Video: Aerodynamic Optimization
Song: Aerodynamic Optimization (Lyrics)
1. Automated Shape Optimization
AI-driven algorithms can autonomously refine wing and body geometries, finding configurations that minimize drag and improve lift without manual trial-and-error.
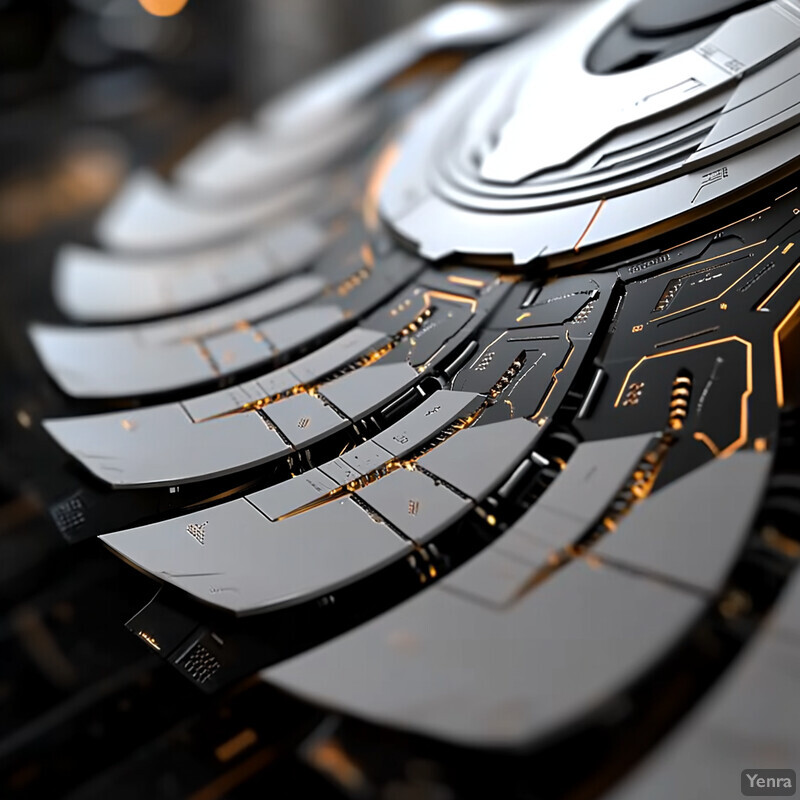
AI-driven automated shape optimization revolutionizes the way engineers approach aerodynamic design. Instead of relying on human intuition or laborious manual adjustments, algorithms such as genetic or evolutionary optimization techniques can autonomously refine wing profiles, fuselage contours, and other aerodynamic surfaces. By iterating through vast design spaces and evaluating performance metrics like lift-to-drag ratio at each step, these methods rapidly converge on near-optimal geometries. This not only reduces the development timeline from months or years to weeks but also often uncovers unconventional shapes that outperform traditional designs. The end result is a more efficient, agile design process that can respond to emerging engineering challenges and market demands.
2. Surrogate Modeling for CFD
Machine learning models act as “surrogates” for expensive Computational Fluid Dynamics (CFD) simulations, predicting aerodynamic performance rapidly and enabling more design iterations.
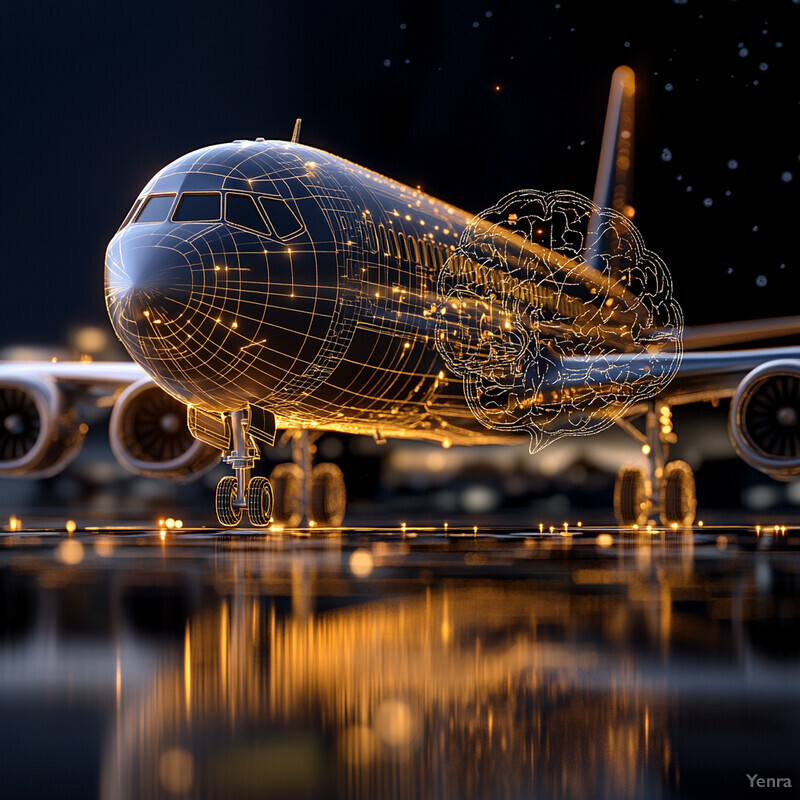
Surrogate models offer a way to bypass the computational intensity of full-scale CFD simulations. Trained on a set of high-fidelity simulation results, these AI-driven approximations can predict aerodynamic metrics—such as pressure distributions, lift, and drag—for new configurations in a fraction of the time. By using machine learning techniques like Gaussian process regression, neural networks, or kriging, engineers can quickly screen thousands of potential designs without running a single full CFD. This speed-up enables a more thorough exploration of design spaces, leading to superior aerodynamic solutions. As a result, aerodynamic optimization becomes more iterative, affordable, and accessible, making advanced analyses feasible even in early design phases.
3. Reduced-Order Flow Modeling
Neural networks create reduced-order models to capture essential flow features, cutting down the computational cost of full-scale simulations.
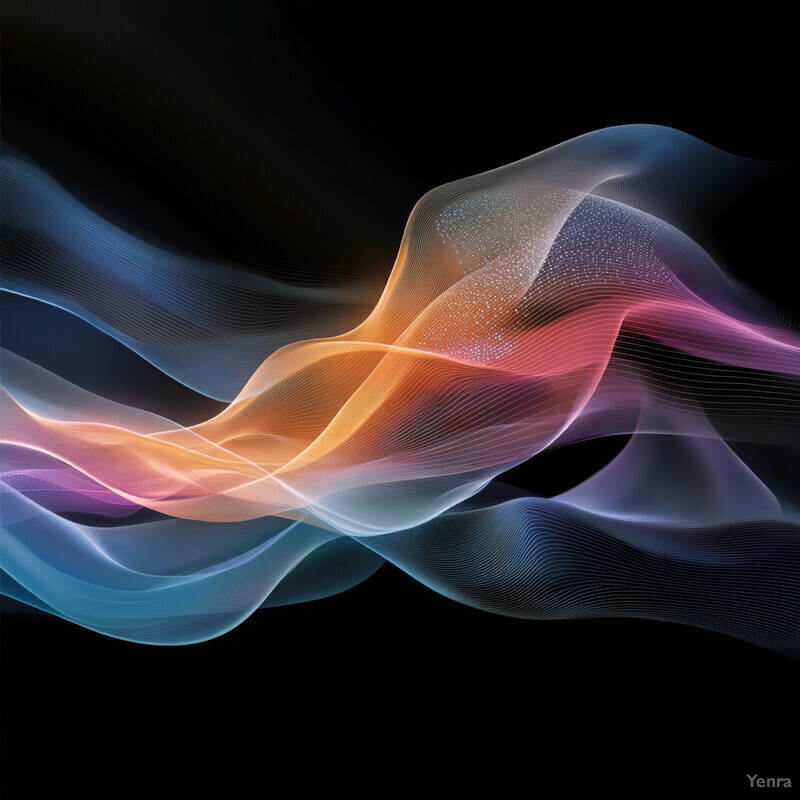
Reduced-order models (ROMs) leverage AI to distill complex fluid dynamics into simpler, computationally inexpensive representations. Traditional CFD simulations may be prohibitively time-consuming, but ROMs learned from simulation data can capture the essential physics and flow characteristics at a much lower cost. Neural networks and other machine learning techniques identify dominant flow patterns—like vortex shedding or turbulent eddies—enabling engineers to predict aerodynamic behavior rapidly. This capability empowers teams to run parametric studies, evaluate sensitivity to design changes, and perform uncertainty analyses. Ultimately, reduced-order models transform complex aerodynamic assessments into manageable, real-time tools for informed decision-making.
4. Turbulence Modeling
Data-driven approaches help improve turbulence closure models, providing more accurate results than conventional RANS or LES methods at a fraction of the computational expense.
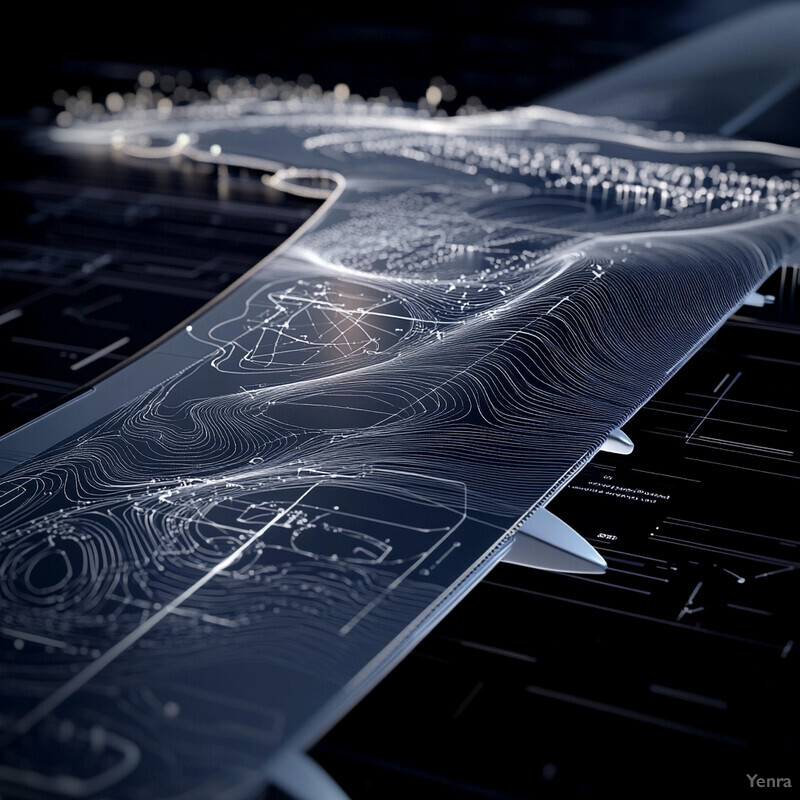
Turbulence remains one of the trickiest challenges in aerodynamic analysis due to its chaotic, multi-scale nature. AI-enhanced turbulence models tap into large datasets—from high-fidelity simulations or experimental measurements—to more accurately predict turbulent flow behavior. By training neural networks or other regression models on fine-grained datasets, engineers can improve upon standard turbulence closures like k-ε or k-ω. This results in more reliable predictions of flow separation, wake dynamics, and shear layer interactions. Enhanced turbulence modeling not only boosts simulation accuracy but also informs more effective aerodynamic designs, reduces drag, and improves overall performance.
5. Inverse Design Techniques
AI systems can start with desired aerodynamic performance criteria and reverse-engineer optimal shapes, removing guesswork and accelerating the development process.
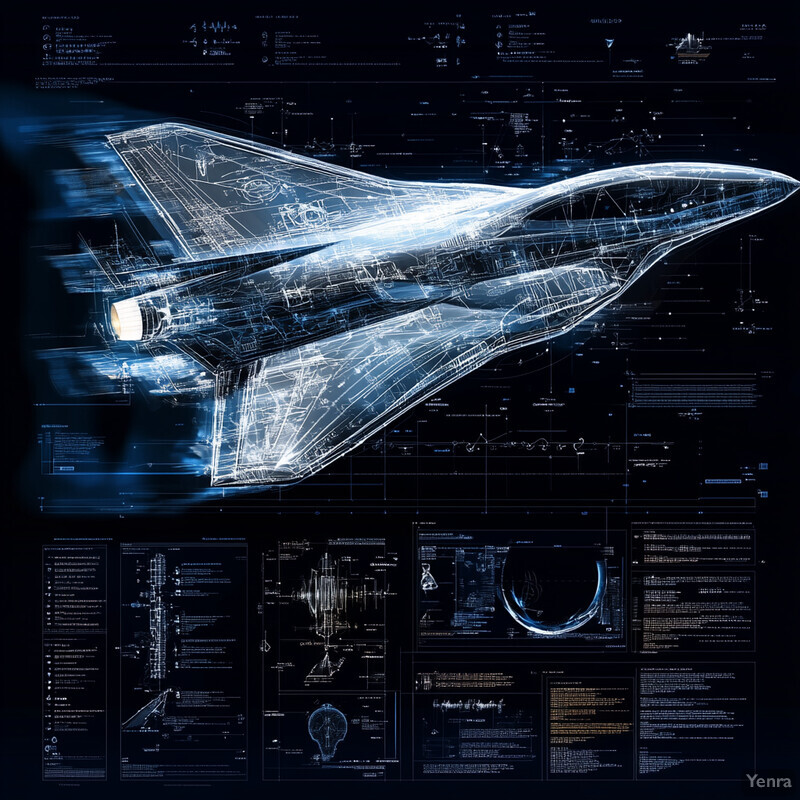
Inverse design powered by AI flips the conventional approach to aerodynamic optimization. Instead of starting with a given shape and iteratively refining it, engineers first specify target performance criteria—like a desired lift-to-drag ratio or pressure distribution—and let AI find the geometry that achieves those goals. Algorithms such as neural networks, adjoint-based methods, or evolutionary optimization navigate the design space efficiently, producing shapes that might elude traditional trial-and-error methods. This approach accelerates innovation, allowing teams to leap directly from performance requirements to high-quality configurations. Inverse design ultimately shortens development cycles and fosters the exploration of novel aerodynamic concepts.
6. Multidisciplinary Optimization
Advanced AI-driven frameworks handle simultaneous optimization of aerodynamic, structural, and control criteria, leading to holistic and well-balanced designs.
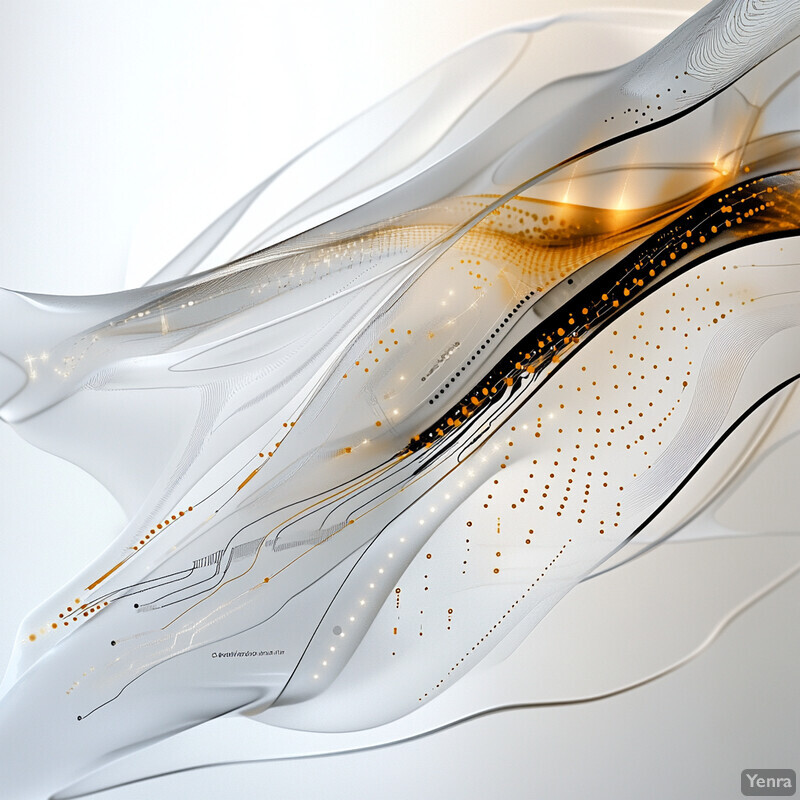
Modern aerodynamic design rarely occurs in a vacuum. Structures, propulsion systems, thermal management, and environmental factors all interconnect. AI-driven multidisciplinary optimization platforms integrate aerodynamic performance objectives with constraints from these other domains. By doing so, engineers can find designs that balance aerodynamic efficiency with structural integrity, minimal noise, reduced environmental impact, and lower production costs. This holistic approach ensures that advances in aerodynamics don’t come at the expense of durability, manufacturability, or sustainability. As a result, multidisciplinary optimization promotes designs that are not only aerodynamically excellent but also practical, economical, and future-ready.
7. Reinforcement Learning for Control Surfaces
By applying reinforcement learning, control surfaces like ailerons or flaps can be automatically adjusted for optimal aerodynamic efficiency in various flight conditions.
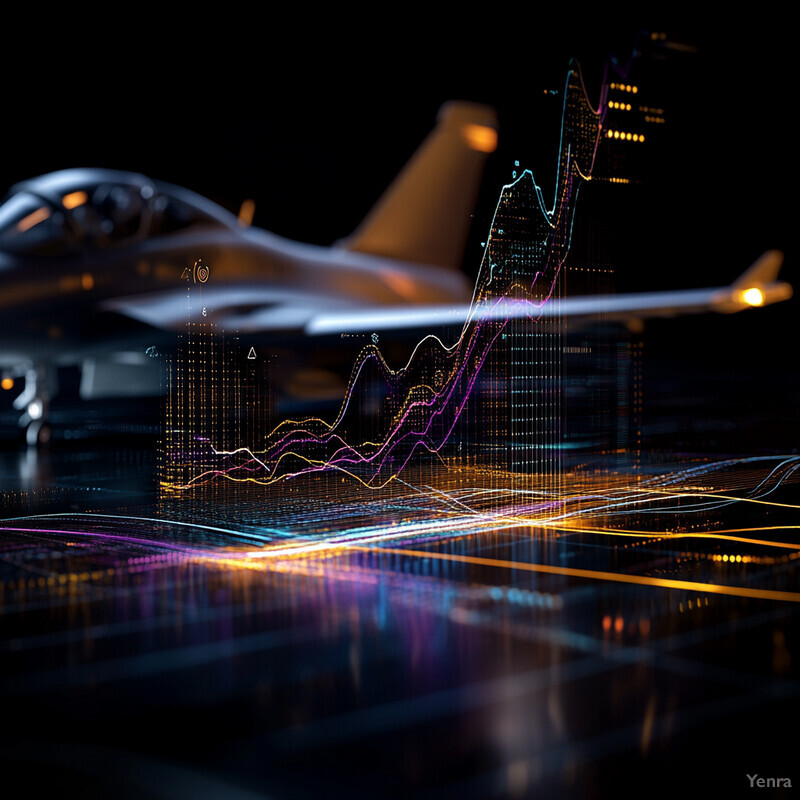
Reinforcement learning (RL), inspired by how animals learn from interaction with their environment, can tune control surfaces like flaps and ailerons to achieve optimal aerodynamic configurations during flight. Instead of relying on fixed control laws, RL agents learn policies that minimize drag, maintain lift, or achieve steady maneuvers by continuously refining their actions based on real-time feedback. This dynamic adaptation outperforms static strategies, as the aircraft can respond instantly to changes in wind conditions, turbulence, or mission profiles. Over time, RL-based control leads to more fuel-efficient operations, extended range, and improved flight safety—significantly advancing aerodynamic efficiency during actual flight conditions.
8. Uncertainty Quantification
AI techniques help incorporate and quantify uncertainties (in material properties, environmental conditions, etc.), ensuring robust aerodynamic solutions under real-world conditions.
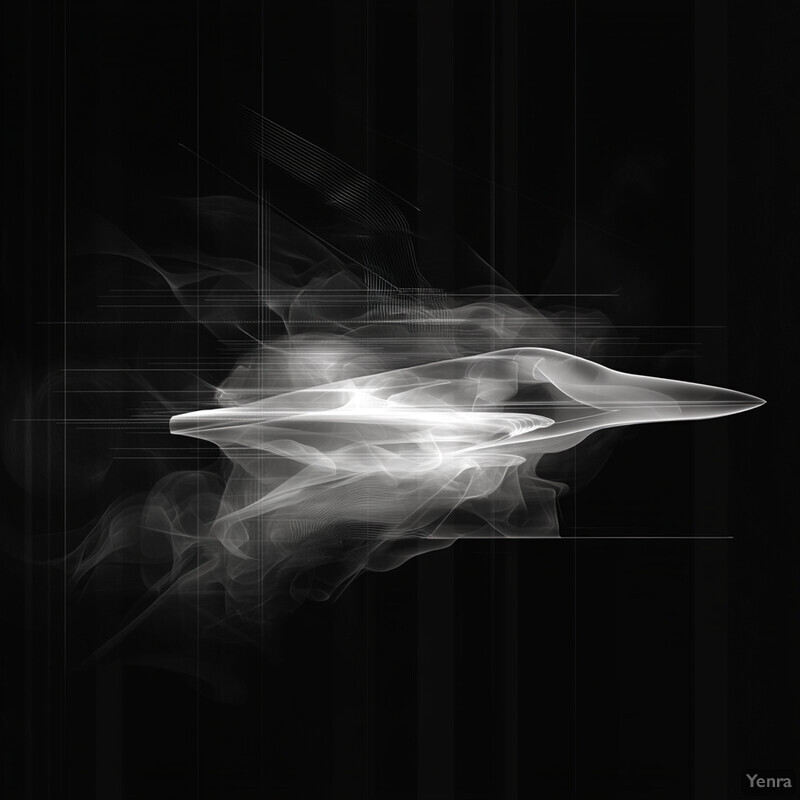
Real-world aerodynamic scenarios involve uncertainties in material properties, operational conditions, and measurement accuracy. AI-based uncertainty quantification methods allow designers to understand how these variations affect aerodynamic performance. By modeling inputs as random variables and using machine learning to propagate uncertainties through aerodynamic models, engineers gain insights into confidence intervals and probability distributions of key performance metrics. This enables more robust designs that still perform well even when conditions deviate from the nominal. Ultimately, integrating uncertainty quantification ensures that the final aerodynamic solutions are resilient, safe, and reliable under a wide range of real-world conditions.
9. Bayesian Optimization
Using Bayesian methods guided by AI, designers can navigate complex aerodynamic landscapes efficiently, quickly honing in on high-performance configurations.
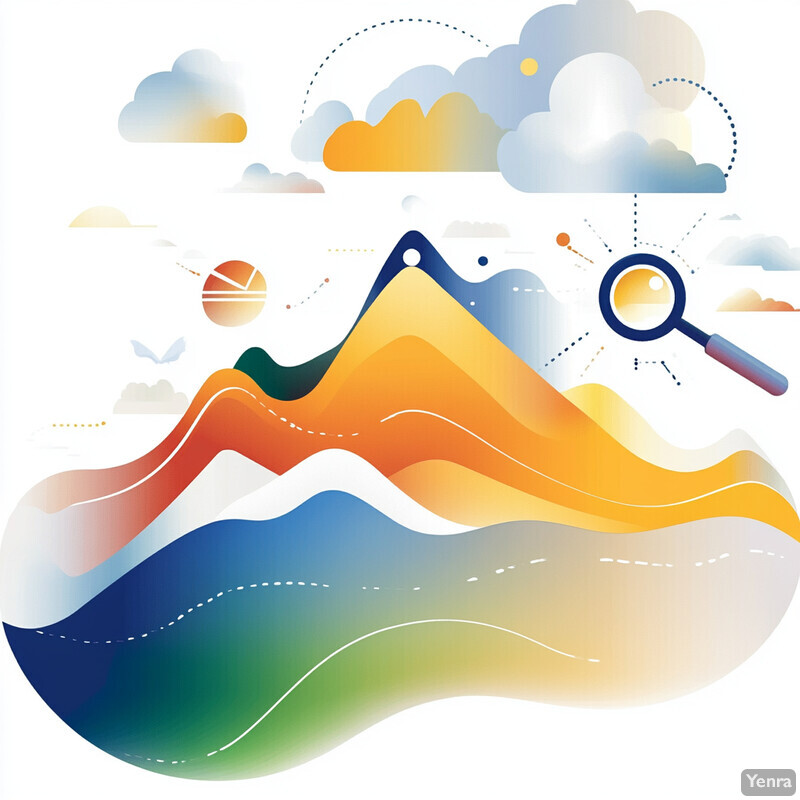
Bayesian optimization leverages probabilistic models to guide aerodynamic searches intelligently. Instead of evaluating every candidate design, it focuses on the most promising regions of the design space, constantly refining its predictions as new information becomes available. By using a Bayesian framework, engineers can incorporate prior knowledge, adaptively sample design points, and zero in on optimal shapes with fewer expensive simulations. This approach reduces the cost and time of optimization while improving the final outcome. Combined with AI-based predictions of aerodynamic performance, Bayesian optimization provides a powerful toolkit for efficiently navigating complex design landscapes.
10. Real-Time Wind Tunnel Data Interpretation
Machine learning models rapidly interpret sensor data from wind tunnel experiments, guiding parameter adjustments on-the-fly to achieve faster convergence.
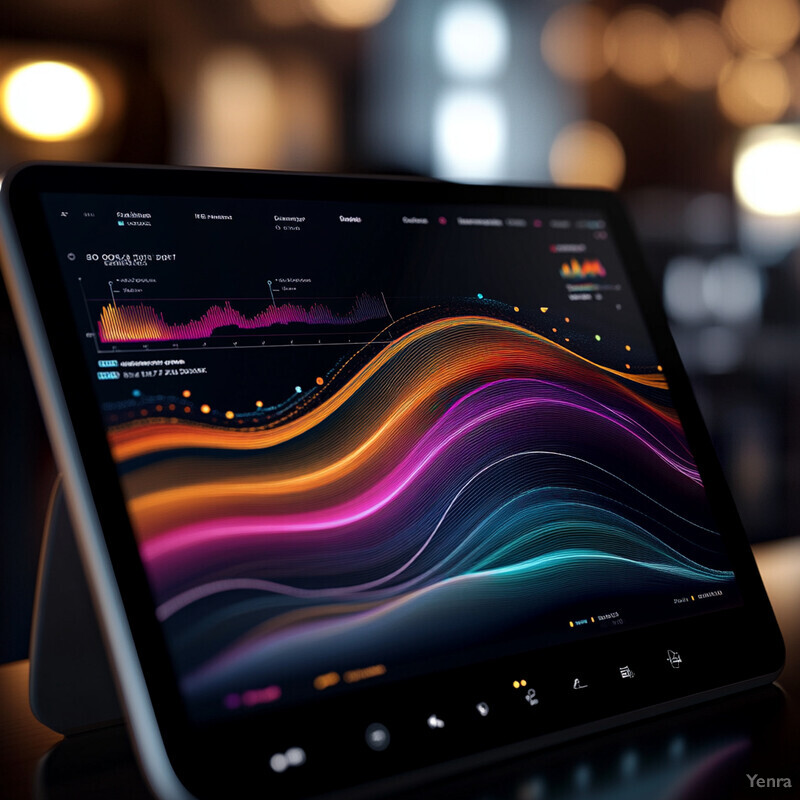
Wind tunnel testing traditionally involves meticulous measurement and subsequent offline analysis. AI-driven systems can now process sensor outputs—pressure taps, particle image velocimetry (PIV), force balances—on the fly, interpreting aerodynamic behavior in real-time. By identifying trends, comparing results to known benchmarks, and suggesting parameter adjustments, AI streamlines the experimental process. Engineers can rapidly iterate on test parameters, shapes, or orientations, drastically accelerating the path to performance improvements. Instantaneous feedback in the wind tunnel helps refine designs more efficiently, bridging the gap between simulation predictions and experimental validation.
11. Adaptive Mesh Refinement
AI can direct where and when to refine computational meshes in CFD, focusing computational resources on critical flow regions for higher accuracy without unnecessary cost.
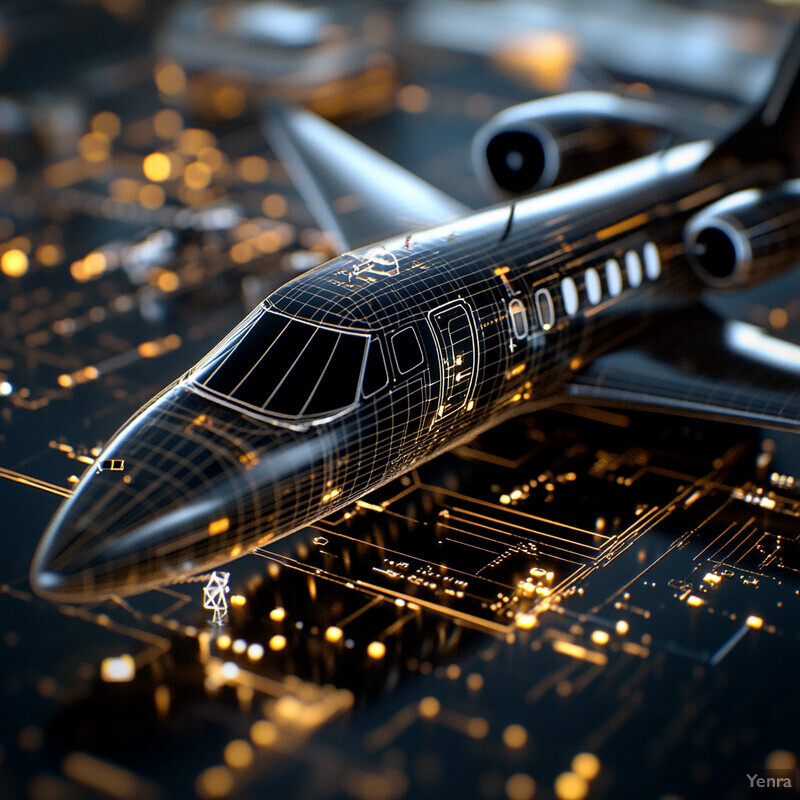
CFD simulations require fine-grained computational meshes, but refining the entire domain uniformly is expensive. AI guides adaptive mesh refinement by identifying flow regions where increased resolution will have the greatest impact on accuracy. By targeting areas of high velocity gradients, turbulent structures, or shocks, AI-based strategies ensure that computational resources are used judiciously. The result is more accurate simulations achieved with less computational cost, facilitating the exploration of more designs in less time. This tailored approach elevates the fidelity of aerodynamic predictions while maintaining manageable simulation runtimes.
12. GAN-based Generative Design
Generative Adversarial Networks produce a wide array of aerodynamic shapes, broadening the design space exploration and enabling breakthrough configurations.
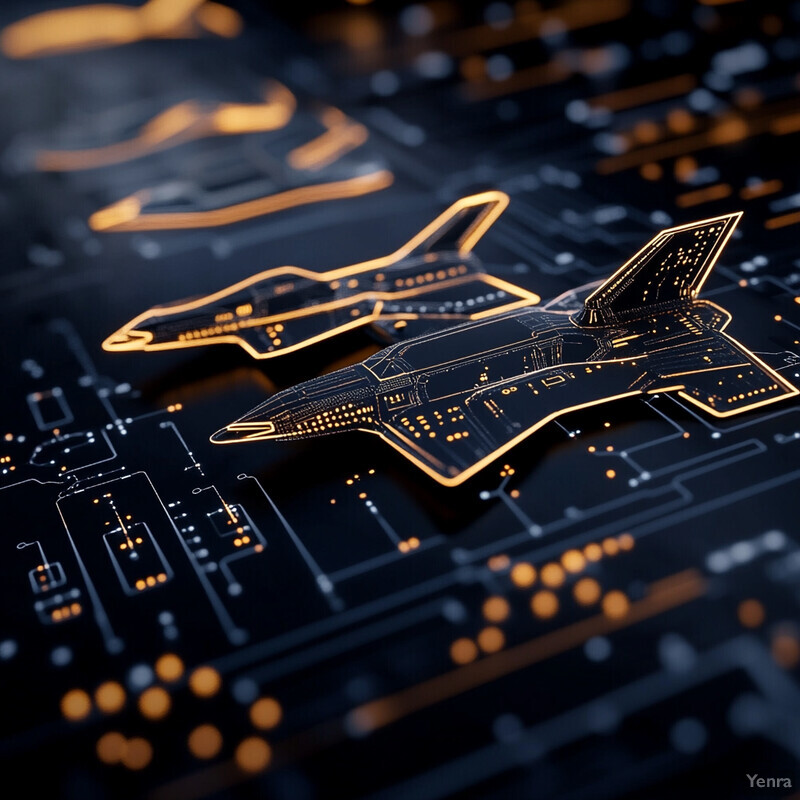
Generative Adversarial Networks (GANs), known for their creative outputs in fields like image synthesis, are now unlocking innovative aerodynamic shapes. After being trained on a large dataset of aerodynamic configurations and performance outcomes, GANs can generate entirely new geometries that satisfy certain performance criteria. This breaks free from conventional design heuristics, potentially discovering unexpected, high-performing shapes. Engineers can use these shapes as starting points for further refinement or as inspiration for unconventional solutions. GAN-based generative design accelerates the ideation phase, enabling a broader, more imaginative exploration of aerodynamic possibilities.
13. Predictive Maintenance for Aero Components
Machine learning analyzes aerodynamic loads and stress patterns to predict component fatigue and maintenance needs, maintaining peak aerodynamic efficiency over time.
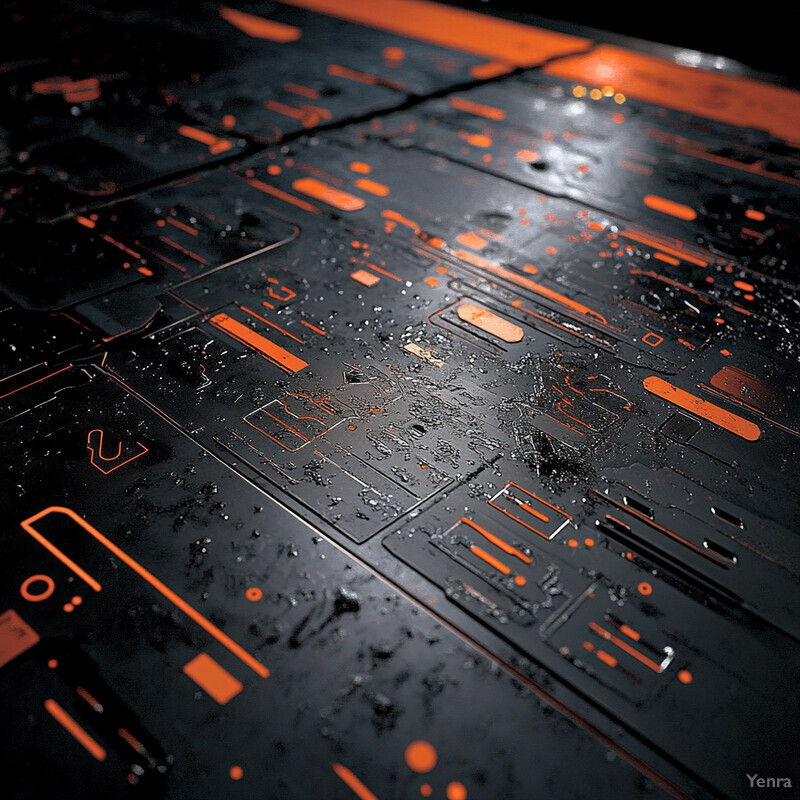
Aerodynamic performance is not static; it can degrade as components wear out, accumulate debris, or suffer minor damage over time. AI-powered predictive maintenance tracks performance metrics, flight data, and sensor information to forecast when parts will need servicing or replacement. By correlating aerodynamic loads with historical maintenance actions, machine learning models can predict when a winglet, flap track, or engine inlet may begin to compromise efficiency. Addressing issues proactively ensures that aerodynamic performance remains optimal throughout the aircraft’s life, reduces downtime, and prevents costly component failures. This leads to safer, more reliable operations and improved overall cost-effectiveness.
14. Real-Time Optimization in Flight
Onboard AI systems can continuously adjust trim, flap positions, and other aerodynamic parameters to maintain optimal flight efficiency as conditions change.
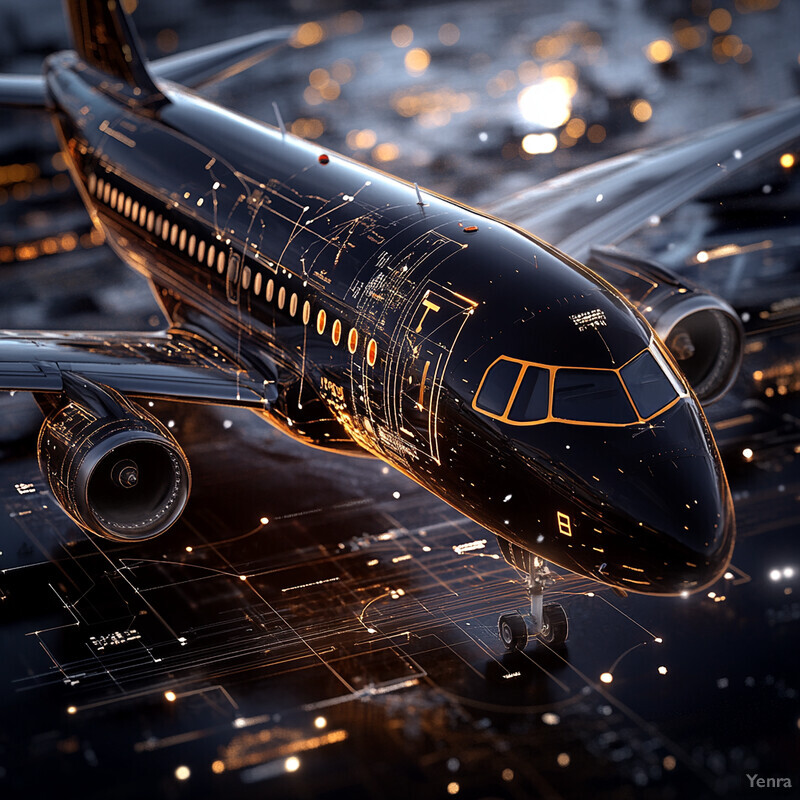
AI doesn’t just optimize designs before they reach the sky; it can also enhance aerodynamic efficiency during flight. By monitoring conditions like air density, angle of attack, and side-slip angle in real-time, onboard AI systems dynamically adjust aerodynamic surfaces for peak efficiency. Rather than relying on static configurations optimized for nominal conditions, the aircraft can adapt continuously, saving fuel and maintaining stable, efficient flight. This capability is particularly valuable in changing weather, varying payload distributions, or different mission segments. Real-time optimization introduces a level of adaptability and responsiveness that was previously unattainable, pushing aerodynamic efficiency to new heights.
15. Improved Boundary Layer Analysis
Deep learning techniques predict boundary layer transitions and separation points more accurately, leading to better flow control strategies.
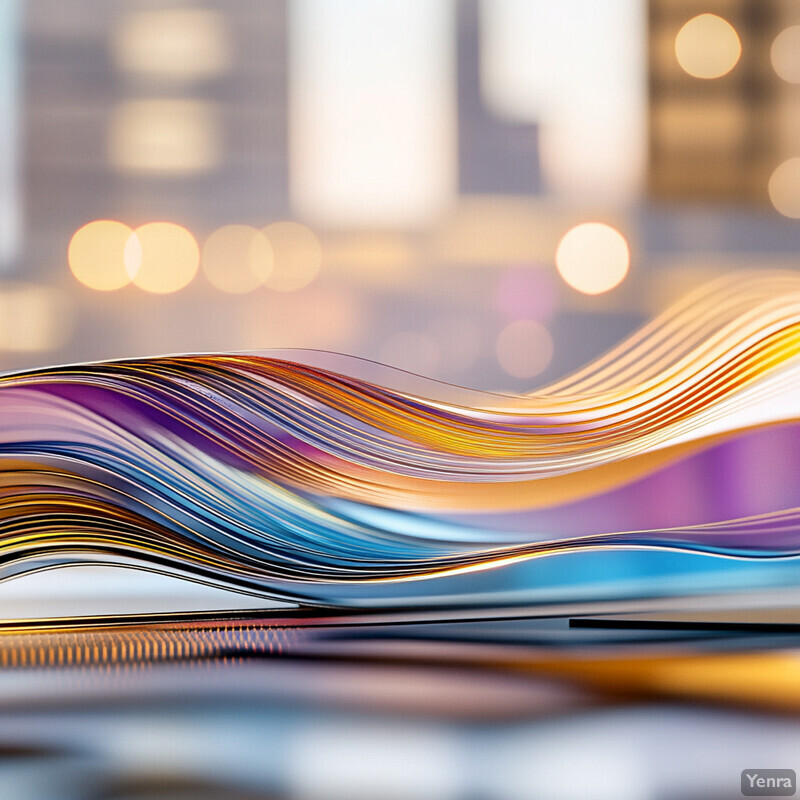
Boundary layers have a profound effect on aerodynamic behavior, influencing drag, flow separation, and lift. AI-based models can predict boundary layer development, transition, and separation points more accurately than traditional correlations. By learning from high-fidelity simulation or experimental data, these models capture subtle nuances in the flow that simpler methods might miss. With better boundary layer predictions, engineers can implement targeted flow control strategies—like surface roughness modifications, suction, or blowing—to maintain laminar flow or delay separation. Improved boundary layer management translates directly into enhanced aerodynamic efficiency, lower drag, and higher performance.
16. Hybrid High-Fidelity/Low-Fidelity Modeling
AI helps blend cheap but less accurate simulations with a small number of expensive high-fidelity CFD runs to achieve both speed and precision in aerodynamic design.
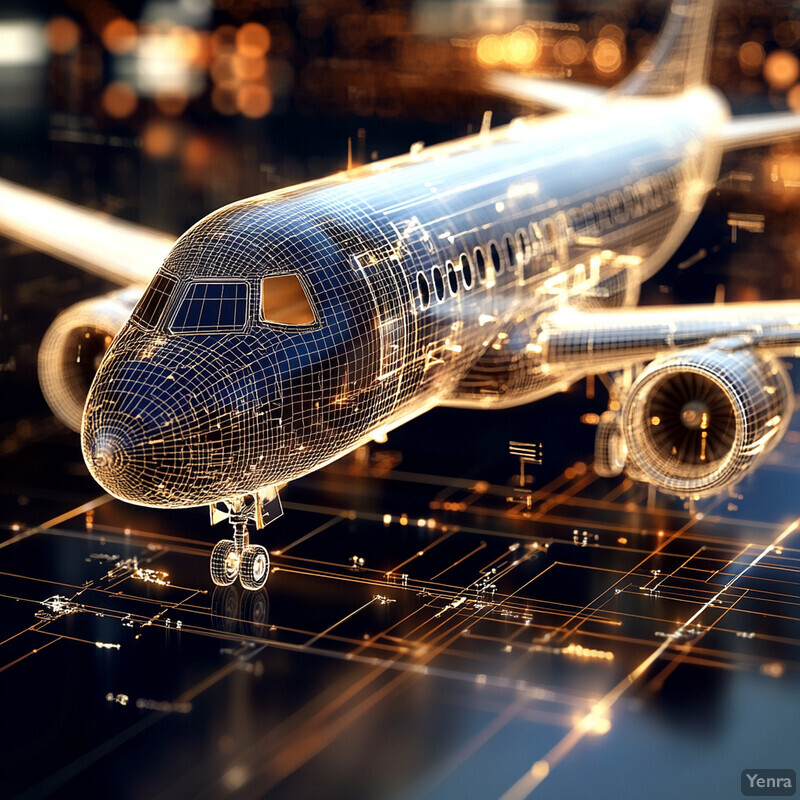
Balancing simulation accuracy and cost has long been a challenge in aerodynamic design. AI enhances hybrid modeling approaches that combine a few high-fidelity CFD simulations with numerous inexpensive low-fidelity evaluations. Machine learning techniques intelligently map corrections between these fidelity levels, ensuring that inexpensive models retain essential physical fidelity. This fusion enables designers to quickly explore large design spaces while still achieving near-high-fidelity accuracy for critical configurations. By bridging the gap between speed and precision, hybrid modeling improves the practicality and thoroughness of aerodynamic optimization efforts.
17. Data-Driven Aero-Load Estimation
Machine learning tools estimate aerodynamic loads on various aircraft surfaces quickly, assisting in real-time decision-making and automated design loops.
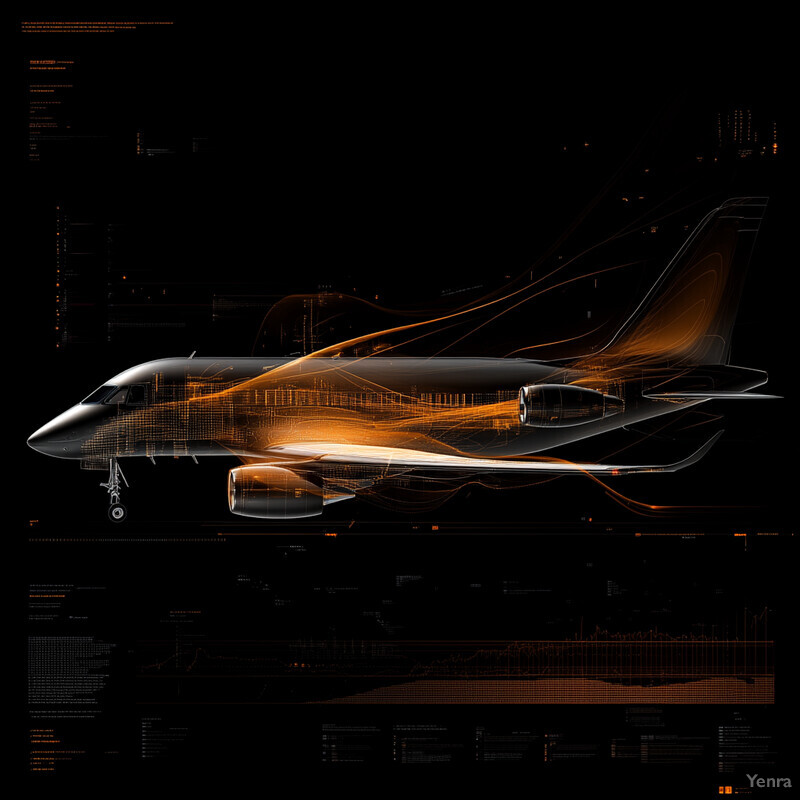
Accurately estimating aerodynamic loads is crucial for structural design, safety analyses, and performance optimization. AI-driven models use operational data, flight test measurements, and simulation outputs to produce accurate load estimates in various conditions. Machine learning excels at identifying complex patterns and correlations, often yielding better predictions than traditional methods. With improved load estimation, engineers can fine-tune structural materials, thicknesses, and component geometry to reduce weight while maintaining safety margins. The result is streamlined structures, improved fuel efficiency, and lower manufacturing costs—key advantages in a competitive industry.
18. Shock Wave Control
AI-based optimization fine-tunes shapes to delay or soften shock formation in transonic and supersonic regimes, boosting aerodynamic efficiency and performance.
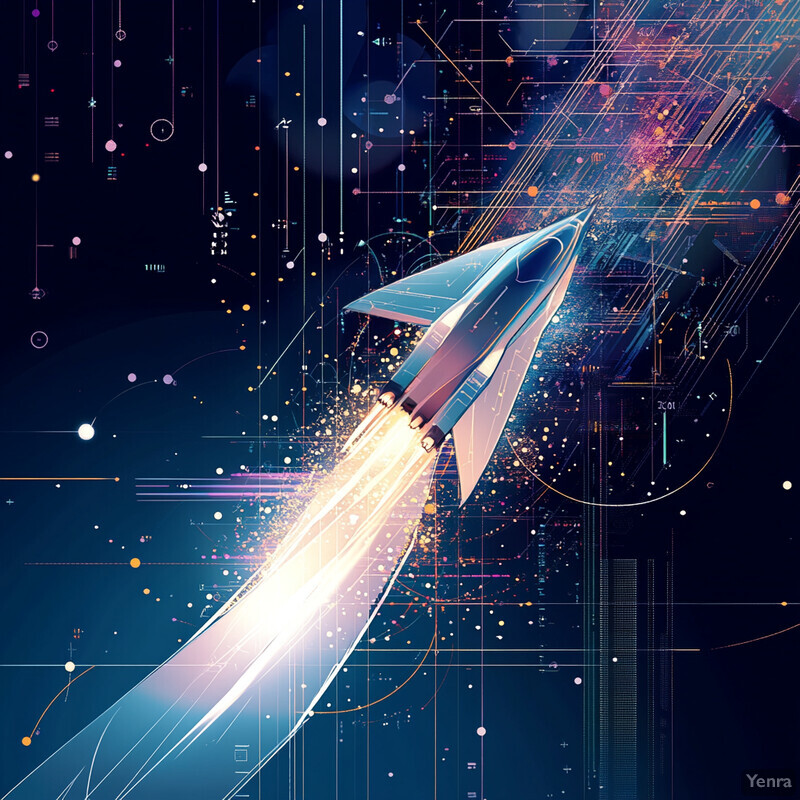
At transonic and supersonic speeds, shock waves can drastically increase drag, reduce efficiency, and cause structural concerns. AI optimization helps designers fine-tune airfoil shapes, fuselage contours, or wing sweep angles to mitigate these effects. Machine learning models trained on computational and experimental data learn the subtle geometric changes that delay shock formation or reduce shock intensity. By diminishing shock-induced pressure spikes, aircraft can fly faster and more efficiently, unlocking new performance envelopes. Shock wave control enabled by AI paves the way for next-generation supersonic transports and hypersonic vehicles.
19. Automated Shape Parametrization
Intelligent algorithms determine the best way to represent complex geometries with minimal parameters, making subsequent aerodynamic optimization more efficient.
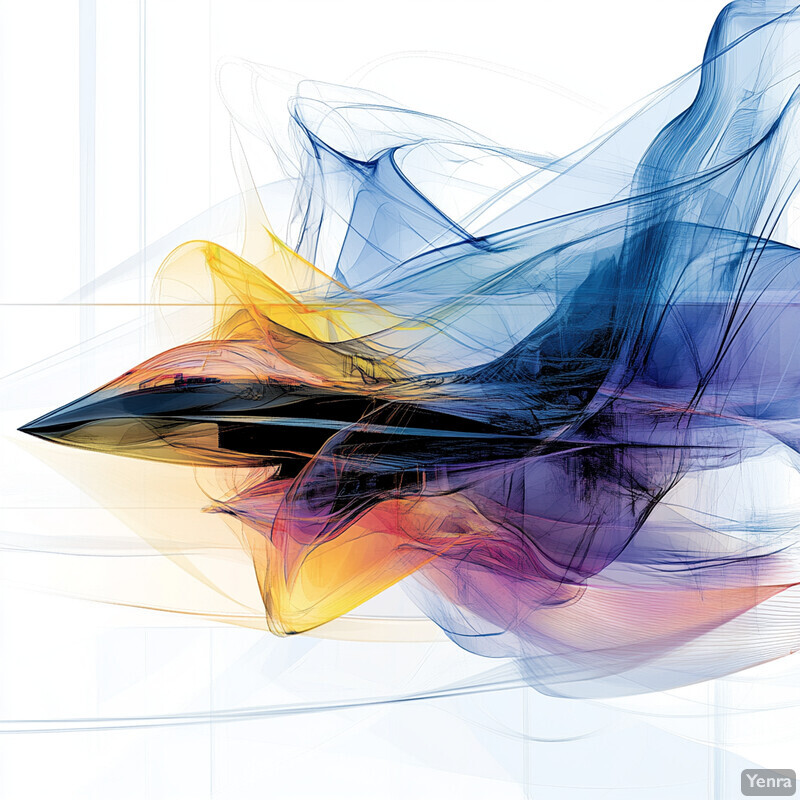
The success of an optimization process often depends on how well a shape is parameterized. AI can automate the discovery of an effective parametrization, identifying a minimal set of parameters that still capture all essential geometric features. With fewer, more meaningful parameters to adjust, optimization algorithms search the design space more effectively and converge faster. Additionally, intelligent parameterization can improve the stability of optimization routines and simplify data handling. Automated parameterization ensures that the aerodynamic optimization process is more efficient, accurate, and scalable across a wide range of applications.
20. Multi-Objective Optimization and Trade-Offs
By simultaneously considering lift-to-drag ratio, stability, noise, and fuel consumption, AI identifies trade-offs and finds balanced designs that meet various performance targets.
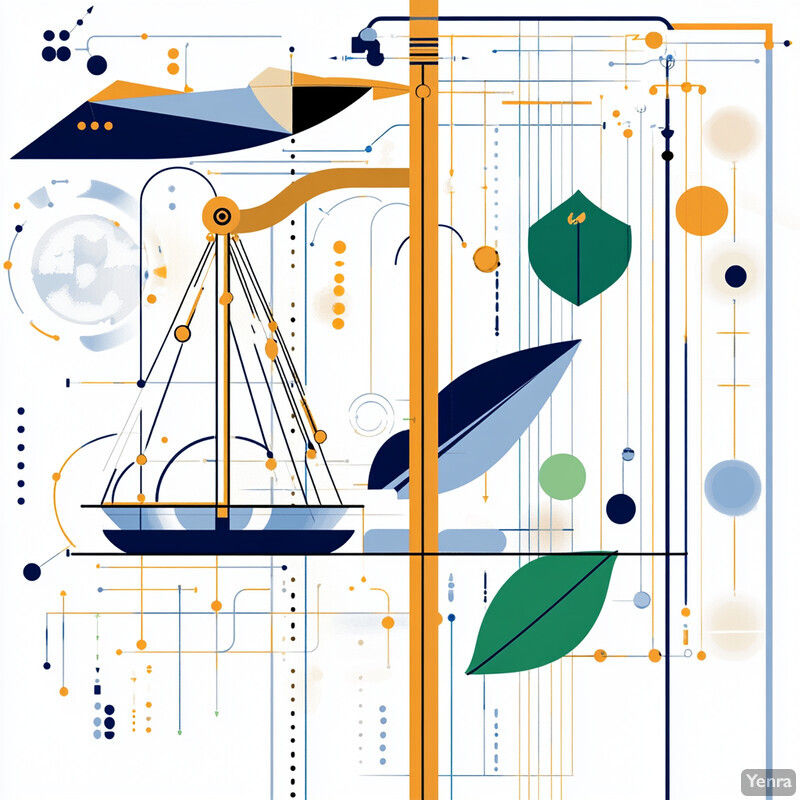
Aerodynamic performance is rarely the only goal; engineers must also consider factors like fuel efficiency, noise pollution, structural weight, and manufacturing costs. AI excels at multi-objective optimization, using methods like Pareto front generation to highlight the trade-offs between competing criteria. By analyzing these trade-offs, designers can identify designs that strike the best balance among various requirements. This comprehensive approach ensures that aerodynamic gains don’t come at the expense of other critical performance metrics. Ultimately, AI-driven multi-objective optimization fosters well-rounded, practical solutions that are ready to meet real-world demands.
Aerodynamic Optimization Song Lyrics
(Intro)
Yo, we talkin’ aerodynamics, future’s now, get it right
AI takin’ flight, no guessin’, just insight
(Verse 1)
I’m automatin’ shapes, I’m cuttin’ down the drag
Refine that wing design, leave the old ways in the bag
With surrogate models for CFD, we skip the heavy load
Fast predictions on the fly, efficiency in code
Reduced-order flows got that complexity on lock
Slimmed down physics, speedin’ up the clock
Turbulence tamed by neural nets, smoothin’ out the ride
Predictin’ where them eddies form, no secrets to hide
(Chorus)
AI in the sky, pushin’ lift to new highs
From every angle we optimize, no compromise
From shapes to shock waves, we keep it precise
20 ways to break through, you can see it in our eyes
(Verse 2)
Inverse design techniques, start with what we need
We say “gimme top performance,” AI plants the seed
Multidisciplinary flows, structure and aero aligned
Unified solutions, leave inefficiencies behind
Reinforcement learnin’ on them flaps and ailerons
Adaptive like nature, never stuck in bygone songs
Uncertainty quantified, we keep it robust
No matter conditions, our jet you can trust
(Chorus)
AI in the sky, pushin’ lift to new highs
From every angle we optimize, no compromise
From shapes to shock waves, we keep it precise
20 ways to break through, you can see it in our eyes
(Verse 3)
Bayesian optimization, we refine the quest
Huntin’ that sweet spot, never settlin’ for less
Real-time wind tunnel reads, data flows on the fly
Adjustin’ as we learn, no design left dry
Adaptive mesh refinement, focus where it counts
Zoomin’ in on crucial zones, keepin’ flow paramount
GAN-based designs from a neural dream
Shapes never seen, on that next-gen team
(Bridge)
Predictive maintenance keepin’ parts on point
No downtime wasted, we stay anointed
Real-time flight optimization, conditions can’t surprise
Your plane so efficient, watch the fuel minimize
Boundary layers tracked, no slip can hide
We feed the model data, results bona fide
Hybrid fidelity, blend high and low
Cost-effective accuracy, watch it all flow
(Verse 4)
Data-driven load estimates keep safety real
Optimize structures, lighten that steel
Shock waves? Controlled, we keep it smooth at speed
Softening sonic booms, fulfilling new needs
Automated parametrization, fewer variables to tweak
Streamlined design loops, solutions unique
Multi-objective moves, trade-offs in mind
Environmental, structural, cost: perfectly aligned
(Chorus)
AI in the sky, pushin’ lift to new highs
From every angle we optimize, no compromise
From shapes to shock waves, we keep it precise
20 ways to break through, you can see it in our eyes
(Outro)
From the lab to the runway, we advanced that game
Aero feats, world elite, remember the name
AI on the rise, aerodynamic dreams realized
20 paths now open, let the future be synthesized.