1. Early Disease Detection via Image Recognition
AI-driven computer vision algorithms can analyze underwater camera footage to identify subtle signs of infection, parasites, or lesions in fish long before these become apparent to the human eye.
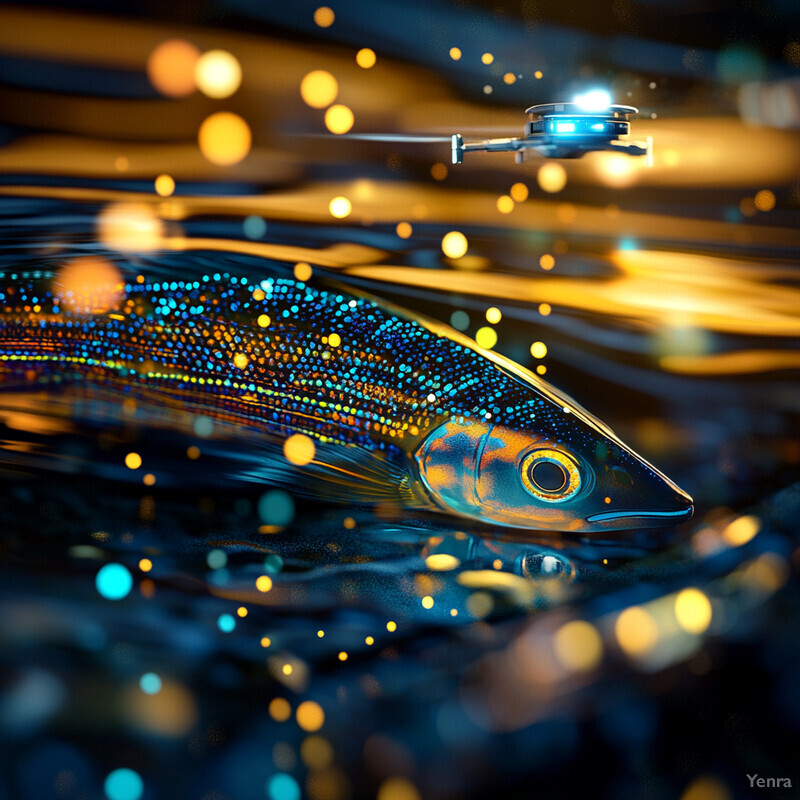
AI-driven computer vision systems leverage sophisticated image recognition algorithms to scrutinize high-resolution underwater videos. By analyzing patterns, textures, and color variations on fish skin and body shape, these systems can detect early indicators of diseases—such as fungal infections, parasitic infestations, or bacterial lesions—days or even weeks before visual signs become noticeable to the human eye. This proactive detection mechanism empowers aquaculture managers to intervene promptly with targeted treatments, reducing both the severity of disease outbreaks and the economic losses associated with delayed responses.
2. Real-Time Behavioral Monitoring
Advanced machine learning models track changes in fish swimming patterns, grouping behavior, and feeding responses, detecting deviations that may indicate health problems or stress.
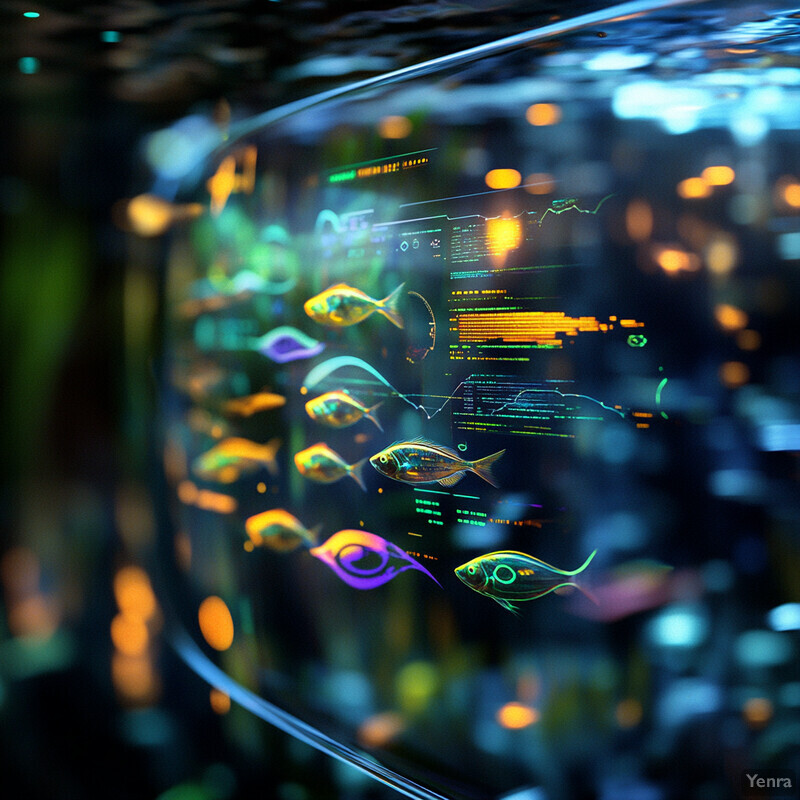
In addition to physical symptoms, behavioral changes can be a strong indicator of compromised fish health. AI-powered tools continuously monitor factors like swimming speeds, schooling formation, feeding aggression, and vertical distribution within the water column. Machine learning models, trained on large behavioral datasets, detect subtle deviations from typical activity patterns. For instance, lethargy, abnormal clustering, or erratic darting can signal stress, infections, or suboptimal environmental conditions. By receiving immediate alerts on behavioral anomalies, aquaculture farmers can initiate timely interventions to restore healthy conditions and improve overall stock welfare.
3. Environmental Parameter Integration
AI systems can continuously synthesize data from multiple sensors (e.g., dissolved oxygen, temperature, pH, ammonia) to provide a holistic view of water quality and its impacts on fish health.
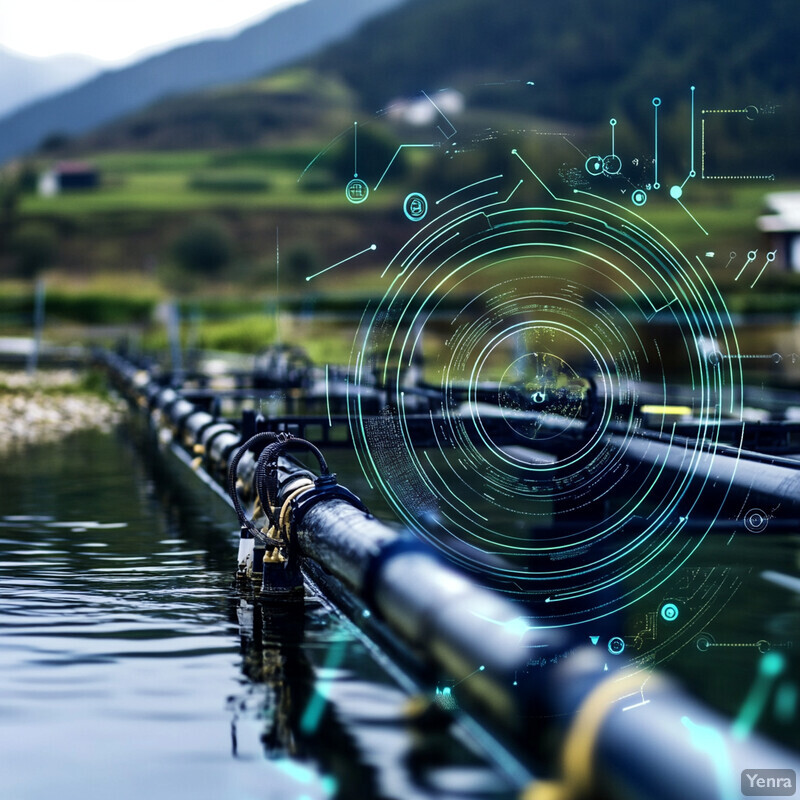
A fish’s well-being depends not only on its internal health but also on the external environmental conditions. AI integrates data streams from sensors measuring temperature, dissolved oxygen, ammonia, salinity, pH, and turbidity, providing a unified, real-time picture of the aquatic environment. Advanced analytics and decision-making algorithms interpret these data together, identifying complex interactions—such as low oxygen levels combined with high ammonia—that predispose fish to disease. This holistic environmental understanding enables tailored adjustments to aeration, feeding, and filtration systems, optimizing conditions and preventing the onset of disease.
4. Predictive Disease Modeling
Through historical data and pattern recognition, AI can forecast the likelihood and timing of disease outbreaks, allowing farmers to take preventative measures before symptoms appear.
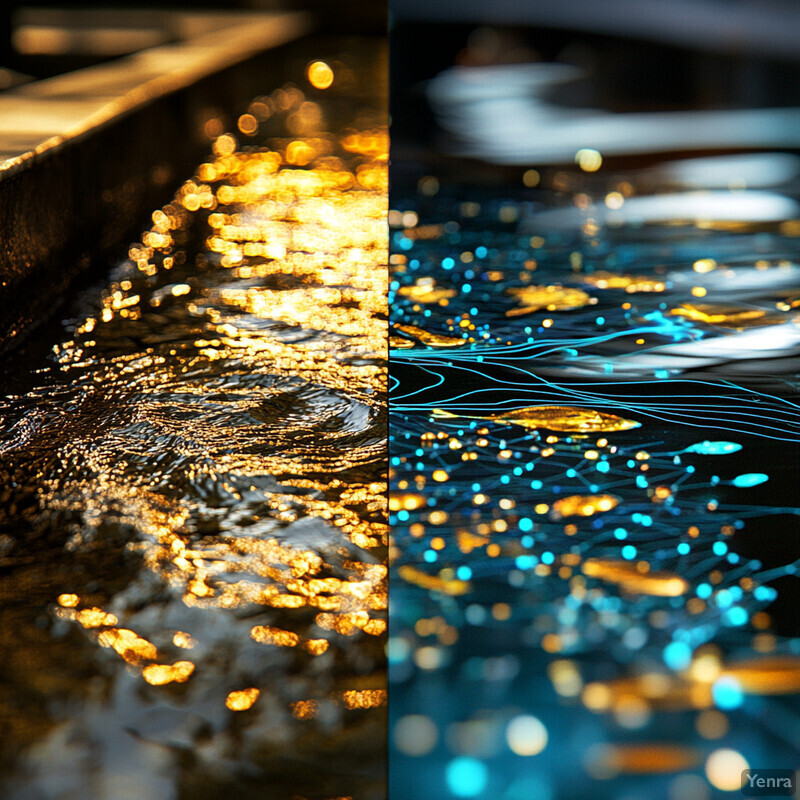
Historical farm data, combined with current conditions, feed into predictive modeling frameworks powered by AI and machine learning. By analyzing past disease outbreaks, environmental fluctuations, and seasonal changes, these models estimate the probability and timing of impending disease threats. For instance, patterns might reveal that certain parasitic blooms typically occur when water temperatures and nutrient levels reach a specific threshold. Armed with this foresight, farm managers can apply prophylactic treatments, adjust feeding regimes, or implement additional hygiene measures before a disease emerges, effectively mitigating its impact.
5. Automated Feeding Optimization
Intelligent feeding systems use AI to adjust feed delivery based on fish appetite, growth rates, and health indicators, ensuring optimal nutrition and reducing waste that can degrade water quality.
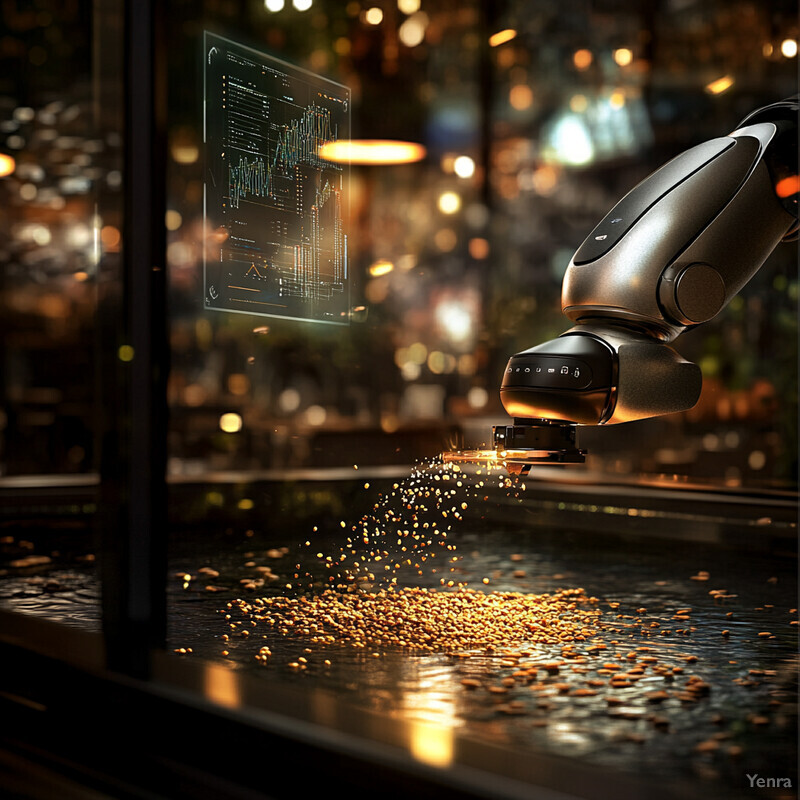
Feeding strategies in aquaculture must strike a balance between maximizing growth and maintaining clean, stable conditions. AI-driven feeding systems use real-time inputs—such as fish appetite indicators, biomass estimates, and environmental parameters—to fine-tune feeding schedules and quantities. By minimizing overfeeding, farmers reduce excess nutrient load and organic waste, which can degrade water quality and foster harmful pathogens. At the same time, ensuring fish receive the right diet at the right times supports robust immune systems and enhances disease resistance. This precision feeding approach leads to healthier stocks and more sustainable resource use.
6. Mortality Risk Assessment
Statistical and machine learning techniques can analyze current and historical farm conditions to predict mortality rates, guiding proactive interventions to reduce losses.
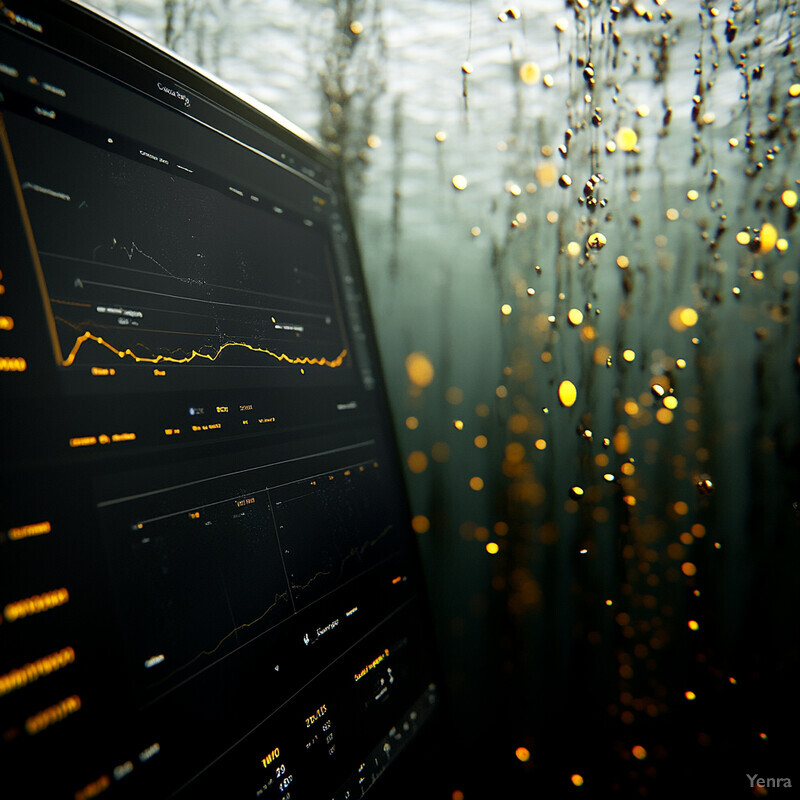
Mortality events often result from complex interactions of disease, environmental stress, and nutritional deficiencies. AI-powered risk assessment tools combine historical mortality data, present farming conditions, and predictive models to estimate future mortality probabilities. By identifying key drivers—such as deteriorating water quality or subclinical infections—farmers gain a practical early warning system. Proactive measures, whether they involve adjusting water parameters, administering preventative medications, or improving biosecurity measures, can then be implemented to minimize losses and protect the farm’s profitability and reputation.
7. Genomic and Metagenomic Analysis
AI can process genetic and microbiome data at large scales, identifying pathogenic organisms or resistance genes, which improves the speed and accuracy of disease diagnosis.
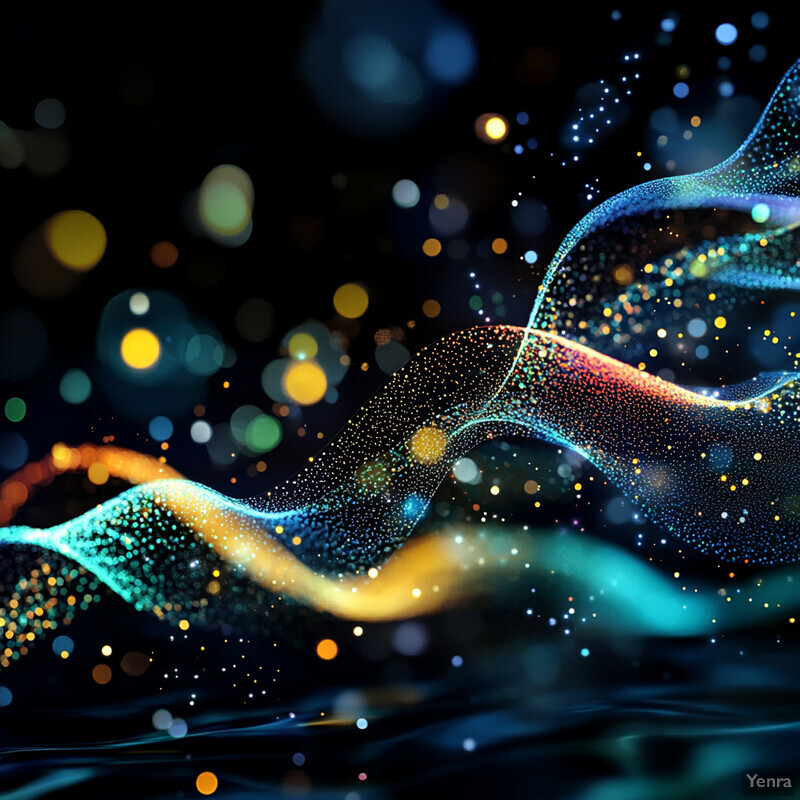
Advanced genetic sequencing techniques produce vast amounts of genomic and metagenomic data on farmed species and their surrounding microbiomes. AI algorithms can sift through these complex datasets to pinpoint the presence of pathogens, identify genes associated with disease resistance, and map the overall microbial balance in the environment. By doing so, aquaculture operators can select robust genetic strains less prone to infection and tailor probiotic or antimicrobial treatments to support beneficial microorganisms. This genomic insight fosters healthier stocks, improves survival rates, and reduces the reliance on antibiotics and chemical treatments.
8. Anomaly Detection in Sensor Data
Unsupervised machine learning models can detect irregular patterns in sensor readings—such as sudden drops in dissolved oxygen—that may signal emerging health threats.
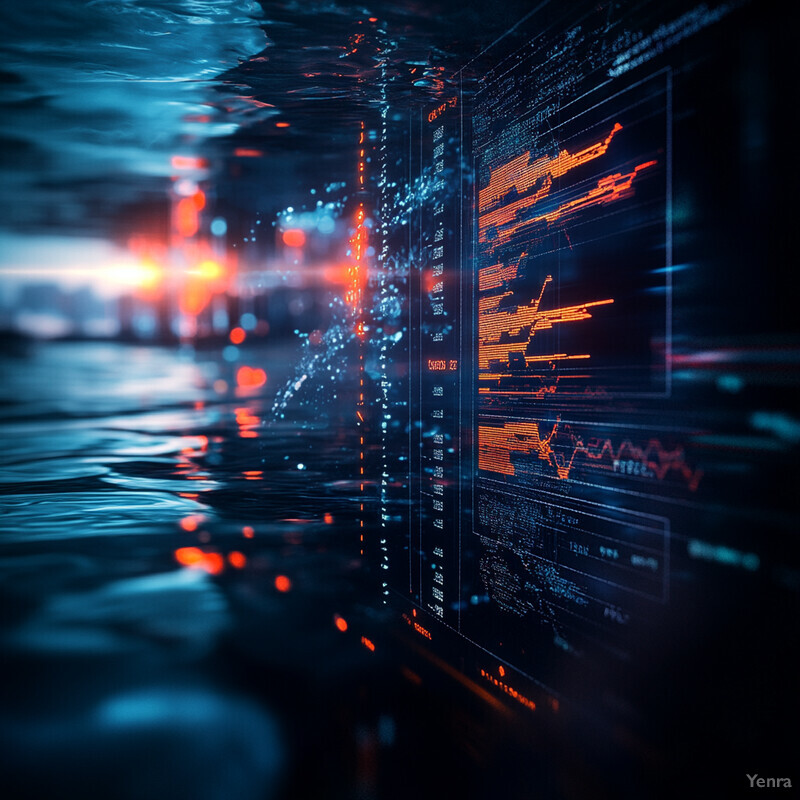
Aquaculture environments rely on a host of continuous data streams from sensors measuring parameters like dissolved oxygen, temperature, pH, and turbidity. Machine learning techniques—especially unsupervised learning—excel at detecting anomalies within these data patterns. Sudden drops in oxygen levels, unexpected spikes in ammonia, or unexplained fluctuations in temperature can all signal emerging health threats. By identifying these anomalies as soon as they arise, AI systems provide an advanced warning, allowing farmers to rapidly adjust aeration, filtration, or feeding regimes before conditions worsen and fish health suffers.
9. Precision Medicine for Aquatic Species
Integrating AI with veterinary diagnostics can tailor treatments and medication dosages, optimizing therapeutic responses and minimizing overuse of antibiotics.
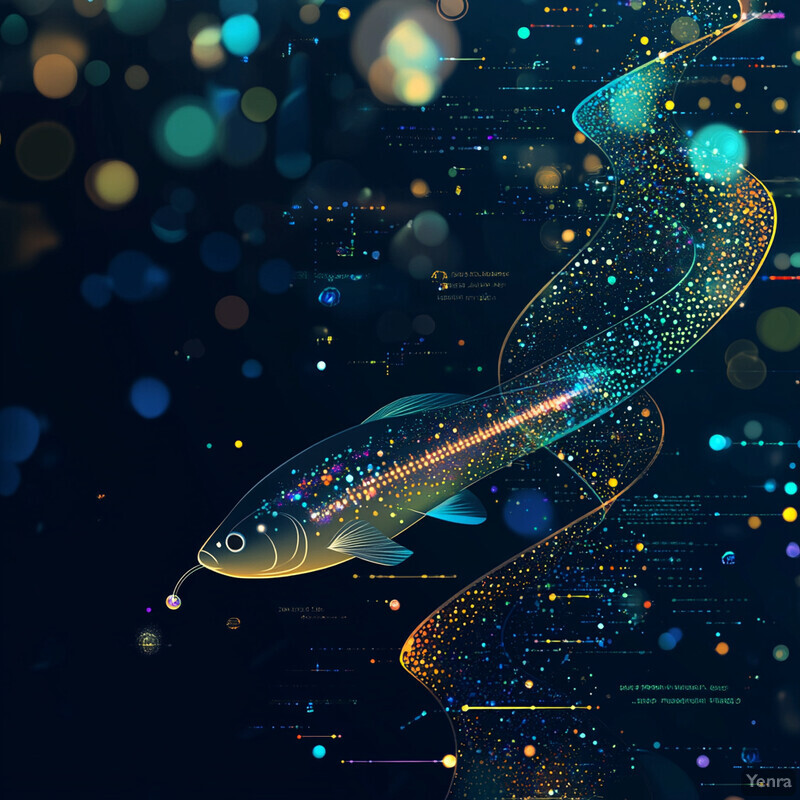
Treating diseases in farmed fish often involves a “one-size-fits-all” approach, which can lead to ineffective treatments, antimicrobial resistance, and ecosystem harm. AI-driven precision medicine adapts treatment strategies by analyzing a wealth of data on the fish’s genetics, immune profile, and current health status, as well as past responses to medications. By determining the optimal dosage and timing of treatments on a case-by-case basis, farmers can achieve better recovery rates, lower drug usage, and help maintain a healthier aquaculture environment conducive to long-term sustainability.
10. Harmful Algal Bloom Prediction
AI-driven environmental forecasting models help anticipate harmful algal blooms, allowing timely mitigation efforts to protect fish health.
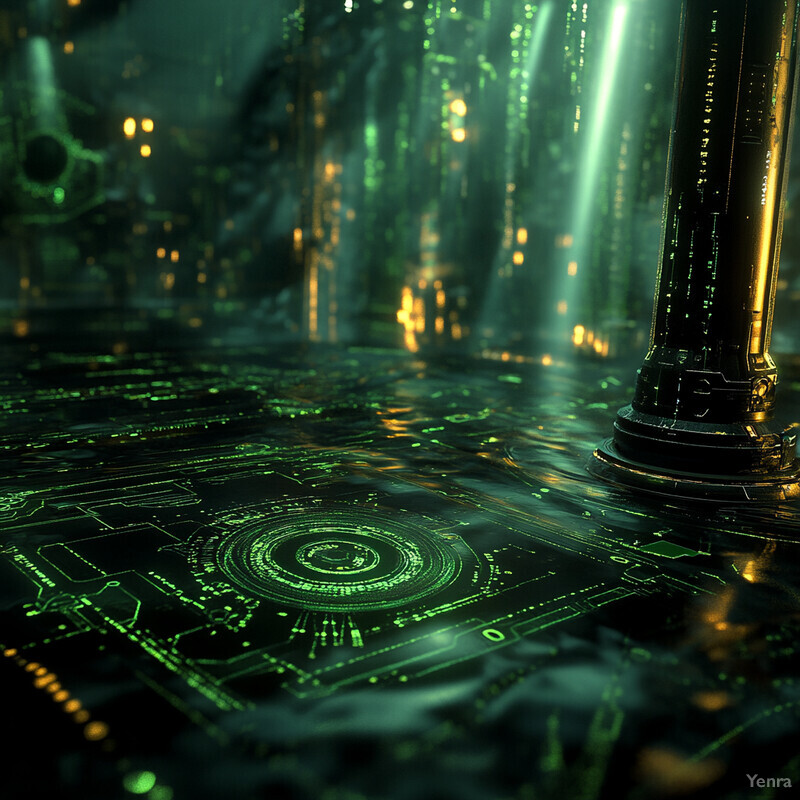
Harmful algal blooms (HABs) pose a serious threat to aquaculture, as toxin-producing algae can cause mass mortalities and significant financial losses. AI models that integrate meteorological data, water chemistry, and historical bloom records can predict the onset and severity of these events. Early detection helps farmers take measures such as adjusting stocking densities, deploying aerators, or relocating cages to safer areas. By anticipating HABs, aquaculture operations reduce the risk of large-scale losses and maintain fish health in the face of natural environmental hazards.
11. Underwater Robotic Inspection
Autonomous underwater vehicles and drones, guided by AI, can systematically scan cages or net pens, detecting injured or diseased fish without human divers.
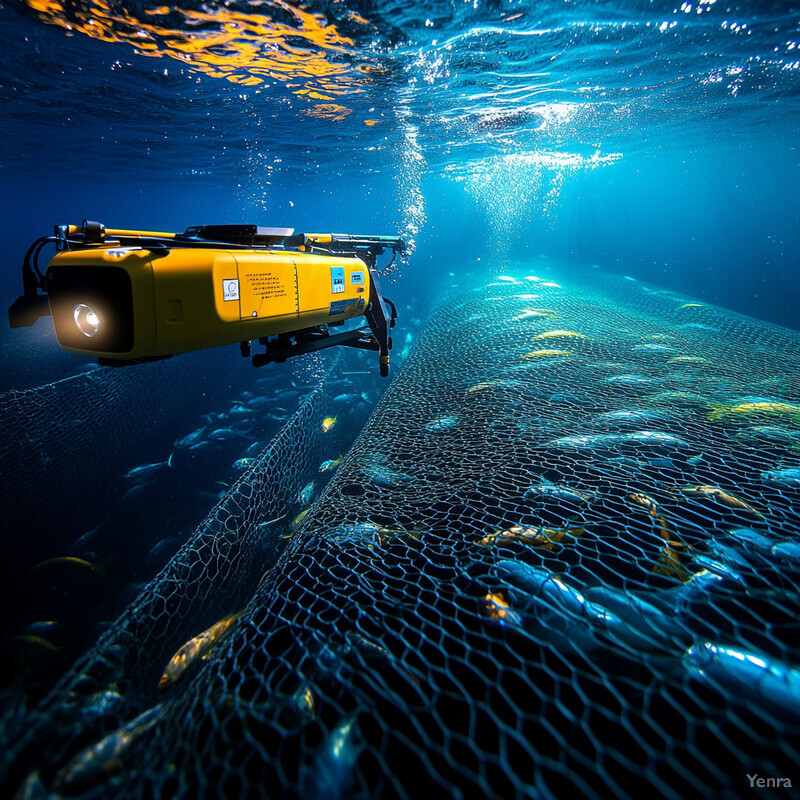
Human divers cannot constantly monitor underwater enclosures, especially in large-scale aquaculture operations or in environments that pose safety risks. AI-guided autonomous underwater vehicles and drones are equipped with cameras, sensors, and navigational aids. These robots patrol pens or cages, scanning fish for external injuries, parasites, or abnormal behaviors. Through computer vision and pattern recognition, anomalies are flagged for human review. This reduces the labor costs, safety concerns, and error margins of manual inspections, ensuring more consistent and thorough assessments of fish health.
12. Biomass Estimation and Health Indicators
AI-enabled image processing provides more accurate biomass estimates and identifies growth irregularities, which can be early signs of health issues.
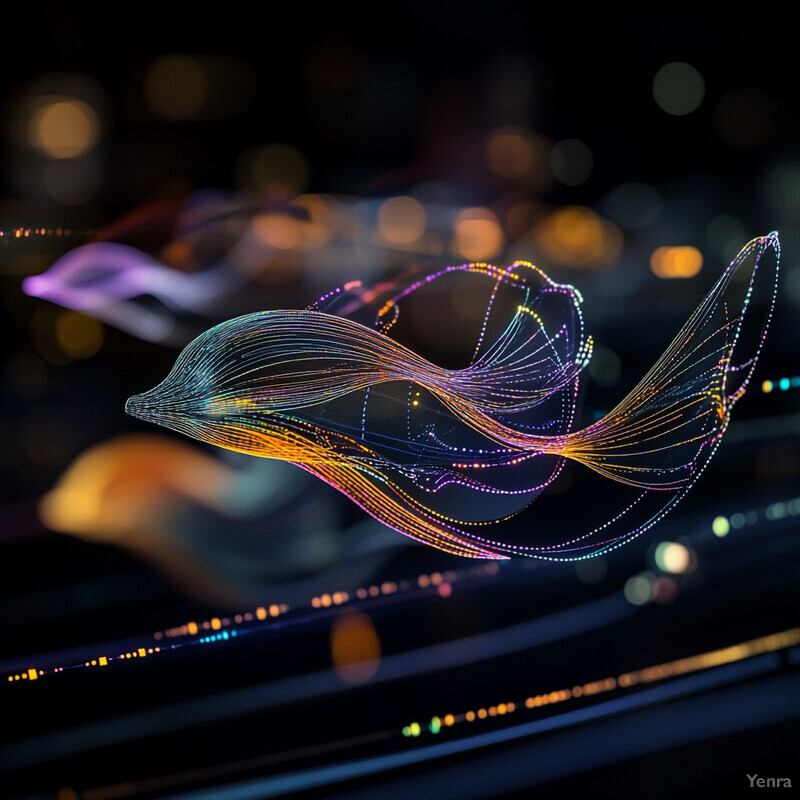
Estimating fish biomass in real-time is critical for managing feed and stock density, as well as monitoring growth rates. AI tools analyze video footage or sonar data to estimate biomass accurately and identify size discrepancies or growth anomalies that may indicate health issues. For example, if a subgroup of fish grows noticeably slower than the rest, it can signify disease, poor nutrition, or genetic weaknesses. By capturing this information early, farmers can isolate affected groups, adjust feeding strategies, or administer health checks, thereby preventing widespread health decline.
13. Stress Level Detection Through Movement Analytics
AI can infer stress levels by analyzing subtle changes in fish acceleration, turning angles, and spatial distribution, prompting early interventions.
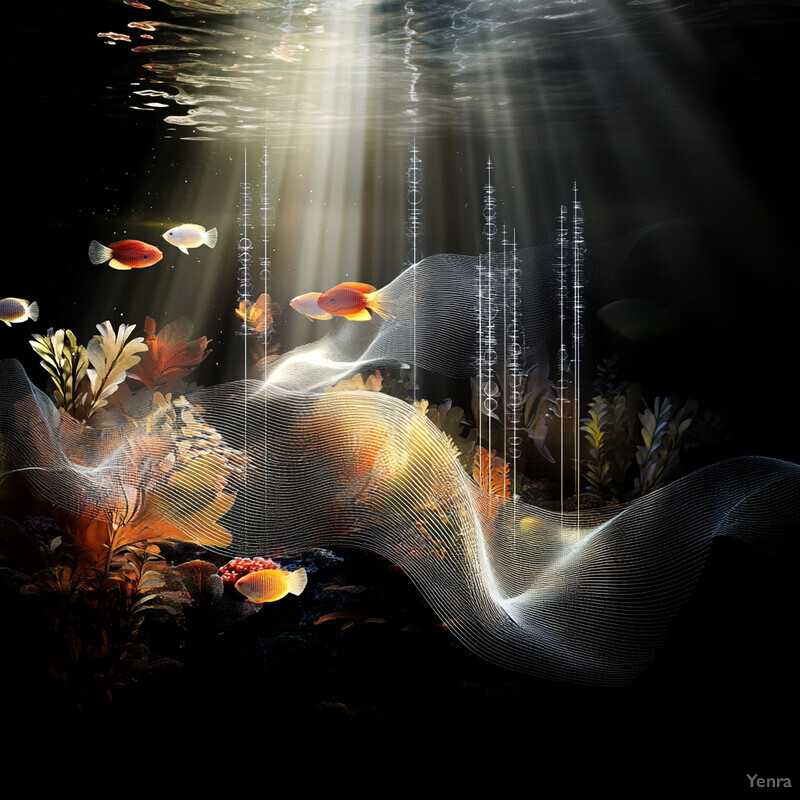
Stress is a precursor to weakened immune response and susceptibility to disease. With AI-based movement analytics, subtle changes in velocity, schooling patterns, turning angles, and depth preferences become quantifiable indicators of stress levels. By detecting trends—such as fish spending more time near the water surface or forming unusually tight schools—farmers can identify periods of stress caused by overcrowding, suboptimal feeding routines, or poor water conditions. Timely corrective measures, like adjusting stocking densities or improving water quality, mitigate these stressors and maintain healthier fish populations.
14. Real-Time Alert Systems
AI continuously evaluates health and environmental metrics, sending automated alerts to farm managers when critical thresholds are reached, ensuring rapid responses.
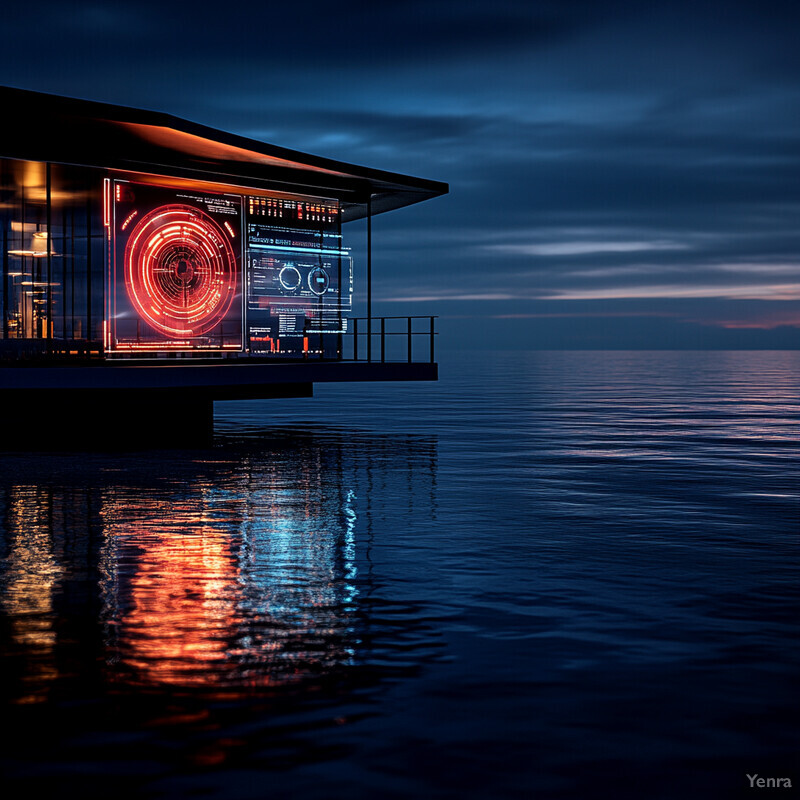
The aquaculture environment is dynamic, with conditions that can shift rapidly. AI-driven real-time alert systems integrate various data streams—behavioral observations, water parameters, growth metrics—and apply threshold-based or predictive triggers. Once a critical threshold is breached or an anomalous pattern is detected, farm managers receive immediate notifications via mobile or desktop dashboards. Rapid responses to these alerts can prevent minor issues from escalating into full-blown crises, safeguarding both animal welfare and the farm’s economic viability.
15. Long-Term Health Trend Analysis
Advanced analytics can highlight multi-season or multi-year health trends, informing improved management strategies and better stock selection.
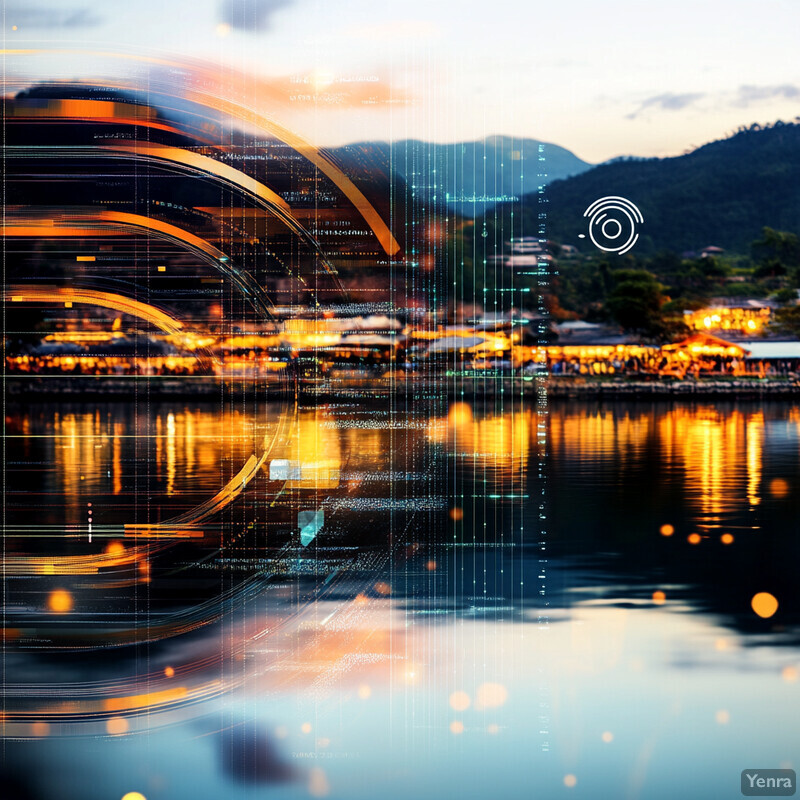
Beyond immediate responses, AI excels at uncovering long-term trends in fish health. By examining seasonality, historical disease outbreaks, feed composition shifts, and genetic changes, these systems help identify recurring patterns or vulnerabilities. For example, if a certain pathogen reappears each spring due to temperature changes and nutrient availability, farmers can implement seasonal preventative measures. Over time, this data-driven approach informs strategic decision-making—such as adjusting feed formulations, upgrading filtration systems, or improving quarantine protocols—that leads to more resilient operations.
16. Adaptive Vaccination Scheduling
By understanding disease cycles and fish immune responses, AI can optimize vaccination timing and protocols, improving overall health outcomes.
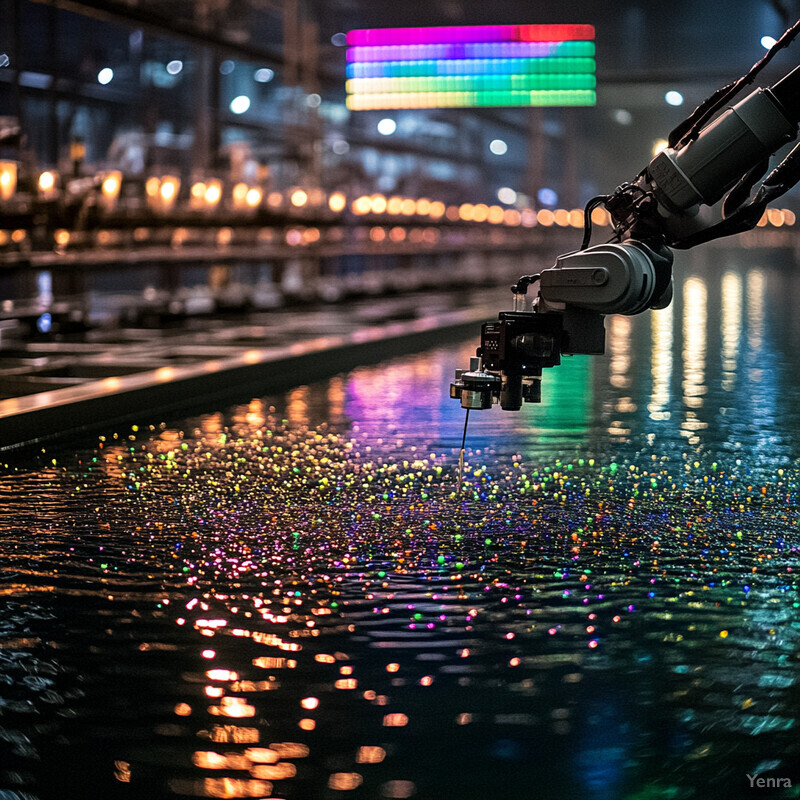
Vaccinations are a cornerstone of disease prevention, but their timing and frequency can significantly influence their efficacy. AI platforms analyze data on pathogen prevalence, environmental cues, fish age, growth rates, and immune response indicators to recommend optimal vaccination windows. By delivering vaccines when fish are most likely to build strong immunity, farmers reduce vaccine waste, limit stress on the animals, and enhance overall disease resilience within the population. This adaptive scheduling ensures a healthier stock while minimizing costs and environmental impacts.
17. Nutritional Profile Optimization
AI can evaluate feed ingredient efficacy and recommend formulations that bolster immune function, resulting in more disease-resistant fish populations.
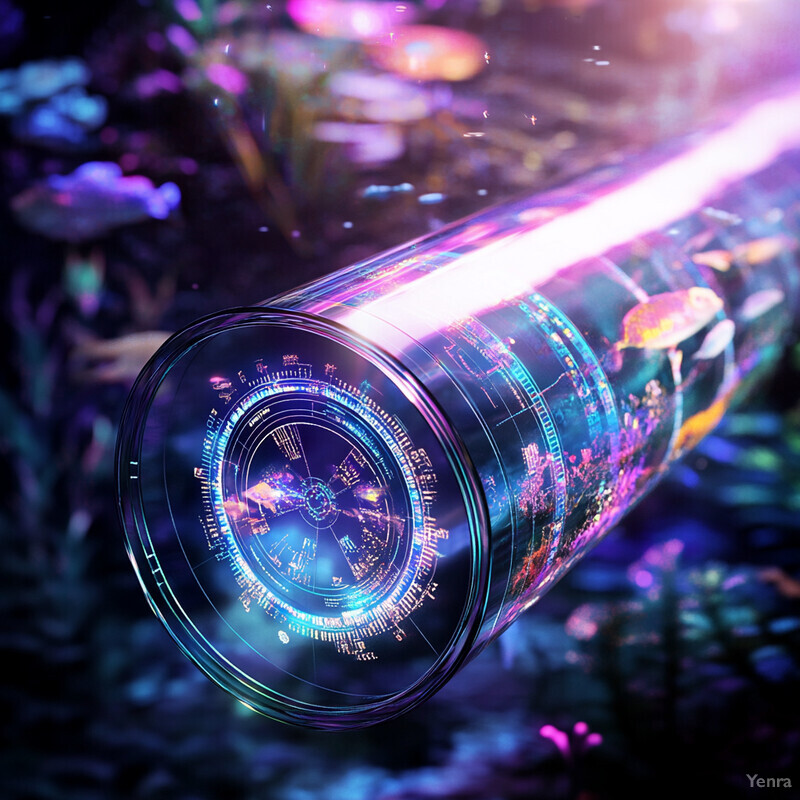
Nutrition is integral to immune system strength, resistance to pathogens, and overall health. AI-driven analysis of feed ingredients, fish metabolic responses, growth rates, and health outcomes leads to highly customized nutritional profiles. For instance, if data shows that certain probiotics or vitamins correlate with lower mortality rates and fewer infections, feed formulas can be refined accordingly. Adjusting nutrient compositions to bolster fish immune function helps prevent disease outbreaks, decreases reliance on medication, and results in more sustainable production practices.
18. Chemical Treatment Reduction
Better early detection and predictive modeling reduce the need for emergency chemical interventions, lowering costs and improving environmental stewardship.
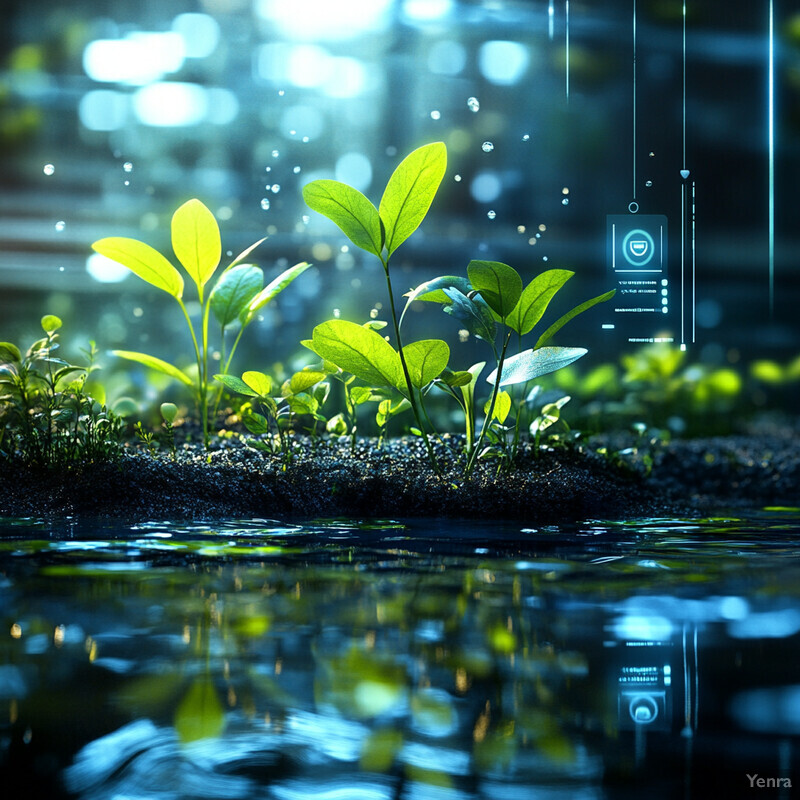
Historically, chemical treatments and antibiotics have been used to counter diseases and parasites. However, overreliance can lead to resistant pathogens and environmental harm. AI’s capacity for early disease detection, environmental monitoring, and predictive modeling reduces the need for emergency chemical interventions. By tackling health issues proactively, farmers can maintain cleaner, healthier systems without resorting to frequent chemical inputs. This approach safeguards the farm’s long-term sustainability, improves consumer perceptions, and aligns with growing regulatory and market demands for environmentally responsible aquaculture practices.
19. Improved Quarantine Protocols
AI-driven risk assessments help isolate and manage suspect batches efficiently, reducing the spread of pathogens throughout the facility.
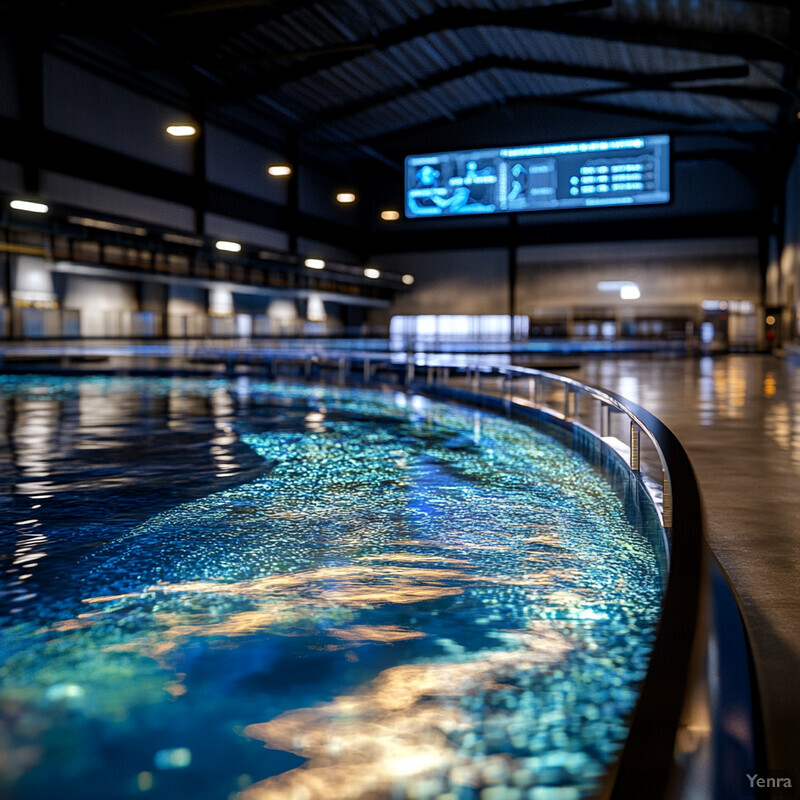
When introducing new fish stocks or suspecting infections, effective quarantine protocols are essential. AI-powered risk assessment tools evaluate the health status of incoming batches by analyzing their origin, historical health data, and current stress markers. This allows for targeted and dynamic quarantine measures, isolating only those sub-populations at higher risk rather than imposing blanket restrictions. By optimizing quarantine protocols, farmers reduce the chance of disease spreading throughout their facilities, minimize unnecessary productivity losses, and maintain healthier, more robust aquaculture systems.
20. Regulatory Compliance and Traceability
By maintaining detailed, AI-managed health and treatment logs, farms can ensure compliance with governmental regulations and provide traceable, transparent health records to consumers.
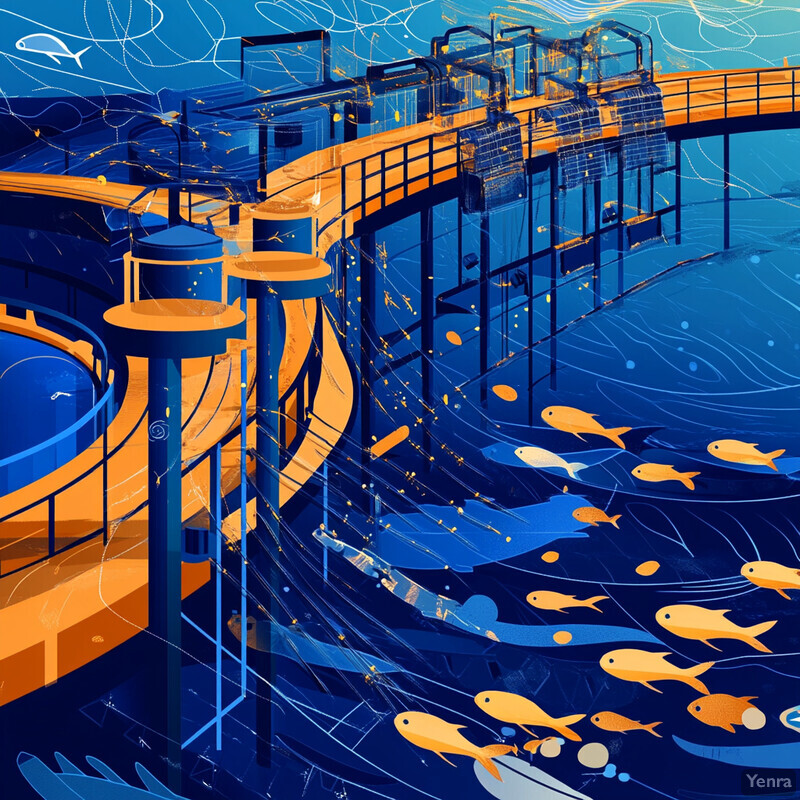
Health monitoring in aquaculture is not only about safeguarding fish but also ensuring compliance with environmental standards and food safety regulations. AI-compiled health records, treatment logs, and environmental data form a comprehensive digital audit trail. These detailed, easily accessible records support transparent reporting to authorities, certification bodies, and end consumers. Improved traceability builds trust with buyers and retailers, reinforces brand integrity, and meets the growing demand for responsibly sourced, high-quality seafood. In this way, AI enhances both operational excellence and market competitiveness.