1. Predictive Modeling for Risk Stratification
AI-driven algorithms analyze large sets of patient data, family history, and environmental factors to predict an individual’s likelihood of developing specific allergies, enabling early preventive strategies.
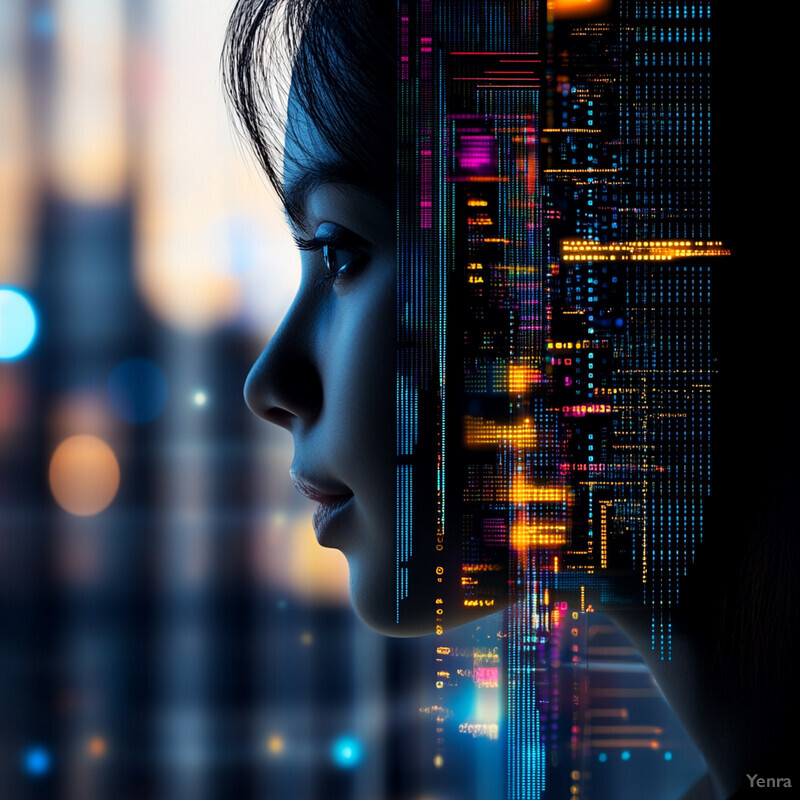
AI-driven predictive modeling involves analyzing extensive datasets encompassing genetic predispositions, family medical histories, dietary habits, environmental exposures, and personal health indicators to estimate an individual’s risk of developing specific allergies. Machine learning algorithms can sift through massive amounts of patient data to identify patterns and key risk factors that a clinician might otherwise miss. This enables proactive interventions, such as recommending early allergy testing, lifestyle modifications, or preemptive immunotherapy to reduce the likelihood or severity of allergen-related diseases. As a result, clinicians and patients can work together to establish preventative strategies well before debilitating symptoms emerge.
2. Real-Time Environmental Allergen Tracking
Machine learning models integrate pollen counts, pollution levels, weather patterns, and seasonal trends from multiple data sources to provide personalized, moment-to-moment allergy risk assessments.
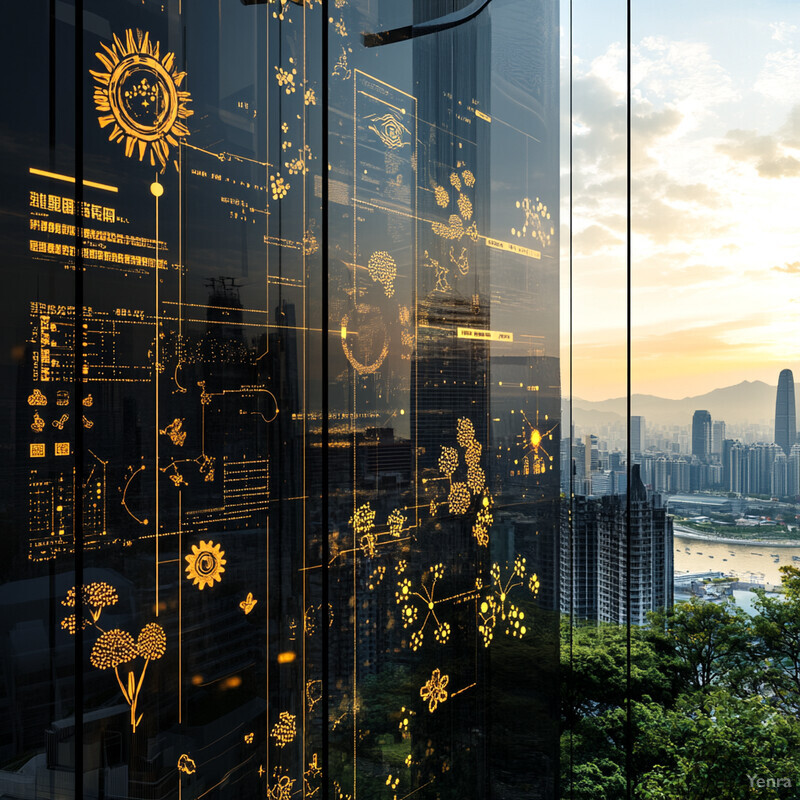
Allergen levels, such as pollen counts, pollution indices, and mold spores, can fluctuate daily or even hourly. AI models integrate various data streams—satellite imagery, weather forecasts, air quality sensors, and historical allergen concentration records—to deliver constantly updated, localized information on potential exposures. Patients gain the ability to access these insights through mobile apps or online platforms, receiving timely alerts and recommendations. This empowers them to take protective measures, like wearing masks, staying indoors at peak allergen times, or adjusting medication use accordingly. The granular real-time guidance significantly improves symptom control and quality of life for those sensitive to environmental triggers.
3. Personalized Allergen Profiles
By examining genetic, immunologic, and lifestyle factors, AI can help create detailed allergen sensitivity profiles unique to each patient, streamlining diagnosis and guiding personalized treatment.
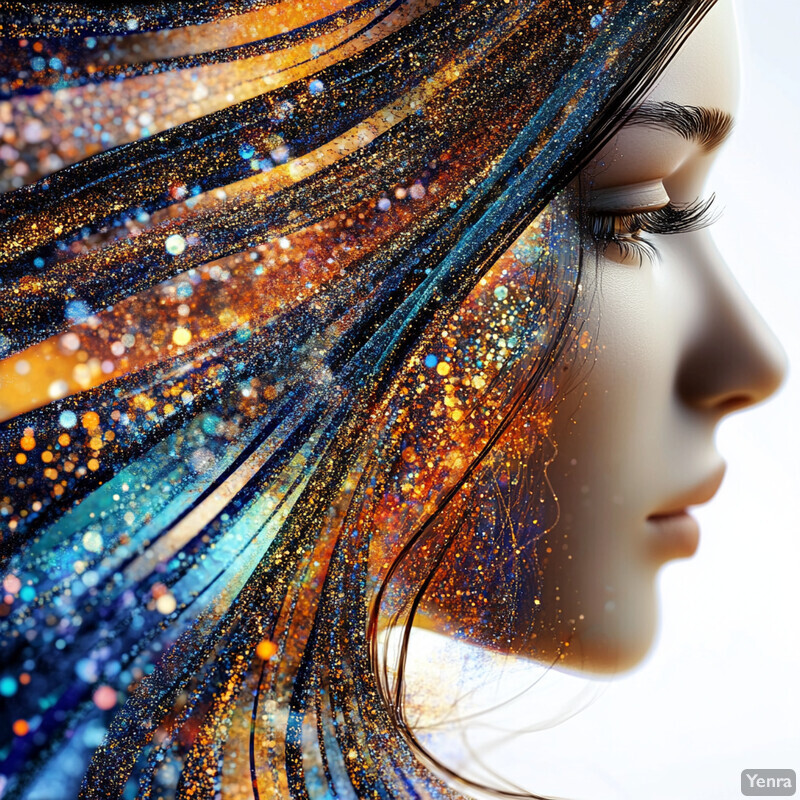
Allergies vary widely among individuals, influenced by unique genetic, immunologic, and lifestyle factors. Advanced AI systems can consolidate a patient’s genetic testing results, immune response biomarkers, dietary patterns, and environmental exposures into a comprehensive allergen profile. This profile goes beyond simple diagnostic labels, pinpointing the precise allergens—and their relative severity—that impact each patient’s health. Clinicians can then tailor treatment plans, recommending targeted immunotherapy, precision nutrition, and specific avoidance strategies. Over time, these personalized profiles can be updated with new data, ensuring that allergy management remains dynamic, accurate, and highly individualized.
4. Machine Learning-Assisted Diagnostic Tools
AI models can augment traditional skin-prick and blood tests by identifying subtle patterns and improving diagnostic accuracy, reducing the number of false positives or false negatives.
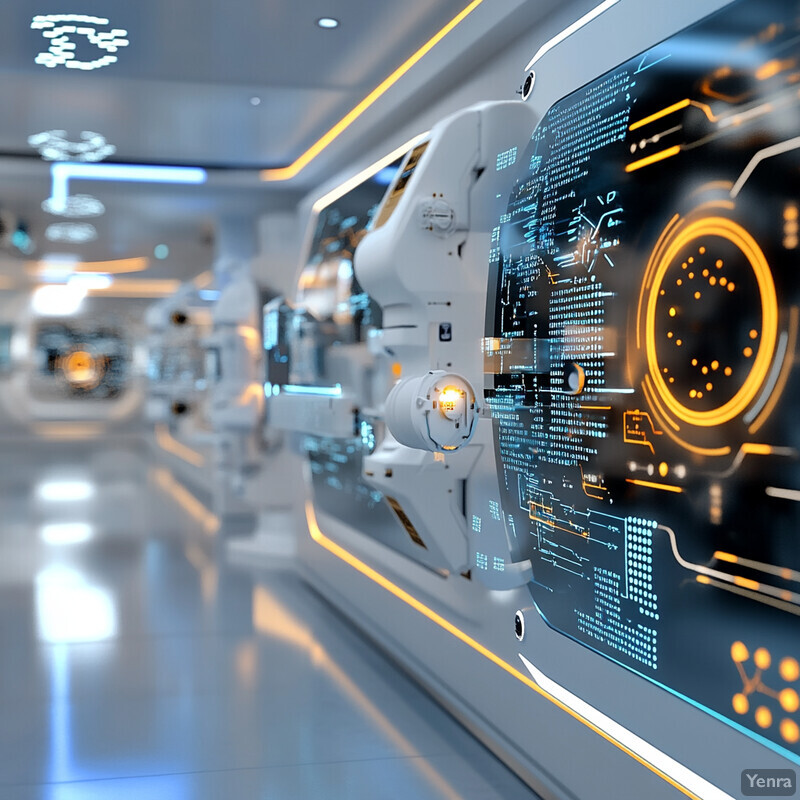
Traditional allergy diagnostics, such as skin-prick tests and blood IgE assays, have limitations, including occasional false positives or negatives. AI-enhanced diagnostic tools leverage pattern recognition to analyze test results more accurately, considering subtle variations in immune response markers or even micro-arrayed allergen panels. These tools compare a patient’s results against large, curated databases of known allergen responses to refine diagnoses. Clinicians receive evidence-based suggestions and confidence scores to guide interpretation, ultimately reducing diagnostic uncertainty. The outcome is a more reliable understanding of a patient’s allergen sensitivities, improving the selection and effectiveness of subsequent interventions.
5. Rapid Detection of Food Allergens in Products
Computer vision and AI-enhanced sensors can quickly identify hidden allergens in packaged foods or cross-contaminated areas, helping patients make informed choices and minimize exposure.
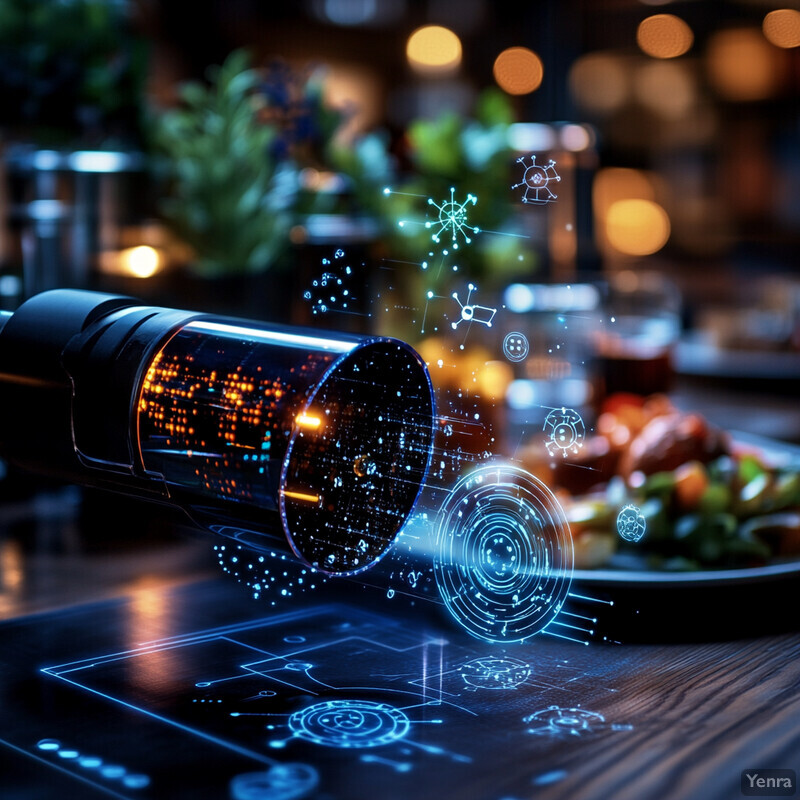
In food allergy management, avoiding hidden allergens is critical. AI-driven computer vision and advanced spectroscopy can be integrated into handheld scanners or smartphone attachments to rapidly identify the presence of allergenic proteins in packaged foods, restaurant dishes, or industrial settings. By analyzing molecular signatures, AI can flag potential contaminants or cross-contact events that standard labeling or human inspection might overlook. This technology empowers consumers to make informed dietary choices, reduces the incidence of accidental allergen exposure, and supports regulatory bodies and manufacturers in maintaining safer food supply chains.
6. Guidance for Allergen Immunotherapy
AI-powered decision-support systems analyze patient responses over time to refine allergen immunotherapy protocols, personalizing dosage schedules for maximum effectiveness and reduced side effects.
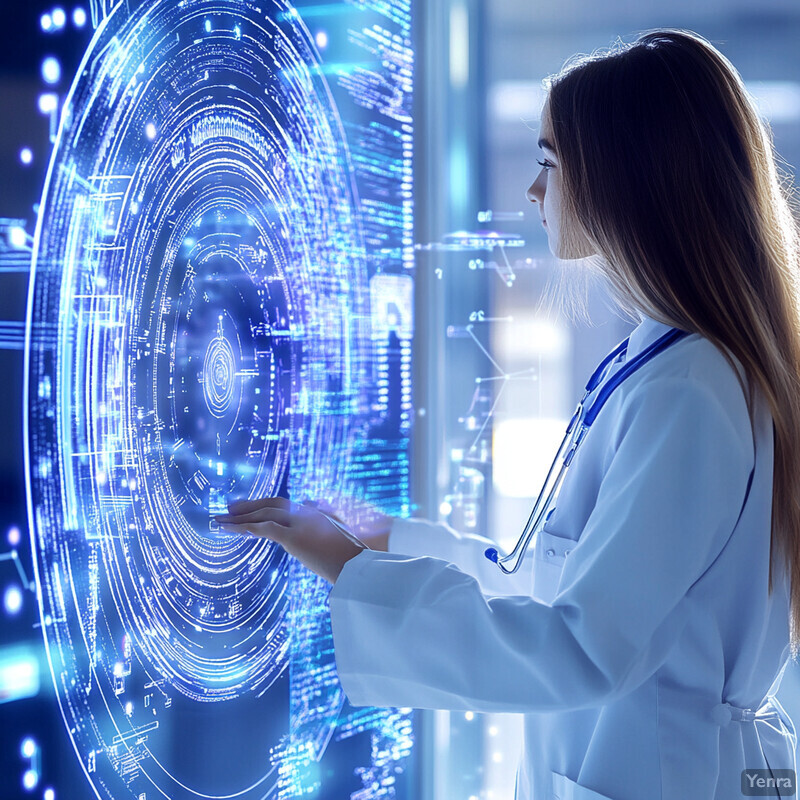
Allergen immunotherapy aims to gradually desensitize patients to specific allergens, but determining the optimal dosage and schedule can be challenging. AI systems can analyze a patient’s treatment history, symptom patterns, immunologic responses, and adherence to therapy to guide adjustments to their immunotherapy regimen. By continuously learning from these data points, the AI can recommend incremental changes—such as adjusting the dose or frequency of allergen exposure—to optimize efficacy and minimize adverse reactions. This personalized, data-driven approach helps ensure that patients receive immunotherapy protocols tailored to their unique biology and tolerance levels, improving long-term outcomes.
7. Advanced Symptom Prediction and Warning Systems
By continuously analyzing wearable sensor data (e.g., heart rate variability, respiratory rate, skin conductance), AI can alert patients to impending allergic episodes before symptoms manifest.
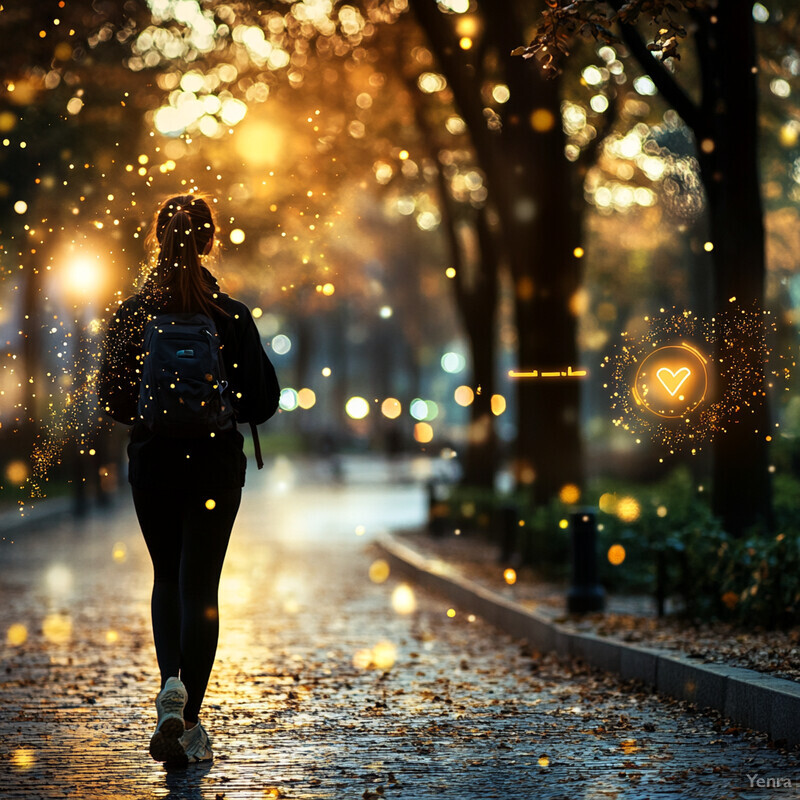
Wearable health devices, such as smartwatches or biometric sensors, generate streams of data related to heart rate, respiratory patterns, temperature, and skin conductance. AI algorithms can correlate these physiological markers with historical symptom logs and environmental allergen fluctuations. By detecting subtle deviations that precede an allergic event, the system can alert patients in advance, giving them time to take preventative medications or remove themselves from the triggering environment. This proactive warning system significantly reduces the severity of allergic reactions and improves patient confidence in managing their conditions.
8. Drug Allergy Identification
AI-driven natural language processing (NLP) can review electronic health records, patient notes, and adverse event reports to identify patterns indicative of drug allergies and prevent potentially dangerous prescriptions.
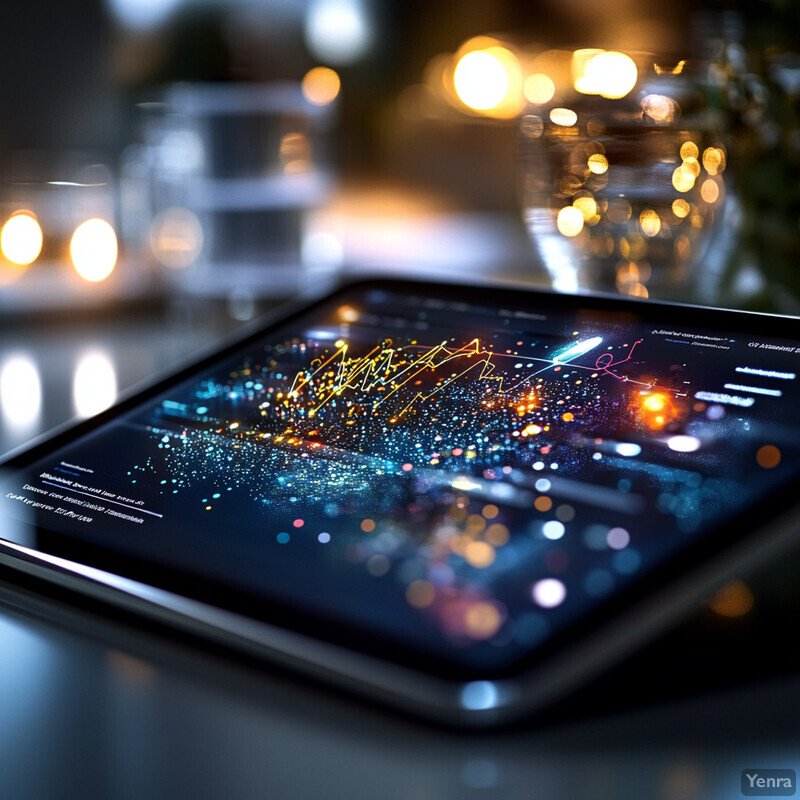
Many patients have drug sensitivities that remain undetected until a severe allergic reaction occurs. AI-driven natural language processing (NLP) can scan electronic health records, clinical notes, and adverse event reports to discover patterns that suggest drug allergies or intolerances. By connecting data points—such as a patient’s previous reactions, comorbidities, and concurrent medications—these tools highlight potential risks. Clinicians gain critical insights before prescribing, enabling them to select safer pharmacological alternatives. Over time, as more data accumulates, these AI systems become increasingly adept at predicting drug allergies, thereby enhancing patient safety.
9. Allergen Avoidance Recommendations
Recommendation engines can synthesize local air quality data, daily routines, and known allergen sensitivities to suggest behavioral changes—like adjusting workout locations or using a specific air purifier—to minimize exposure.
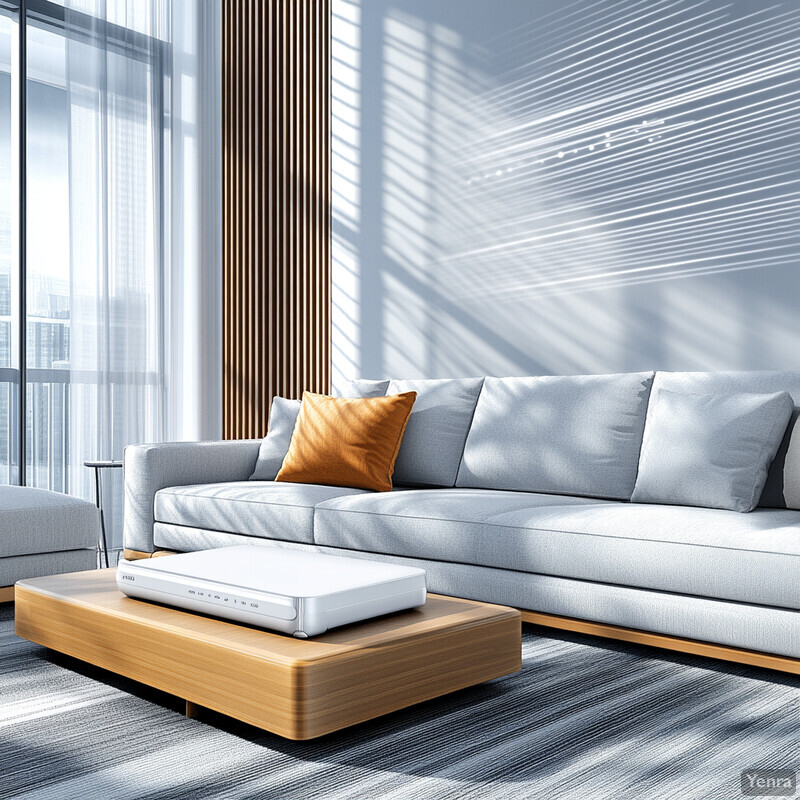
For effective allergy management, patients must often make adjustments in their daily routines—altering exercise times, selecting safer travel routes, or investing in specific air filtration systems. AI recommendation engines integrate each patient’s allergen sensitivities with real-time environmental data and their personal behavior patterns to propose actionable avoidance strategies. By anticipating times of high pollen release or identifying indoor environments with problematic mold growth, these systems help patients optimize their habits and surroundings. Such personalized guidance reduces exposure risk, stabilizes symptoms, and improves overall quality of life.
10. Virtual Allergen Coaches and Chatbots
Conversational AI systems can provide personalized support by answering patient questions, offering educational materials, and guiding them through immediate management steps during allergic flare-ups.
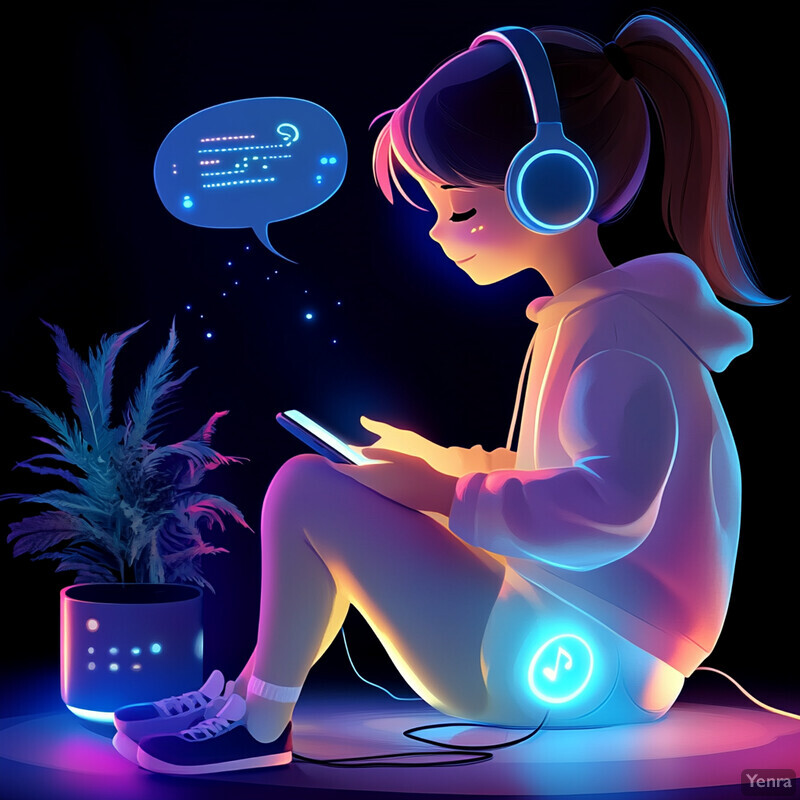
Virtual allergen coaches, often implemented as AI-powered chatbots, serve as accessible resources for patients seeking immediate guidance. These systems can answer questions about symptom triggers, provide step-by-step instructions for using inhalers or EpiPens, and offer educational materials on effective lifestyle changes. They can also store individual patient data to tailor advice, and connect patients with healthcare professionals when more complex interventions are needed. The convenience and round-the-clock availability of virtual coaches enhance patient empowerment, ensuring that managing allergies becomes a more continuous and supportive process.
11. Predicting Cross-Reactivity Between Allergens
Machine learning techniques can discover molecular similarities between different allergens, helping clinicians anticipate cross-reactivities (e.g., between certain pollens and foods) and advise patients accordingly.
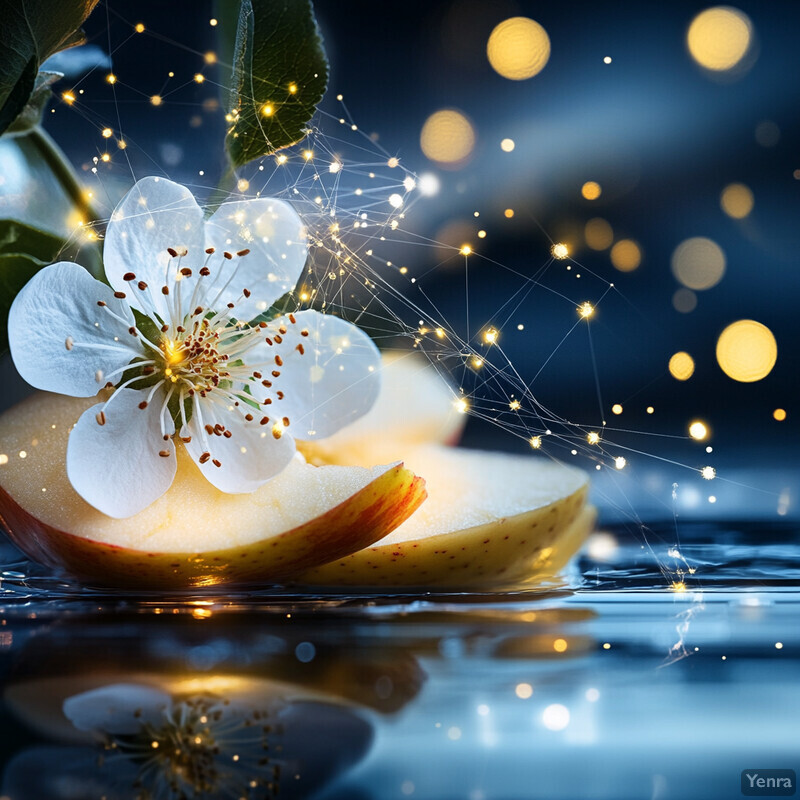
Certain allergens share molecular structures, leading to unexpected cross-reactions—where someone allergic to birch pollen might also react to apples. AI-driven bioinformatics tools can analyze protein databases and immune response profiles to detect patterns of structural similarity. By predicting which allergens are likely to elicit cross-reactive responses, clinicians can better advise patients about foods, environmental triggers, or substances to avoid. This knowledge helps prevent baffling allergic episodes and informs the design of more precise diagnostic panels and therapies.
12. Integration of Genomic and Proteomic Data
Advanced AI methods help identify genetic and protein-level biomarkers associated with allergy risks, leading to new diagnostic criteria and potential therapeutic targets.
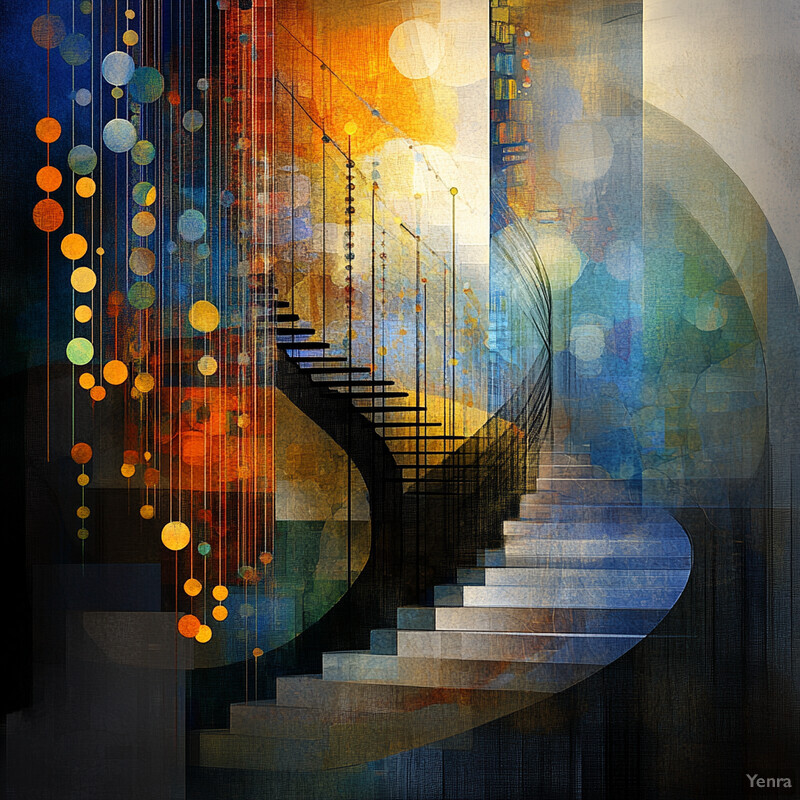
As genomic and proteomic technologies advance, the vast amount of biological data they produce can overwhelm traditional analytical methods. AI excels at correlating genomic variants or proteomic signatures with allergic disease phenotypes. By identifying genetic markers or protein expression patterns strongly associated with specific allergies, researchers can discover novel diagnostic biomarkers or therapeutic targets. This knowledge enables the development of precision therapies that modulate immune responses at their biological roots, potentially improving treatment effectiveness and patient outcomes.
13. Enhanced Biostatistical Analysis of Clinical Trials
AI tools can streamline the design and analysis of allergy-related clinical trials, identifying which subpopulations benefit most from new treatments and optimizing therapy outcomes.
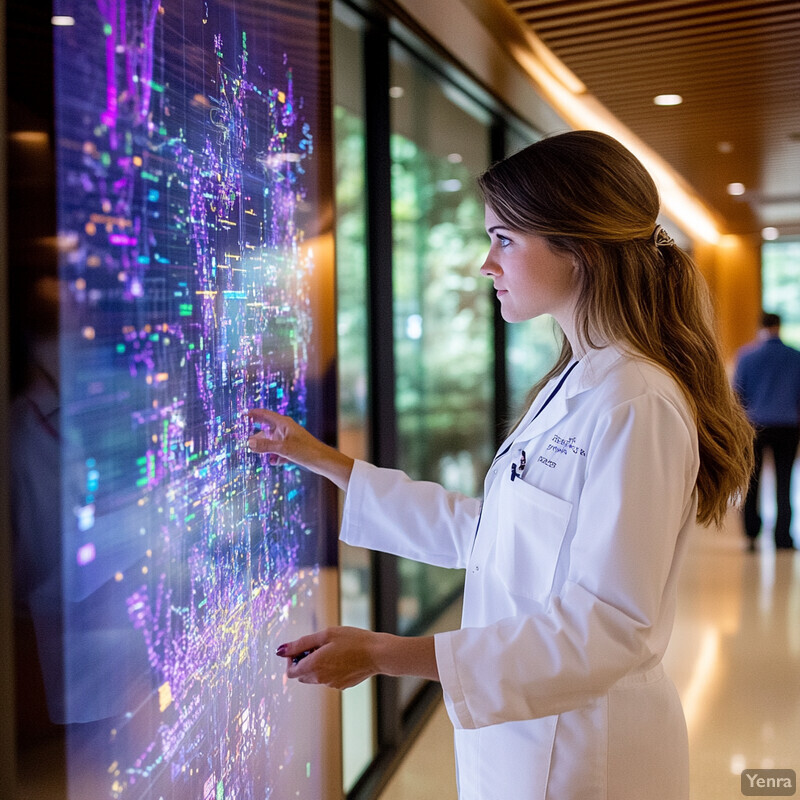
Clinical trials for allergy treatments generate complex datasets, encompassing demographic information, biomarkers, patient-reported outcomes, and long-term follow-up data. AI-based analytical methods can untangle these complexities, identifying which subgroups of patients respond best to new interventions, determining the most relevant endpoints, and suggesting ways to optimize trial design. By providing robust statistical insights, AI facilitates the development of safer, more effective allergy therapies, accelerates regulatory approvals, and ensures that promising treatments reach the patients who need them most.
14. Precision Medicine for Pediatric Allergies
Children’s immune systems evolve rapidly, and AI-based models can track changes over time to forecast which children outgrow allergies and which need longer-term management.
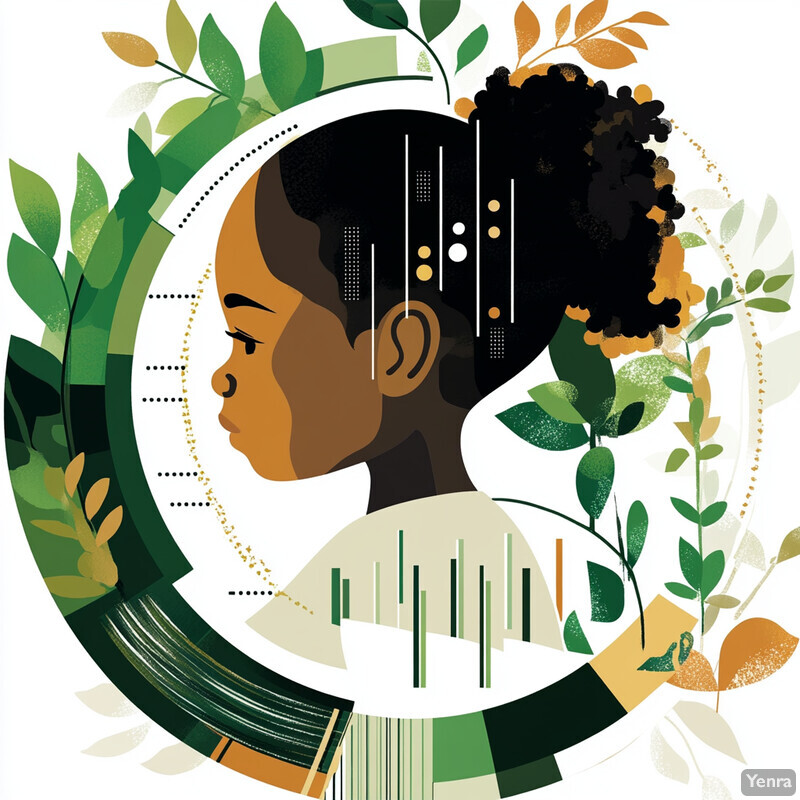
Children’s immune systems are dynamic and evolve as they grow. AI models can track these changes over time, identifying patterns that suggest whether a child is likely to outgrow certain allergies or if more intensive management is required. By analyzing growth rates, dietary exposures, early-life environmental factors, and family history, AI can also predict which preventive measures—such as early introduction of certain foods—are beneficial. With these insights, pediatricians and parents can implement personalized strategies that guide a child’s immune development toward tolerance rather than hypersensitivity.
15. Continuous Quality Improvement in Clinics
AI-driven feedback systems can identify patterns in clinical practice, helping allergists refine diagnostic criteria and tailor interventions, ensuring consistent, high-quality patient care.
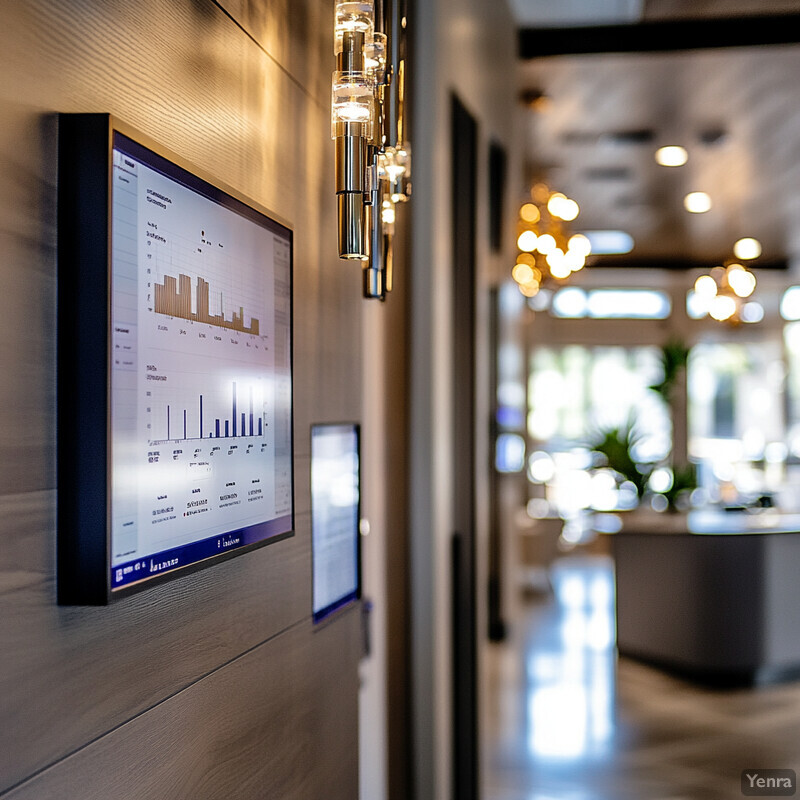
Allergy clinics can integrate AI-driven analytics into their workflows, examining patient outcomes, diagnostic accuracy, and treatment efficacy across multiple practitioners and time frames. By identifying inconsistencies, knowledge gaps, or underutilized therapies, AI helps clinics refine their clinical protocols. This results in more standardized practices, improved patient satisfaction, and better health outcomes. Over time, the continuous loop of data collection, analysis, and feedback fosters a culture of evidence-based improvement, ensuring that allergy care remains at the cutting edge.
16. Allergy Management Apps with Predictive Features
Smartphone apps integrated with AI can predict high-risk days for exposure, track medication use and symptom severity, and coach patients through preventive measures.
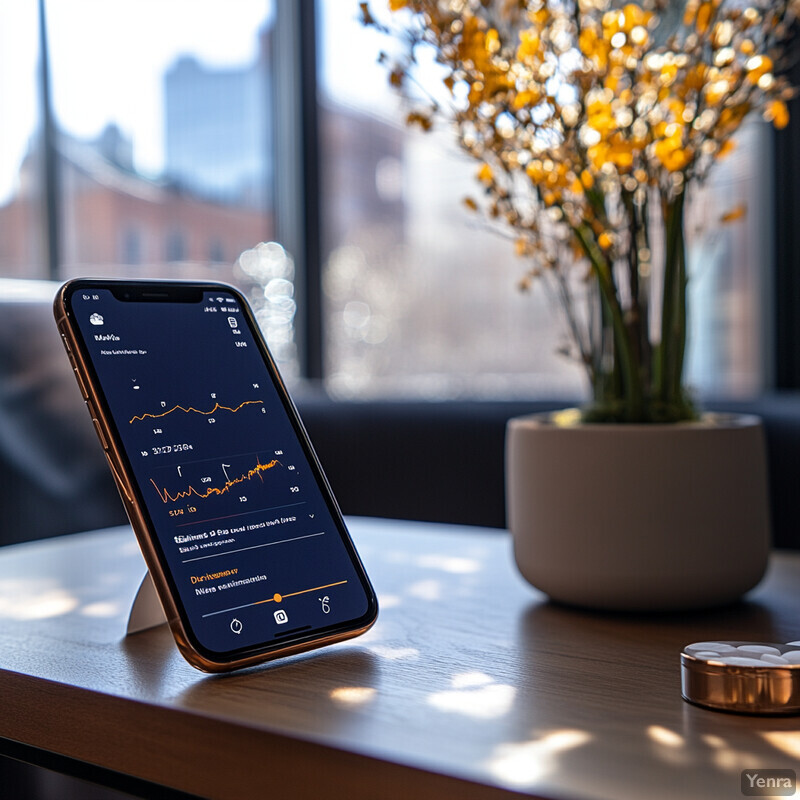
Smartphone apps that incorporate AI can serve as personal health assistants for allergy sufferers. These apps might track medication adherence, symptom severity, and daily activities, integrating the data with external factors like weather forecasts or localized allergen counts. By leveraging machine learning, they predict high-risk days or suggest preventative steps, such as taking antihistamines earlier than usual. Users can receive dynamic, personalized recommendations directly at their fingertips, encouraging better symptom control and improved long-term management adherence.
17. Remote Patient Monitoring and Intervention
Internet of Things (IoT) devices paired with AI algorithms can continuously monitor air quality inside homes and deliver automatic adjustments, like activating air filtration systems, to maintain allergen-safe indoor environments.
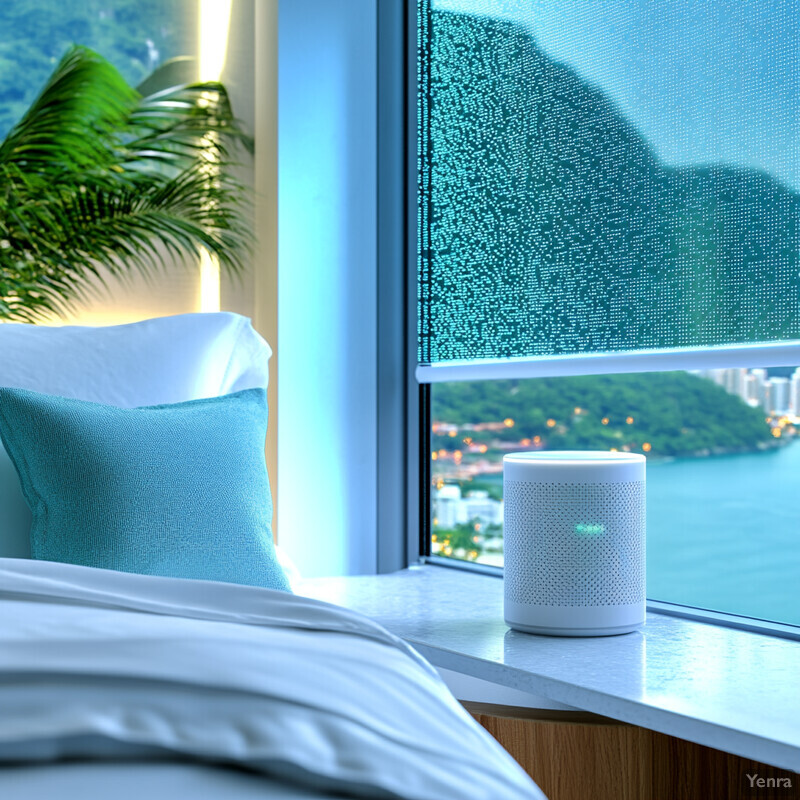
As part of the Internet of Things (IoT), air quality sensors, humidity monitors, and connected ventilation systems collect data from patients’ homes. AI processes this real-time environmental information to maintain allergen-safe indoor conditions by triggering automatic adjustments—like turning on air purifiers or adjusting humidity levels. This remote monitoring reduces the likelihood of allergen accumulation and ensures a consistently safer environment for patients. Additionally, the data collected can provide healthcare professionals with actionable insights into the patient’s living conditions, guiding further recommendations and interventions.
18. Early Intervention in High-Risk Populations
AI models can highlight populations at increased allergy risk due to socioeconomic factors, poor housing conditions, or limited healthcare access, guiding targeted public health interventions.
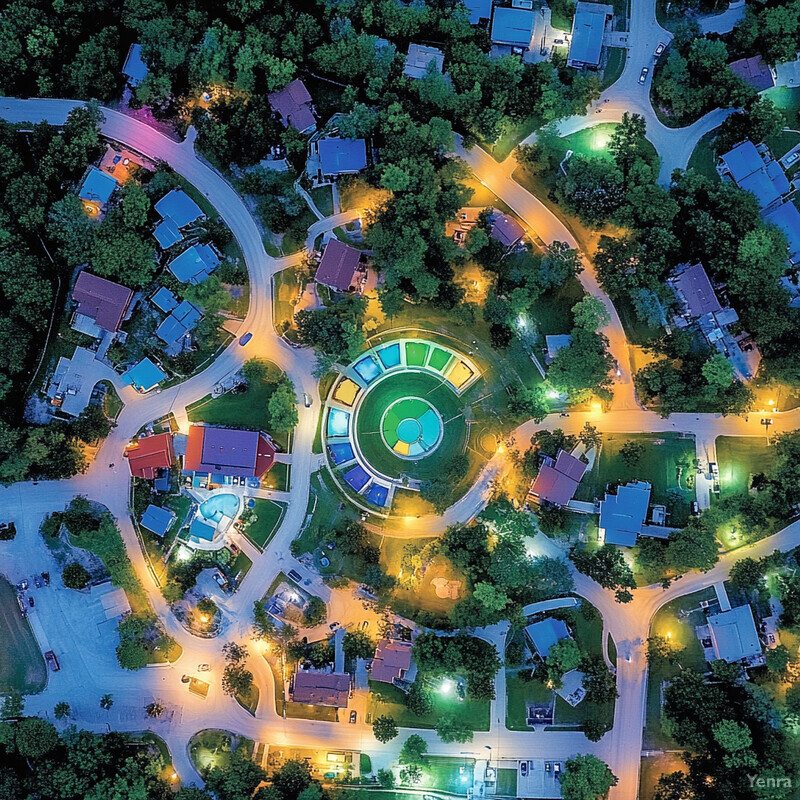
Socioeconomic and environmental disparities contribute to unequal allergy burdens. AI can highlight communities at heightened risk by analyzing housing quality, proximity to pollutants, access to healthcare, and prevalence of allergen sources. Public health officials and non-profit organizations can use these insights to implement targeted interventions—offering better screening, education, improved ventilation in schools, or policy changes to curb local allergen sources. Early, community-level responses driven by AI data help prevent severe allergic conditions and reduce healthcare disparities.
19. Adaptive Allergen Desensitization Strategies
By analyzing patient response data over multiple sessions, machine learning algorithms can recommend incremental adjustments to allergen exposure therapy, making treatments both safer and more effective.
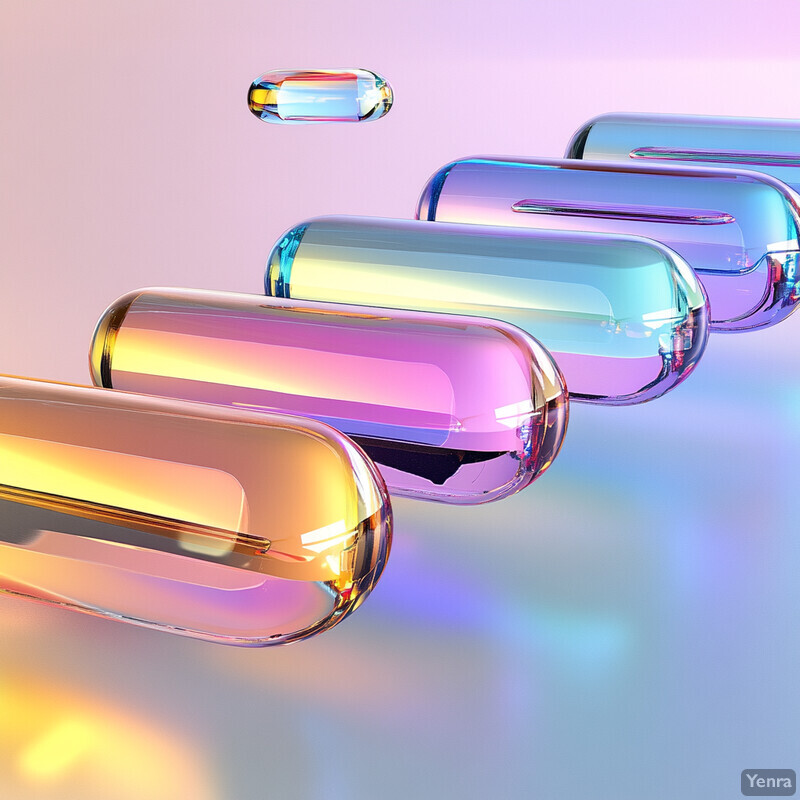
Desensitization therapies, including oral immunotherapy, rely on gradually increasing exposure to the allergen to build tolerance. AI systems can evaluate the patient’s response over time, adjusting the dose, speed of escalation, or supportive treatments based on personalized feedback. Such adaptive strategies ensure that patients progress at a pace aligned with their physiological responses, reducing dropouts due to adverse reactions and improving the therapy’s success rates. As a result, patients are more likely to achieve lasting tolerance with fewer setbacks and complications.
20. Data-Driven Policy and Public Health Recommendations
Large-scale AI analyses of allergic trends and environmental changes can inform public health policies, such as urban planning for vegetation management or regulations on food labeling, ultimately improving community-wide allergy management.
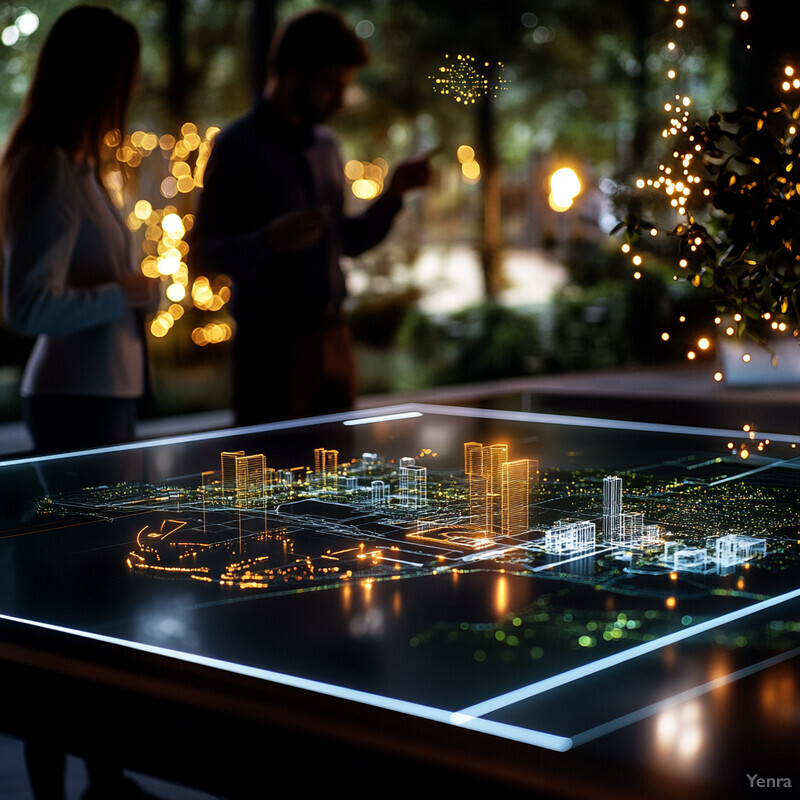
On a broader scale, AI’s ability to process environmental, epidemiological, and clinical data supports informed policy-making. By identifying trends—such as increasing pollen loads due to climate change or emerging food allergens—policymakers and healthcare leaders can implement evidence-based regulations. This might include improving food labeling laws, allocating resources to allergy research, optimizing urban planting schemes, or strengthening guidelines for school-based epinephrine access. Such data-driven decision-making fosters healthier communities and ensures that large-scale efforts to mitigate allergy risks are both targeted and effective.