Song: Algorithmic Art Curation
1. Automated Artwork Classification and Tagging
AI-driven image recognition systems can analyze artworks to generate descriptive keywords (e.g., medium, style, era) and themes, making it easier for curators to catalog and search collections.
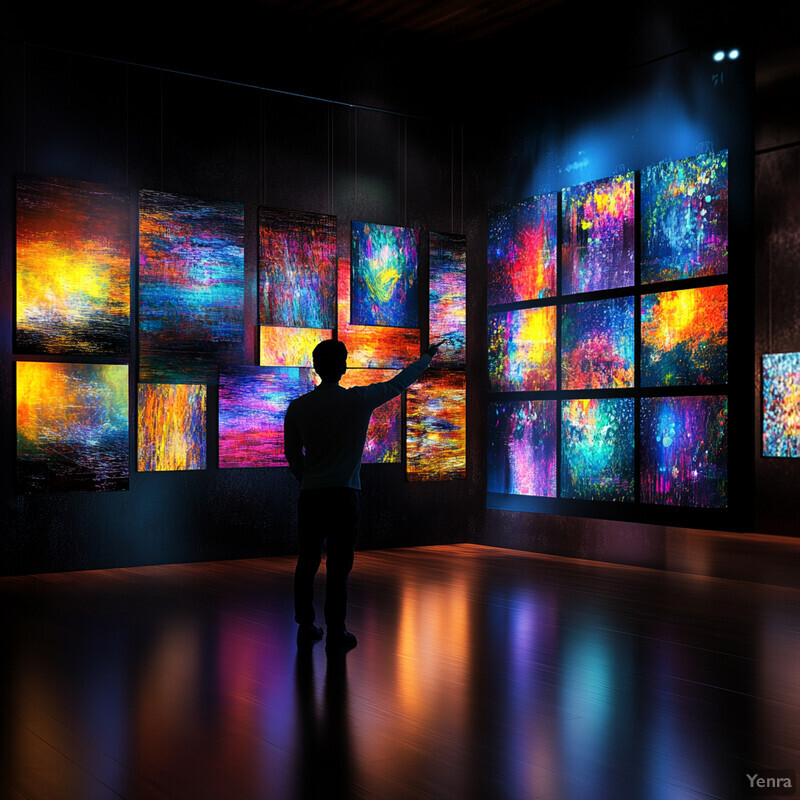
Modern computer vision models excel at extracting features like color distributions, shape patterns, and subject matter from images. Using these capabilities, AI can rapidly classify artworks into categories such as “Impressionist landscapes” or “Contemporary abstract sculptures.” With large collections, this automated tagging drastically reduces the burden on curatorial teams, who might otherwise need to examine each piece individually. As a result, institutions can quickly produce rich, searchable metadata that supports more nuanced browsing experiences, enabling visitors and researchers alike to find relevant works based on specific criteria rather than navigating through broad, generic categories.
2. Visual Similarity and Style Clustering
Sophisticated computer vision models group artworks by visual similarity—color palettes, brushstrokes, or composition—enabling curators to quickly identify related pieces and arrange coherent thematic exhibitions.
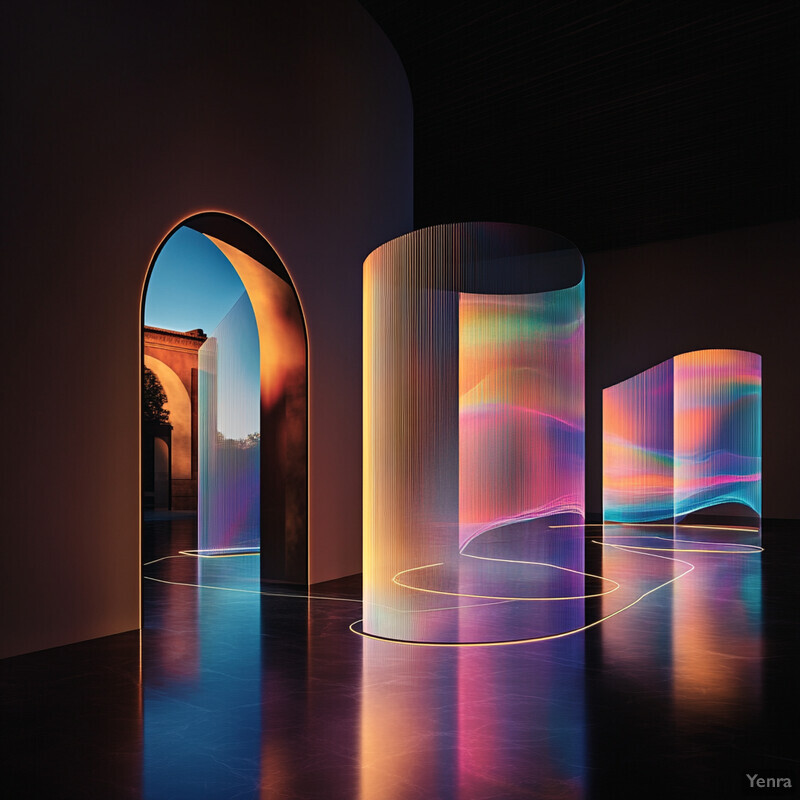
Beyond simple tagging, AI-powered clustering tools identify subtle visual relationships among artworks. Such systems consider thousands of aesthetic parameters—ranging from brushstroke texture and color harmonies to compositional balance—to group pieces by similarity. This method allows curators to discover unexpected connections, such as linking a 19th-century painting with a 21st-century digital print that share an uncanny color palette or geometric motif. For exhibition planning, these insights can spark new thematic narratives and help curators create more cohesive visual experiences that tell compelling, layered stories about art’s evolution and interconnectedness.
3. Personalized Recommendations for Visitors
Machine learning algorithms draw on user preferences, engagement history, and demographic data to recommend artworks that resonate with individual visitors, enhancing the personalization of both physical and virtual experiences.
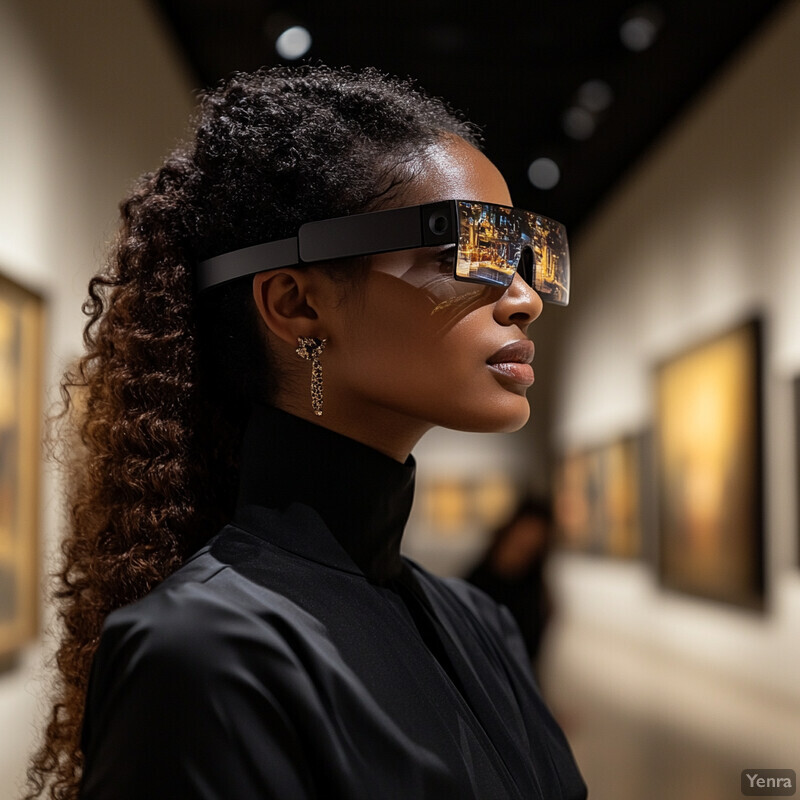
Just as streaming platforms suggest movies or music based on your viewing habits, AI-driven recommendation engines for art can guide museum-goers or online visitors to pieces matching their preferences. By analyzing a user’s browsing history, their interactions—such as dwell time on certain artworks or the artists they follow—and demographic information, these recommendation systems suggest items that resonate on a personal level. This tailored approach not only increases user engagement and satisfaction but also exposes visitors to artworks they might never have encountered otherwise, broadening cultural horizons and enriching their overall experience.
4. Intelligent Exhibition Planning Tools
AI-powered dashboards allow curators to simulate various exhibition layouts, test theme cohesiveness, and forecast visitor response, streamlining the planning process and reducing trial-and-error costs.
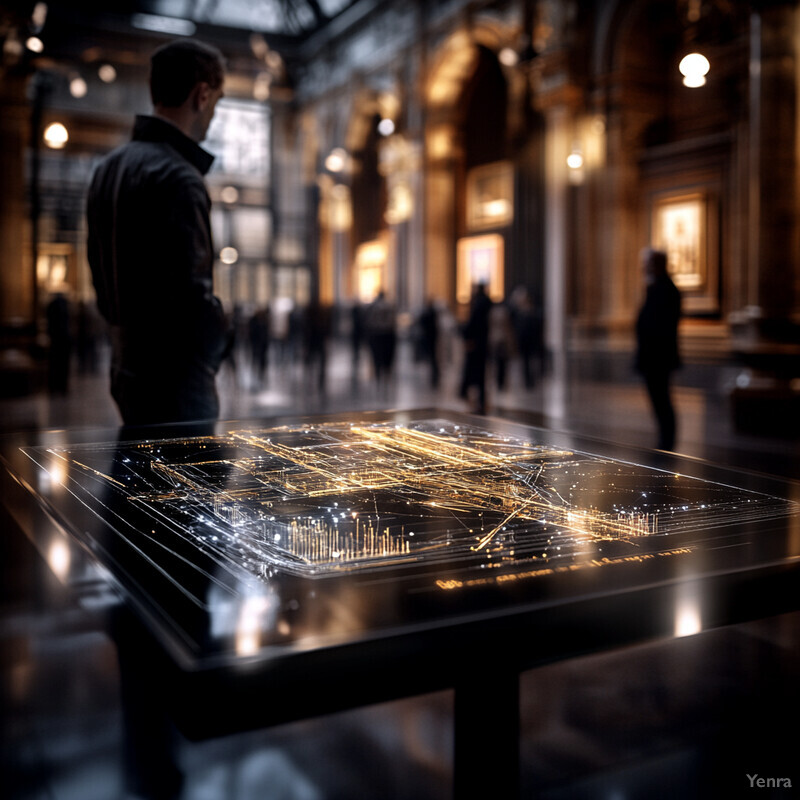
Curating an exhibition involves considering available space, thematic links, narrative flow, and visitor expectations. AI-powered planning tools integrate floor plan data, artwork metadata, and visitor traffic patterns to help curators visualize different layouts virtually before committing to final decisions. These systems can highlight potential bottlenecks, suggest logical sequences of artworks, and even forecast how a particular theme might resonate with audiences. By augmenting human intuition with data-driven insights, these tools streamline the planning process and empower curators to design more impactful, smoothly orchestrated exhibitions.
5. Predictive Trend Analysis
By analyzing global art market data, social media chatter, and museum attendance patterns, AI can predict emerging aesthetic trends, guiding curators to highlight rising artists or niche movements.
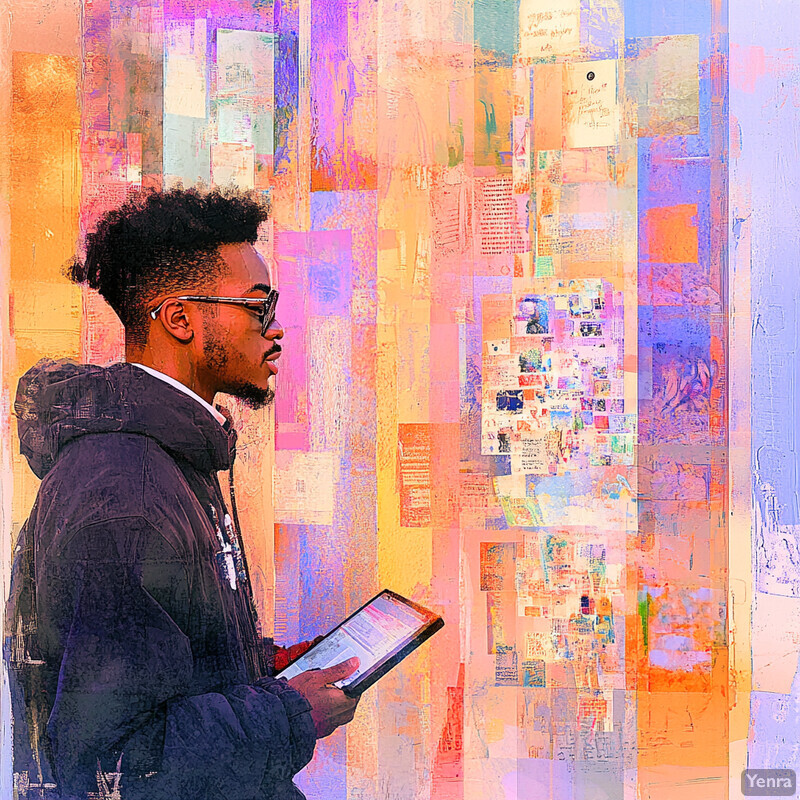
The art world’s shifting tastes and emerging movements can be difficult to anticipate. AI models, trained on market data, auction results, social media sentiment, and scholarly research, can identify patterns and predict trends with greater accuracy. Such predictive analytics help galleries and museums stay ahead of the curve by informing acquisition strategies and highlighting underrepresented artists or genres poised for broader recognition. By tapping into these insights, institutions can curate more future-oriented exhibitions that energize audiences and ensure their collections remain culturally relevant over time.
6. Contextualizing Art with Metadata
AI-driven natural language processing (NLP) can extract and summarize historical, cultural, and critical references from large text corpora, providing curators with richer contextual material for storytelling in exhibitions.
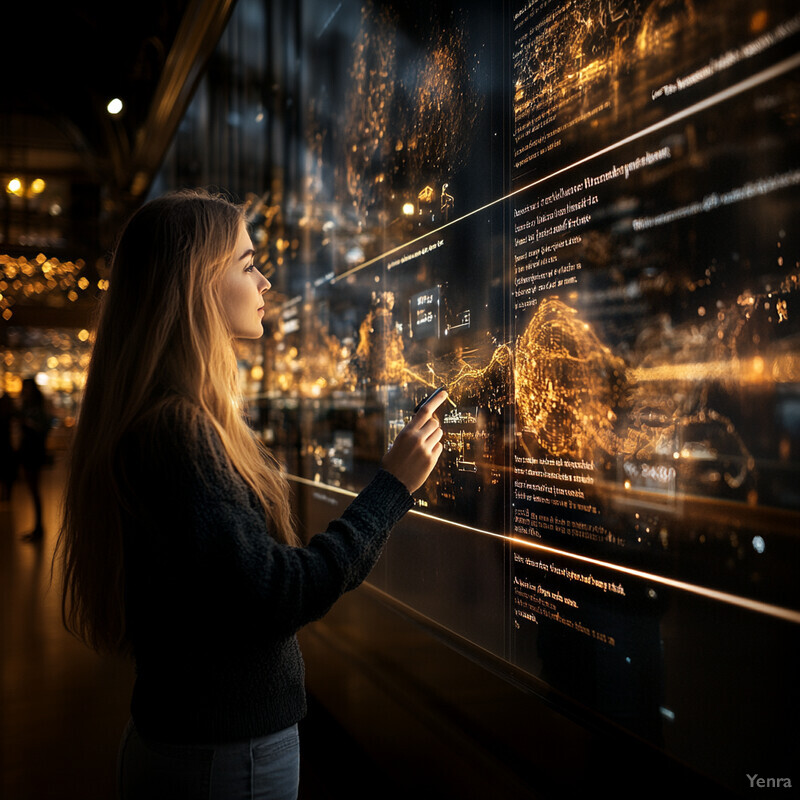
Art does not exist in a vacuum; it reflects its historical, cultural, and social context. AI-driven natural language processing (NLP) tools can mine large corpora of art criticism, historical documents, and scholarly essays to generate rich explanatory content. Through automated summarization and semantic analysis, the AI can present curators with ready-to-use narrative elements—key themes, influences, or references—that deepen the interpretation of artworks. Armed with these insights, curators can craft explanatory panels, catalogs, and digital guides that provide visitors with a richer, more layered understanding of each piece’s significance.
7. Quality and Novelty Assessment
Advanced models can evaluate the visual complexity or stylistic originality of artworks, giving curators data points to identify standout pieces and set them apart from large collections.
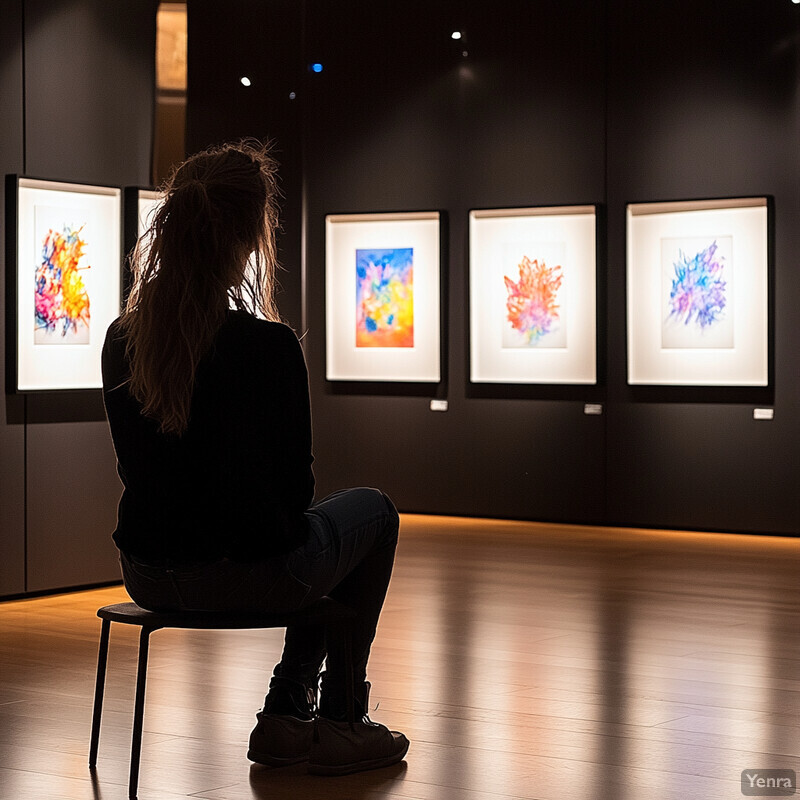
While subjective, perceptions of an artwork’s quality or originality can be partially informed by computational metrics. AI models can evaluate composition complexity, color uniqueness, and stylistic deviations from known canons to infer degrees of aesthetic innovation. Though not a replacement for human judgment and critical taste, these computational assessments can serve as helpful reference points for curators looking to highlight standout pieces. By integrating such objective indicators, curators can more confidently identify groundbreaking artworks that push boundaries and offer something genuinely new to audiences.
8. Cohesive Collection Management
Large-scale AI systems track thousands of artworks and automatically flag redundancies, stylistic gaps, or under-represented movements, supporting curators in maintaining a balanced, representative collection.
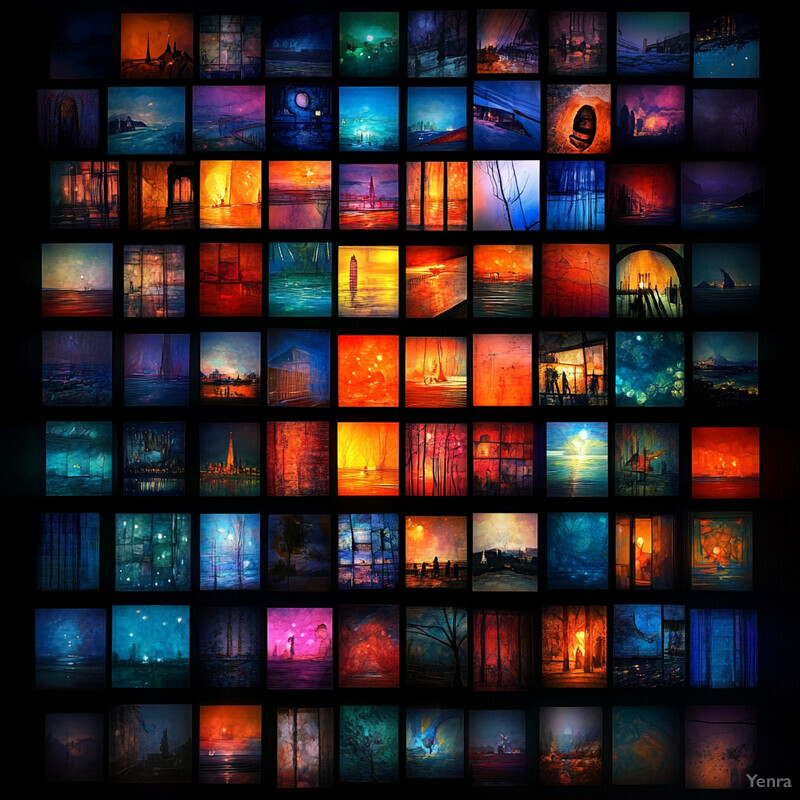
Museums and galleries manage vast inventories of artworks from multiple periods, styles, and media. AI can sift through these extensive collections, detecting imbalances and patterns that are easy to miss. For instance, an algorithm might indicate that Renaissance artworks are overrepresented while modern photography is underrepresented. By presenting these insights, AI enables curators to address collection gaps, diversify holdings, and ensure their galleries reflect a wide spectrum of artistic expressions. Ultimately, this leads to a more inclusive and representative cultural narrative that resonates with a broad range of visitors.
9. Audience Sentiment and Response Analysis
By analyzing visitor feedback, reviews, and social media engagement, AI can gauge public sentiment toward certain styles or artworks, informing curators which pieces to spotlight or rotate out.
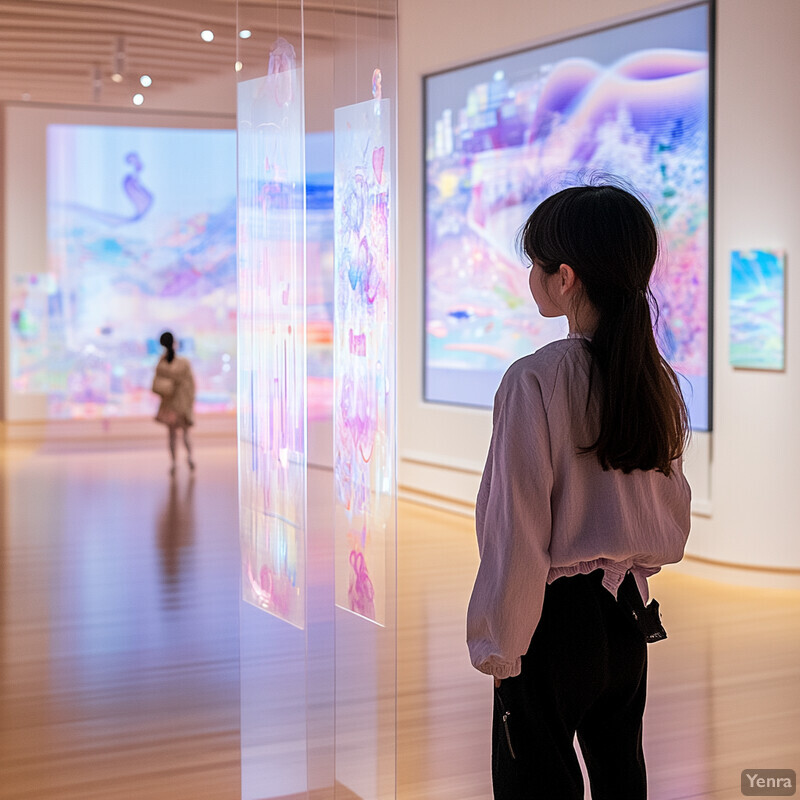
Understanding public reception of artworks is essential for dynamic curation. AI-driven sentiment analysis scans social media, review platforms, and visitor feedback forms to gauge how audiences feel about specific exhibitions or pieces. Identifying trends in praise or criticism helps curators refine their selections and adapt future shows to better align with visitor interests. By embracing a data-driven dialogue with audiences, institutions can remain agile, continuously improving the visitor experience and staying culturally relevant.
10. Enhanced Accessibility and Discovery
AI algorithms help surface lesser-known artworks and neglected artists. Through recommendation engines and intuitive search interfaces, both novices and scholars discover hidden gems.
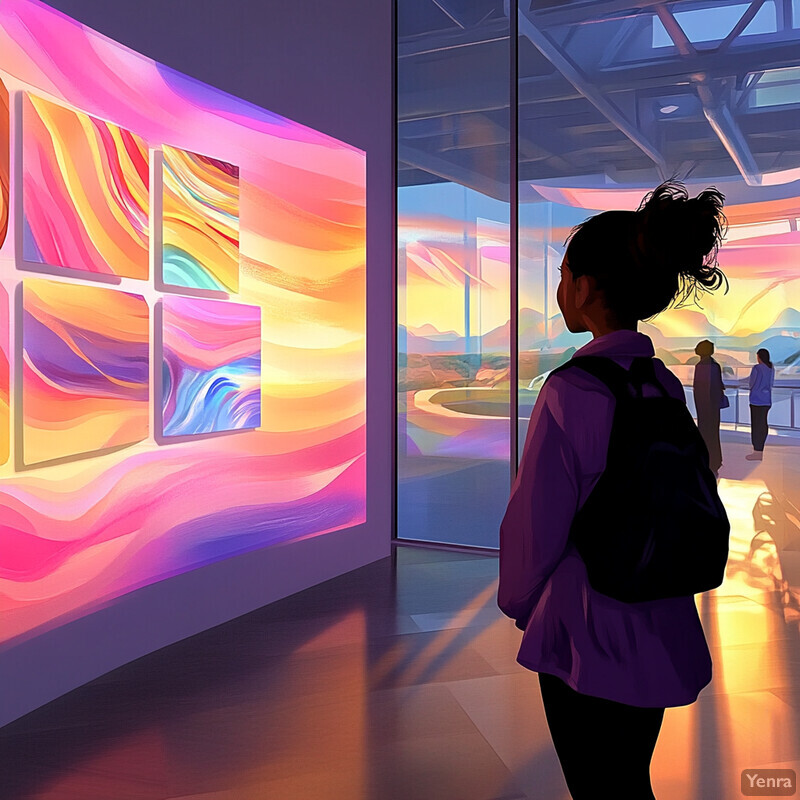
With AI-driven search and discovery tools, visitors can navigate collections in more intuitive and adventurous ways. Instead of searching solely by artist or era, users might look for “warm landscapes” or “minimalist sculpture about identity.” The AI’s ability to parse these nuanced queries allows visitors to stumble upon surprising connections that transcend traditional classification. By providing flexible pathways through a collection, these tools engage visitors’ curiosity, encouraging deeper exploration and fostering a more personal, self-directed encounter with art.
11. Dynamic Online Galleries and Virtual Exhibitions
In online platforms, AI can dynamically rearrange virtual gallery spaces based on viewer preferences, visitation patterns, or thematic connections, making digital exhibitions more engaging and personalized.
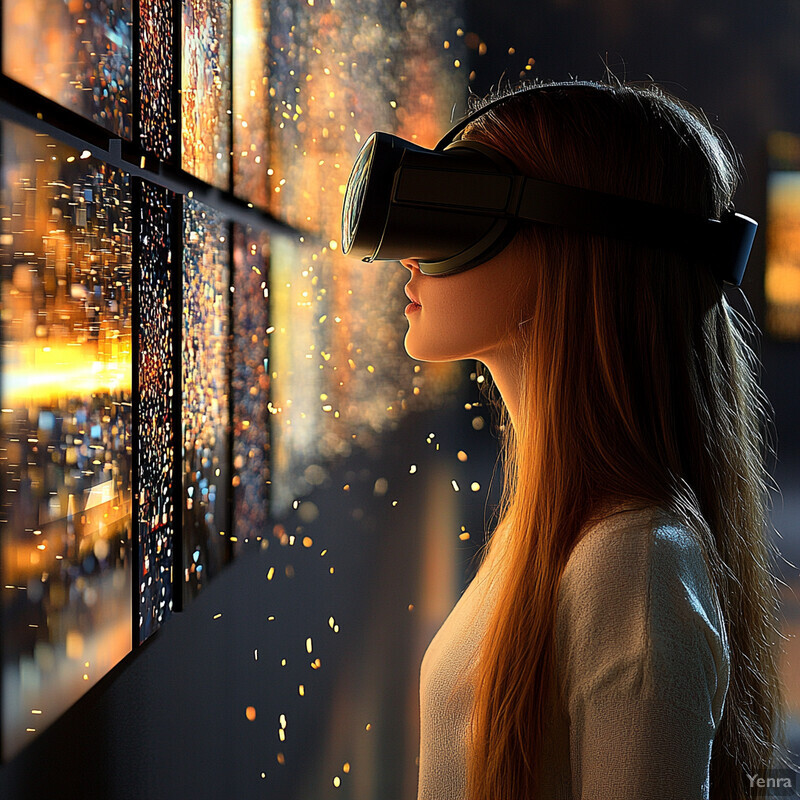
As digital platforms evolve, AI can dynamically curate online exhibitions that adapt in real-time to viewer behavior and global cultural events. For example, if a sudden interest in a particular artist emerges online, the virtual gallery’s algorithm can instantly highlight related works. This responsiveness creates a living, breathing digital space where content stays fresh and relevant. By removing static constraints, AI-driven curation in virtual realms enriches user engagement and allows art experiences to evolve in sync with audience interests and cultural currents.
12. Automated Attribution and Provenance Research
Machine learning models aid in attributing unsigned or disputed artworks to specific artists or periods by analyzing subtle stylistic signatures, helping ensure the accuracy and credibility of curated collections.
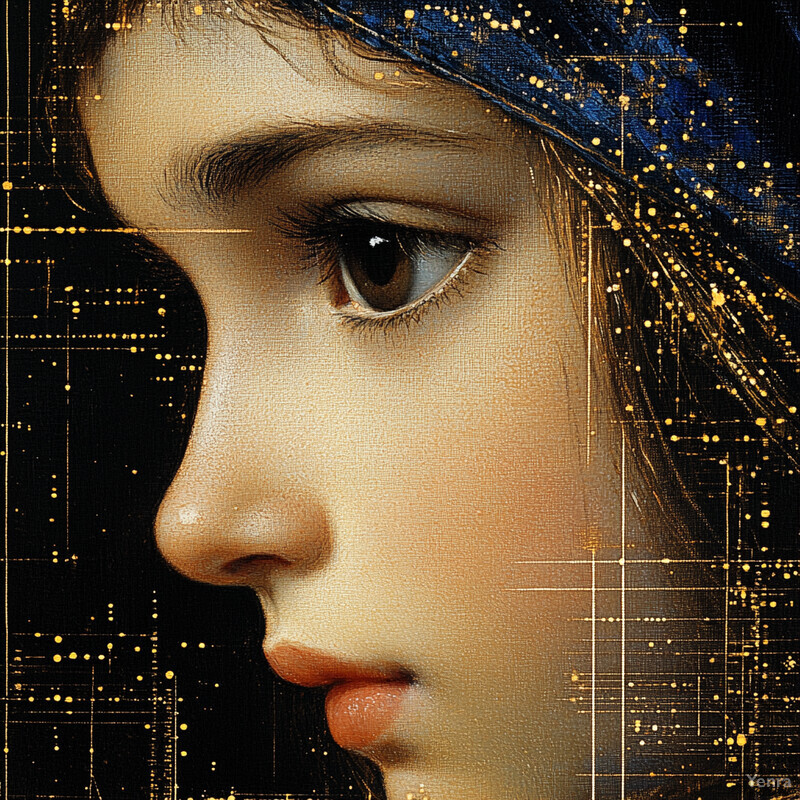
Determining an artwork’s creator or verifying its authenticity can be a painstaking process. AI models trained on known works by specific artists can identify stylistic signatures—such as brushstroke patterns or choice of subject matter—to support curatorial staff in making informed attributions. Similarly, AI can cross-reference historical sales records, exhibition catalogs, and art databases to trace an artwork’s provenance. By accelerating and enhancing these research processes, AI helps maintain the intellectual rigor and authenticity underlying curatorial practice.
13. Adaptive Educational Content
AI-driven personalization tailors explanatory texts, comparison images, and artist biographies to a visitor’s knowledge level and interests, enhancing educational value in both museums and online viewing platforms.
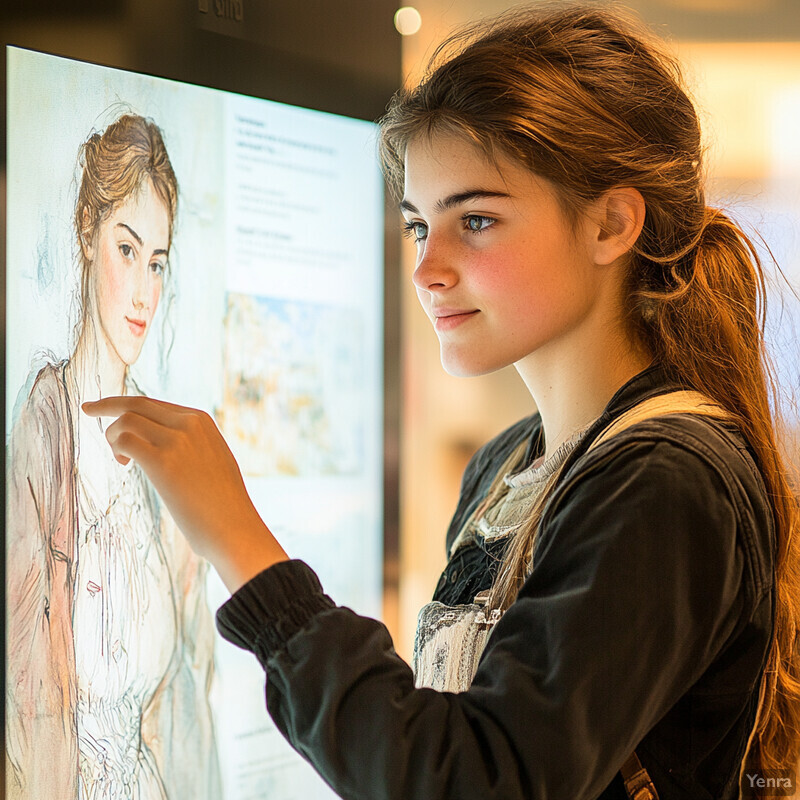
Museums serve educational roles, but different visitors have different levels of knowledge and interest. AI-driven personalization can tailor interpretive materials—such as audio guides or interactive displays—based on a visitor’s background and engagement patterns. A novice may receive simple explanations of an artwork’s basic elements, while a seasoned enthusiast might encounter in-depth analyses or connections to related literary works. This level of customization ensures that each visitor can learn at their own pace, making art more accessible, stimulating, and rewarding.
14. Multilingual and Cross-Cultural Curation
NLP-based translation and cultural adaptation tools help curators present collections to international audiences, ensuring that textual descriptions and contextual information remain accurate and culturally resonant.
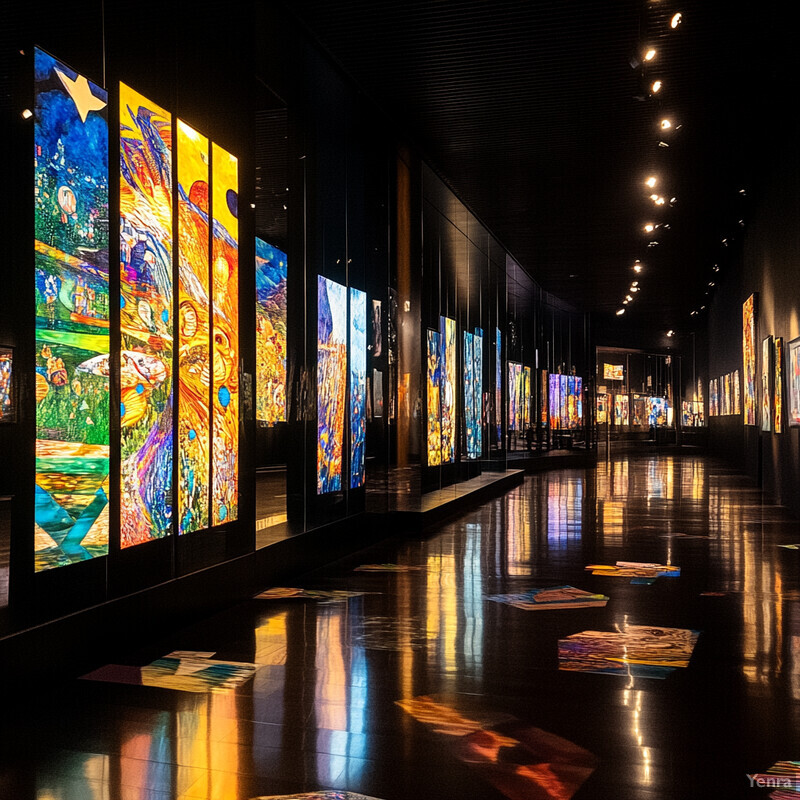
With global audiences, multilingual support is crucial. NLP-based translation and cultural adaptation tools can ensure that text panels, website descriptions, and educational materials resonate across languages and cultures. AI can detect nuances in language usage and regional references, helping curators present collections in ways that are culturally sensitive and contextually appropriate. This broadens the institution’s appeal, allowing it to serve diverse audiences and foster international dialogues around art.
15. Fraud and Forgery Detection
Advanced imaging and pattern recognition systems help identify suspicious patterns that might indicate forged works, safeguarding the integrity of collections and ensuring authenticity.
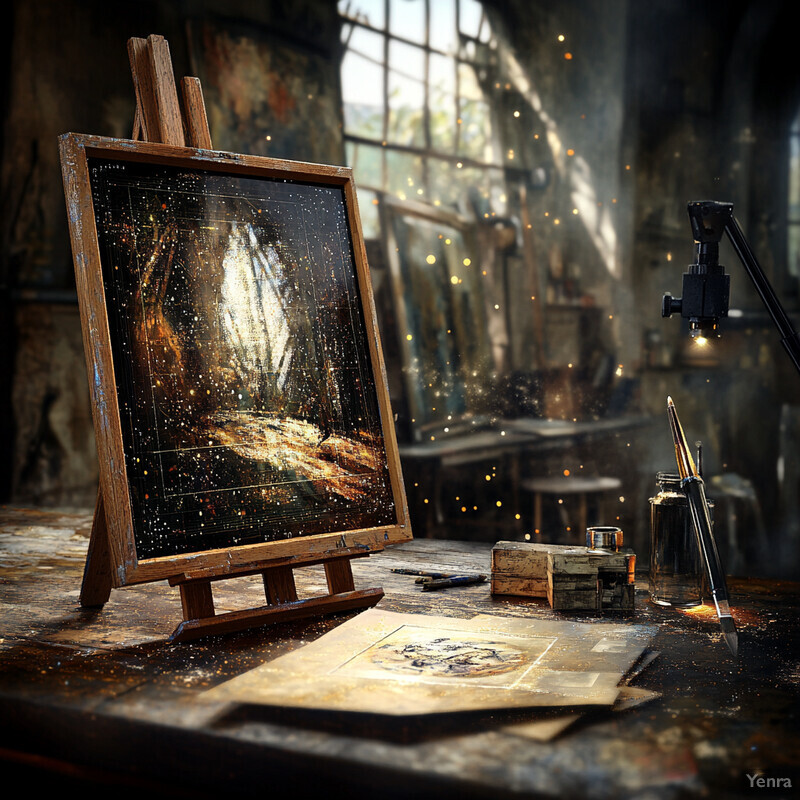
The art market’s high stakes can attract deceptive practices. AI tools trained on authentic works identify microscopic details—such as pigment composition or irregularities in brushwork patterns—that help detect forgeries. These tools assist curators, buyers, and conservators by providing a digital “second opinion” that raises red flags when something seems amiss. With strengthened trust in authenticity, institutions can maintain integrity and confidence in the works they exhibit and study.
16. Event and Marketing Optimization
Predictive analytics show when certain works or exhibitions are likely to draw crowds, enabling institutions to schedule high-profile shows, time new installations, or tailor marketing campaigns more effectively.
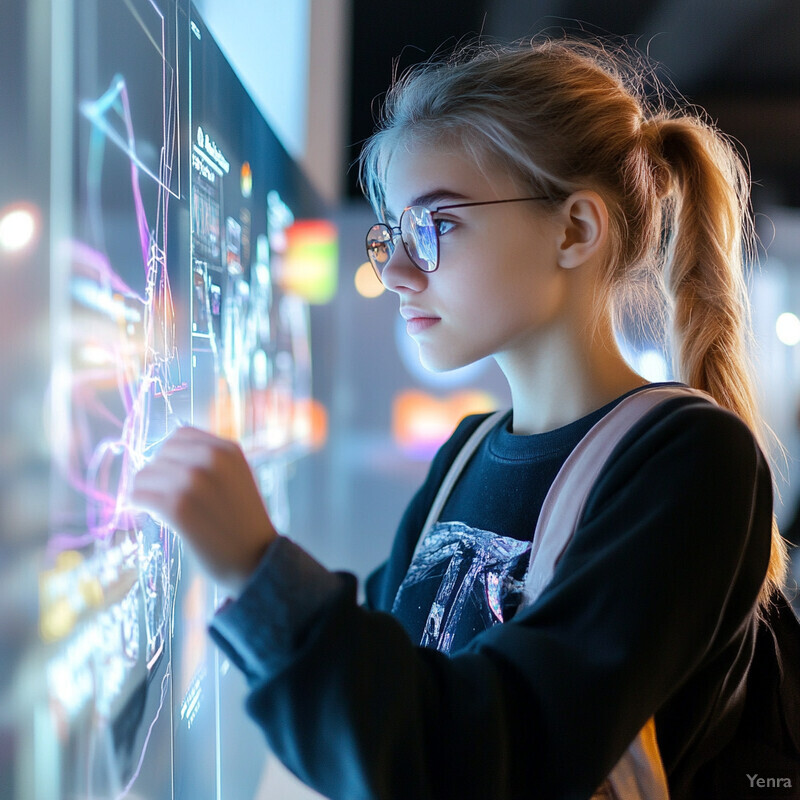
AI-based analytics can forecast which exhibitions are likely to draw the largest crowds based on historical data, promotional efforts, and seasonal trends. Knowing this, curators and marketing teams can time major shows for peak visitor interest, optimize promotional content, and schedule events or lectures that align with audience demand. The result is more efficient use of resources, improved visitor satisfaction, and a stronger return on investment in both cultural and financial terms.
17. Optimized Gallery Layouts
AI systems can model visitor flow patterns and suggest artwork placements that balance crowd movement, sightlines, and thematic coherence, enhancing the overall gallery experience.
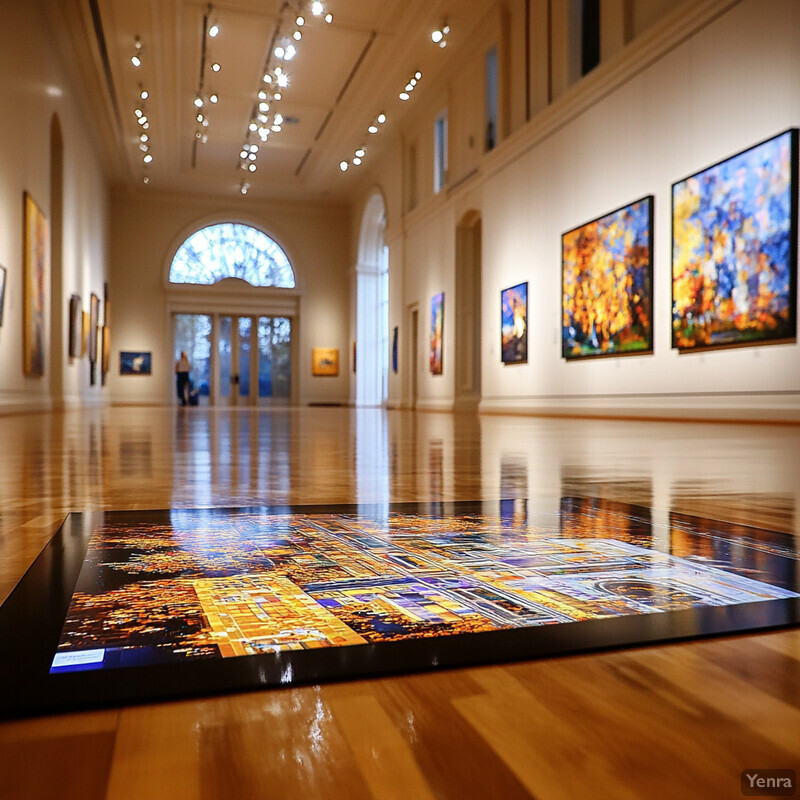
Physical space plays a significant role in how visitors experience art. AI can simulate visitor flows, evaluating factors such as line-of-sight visibility, signage placement, and the distribution of popular works. By testing scenarios virtually, curators can optimize gallery layouts that minimize congestion, ensure proper sightlines, and create a balanced progression through the exhibition. This thoughtful approach to spatial arrangement improves navigation, encourages deeper engagement, and ensures every visitor can enjoy the artwork comfortably.
18. Facilitating Cross-Institutional Collaborations
AI-driven platforms can match complementary artworks held at different institutions, encouraging partnerships and traveling exhibitions that bring fresh curatorial concepts to life.
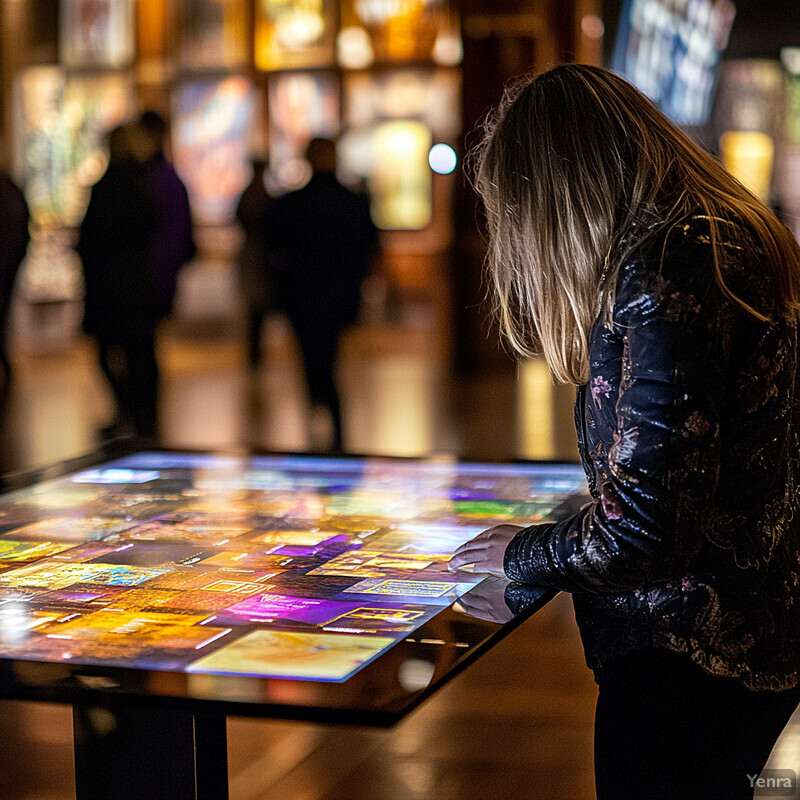
Many artworks are dispersed among institutions worldwide. AI can scan multiple collections simultaneously, identifying pieces that share themes, artists, or historical significance. This makes it easier for curators to propose traveling exhibitions, joint shows, or loan exchanges that enrich each institution’s programming. By uncovering synergies between distant collections, AI fosters cultural exchange and collaborative storytelling, promoting a more interconnected global art community.
19. Sustainability and Cost Management
Analyzing operational data, AI can recommend cost-effective exhibition designs and resource usage patterns, guiding institutions toward more sustainable curatorial strategies without compromising artistic impact.
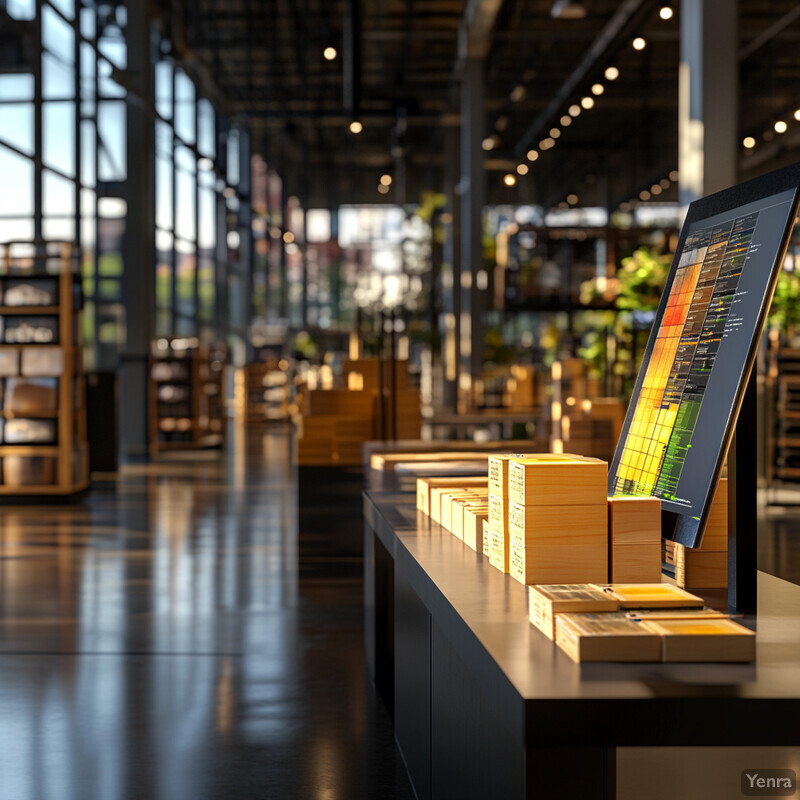
Operating exhibitions comes with environmental and financial considerations, from energy usage to shipping and storage costs. AI models that consider energy consumption, material requirements, and visitor numbers can recommend more sustainable setups—like choosing LED lighting over traditional halogens or grouping artworks to reduce shipping trips. These data-driven optimizations allow curators to maintain high-quality exhibitions while also aligning with ecological and fiscal responsibility, ensuring that art presentation remains sustainable in the long run.
20. Long-Term Collection Strategy
With historical data analysis and predictive modeling, AI provides curators insights on how collections might evolve, what acquisitions would fill gaps, and which pieces should be highlighted or archived, helping shape a long-term curatorial vision.
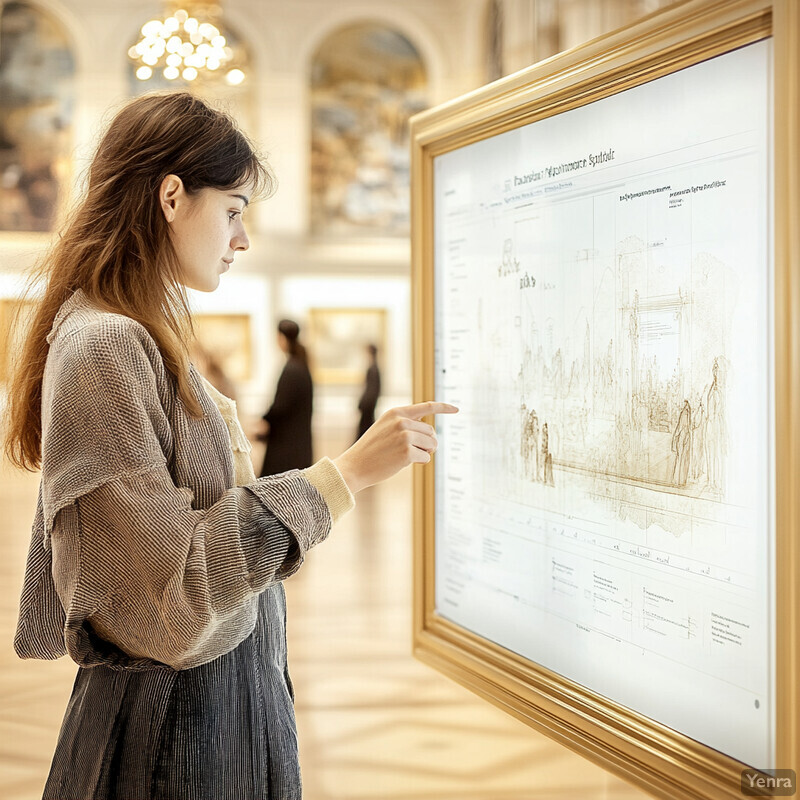
Collections evolve as cultural dialogues shift. AI can analyze historical acquisition patterns, shifting audience interests, and emerging art movements to inform strategic decisions about what to acquire, highlight, or deaccession. For example, the system might identify a growing interest in digital media, prompting the museum to seek more contemporary works. Over time, this guidance ensures that collections remain dynamic, meaningful, and relevant, allowing curators to steer their institutions into the future with confidence and vision.