1. Early Prediction of Disease Onset
AI-driven models can analyze genetic data, demographic factors, and clinical biomarkers to predict the risk and timing of arthritis onset before symptoms appear.
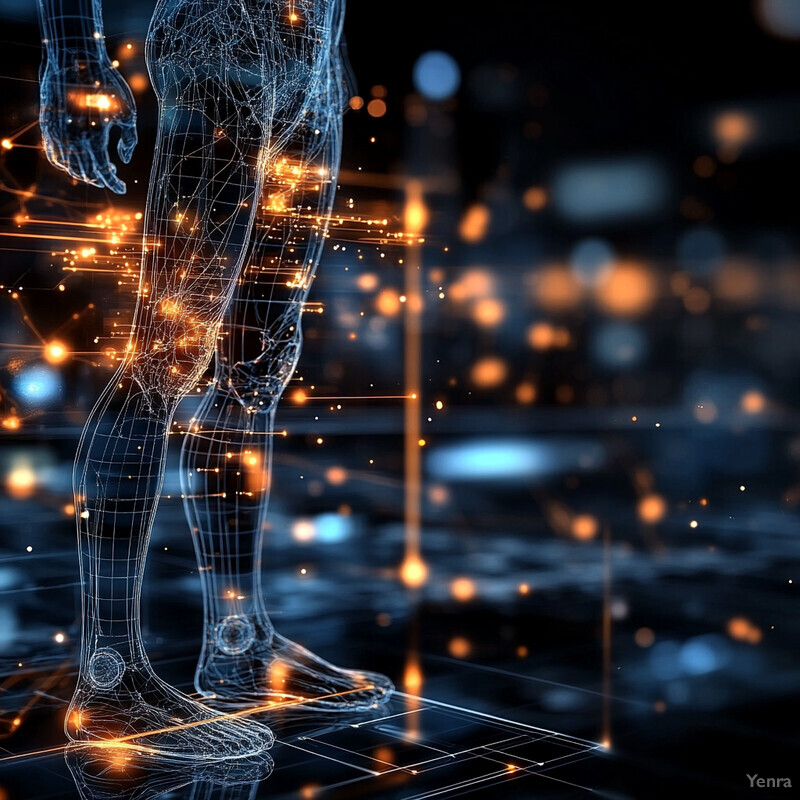
AI-driven models leverage patient-specific data, including genetic predispositions, demographic factors, and subtle biomarkers found in blood tests, to estimate the likelihood and timing of arthritis onset before overt clinical symptoms emerge. These systems can employ complex machine learning algorithms such as gradient boosting or neural networks to detect minuscule patterns that a human might overlook. By scrutinizing family histories, lifestyle attributes, and even environmental exposures, these models help clinicians identify at-risk individuals well in advance. As a result, patients and doctors can initiate preventive measures, lifestyle changes, or early pharmacological interventions that may slow down or even prevent the disease from fully manifesting.
2. Sophisticated Imaging Analysis
Machine learning algorithms can interpret medical images (X-ray, MRI, ultrasound) with greater accuracy, detecting subtle joint and cartilage changes indicative of early disease progression.
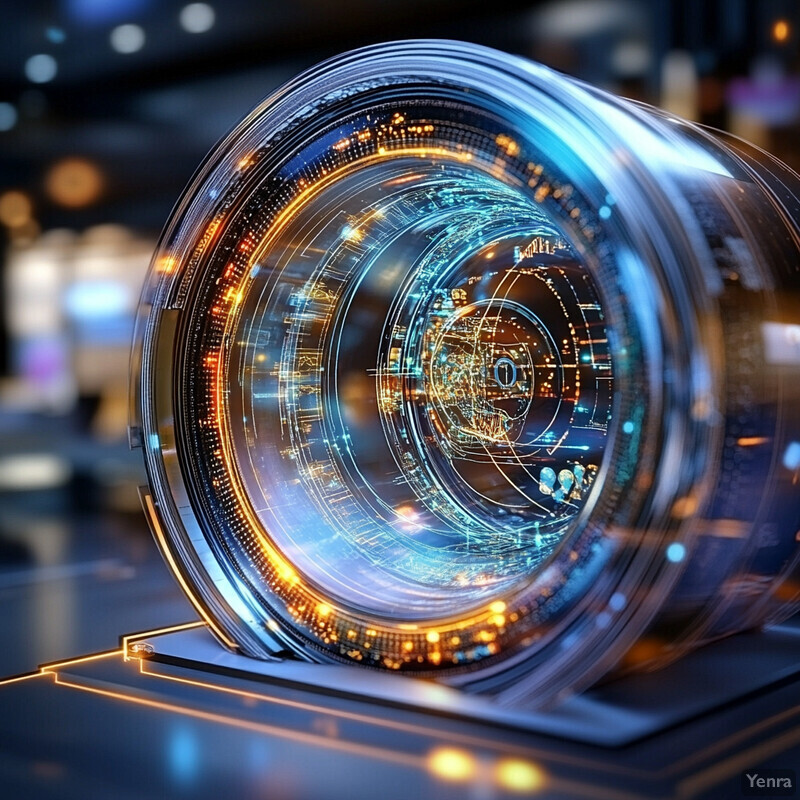
Cutting-edge AI solutions can process various medical imaging modalities—X-ray, MRI, and ultrasound—at a detail level beyond the human eye’s capability. Convolutional neural networks, for instance, can identify subtle changes in joint structures, cartilage wear, or synovial inflammation from imaging data with exceptional accuracy. This deeper insight can highlight early-stage disease markers, measure disease severity more objectively, and guide more targeted therapy. Moreover, these image-based analyses can be integrated with other patient data sources to produce a comprehensive clinical picture, streamlining the diagnostic process and giving patients a better prognosis.
3. Multi-Modal Data Integration
AI can fuse diverse data streams—imaging, lab tests, patient-reported outcomes, and wearable sensor metrics—to create more holistic progression models.
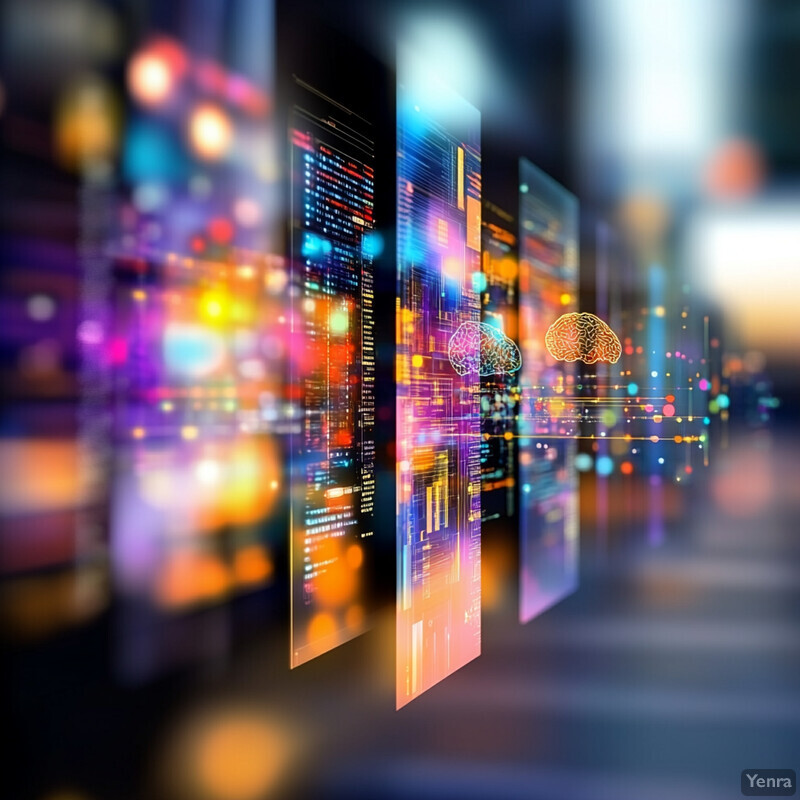
Arthritis is a multifactorial disease influenced by genetics, environment, lifestyle, and ongoing physiological changes. AI excels at merging diverse data streams—imaging results, laboratory tests, genomic data, patient-reported outcomes, wearable sensor readings, and clinical notes—into a cohesive analysis. Advanced algorithms can weigh the relative importance of each data type, identify patterns across different domains, and construct a unified model of disease progression. This holistic approach leads to more accurate predictions, reduces uncertainty in treatment decisions, and provides insights that might be missed by focusing on any single data source alone.
4. Identification of Novel Biomarkers
Through deep learning and feature extraction, AI can uncover new molecular or cellular biomarkers linked to disease severity and progression not easily identified by human experts.
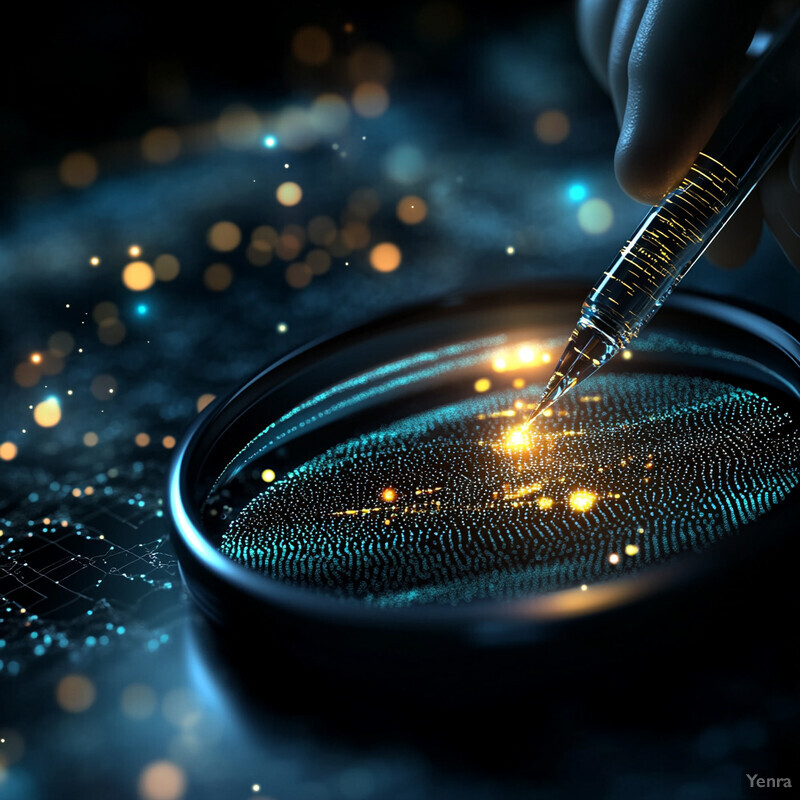
Traditional clinical biomarkers of arthritis progression often center around known inflammatory markers or imaging findings. However, AI’s pattern recognition capabilities enable the discovery of entirely new biomarkers—molecular, cellular, or metabolic signatures that correlate strongly with progression or severity. Techniques like deep learning and dimensionality reduction can mine large omics datasets, uncovering unusual patterns in gene expression or protein levels. By spotlighting these novel indicators, AI provides researchers and clinicians with fresh targets for diagnostics and therapies, potentially catalyzing the development of more effective intervention strategies and personalized treatment protocols.
5. Precision Prognostication
Advanced predictive models can forecast patient-specific trajectories, distinguishing which patients are likely to experience rapid joint deterioration versus slower, more manageable disease progression.
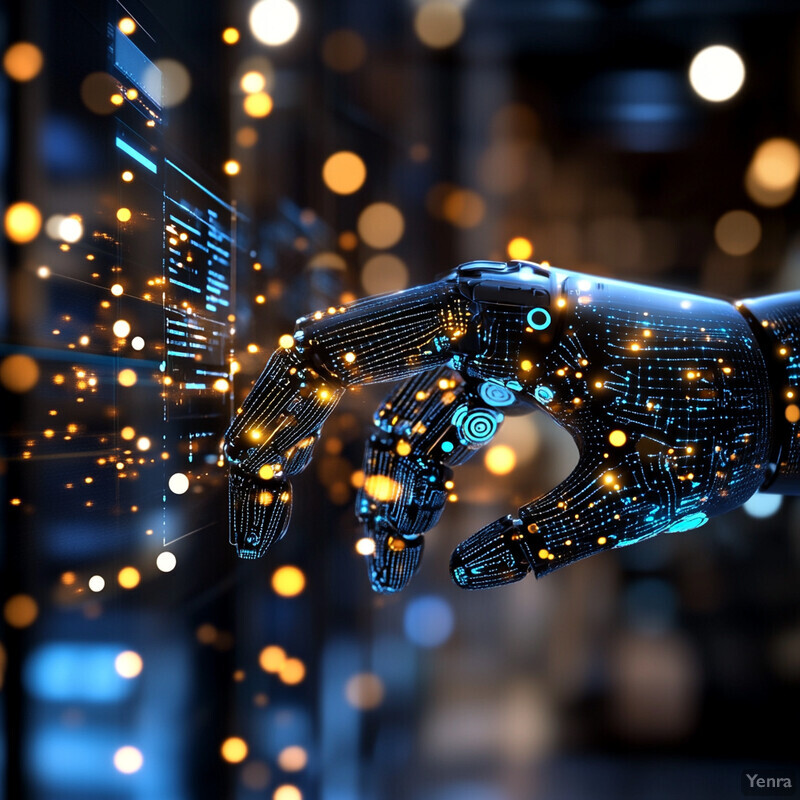
The course of arthritis varies greatly between patients, making it challenging for clinicians to provide personalized forecasts. AI-based predictive models apply machine learning techniques to patient histories, lab results, imaging, and other factors to construct detailed, individualized disease trajectories. These models can distinguish patients who may experience rapid joint deterioration from those who might have a more indolent disease course. With such forecasts, clinicians can refine treatment plans, initiate aggressive therapies earlier for those at high risk, and spare less vulnerable patients from unnecessary interventions.
6. Automated Joint Damage Scoring
Computer vision techniques can score joint damage automatically from imaging scans, improving consistency and reducing the time clinicians spend on manual assessments.
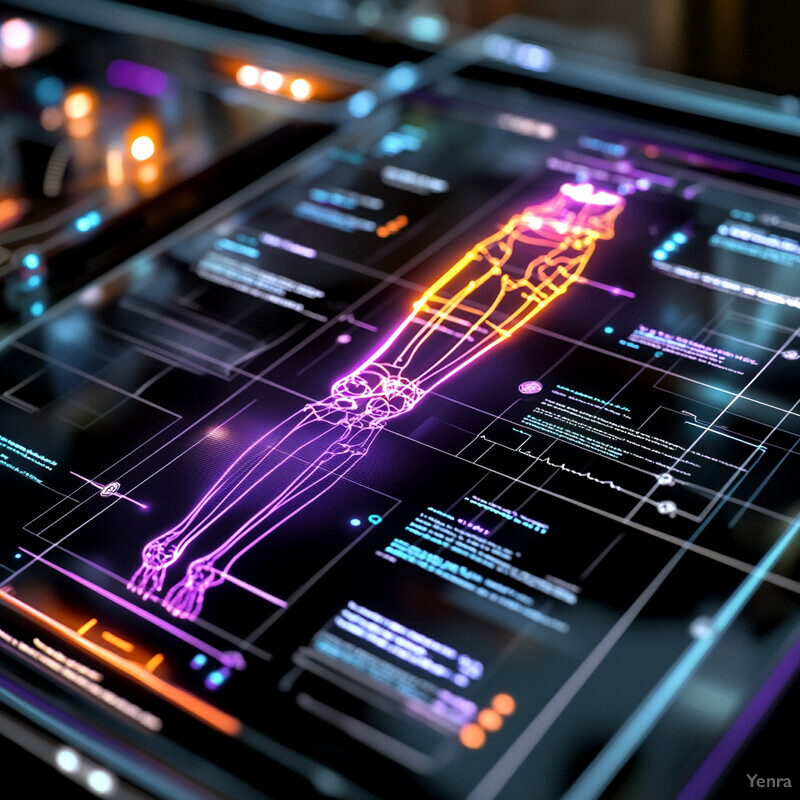
Traditionally, scoring joint damage from imaging scans is a time-consuming and sometimes subjective process. AI-driven computer vision models can rapidly analyze thousands of images, detecting and quantifying erosion, joint space narrowing, and cartilage damage in a consistent and reproducible way. These automated scoring systems reduce the burden on radiologists and rheumatologists, minimize inter-observer variability, and ensure that even subtle differences in disease progression are captured. More reliable data about damage levels allow for better monitoring, more precise outcome measurements in clinical trials, and more effective patient management strategies.
7. Personalized Treatment Recommendations
Reinforcement learning and predictive modeling can suggest optimal treatment regimens tailored to an individual patient’s disease subtype, genetic makeup, and response history.
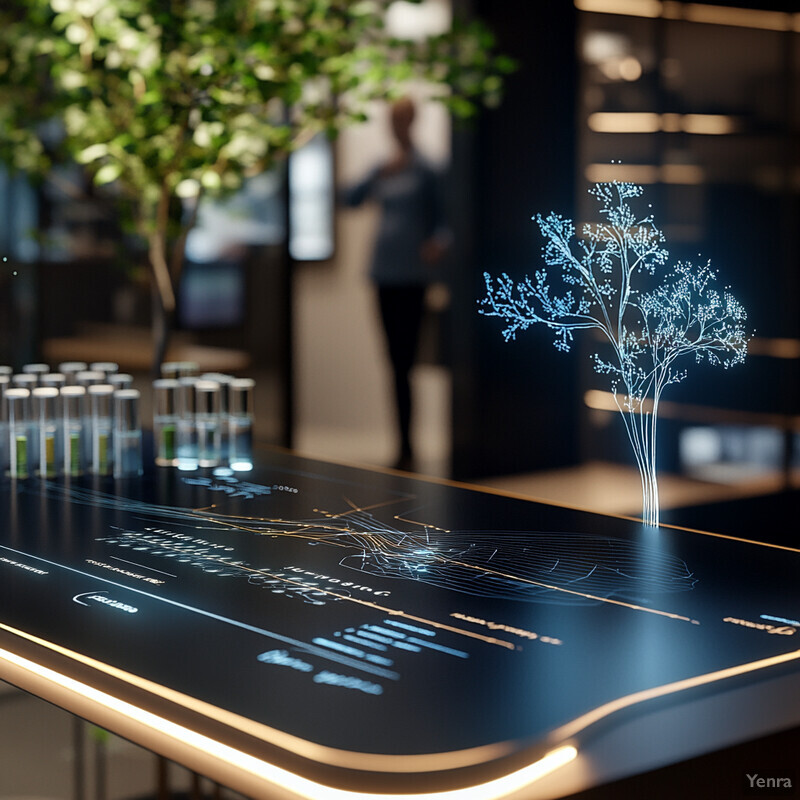
Patients with arthritis vary in their response to medications, from NSAIDs to biologics and JAK inhibitors. AI’s ability to analyze individual patient profiles, including genetics, prior treatment responses, comorbidities, and demographic factors, can guide the selection of the most beneficial therapy. Reinforcement learning and predictive analytics help determine which treatments are likely to produce the best outcomes for specific patient subtypes. By enabling clinicians to prescribe more effective treatments from the outset, AI reduces the trial-and-error period, improves patient quality of life, and can help cut costs associated with ineffective medications.
8. Monitoring Subclinical Changes
AI can detect subtle shifts in symptoms and biomarkers that precede clinical flare-ups, enabling timely interventions to prevent severe exacerbations.
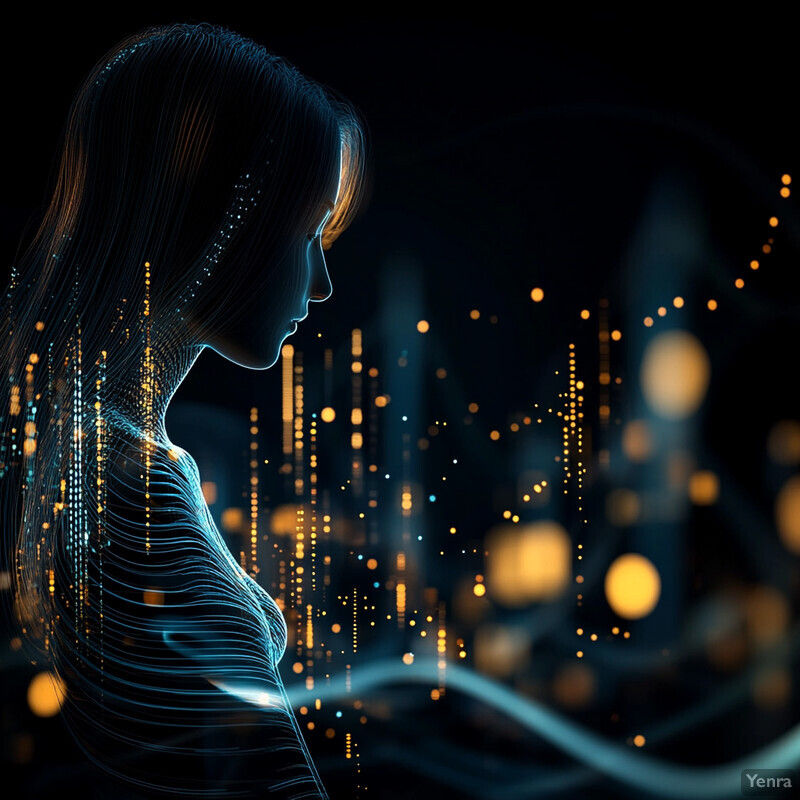
Before a full-blown arthritis flare emerges, subtle shifts in inflammation markers, pain levels, joint function, or patient activity might signal an impending exacerbation. AI can continuously track these subtle trends—analyzing wearable sensor data, lab values, and patient-reported pain scores—detecting patterns that presage a flare. With early warnings, patients and physicians can adjust treatments, increase anti-inflammatory measures, or rest the affected joints in a timely fashion. This proactive approach helps prevent severe pain episodes, joint damage, and the irreversible progression of the disease.
9. Drug Response Modeling
Predictive models can identify which patients are most likely to respond to certain biologics or small-molecule therapies, streamlining trial design and clinical decision-making.
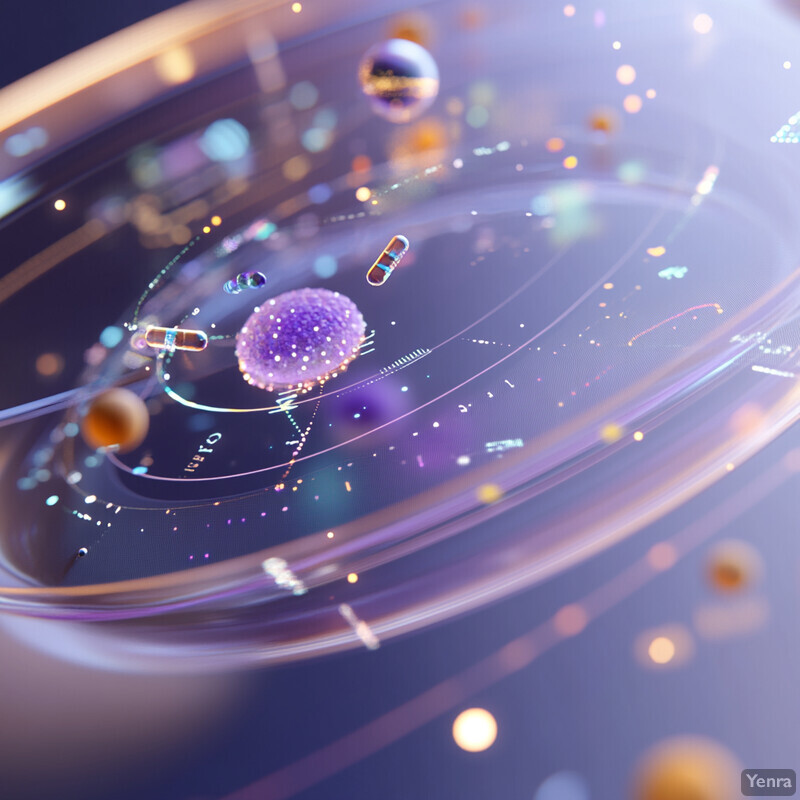
Understanding which patients will benefit from a particular medication is a persistent challenge in arthritis treatment. AI-driven drug response models integrate patient genotypes, phenotypes, and treatment histories, learning from large-scale patient databases and clinical trial results. By projecting the likely efficacy and potential side effects of drugs for specific patients, these models expedite therapeutic decision-making. The result is improved medication adherence, reduced adverse effects, and more rapid relief of symptoms, since the chosen interventions are more precisely aligned with the patient’s unique disease mechanism.
10. Adaptive Treatment Adjustments
Machine learning techniques can continuously analyze patient responses, adjusting medication doses and treatment plans as the patient’s condition evolves.
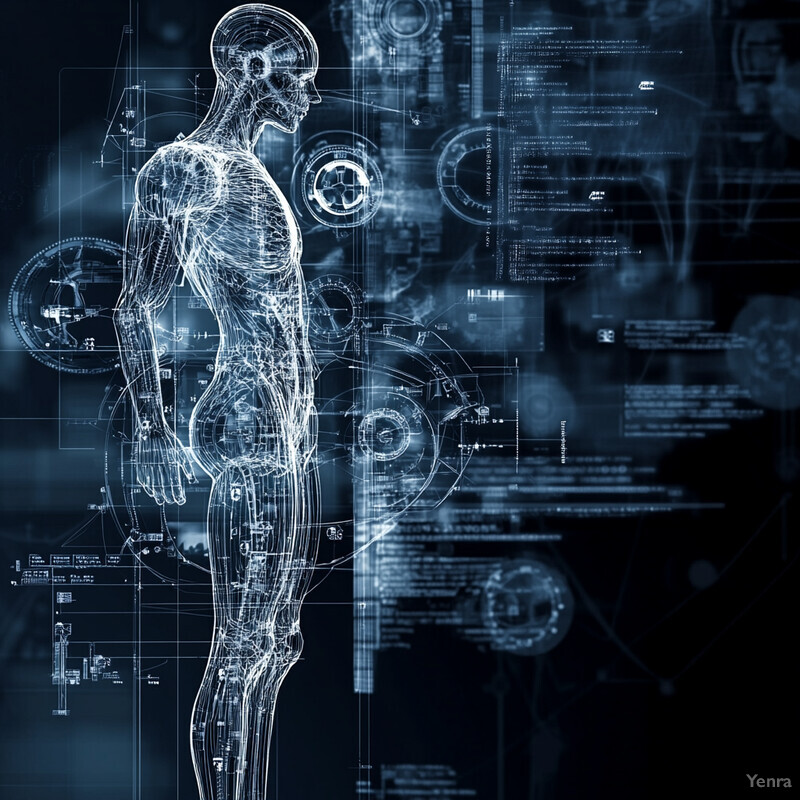
As patients undergo therapy, their condition evolves. AI-powered feedback loops analyze ongoing patient data—clinical check-ups, lab tests, wearable metrics—and detect when a patient is not responding optimally or when the disease is progressing despite treatment. By identifying these shifts early, clinicians can promptly adjust dosages, switch medications, or incorporate adjunct therapies. These adaptive strategies help maintain disease control, reduce the risk of long-term damage, and ensure that care is continuously aligned with the patient’s changing health status.
11. Multi-Omics Integration
AI can integrate genetic, proteomic, metabolomic, and microbiome data to understand molecular pathways driving arthritis progression, potentially revealing new therapeutic targets.
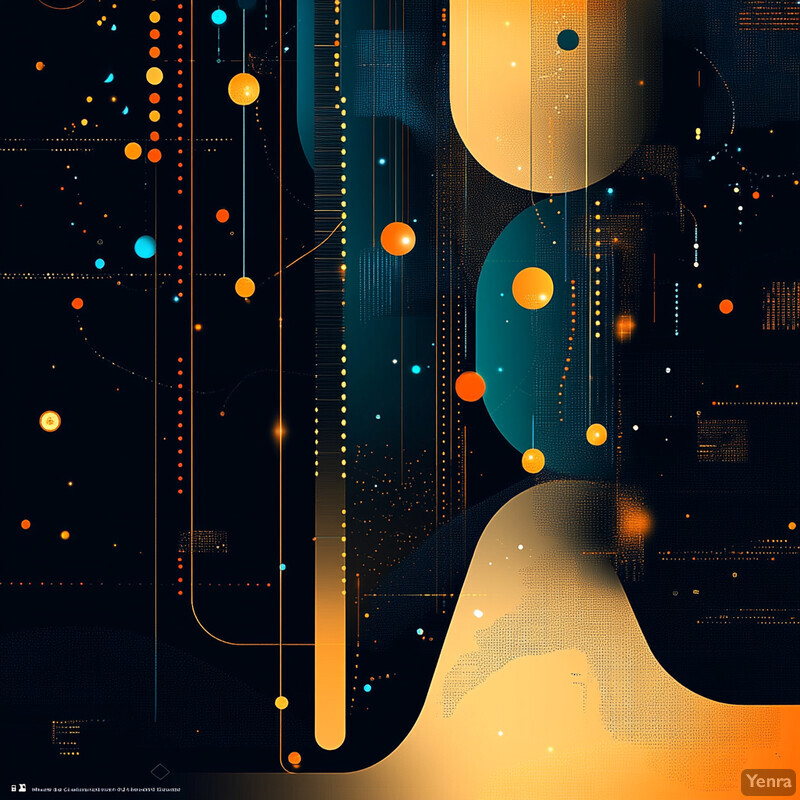
Arthritis progression is deeply rooted in intricate molecular and cellular pathways. AI can synthesize data from diverse omics layers—genomics, transcriptomics, proteomics, metabolomics, and even microbiome profiles—into a unified framework. By doing so, patterns that link molecular changes to clinical progression become more apparent, guiding the discovery of novel molecular targets for intervention. This integrative approach can accelerate research into new biomarkers and treatments, informing precision medicine strategies that directly address the underlying biological drivers of arthritis.
12. Subtyping and Phenotyping Arthritis
Unsupervised learning can cluster patients into distinct subgroups based on molecular and clinical features, helping physicians tailor interventions more effectively.
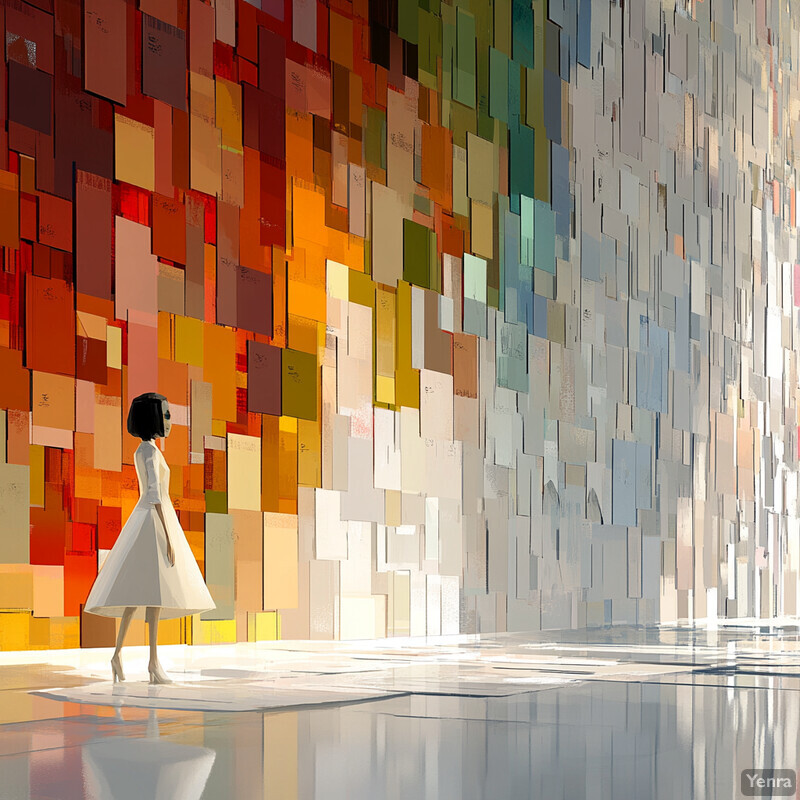
Arthritis encompasses multiple subtypes, each with distinct pathophysiology, progression patterns, and treatment responses. By applying unsupervised machine learning methods to large patient datasets, AI can identify clusters of patients who share similar molecular signatures, symptoms, or progression trends. These refined subtypes, or phenotypes, improve our understanding of disease heterogeneity and ensure that patients receive care that is tailored to their specific disease variant. The result is more accurate prognoses, more targeted treatments, and potentially improved outcomes.
13. Risk Stratification Models
Clinicians can use AI-derived risk scores to identify high-risk patients early, enabling closer monitoring, preventive therapies, and resource prioritization.
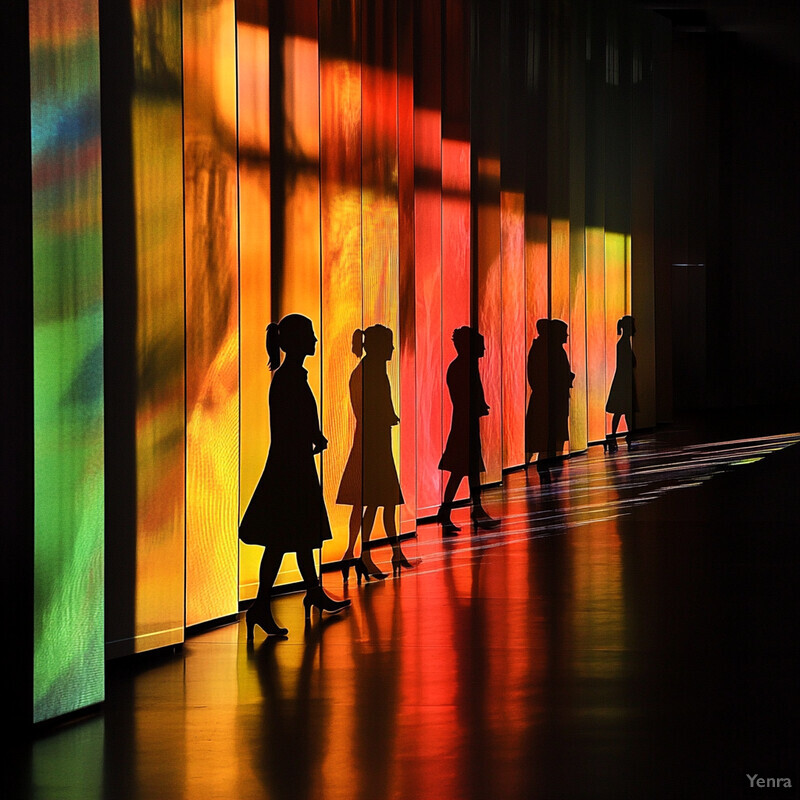
Not every arthritis patient faces the same risk of rapid disease progression, joint deformity, or complications. AI-derived risk scores integrate various predictive factors to stratify patients into high-, medium-, or low-risk categories. These categories help clinicians prioritize who might need more frequent monitoring, aggressive therapies, or early surgical interventions. By aligning healthcare resources more effectively, such risk models optimize patient care delivery, improve resource allocation, and can lead to better patient satisfaction and quality of life.
14. Digital Twin Simulation
Creating ‘digital twins’—virtual patient models—allows simulation of disease progression under various treatment strategies, guiding informed clinical decisions before they’re applied in real life.
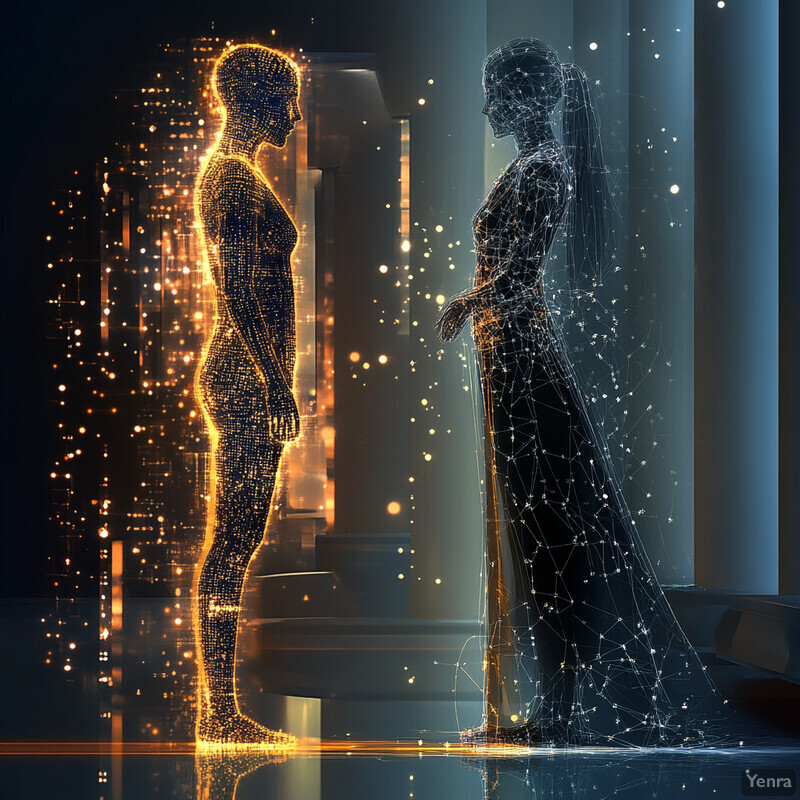
Imagine a virtual replica of a patient—called a “digital twin”—that simulates the patient’s disease progression under various hypothetical conditions. AI enables the creation and continuous refinement of these digital twins by integrating real-time patient data. Clinicians can “test drive” different treatments on the digital twin to predict which intervention might yield the best real-world outcome. This approach informs treatment decisions, helps avoid harmful side effects, and can potentially shorten the time to achieve optimal disease control.
15. Longitudinal Data Analysis
Recurrent neural networks and other temporal modeling techniques excel at analyzing how patients’ disease states evolve over years, extracting patterns from long-term health records.
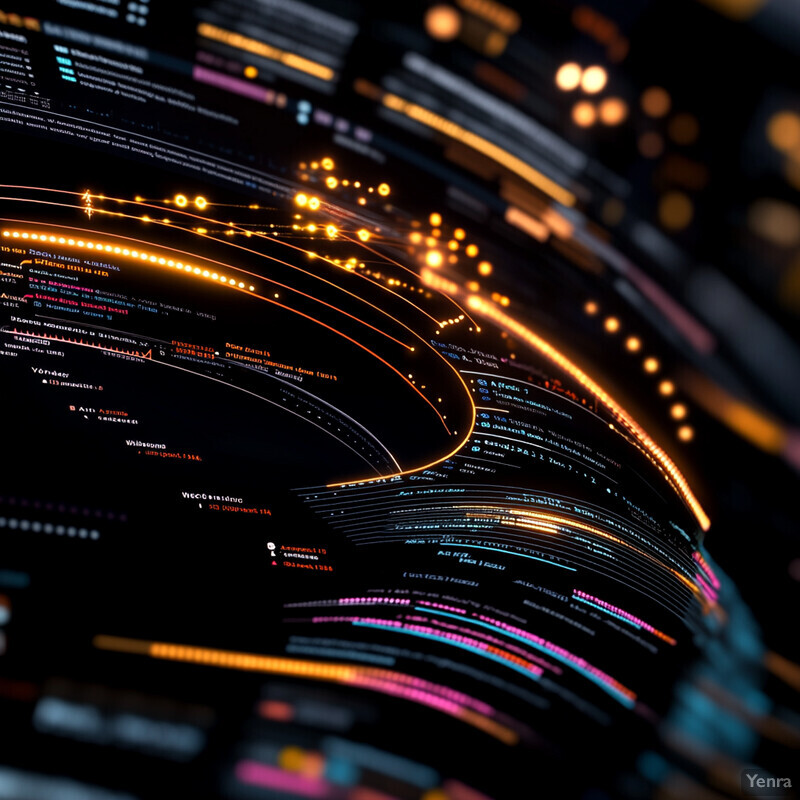
Arthritis unfolds over years or even decades, making longitudinal data crucial for understanding disease progression. AI models specialized in sequence or time-series analysis—such as recurrent neural networks or temporal convolutional networks—can reveal patterns in how a patient’s disease evolves over time. They integrate data from regular check-ups, imaging follow-ups, and treatment records, identifying meaningful trends and key turning points. Such insights enable more proactive disease management, guiding preventative measures and timing therapeutic interventions with greater precision.
16. Comorbidity Management
Since arthritis often co-occurs with other conditions (e.g., cardiovascular disease), AI can model the interplay between comorbidities, improving integrated care plans and outcomes.
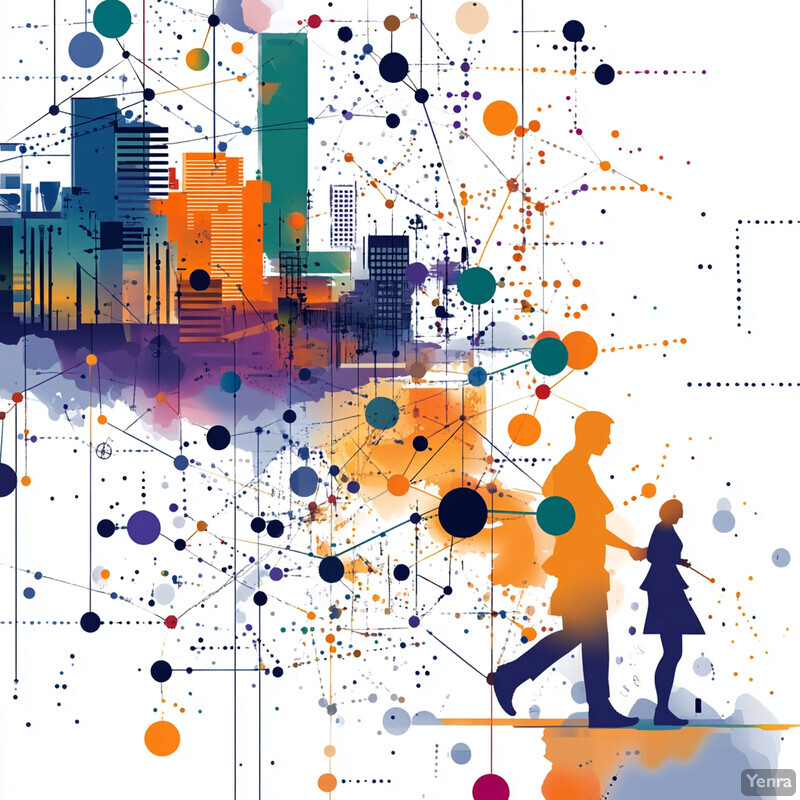
Patients with arthritis often contend with additional health issues, such as cardiovascular disease, diabetes, or mental health challenges. AI’s holistic data-crunching capabilities can model interactions between arthritis and comorbid conditions, highlighting how one disease might influence the course of another. By understanding these complex relationships, clinicians can design integrated treatment plans that address the whole patient rather than treating each condition in isolation. This integrated care improves overall outcomes, reduces complications, and enhances quality of life.
17. Predicting Surgical Outcomes
By evaluating patient history, imaging findings, and biomechanical data, AI can help predict joint replacement longevity, aiding in timing and selection of surgical interventions.
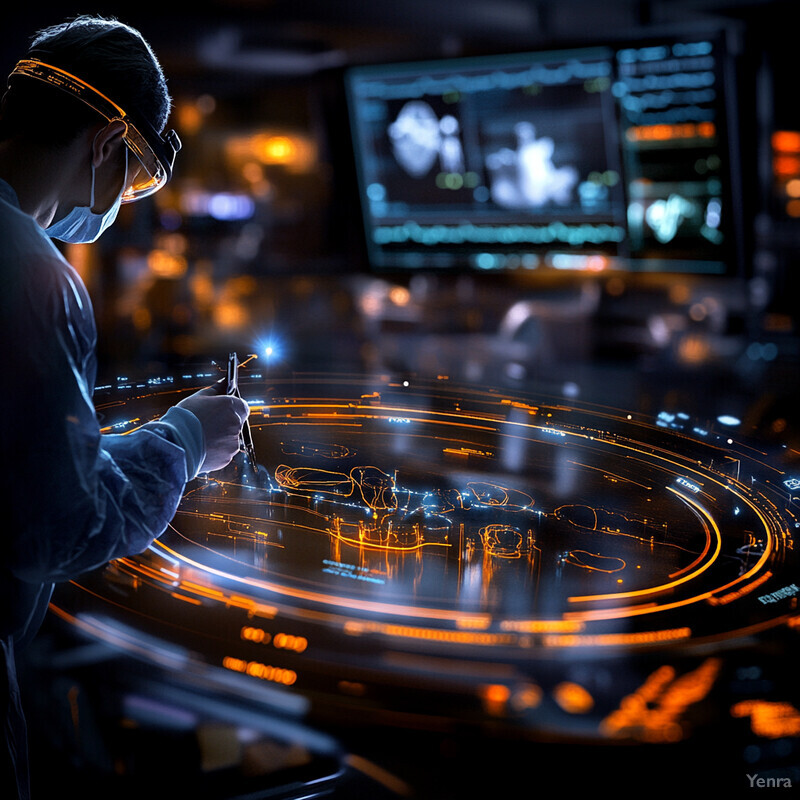
For some arthritis patients, surgery—such as joint replacement—becomes an eventual necessity. AI can draw upon demographic information, imaging findings, biomechanical assessments, and laboratory data to predict how well a patient might fare after surgery. Such predictions help surgeons refine patient selection criteria, determine the optimal timing for the procedure, and anticipate the need for postoperative rehabilitation. As a result, patients experience better long-term function and satisfaction, and healthcare systems can allocate surgical resources more efficiently.
18. Real-Time Wearable Data Utilization
Wearable health-monitoring devices capture subtle changes in gait, activity level, and pain. AI models can interpret this continuous data to track disease activity and recommend timely care adjustments.
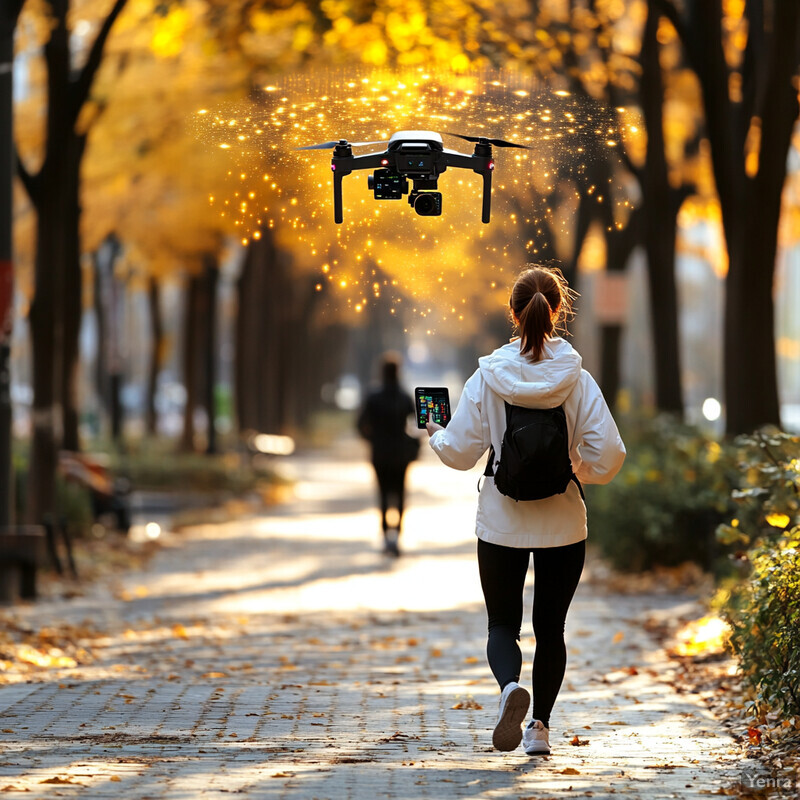
Continuous monitoring devices—smartwatches, smart insoles, and advanced activity trackers—provide valuable, round-the-clock data on patient mobility, joint loading, pain patterns, and fatigue. AI can interpret these data streams to correlate fluctuations in daily activity or gait changes with disease flare-ups or treatment responses. This ongoing feedback loop allows clinicians to adjust therapies in real-time and encourages patients to better manage their condition through timely behavior modifications. Ultimately, it supports more responsive, patient-centered care.
19. Natural Language Processing of Clinical Notes
NLP models can extract valuable progression indicators from clinician notes, patient diaries, and research literature, enriching data sources beyond structured charts and lab results.
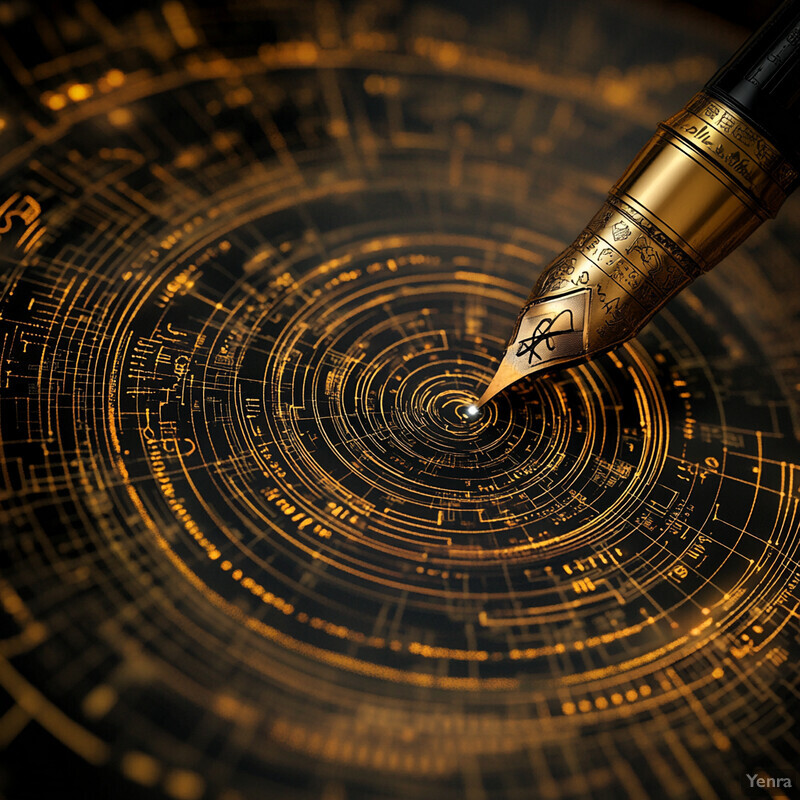
Medical records and clinician notes are rich sources of unstructured information that can contain subtle clues about disease progression. Natural language processing (NLP) algorithms can sift through these texts, extract relevant details, and integrate them into patient models. By converting narrative descriptions into structured, actionable data, NLP helps ensure that no valuable clinical insight is lost. Over time, this leads to more accurate patient histories, better predictions of disease course, and improved collaboration among care teams.
20. Accelerated Clinical Research
By quickly sifting through extensive datasets, AI can identify patterns and generate hypotheses that guide clinical studies, speeding up the discovery of effective arthritis treatments and management strategies.
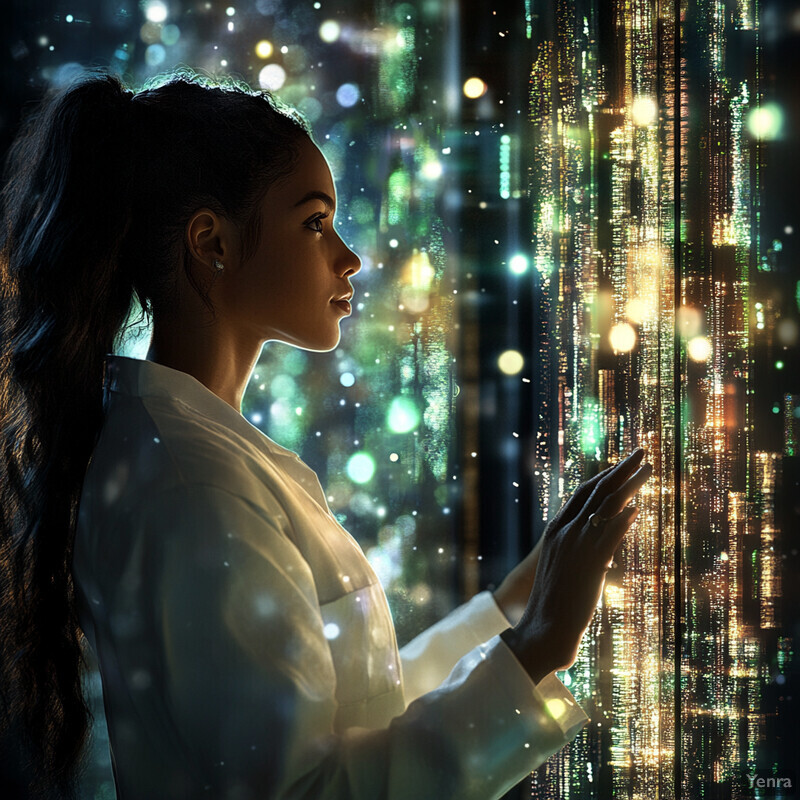
As AI can handle massive amounts of data at remarkable speed, it aids researchers by rapidly identifying patterns, testing hypotheses, and generating new leads for clinical studies. Machine learning models can detect subtle associations between treatments, genetics, and disease outcomes that would take humans years to uncover. This capacity to accelerate insight generation helps refine clinical trial designs, shortens drug development cycles, and ensures that effective new therapies reach patients faster. By empowering research teams, AI significantly contributes to a more efficient scientific pipeline and ultimately improves the standard of care for arthritis patients.