1. Advanced Transaction Monitoring
AI algorithms can process vast volumes of transaction data in real-time, quickly flagging unusual patterns or behaviors that may indicate money laundering.
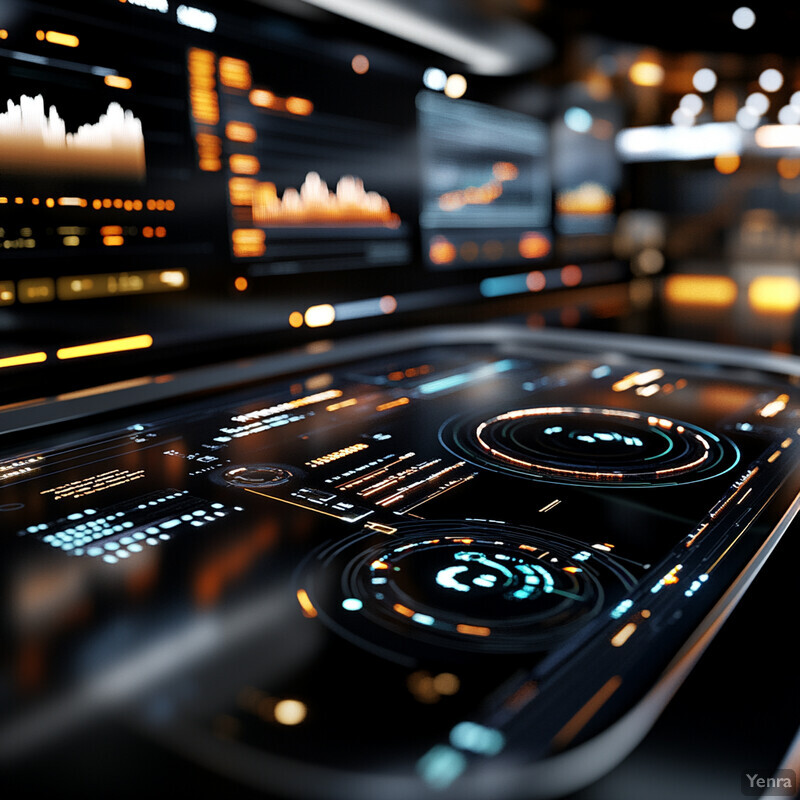
Traditionally, transaction monitoring relied on a set of static rules and thresholds that produced overwhelming numbers of alerts without sufficient context. AI-driven systems, however, analyze massive volumes of transactional data in near real-time and identify complex behaviors that may indicate money laundering. Instead of solely relying on predefined scenarios, these models learn patterns from historical data, continually adjusting to new methods of illicit activities. As a result, compliance teams can detect anomalies faster and more accurately, enabling them to intervene earlier and reduce potential financial and reputational damage.
2. Improved Pattern Recognition
Machine learning models excel at detecting subtle and complex patterns that human analysts might miss, allowing institutions to identify sophisticated laundering techniques.
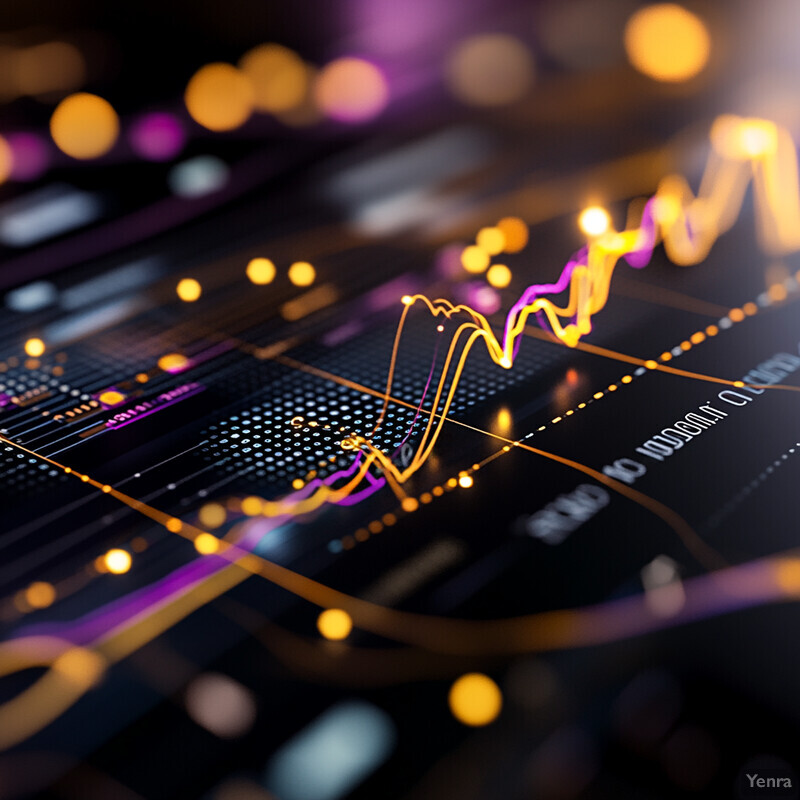
Money launderers are constantly evolving their strategies, which can become incredibly intricate and difficult to identify with traditional analytical tools. AI excels at recognizing subtle patterns and relationships hidden within large datasets, revealing complex layering techniques, unusual transaction flow, and suspicious interconnections among entities. By leveraging machine learning, institutions can keep pace with criminals’ adaptive methodologies, discovering patterns that would be virtually impossible for human analysts to detect unaided. This heightened level of pattern recognition provides a formidable defense against increasingly sophisticated laundering schemes.
3. Enhanced Know Your Customer (KYC) Procedures
AI-driven identity verification tools, including facial recognition and document analysis, streamline onboarding processes and ensure more accurate customer profiling.
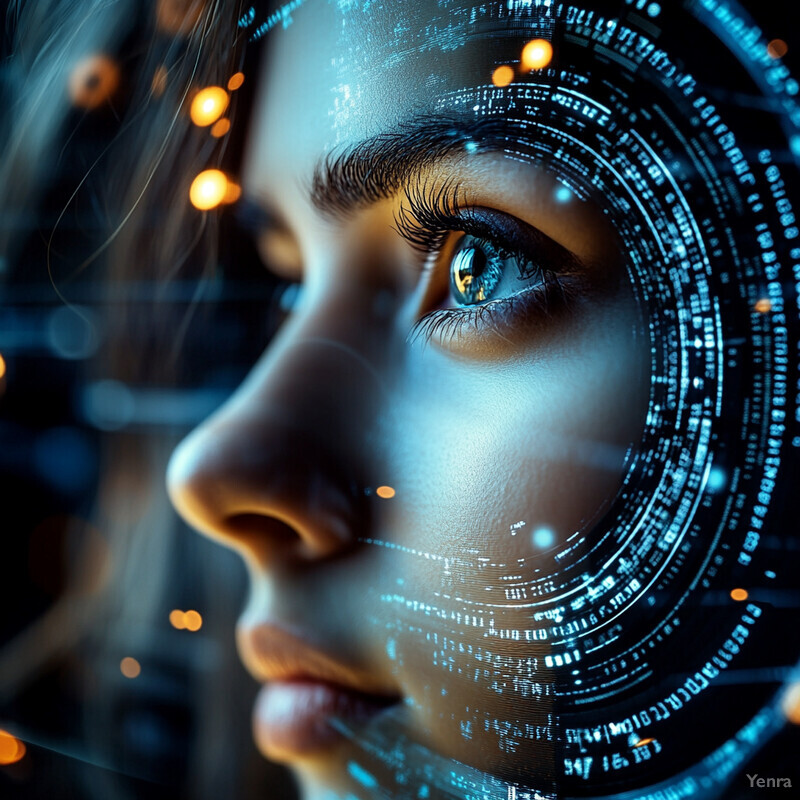
A robust KYC process is foundational in AML compliance, ensuring that financial institutions have a complete and accurate understanding of their customers. AI technologies, such as optical character recognition (OCR), facial recognition, and advanced identity verification algorithms, streamline the onboarding process. They automatically verify identification documents, cross-check multiple data points, and confirm the legitimacy of customer profiles. By doing so, AI reduces manual workload, speeds up account openings, and minimizes the risk of onboarding high-risk or fraudulent customers who could later exploit the financial system for illicit activities.
4. Automated Customer Risk Scoring
AI can continuously update and refine risk scores for customers by analyzing changes in their transactional behavior, jurisdictional exposures, and network associations.
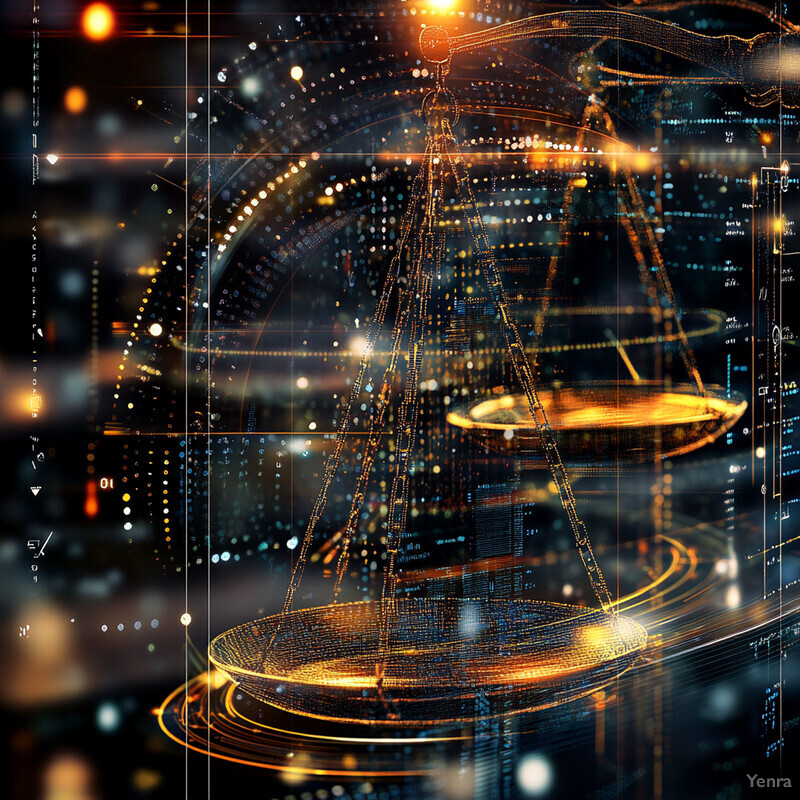
Assigning and managing customer risk scores is critical to prioritizing AML efforts and determining appropriate levels of scrutiny. AI-driven tools dynamically update a customer’s risk score based on evolving factors like transaction velocity, associated counterparties, geographic regions of activity, and even external intelligence signals. Unlike static scoring approaches that must be manually recalibrated, machine learning models continuously learn from new data, ensuring risk assessments remain current and accurate. This adaptability helps institutions focus limited investigative resources on the highest-risk customers and transactions at any given time.
5. Reduced False Positives
By learning from historical data, AI models can distinguish between genuinely suspicious activity and harmless anomalies, dramatically reducing the number of unnecessary alerts.
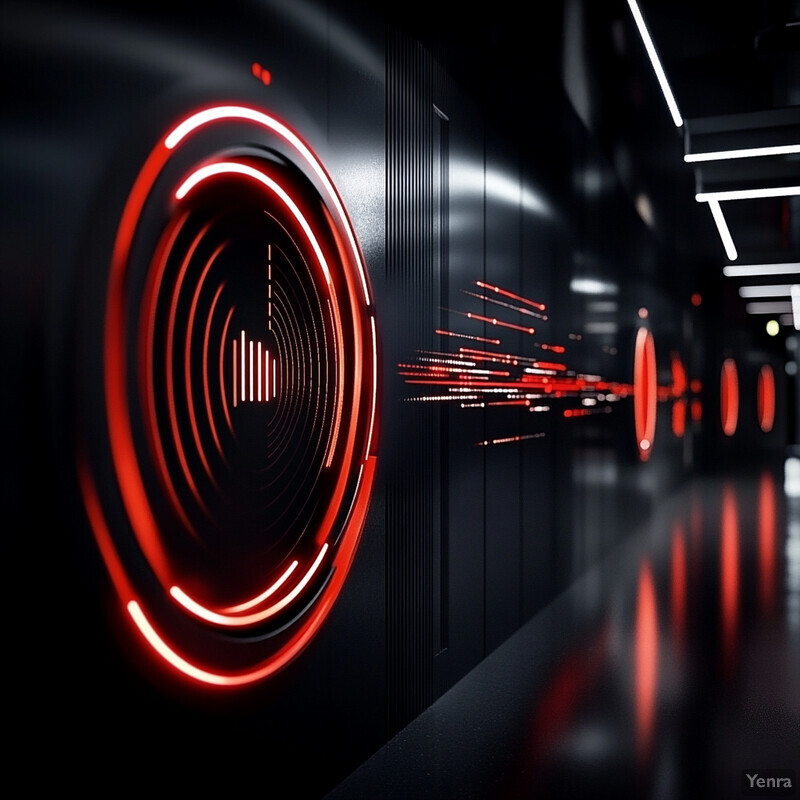
One of the greatest challenges facing AML teams is the sheer volume of alerts generated by rigid rule-based systems, many of which turn out to be false alarms. AI models significantly reduce false positives by distinguishing genuinely suspicious activities from harmless anomalies. These models refine their understanding of normal customer behavior over time, reducing the noise and enabling analysts to concentrate on true red flags. The outcome is a more efficient AML operation, with diminished alert fatigue among compliance staff and lower operational costs in managing unproductive investigations.
6. Real-Time Suspicious Activity Reporting (SAR) Drafting
AI can pre-populate SARs with relevant details from multiple data sources, accelerating the reporting process and ensuring more consistent documentation.
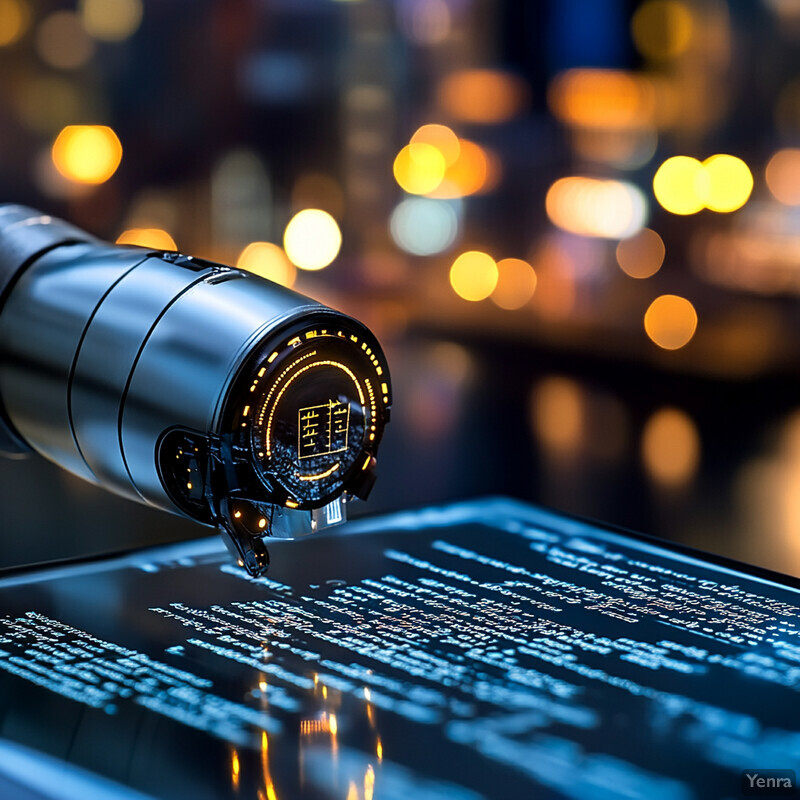
Crafting Suspicious Activity Reports involves gathering details, cross-referencing internal records, and ensuring accuracy and consistency. AI can streamline this process by automatically populating draft reports with relevant transaction details, customer profiles, and potential risk indicators. Natural language generation techniques help ensure that narratives are clear and comprehensive. By reducing the time and complexity involved in SAR preparation, AI technologies enable AML professionals to file timely and high-quality reports, ultimately enhancing regulatory compliance and the effectiveness of the overall AML program.
7. Dynamic Regulatory Rule Updating
AI systems can rapidly incorporate new regulations and typologies, allowing AML teams to stay current with evolving compliance standards and adapt controls accordingly.
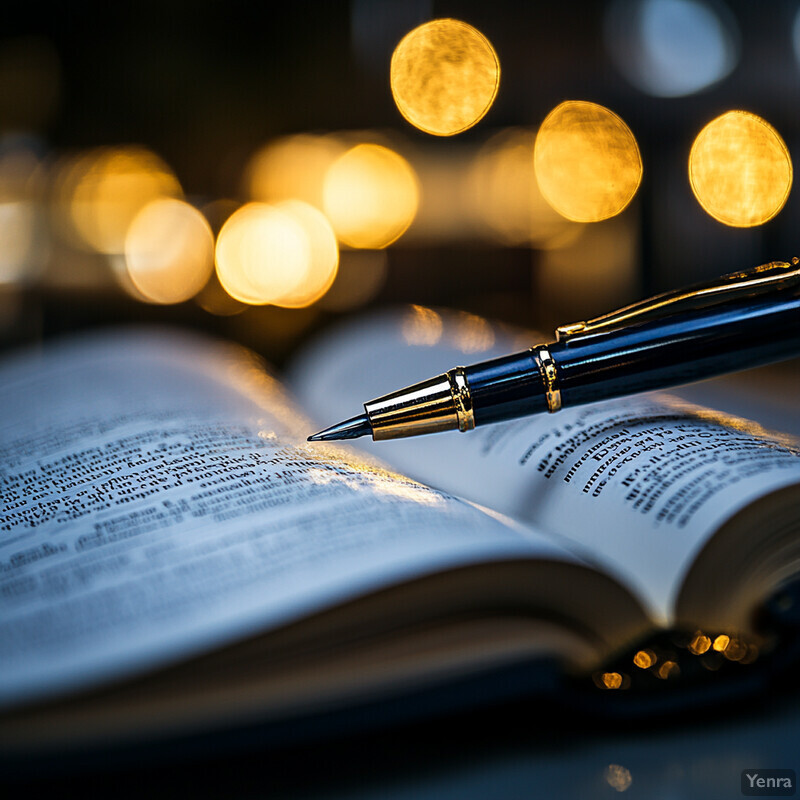
AML regulations and guidelines evolve as money laundering techniques transform and global standards shift. AI can help institutions adapt swiftly to these changes by using machine learning models that learn new typologies as they emerge. Instead of waiting for periodic manual rule reviews, AI systems can incorporate new regulatory directives, suspicious patterns, or red flags immediately into their monitoring frameworks. This agility keeps compliance measures aligned with current requirements, ensuring that financial institutions remain prepared for audits and maintain a proactive stance against evolving criminal strategies.
8. Network Analysis and Link Discovery
Graph analysis and AI-based entity resolution techniques help identify hidden relationships, beneficial ownership structures, and complex laundering networks spanning multiple countries.
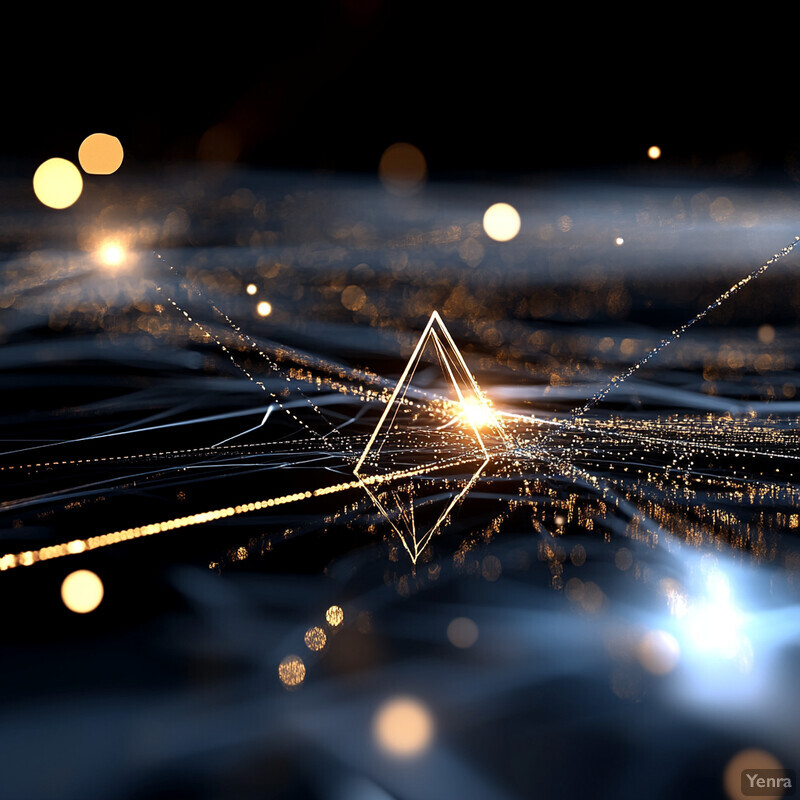
Criminal networks often involve multiple layers of shell companies, straw men, and indirect relationships that are challenging to unravel. AI-powered network analysis uses graph theory and machine learning to identify and visualize relationships between entities, transactions, and accounts. It can detect clusters, central nodes, and patterns of behavior that indicate hidden beneficial owners or complex laundering webs. By shining a light into these opaque structures, AI-driven network analysis allows investigators to pinpoint the ultimate beneficiaries and key collaborators behind illicit activities.
9. Text Analytics and Natural Language Processing (NLP)
NLP allows AML teams to mine unstructured data sources (e.g., news reports, legal documents, and sanctions lists) for insights that inform risk assessments and identify red flags.
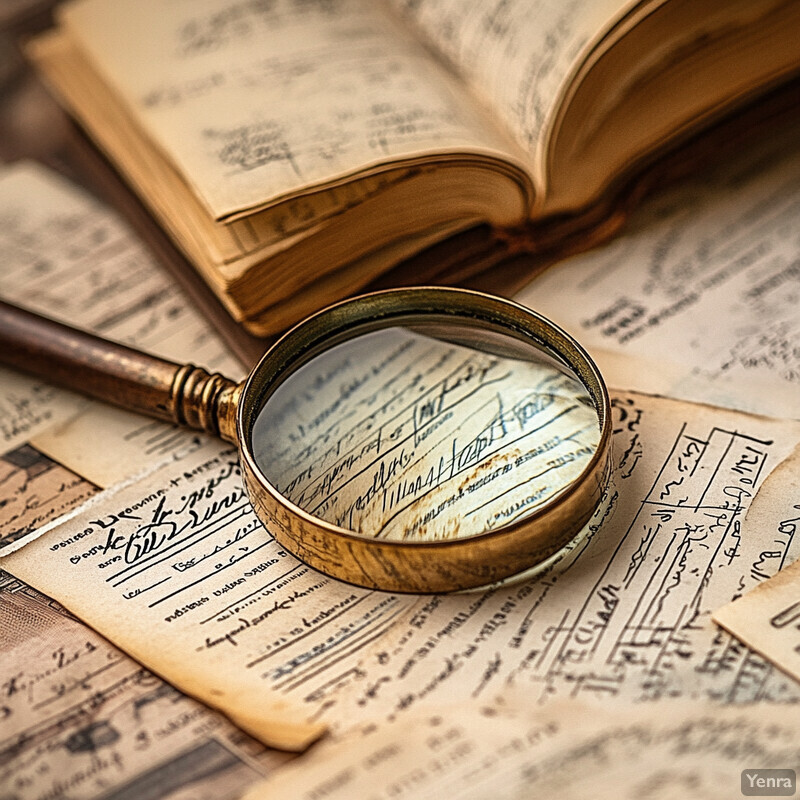
A wealth of AML-relevant information lies in unstructured data, including news articles, legal documents, social media, and regulatory announcements. NLP-powered tools can sift through vast amounts of text-based data, extracting entities, identifying suspicious phrases, and spotting emerging risk narratives. By transforming unstructured content into actionable intelligence, these tools help AML teams identify adverse media mentions, follow global enforcement trends, and factor non-transactional signals into their risk assessments. The result is a more holistic and context-rich approach to detecting and preventing money laundering.
10. Predictive Analytics for Emerging Risks
AI models can anticipate changes in criminal methodologies and highlight emerging risks, allowing proactive adjustments to AML strategies.
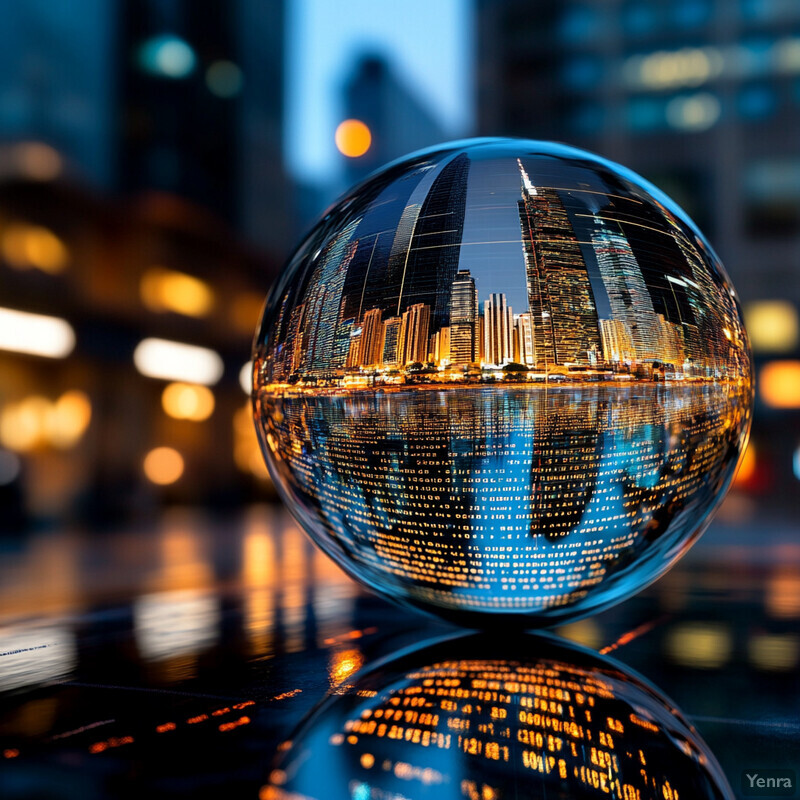
Money laundering techniques do not remain static. Criminals consistently innovate, testing new channels, payment methods, and geographic corridors. AI-based predictive analytics models can forecast these shifts by recognizing precursors to emerging typologies. Leveraging historical transaction data, external threat intelligence, and market trends, these tools predict changes in laundering strategies before they become widespread. Institutions can use these insights to proactively adjust their AML frameworks, invest in new controls, and preemptively address vulnerabilities before they are exploited.
11. Continuous Learning from Feedback Loops
Machine learning models improve over time as they incorporate feedback from investigators and regulators, refining their detection capabilities and reducing error rates.
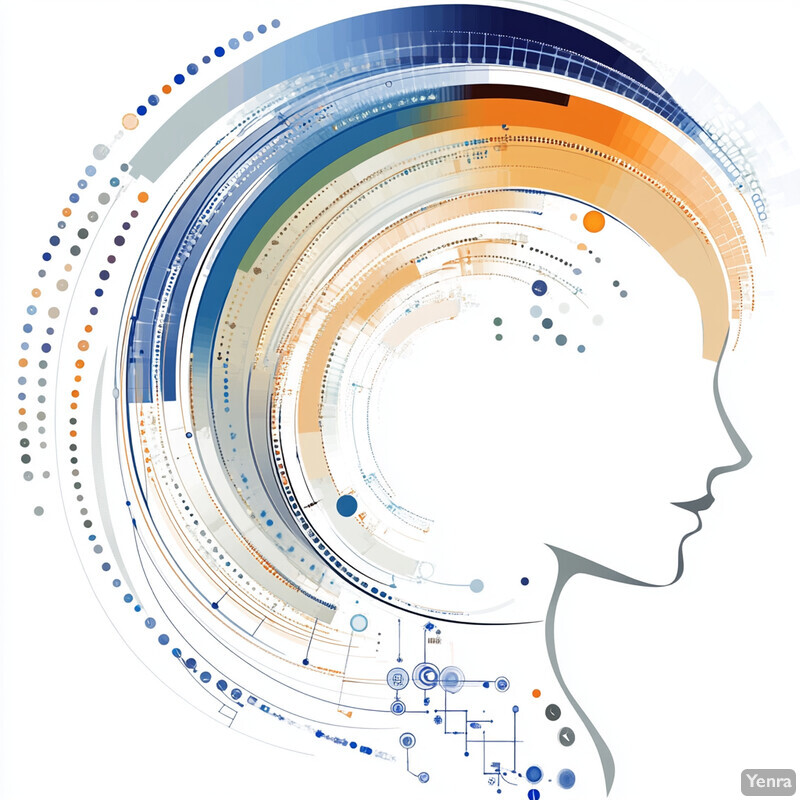
Machine learning models thrive on feedback. As AML investigators review and classify alerts, their decisions form valuable inputs that can refine AI models over time. By integrating these feedback loops, the system grows increasingly adept at discriminating between suspicious and benign activities. This self-improvement process leads to ongoing enhancement in detection accuracy, fewer unnecessary alerts, and a more seamless collaboration between human analysts and automated systems. Over time, the synergy between human expertise and AI-driven insights significantly boosts the AML program’s effectiveness.
12. Automated Watchlist and Sanctions Screening
AI can rapidly scan customer databases against multiple watchlists, sanctions lists, and adverse media, providing faster and more accurate alerts than manual checks.
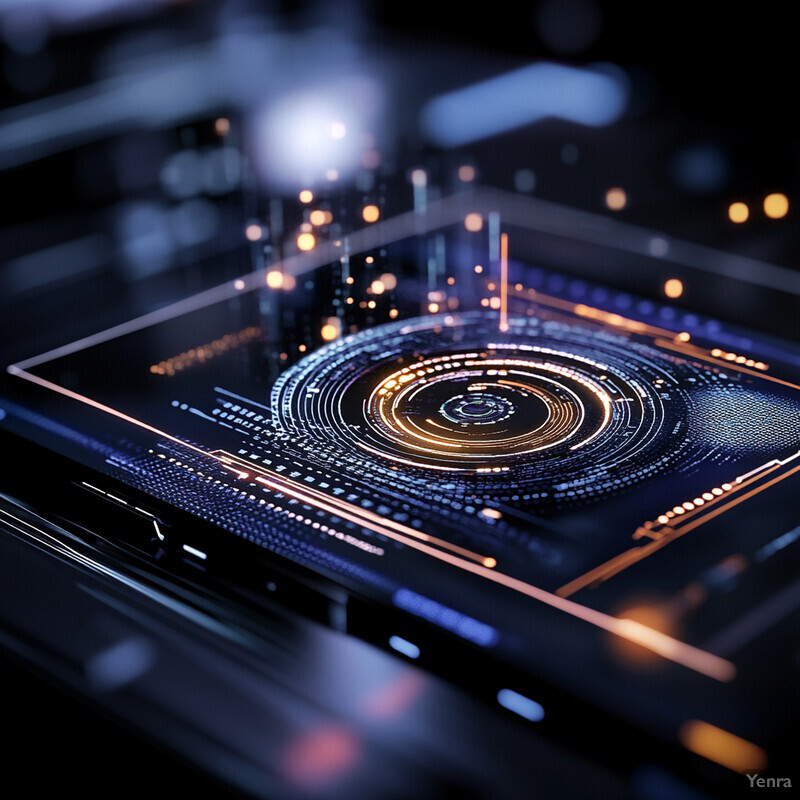
Continuously screening customers and transactions against a myriad of watchlists, sanctions lists, and politically exposed persons (PEP) databases is crucial for AML compliance. AI automates this process, rapidly comparing customer profiles and payment details against these lists. More advanced techniques use fuzzy matching, translation tools, and name-variation detection to reduce missed matches. As a result, institutions can handle higher volumes of screenings with greater accuracy and speed, ensuring they remain in compliance with sanctions regimes and avoid costly penalties.
13. Behavioral Profiling of Customers
AI-driven behavior analytics identify deviations from established customer profiles, highlighting activities that are inconsistent with previous transaction histories.
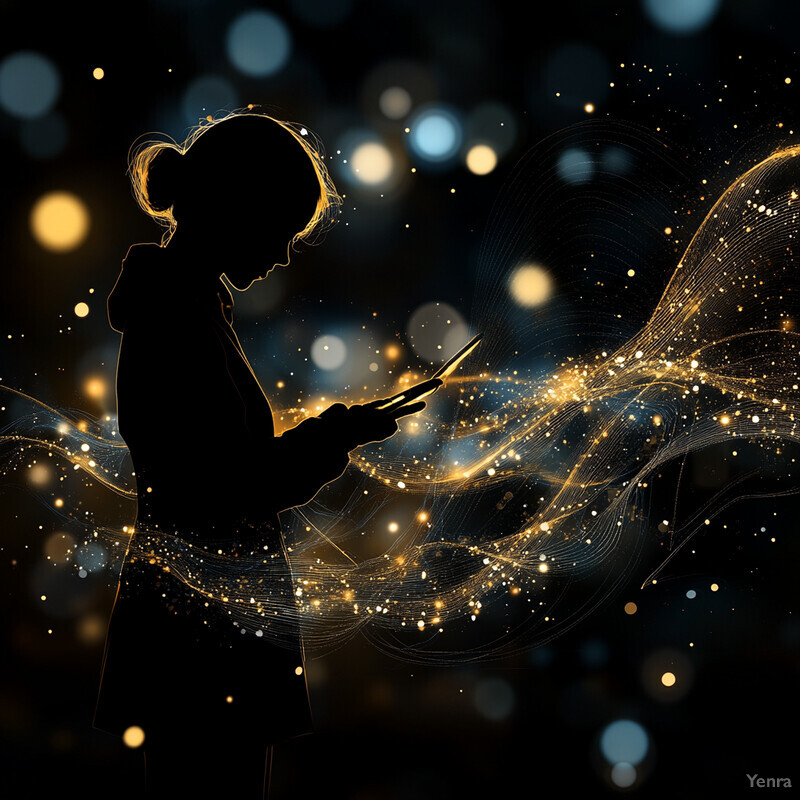
By establishing a baseline understanding of how each customer typically transacts—frequency, amounts, destinations, and counterparties—AI can quickly flag deviations that may indicate illicit behavior. Behavioral profiling goes beyond static attributes like customer type or geographic region and considers evolving behavioral patterns over time. This contextualization helps spot suspicious changes, such as sudden escalations in transaction volume or unusual shifts in transfer destinations. With a deeper understanding of customers’ normal patterns, institutions can more effectively detect anomalies tied to money laundering attempts.
14. Efficient Case Prioritization and Investigation
Intelligent case management tools use AI to rank alerts by severity and potential impact, enabling investigators to focus on the highest-risk cases first.
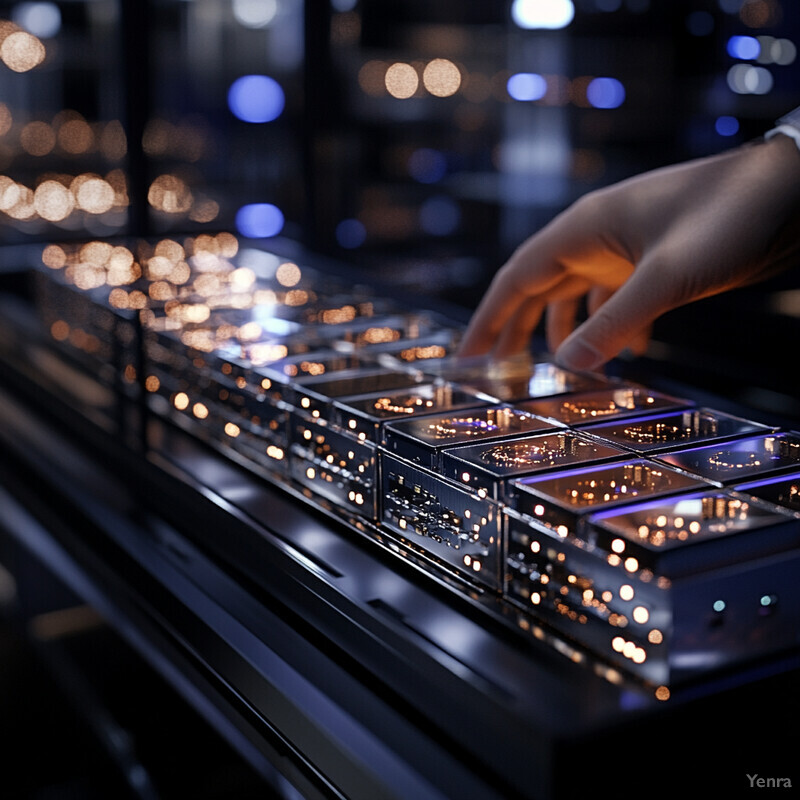
When thousands of alerts flood AML teams each day, prioritization becomes critical. AI-driven case management tools can rank alerts based on factors such as risk scores, potential financial impact, similarity to known suspicious patterns, and links to high-risk jurisdictions. This helps investigators focus on the alerts most likely to lead to significant findings. By reducing time spent on lower-risk cases and organizing investigations efficiently, AML teams can accelerate their decision-making processes, bolster their productivity, and strengthen their overall compliance posture.
15. Cross-Channel Monitoring
AI models integrate data from multiple channels—such as online banking, mobile apps, and branch transactions—offering a holistic view of customer activity.
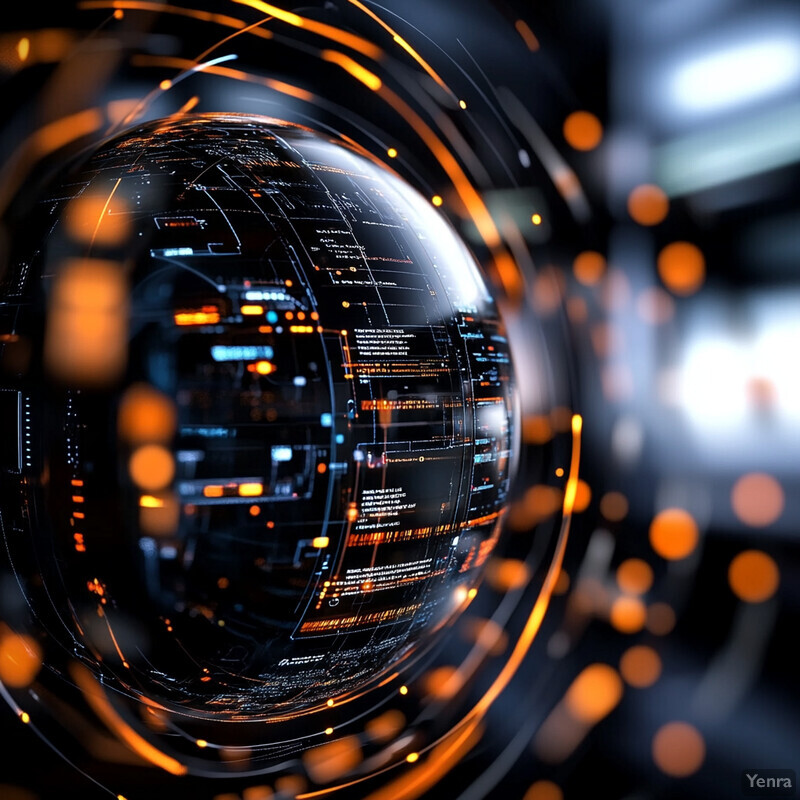
Customers engage with financial institutions through multiple channels—online banking, mobile apps, ATMs, branches, and third-party platforms. Integrating and analyzing data from these sources is challenging but essential for a complete risk picture. AI-powered platforms unify cross-channel data streams to identify suspicious behaviors that might only become evident when viewed collectively. This holistic perspective ensures that institutions are not missing money laundering indicators hidden within isolated channels, thereby providing a comprehensive, 360-degree view of customer activity.
16. Scenario Optimization and Model Calibration
AI can run simulations to test different AML scenarios, adjusting thresholds and detection logic to improve efficiency and accuracy over time.
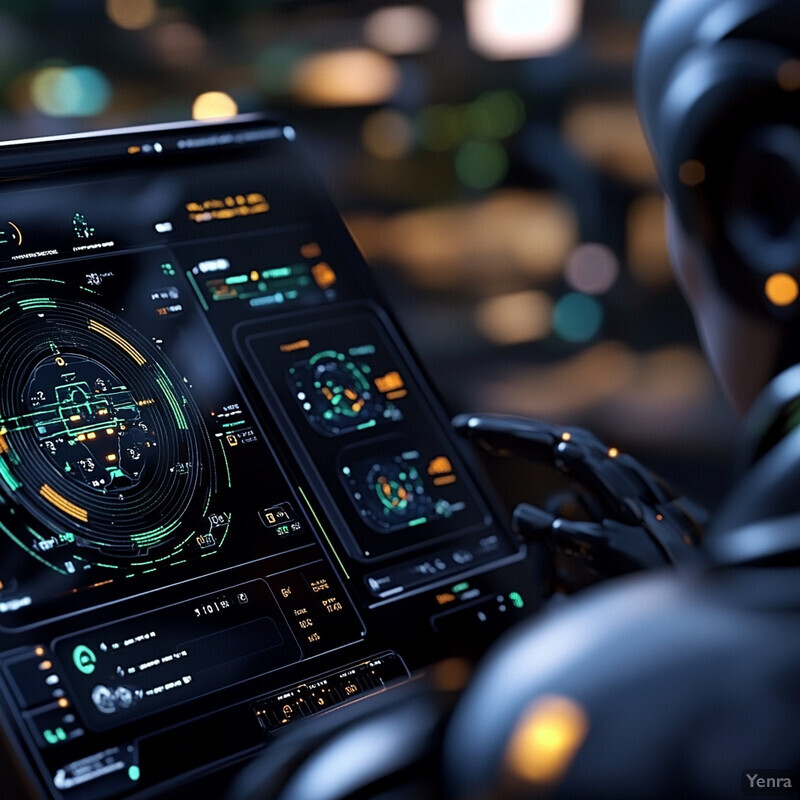
Developing detection scenarios and calibrating thresholds is an ongoing task for AML teams, as conditions and criminal tactics constantly evolve. AI helps optimize these scenarios by testing different configurations, thresholds, and parameters against historical and current data. This simulation-based approach allows compliance officers to identify the most effective rules, reduce false alarms, and enhance true detection rates. The result is a more agile AML platform that can be fine-tuned continuously to align with both regulatory expectations and an evolving threat landscape.
17. Early Detection of Complex Laundering Schemes
By recognizing complex transaction structuring, layering, and integration techniques, AI helps uncover illicit schemes earlier in their lifecycle.
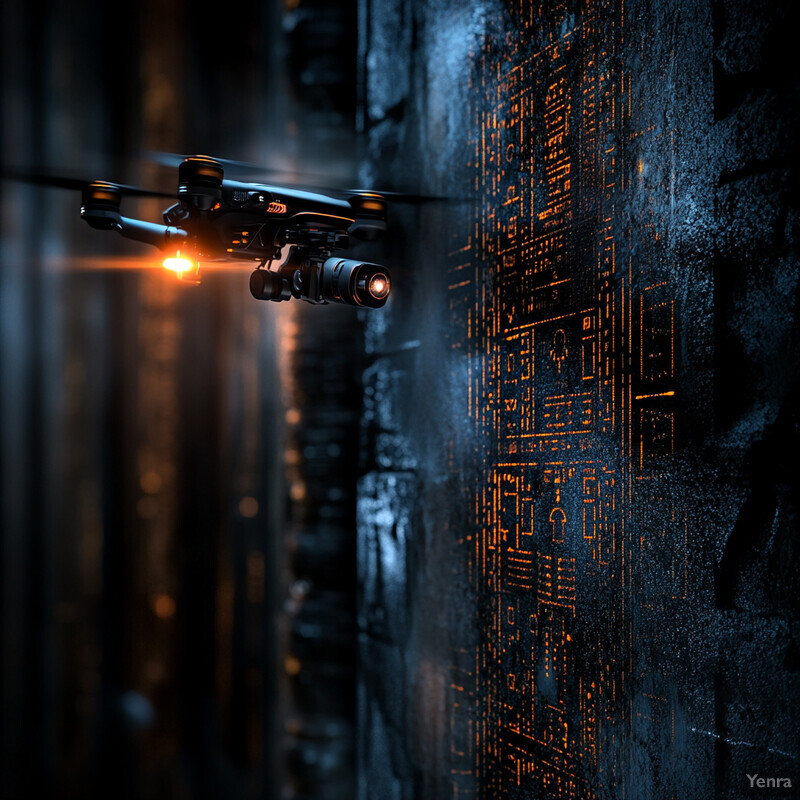
Complex laundering often involves intricate layering—transferring funds through a series of intermediaries, currencies, and jurisdictions. AI’s ability to process large datasets quickly and apply advanced analytics makes it possible to catch these schemes in earlier stages, rather than discovering them after substantial laundering has occurred. Early detection not only limits financial losses and reputational damage but also improves the likelihood of recovering illicit funds and supporting effective law enforcement actions.
18. Support for Regulatory Compliance Reviews
During audits and examinations, AI tools can quickly extract and organize relevant information, making it easier for financial institutions to demonstrate compliance.
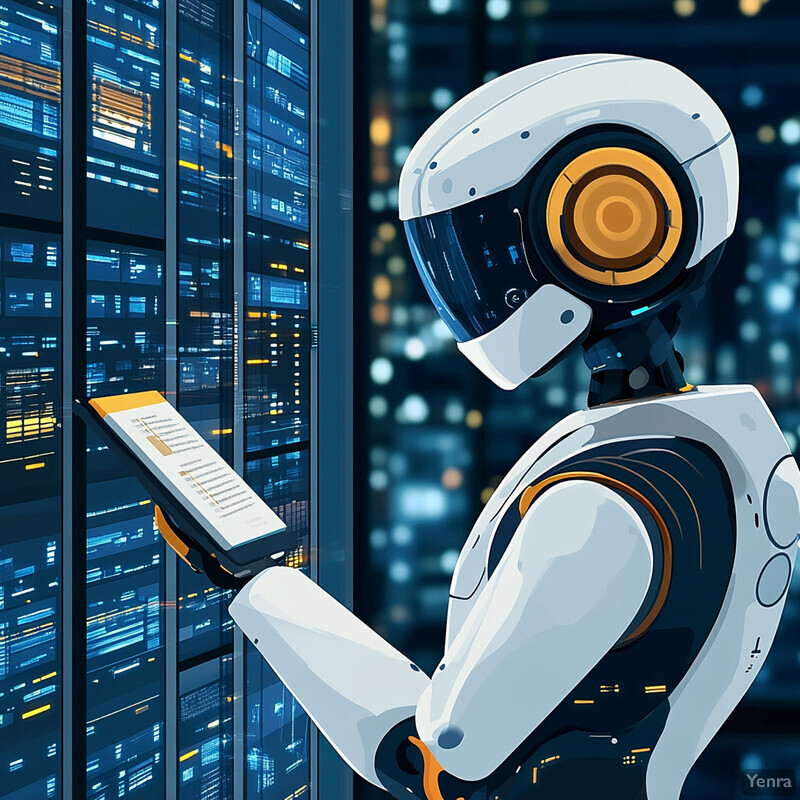
Regulatory examinations and audits demand quick and accurate responses to inquiries. AI tools can rapidly extract, categorize, and summarize vast quantities of transactional and KYC data, as well as records of alerts and investigations. This speed and accuracy simplify the audit process, helping financial institutions demonstrate the robustness of their AML frameworks and processes. By maintaining organized, easily accessible records, institutions can respond efficiently to regulator queries, minimize disruption, and build trust with supervisory bodies.
19. Improved Data Quality Management
AI-powered data validation and cleansing ensure that AML systems rely on accurate, consistent information, enhancing the reliability of detection models.
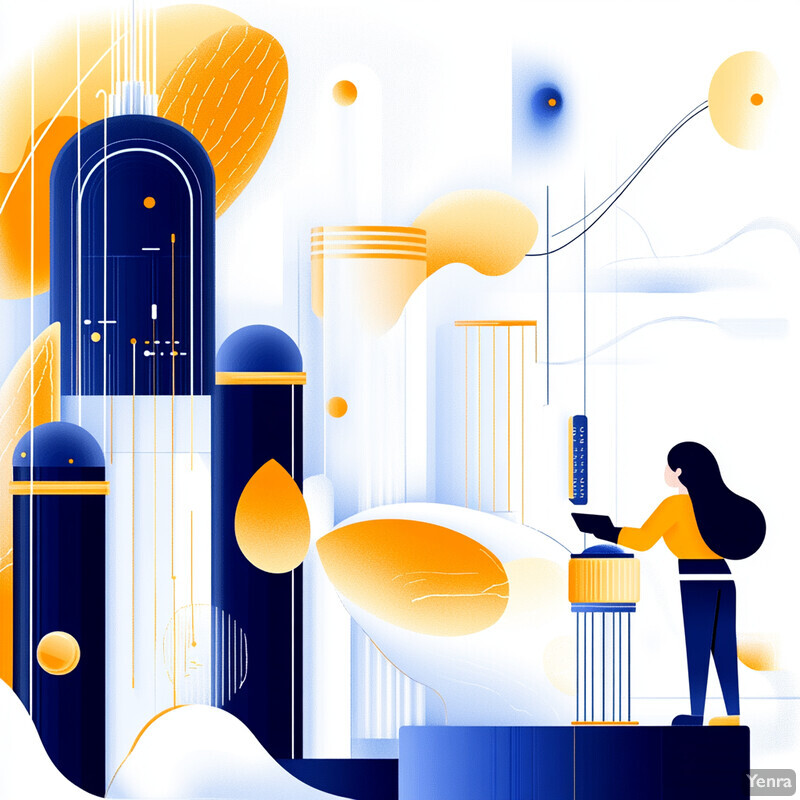
AML compliance depends on reliable, high-quality data. Erroneous or incomplete customer records, inconsistent transaction details, and fragmented data sources hamper effective monitoring and investigation. AI-powered data validation and cleansing tools help standardize and enrich data, identify missing fields, correct typographical errors, and merge duplicate records. By maintaining accurate and consistent datasets, institutions improve the reliability of detection models, reduce false positives, and enhance the overall efficiency of their AML systems.
20. Scalability and Adaptability
As transaction volumes grow and criminals adopt new laundering techniques, AI systems can scale and adapt more readily than traditional rule-based systems, ensuring ongoing effectiveness.
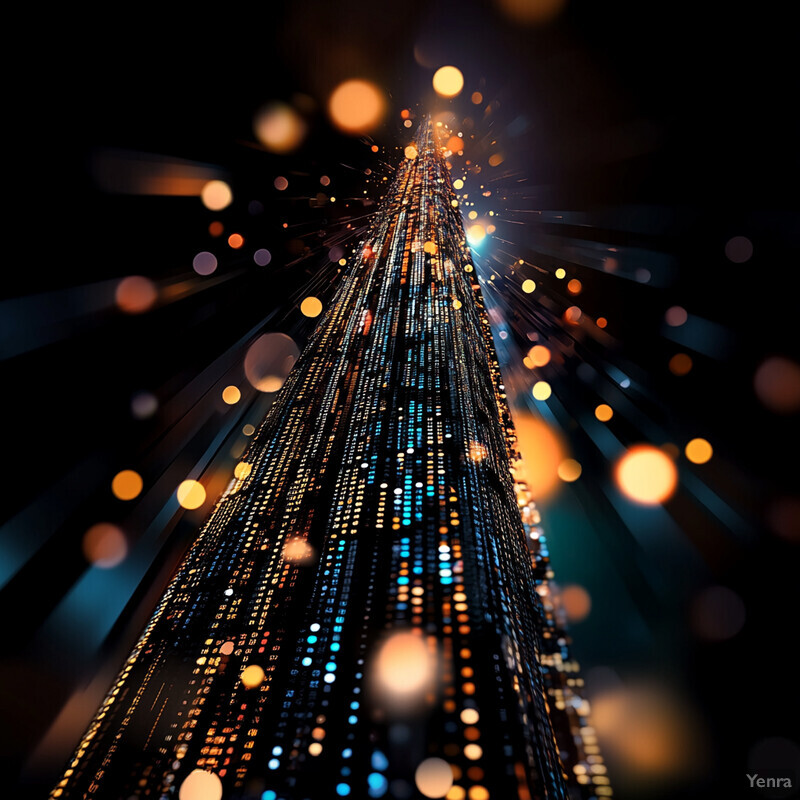
As financial institutions grow and transaction volumes surge, scaling traditional AML operations often proves difficult. AI-driven solutions, hosted on cloud platforms or advanced data infrastructures, can easily scale to handle more data, more customers, and more jurisdictions. Moreover, they can adapt to new trends, criminal tactics, and regulatory requirements as they arise. This scalability and adaptability ensure that AML compliance frameworks remain effective even under increasing transaction loads and the continuous evolution of global money laundering threats.