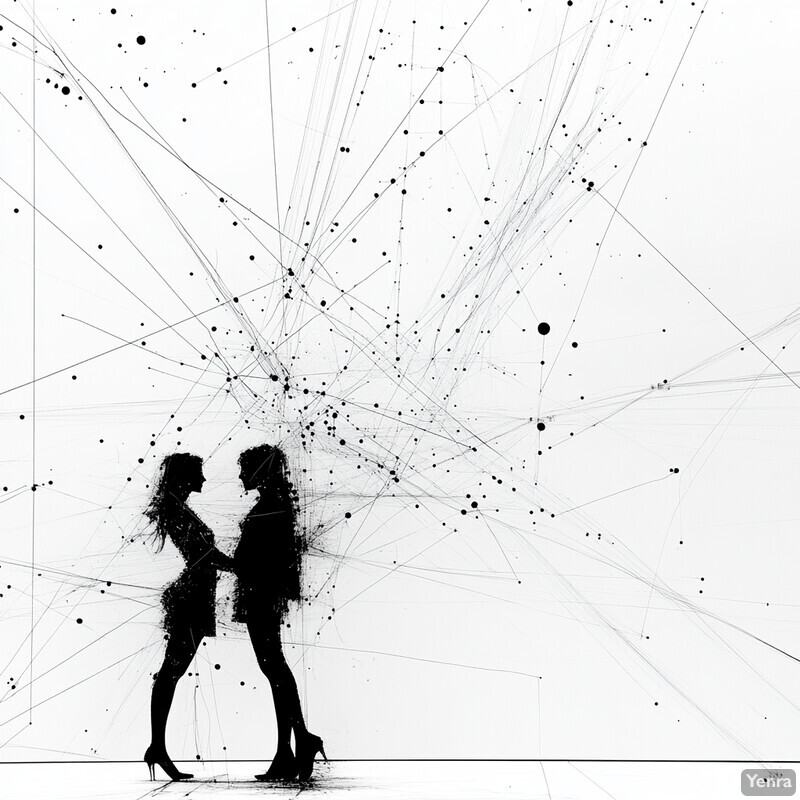
Choreographers can treat AI as a creative partner, engaging in a digital dialogue of suggestions, refinements, and evaluations. They might provide a theme, a narrative arc, or a particular stylistic constraint, and the AI responds with movement ideas. The human creator then selects, modifies, or rejects these suggestions, prompting the AI to learn from these choices. Over time, the system adapts, better anticipating the choreographer’s aesthetic preferences, resulting in a truly collaborative creative process that blends human artistry with computational innovation.
14. Automated Improvisation Prompts
AI can provide real-time improvisational prompts for dancers, encouraging experimentation and developing a choreographer’s creative vocabulary.
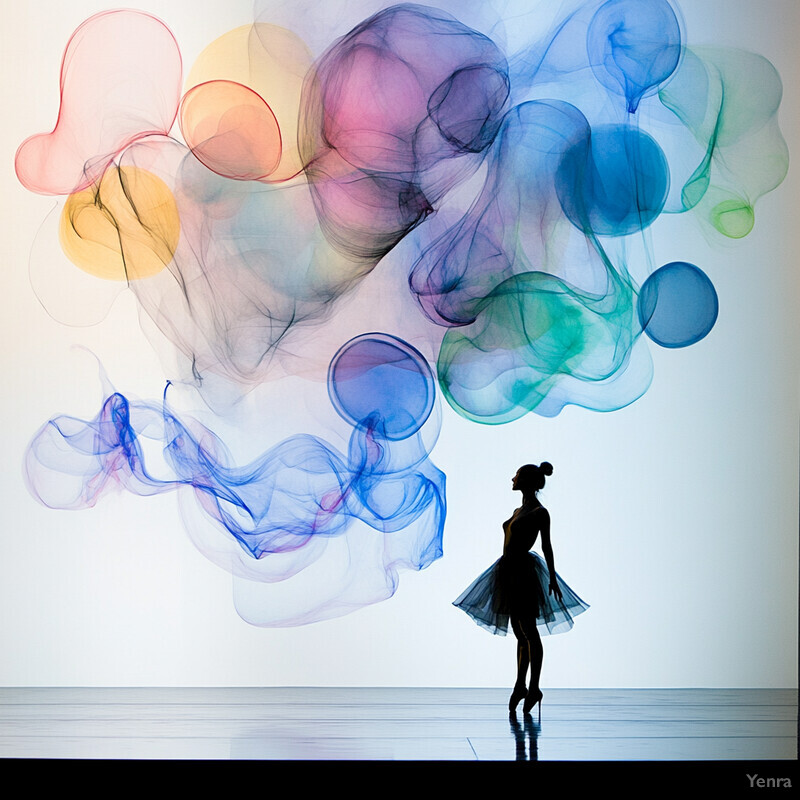
Improvisation exercises are key to generating fresh movement ideas. AI can feed dancers prompt after prompt—“explore a movement that contracts inward,” “transition from high leaps to floor work,” or “express a feeling of weightlessness”—based on the choreographer’s goals. This helps dancers break out of habitual patterns and embrace a wider movement vocabulary. Such prompts can be tuned to emphasize certain qualities, techniques, or emotional tones, turning the AI into a wellspring of inspiration for developing unique choreographic fragments.
15. Transformation from 2D to 3D Movements
AI can take a sketch or a simple series of steps and extrapolate it into a fully realized three-dimensional choreography, preserving stylistic nuances.
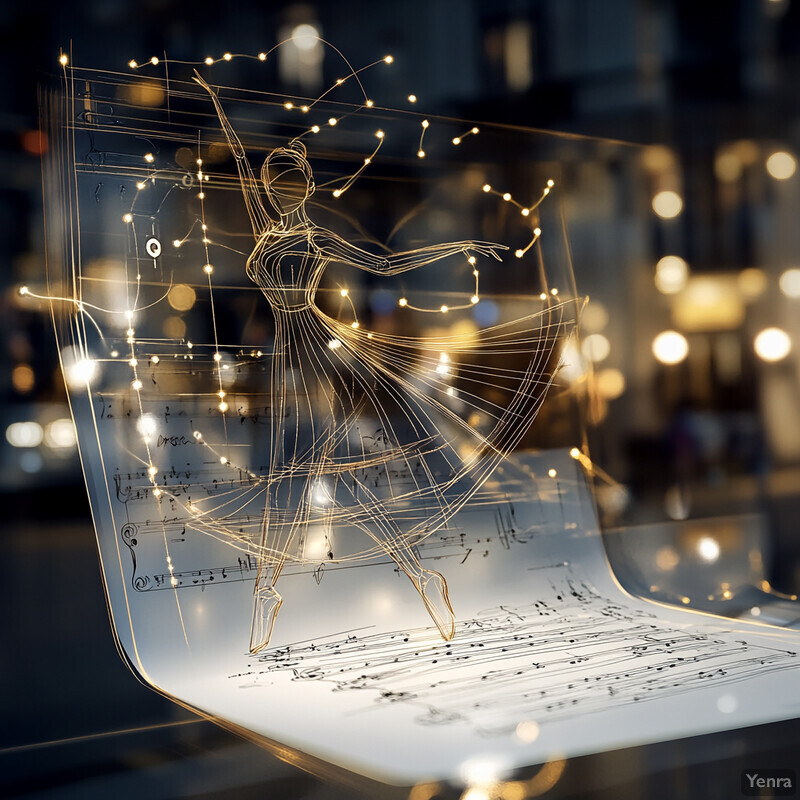
Choreographers may sketch initial ideas in 2D drawings or simplistic movement sequences. AI can interpret these rudimentary inputs and extrapolate full-body, three-dimensional dances with realistic timing, dynamics, and style. This transformation allows creators to visualize how a flat conceptual idea would appear in a fully realized performance space. By bridging the gap between abstract concepts and tangible choreographic sequences, AI helps choreographers refine their visions early in the creative process, ultimately saving time and energy.
16. Historical Choreography Retrieval
Deep learning-based indexing can sift through large archives of historical dance footage, helping choreographers discover forgotten techniques and integrate them into new routines.
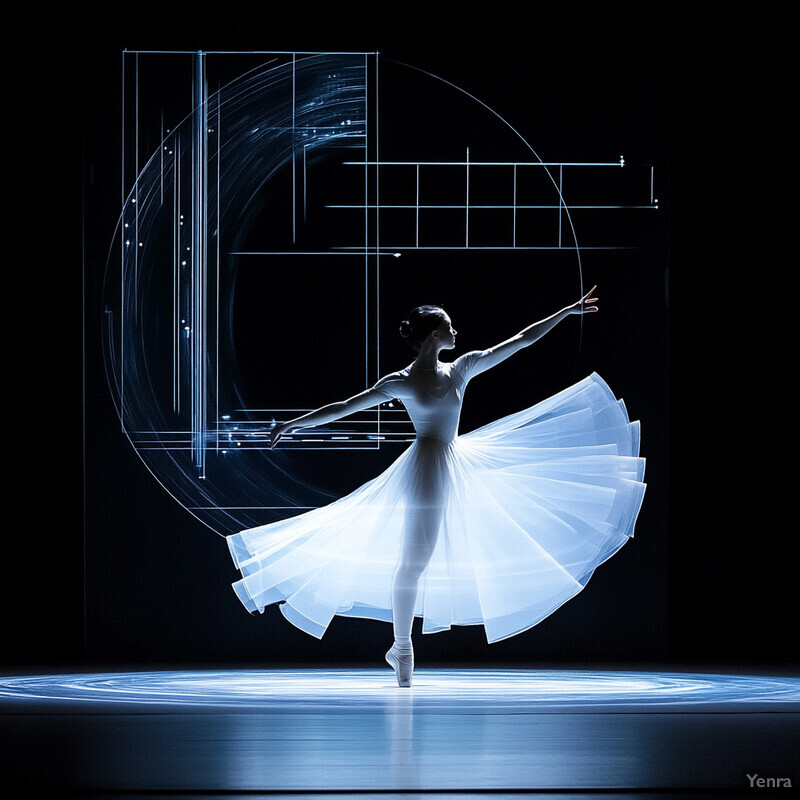
Over centuries, countless dances have been performed and recorded, but much of this rich history can remain untapped. AI systems equipped with deep learning-based indexing can comb through large archives of dance videos, analyzing movement styles, cultural contexts, and compositional structures. By retrieving similar motifs or techniques from historical performances, choreographers gain access to a treasure trove of inspiration that might otherwise be buried. This ensures that the evolving art form continually learns from its past, fostering innovation anchored by tradition.
17. Kinetic Visual Effects Integration
AI can synchronize choreographed movements with stage lighting and projections, enhancing performances with perfectly timed visual and spatial effects.
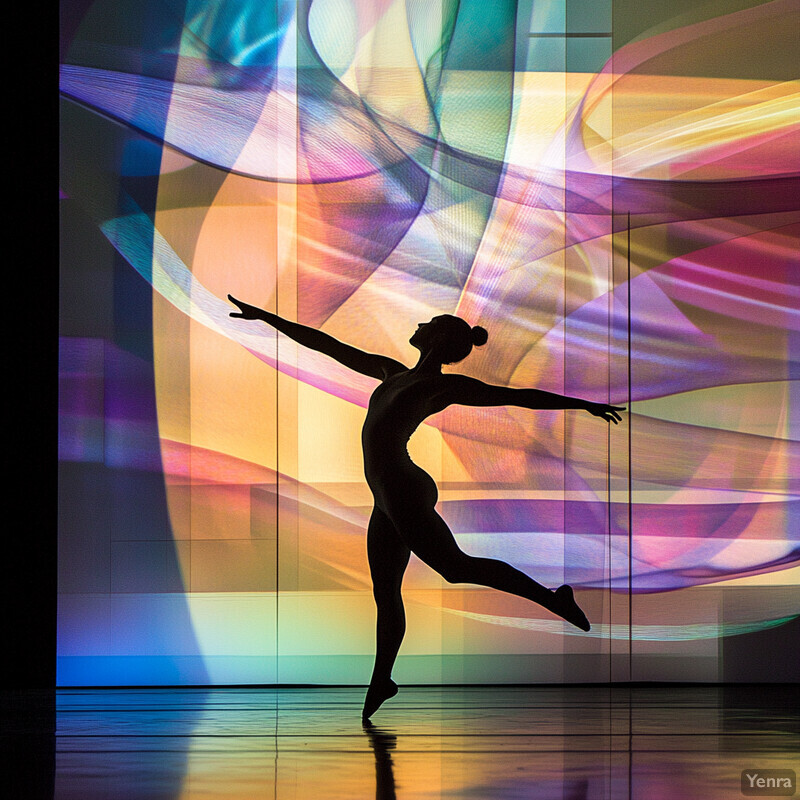
Integrating lighting cues, projections, and stage effects seamlessly with choreography can be challenging. AI can synchronize movement with visual and auditory effects, analyzing timing, spacing, and dancer pathways to ensure that special effects enhance rather than distract. For instance, lights might brighten at the apex of a leap or projections change in sync with a wave of arms. This synergy transforms the stage into a unified multimedia canvas, helping choreographers achieve a cohesive sensory experience that elevates their artistic storytelling.
18. Interactive Tutorials and Training Modules
AI-driven virtual instructors can break down complex sequences into manageable chunks, providing multi-angle demonstrations and progressive skill-building exercises.
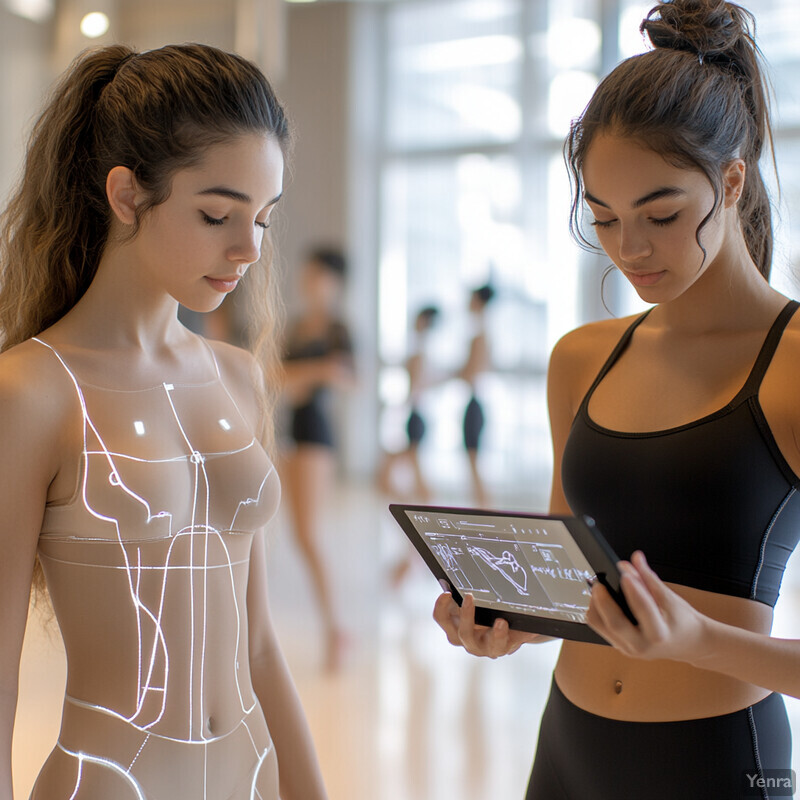
AI-driven instructional tools can break down complex dance sequences into manageable segments, providing learners with step-by-step guidance. Virtual instructors, powered by motion capture and speech synthesis, can offer corrective feedback and re-demonstrate tricky moves from multiple angles. Users can slow down or speed up the movement, request different camera perspectives, and even receive personalized practice exercises. As a result, dance education becomes more accessible and flexible, enabling students to learn and perfect sequences at their own pace.
19. Cross-Cultural Motion Synthesis
Models can blend patterns from different cultural dance forms, enabling choreographers to explore unique, globally inspired choreography.
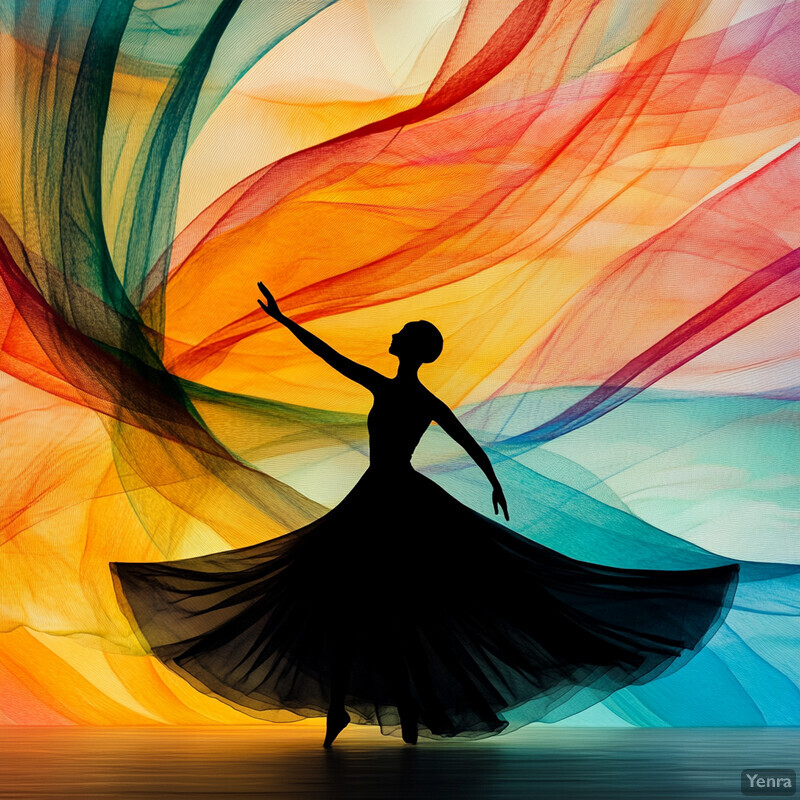
By absorbing the nuances of dances from around the world, AI can offer choreographers the opportunity to combine elements from diverse cultural styles in authentic and respectful ways. Through careful modeling, AI can identify shared motifs and contrasts, guiding the choreographer in melding different traditions into a novel form that still respects the integrity of each source culture. This cross-pollination can lead to new dance genres, forge multicultural collaborations, and broaden the artistic horizons of both dancers and audiences.
20. Continuous Improvement Loops
As dancers perform AI-suggested sequences, feedback on their execution feeds back into the system, refining future suggestions and continually elevating the quality of the choreographic output.
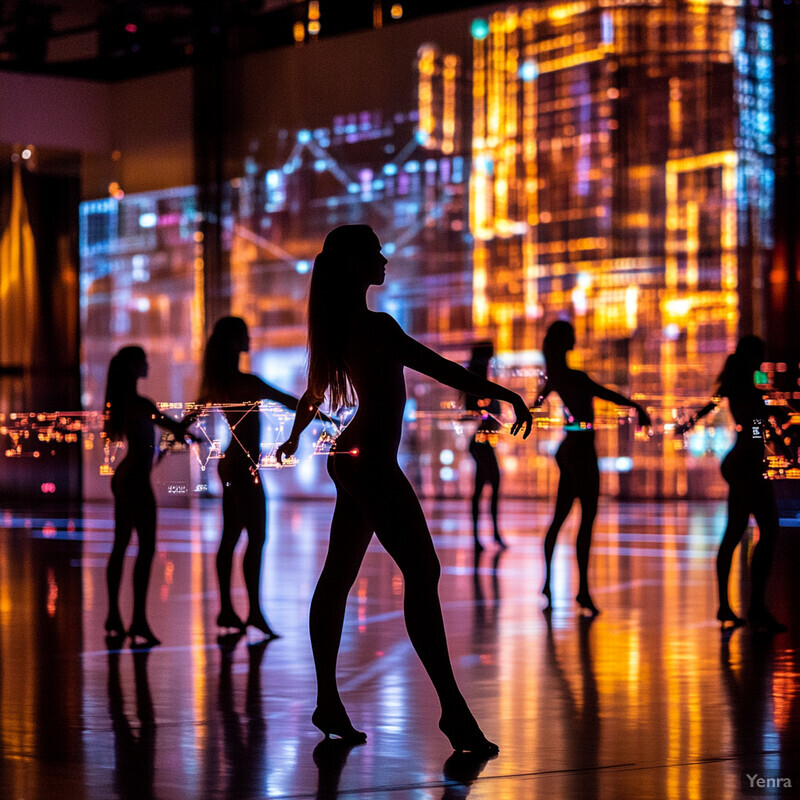
As dancers rehearse and perform AI-suggested sequences, their feedback and recorded performances feed back into the system, improving its future recommendations. Over time, the AI learns which suggestions are most effective, which transitions feel natural, and which movements suit certain body types or musical genres. This iterative process enables the system to grow more intelligent and nuanced, essentially “learning” the choreographer’s and dancers’ evolving artistic language. Such continuous improvement ensures that the AI remains a relevant and increasingly valuable tool, fostering a sustainable cycle of co-creation and artistic refinement.