Song: Aerial Imagery Land Management
1. High-Resolution Land Classification
AI-driven image analysis algorithms leverage machine learning models to categorize land cover types at a granular scale—distinguishing among forests, croplands, water bodies, urban areas, and more. This enhanced classification accuracy helps land managers make better decisions related to zoning, agriculture, and conservation efforts.
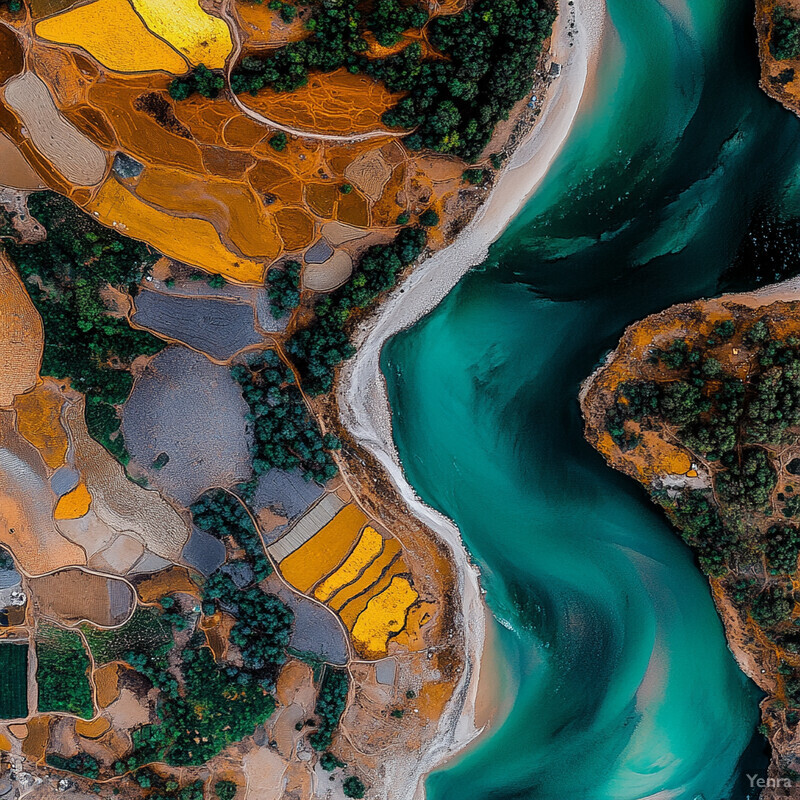
AI systems have revolutionized the way aerial imagery is processed by applying advanced machine learning techniques to distinguish subtle differences in land cover. These algorithms can identify a diverse range of features—such as forests, farmland, water bodies, urban infrastructure, and barren land—at extremely high resolutions. By training on large annotated datasets, the models learn to recognize patterns in texture, color, reflectance, and shape. Once deployed, they can rapidly and consistently classify extensive areas, providing more accurate land cover maps than traditional manual methods. This level of detail helps land managers and policymakers to understand current land use patterns, plan zoning regulations, prioritize conservation areas, and guide sustainable development strategies.
2. Automated Change Detection
By comparing historical and current aerial imagery, AI can rapidly identify changes in land use, vegetation health, infrastructure expansions, and erosion patterns. Automated change detection allows for timely interventions and more adaptive management strategies.
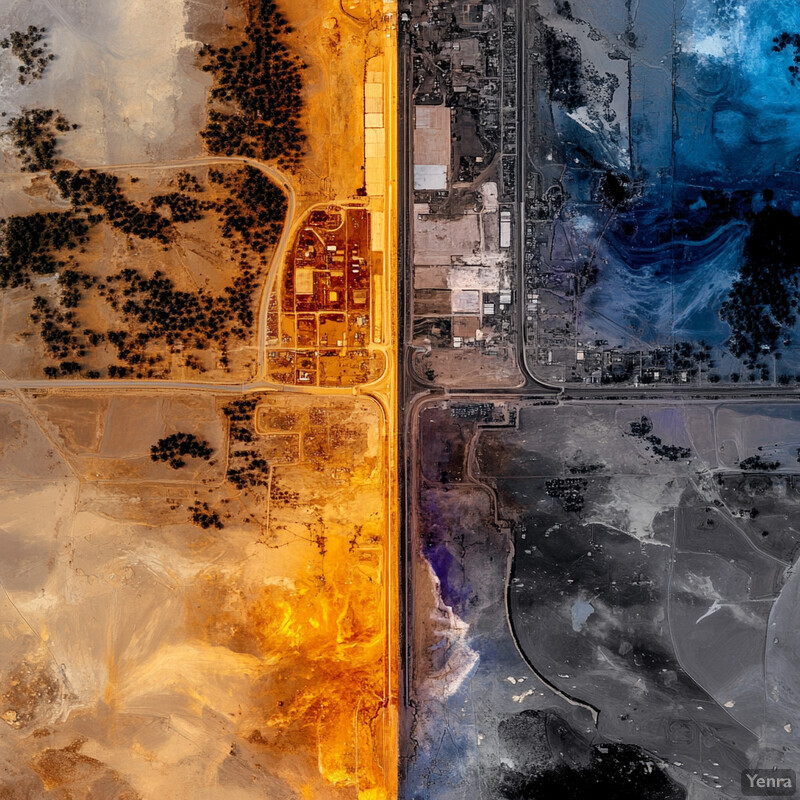
Change detection relies on comparing historical aerial photographs or satellite images with newly acquired imagery to determine what has evolved over time. AI-driven models use image differencing, deep learning segmentation, and object-based detection to highlight even minor alterations in landscape features. This includes infrastructure growth, deforestation, shifting river paths, and the advance or retreat of coastlines. By automating the process, land managers no longer need to spend countless hours manually comparing images. Instead, they receive timely alerts about significant changes, enabling quick responses—such as adjusting management plans, enforcing regulatory compliance, or responding to environmental threats.
3. Precision Agriculture
Machine learning models can analyze aerial imagery of farmland to identify crop stress, nutrient deficiencies, pest infestations, and weed encroachments. This enables more targeted use of inputs, such as fertilizer or pesticides, and leads to improved yields and sustainability.
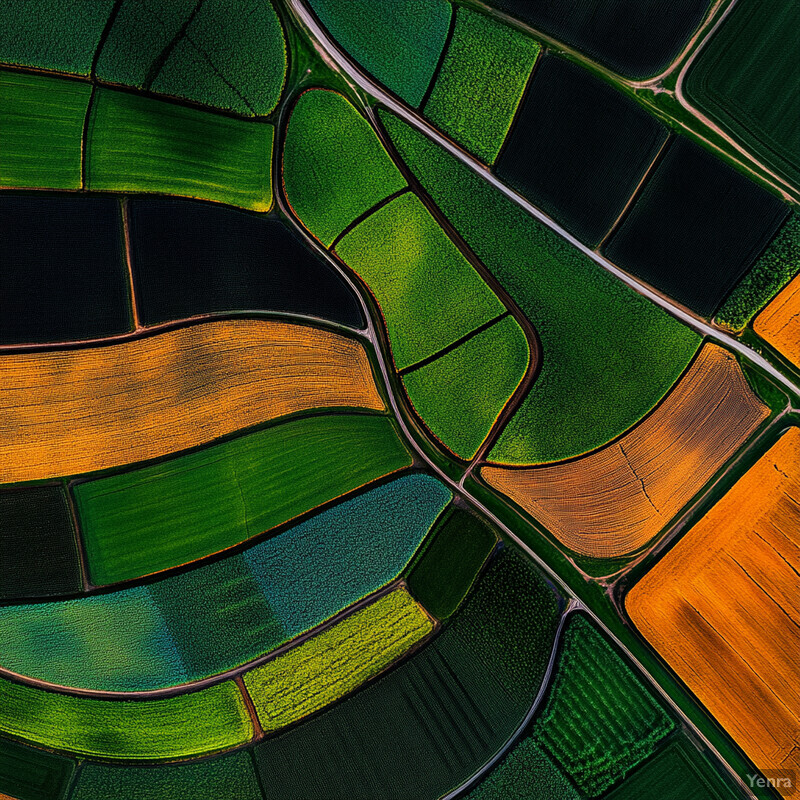
In agriculture, AI’s integration into aerial imaging technology enhances yield, reduces resource wastage, and promotes sustainable farming practices. Machine learning algorithms can identify crop health stressors like nutrient deficiencies, water stress, pest infestations, and weed encroachment. By analyzing spectral signatures, canopy patterns, and thermal data, these systems guide farmers and agronomists to apply fertilizers, pesticides, or irrigation precisely where needed. This precision reduces input costs, mitigates environmental harm, and ensures healthier, more uniform crop growth. Over time, farmers gain deeper insights into their fields, improving productivity and resilience against climate change.
4. Vegetation Health Monitoring
Advanced computer vision techniques can process multispectral and hyperspectral imagery to assess plant health and canopy density. Identifying stressed or diseased plants early helps land managers mitigate damage and maintain ecosystem stability.
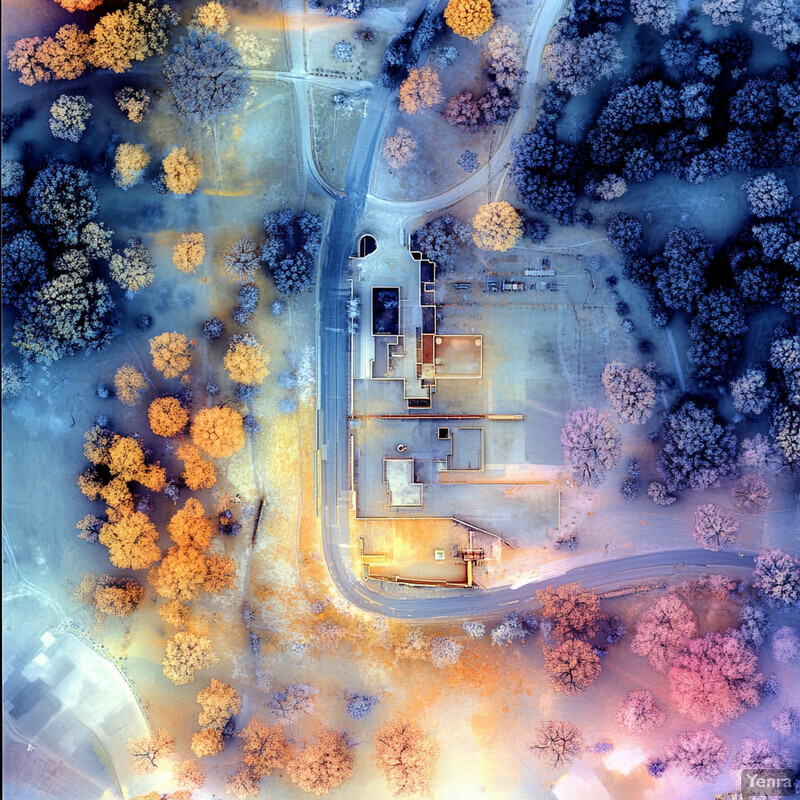
Multispectral and hyperspectral aerial imagery provides valuable data on plant health by capturing wavelengths of light that the human eye cannot see. AI models analyze these spectral bands to detect early signs of plant stress, nutrient deficiencies, or disease infestations. By interpreting subtle variations in leaf pigmentation, canopy density, and reflectance patterns, AI enables early intervention before a problem spreads. Land managers use these insights to enhance biodiversity, maintain ecosystem balance, and support informed decision-making in forestry, agriculture, and conservation settings, ultimately ensuring healthier landscapes that can support both wildlife and human communities.
5. Soil Moisture and Irrigation Planning
AI can extract valuable information about soil moisture content from imagery by analyzing ground reflectance and thermal signatures. This helps optimize irrigation schedules and water resource management, reducing both costs and environmental impact.
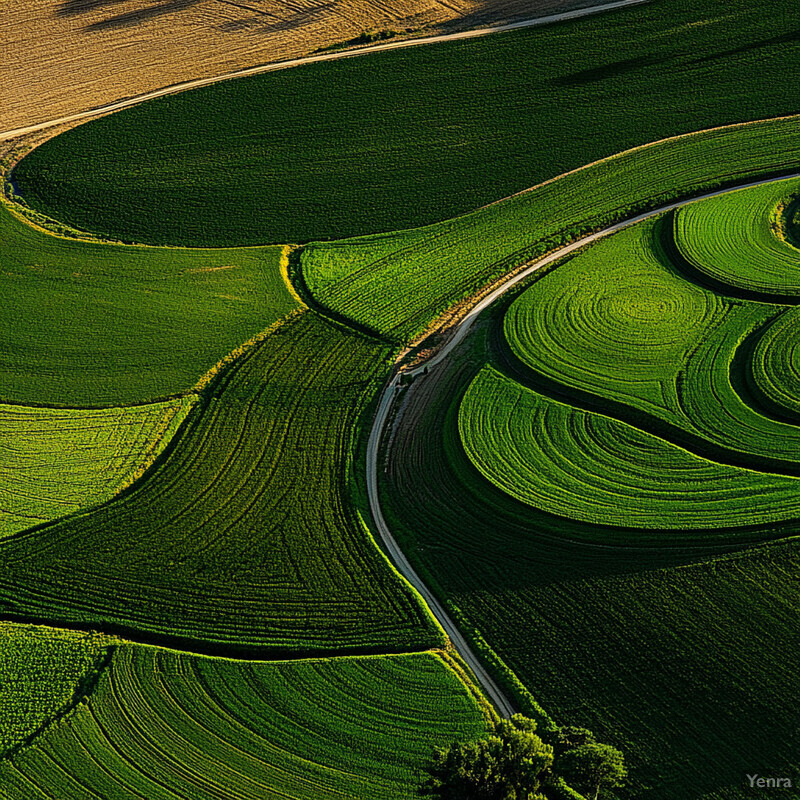
Aerial imagery, combined with thermal infrared sensors and machine learning algorithms, can estimate soil moisture levels across wide expanses of land. AI models correlate surface temperature fluctuations, vegetation indices, and topographical features to provide accurate, large-scale soil moisture maps. These maps guide farmers and land managers in optimizing irrigation scheduling and coverage, ensuring water is used efficiently and sustainably. This approach not only conserves a critical resource but also reduces energy costs related to pumping water, and helps maintain crop health, improving yields and profitability.
6. Wildfire Risk Assessment and Management
Deep learning models can interpret patterns in vegetation density, dryness levels, and historical burn scars to predict areas at high risk of wildfire. Proactive management, such as controlled burns or vegetation thinning, can then be implemented to prevent catastrophic fires.
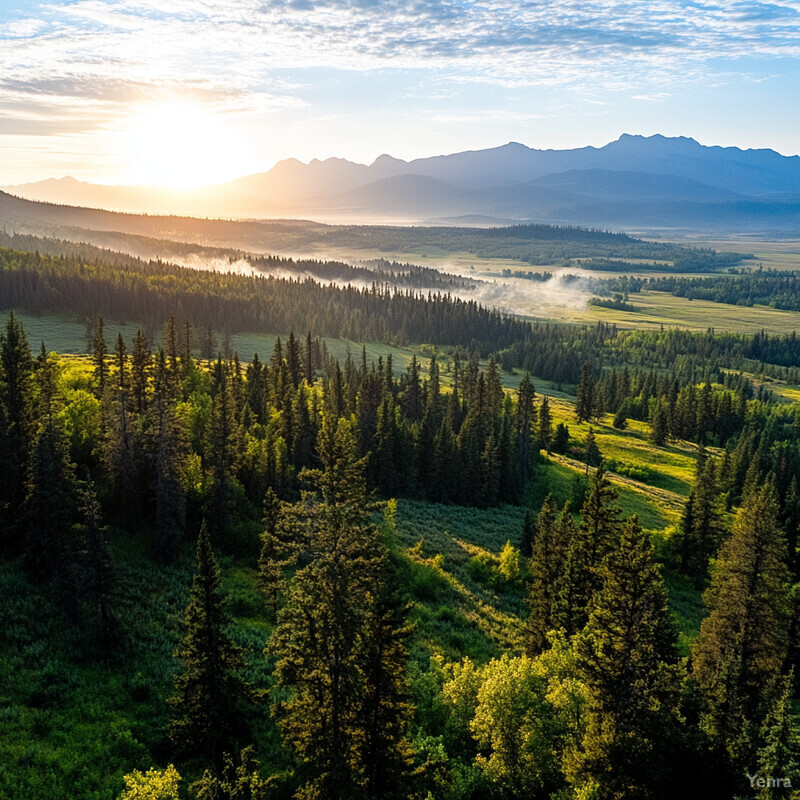
AI-driven analysis of aerial imagery plays a crucial role in preventing catastrophic wildfires. By studying vegetation density, dryness levels, historical burn patterns, and topographical factors, AI models can identify areas of heightened risk. Land managers receive data-driven insights that inform controlled burns, selective thinning of vegetation, and the placement of firebreaks. The early warnings and risk maps produced by these analyses also support emergency services, guiding where to pre-position firefighting resources and how to evacuate at-risk communities. Ultimately, AI-based wildfire risk assessment contributes to more resilient landscapes and safer human settlements.
7. Coastal Erosion and Flooding Analysis
AI can track shoreline changes, sediment deposition, and storm surge patterns in coastal areas. Land managers use this data to implement erosion control measures, fortify coastal infrastructure, and improve flood preparedness.
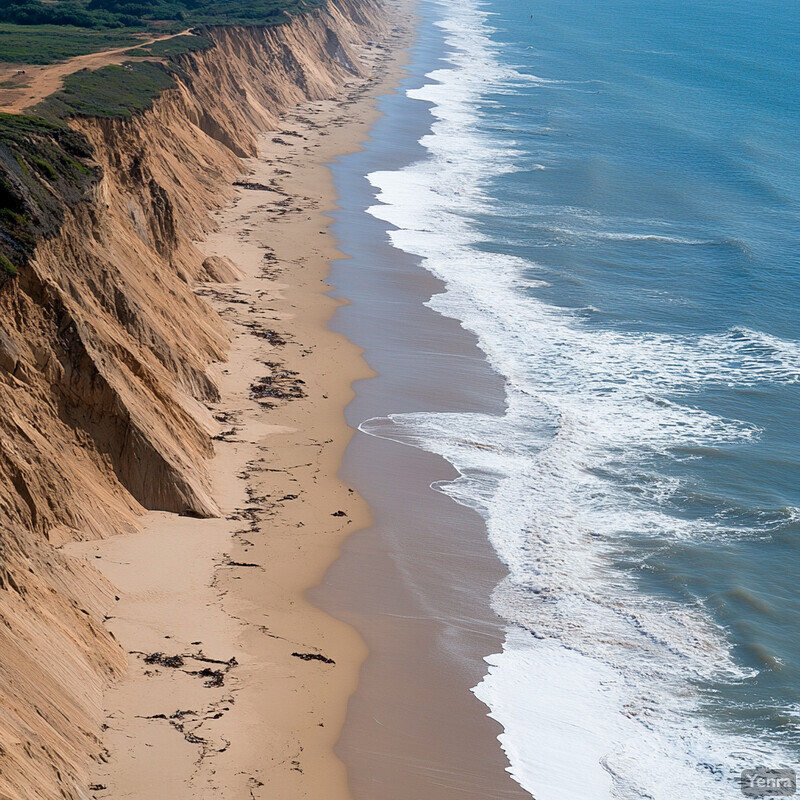
Shorelines are dynamic environments influenced by tides, currents, and storms. AI models process time-series aerial imagery to track changes in coastal features such as beaches, dunes, and wetlands, as well as the migration of sandbars. By understanding how these features shift over time, land managers can predict areas most vulnerable to erosion or flooding due to sea-level rise or severe weather events. This knowledge guides the design of protective measures—like breakwaters, dune restoration projects, and strategic relocation of infrastructure—minimizing damage and preserving valuable coastal ecosystems and economies.
8. Invasive Species Detection
Computer vision models trained on aerial imagery can spot invasive plant species and pests. Early identification allows for rapid response, containment, and restoration, preventing severe economic and ecological damage.
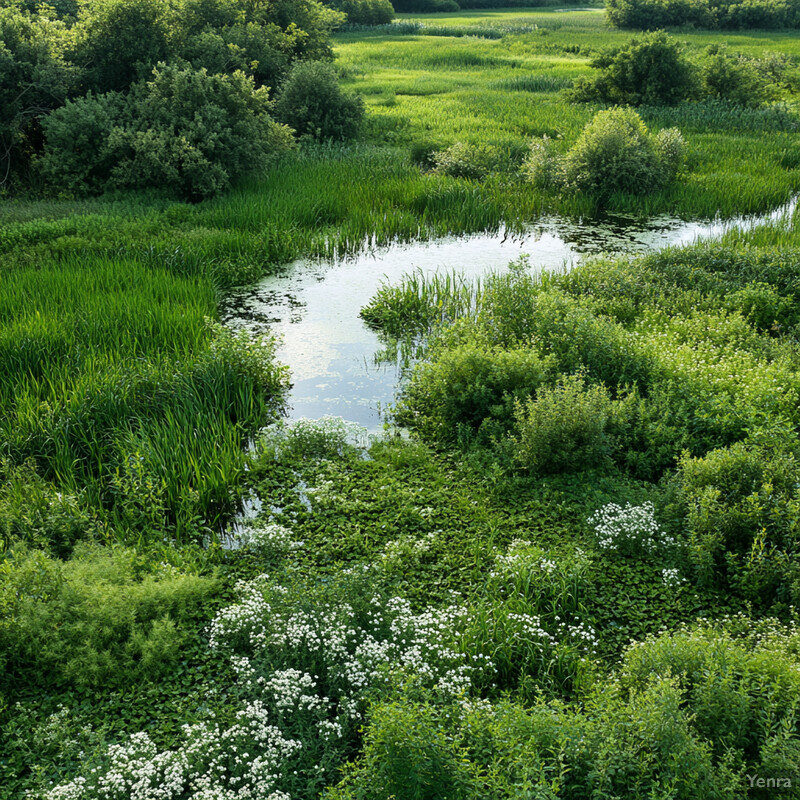
Invasive plant species can dramatically alter local ecosystems by outcompeting native vegetation. AI-driven aerial image analysis helps detect these intruders early by identifying unique spectral signatures, shapes, or canopy textures associated with certain invasive species. Early detection allows for prompt and targeted interventions—herbicide application, mechanical removal, or biological controls—before an infestation becomes unmanageable. This proactive approach saves time and resources, maintains ecological balance, and prevents long-term habitat degradation that can be caused by unchecked invasive species spread.
9. Urban Planning and Infrastructure Monitoring
With AI’s ability to detect buildings, roads, and utilities from aerial imagery, planners can map out expansion areas, identify illegal encroachments, monitor infrastructure health, and streamline resource allocation.
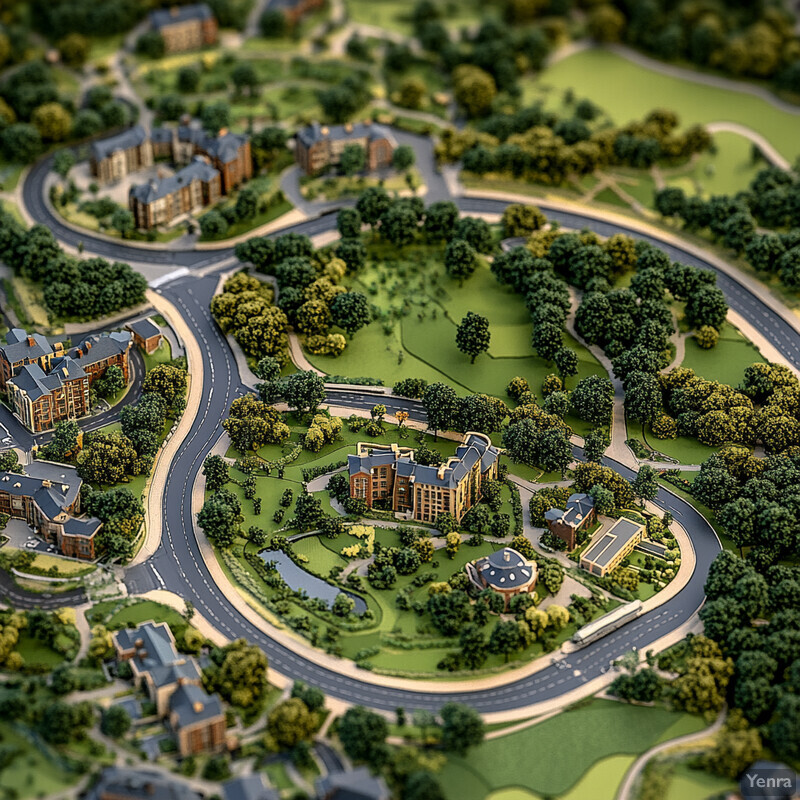
Municipalities and developers rely on current, accurate maps of urban environments to guide growth and infrastructure maintenance. AI models interpret aerial images to identify buildings, roads, utility lines, and construction activities with high precision. By having updated information on urban sprawl, transportation networks, and resource distribution, city planners can optimize zoning decisions, improve traffic management, and ensure public utilities are functioning efficiently. This data also helps monitor illegal encroachments and enforce land-use policies, ensuring orderly development and sustainable urban expansion.
10. Forest Inventory and Biomass Estimation
Aerial images analyzed by AI can estimate tree heights, species composition, and biomass. This data assists in sustainable forest management, carbon accounting, and forestry operations planning.
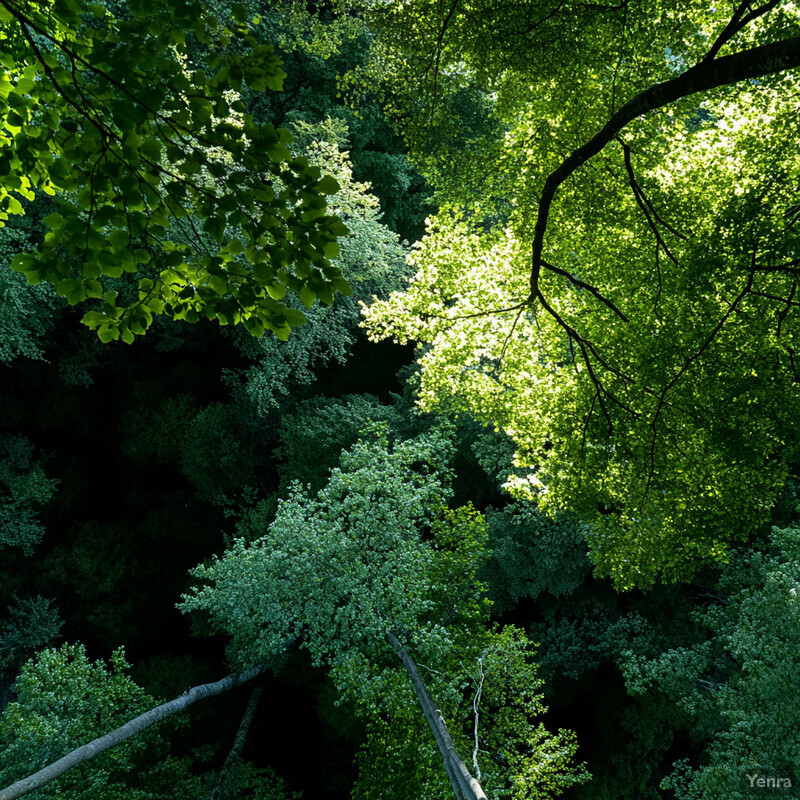
Sustainable forest management depends on understanding forest composition, structure, and health. AI algorithms analyze aerial imagery to estimate the number of trees, their heights, species distribution, and overall forest density. By deriving biomass estimates, land managers can track carbon storage, plan logging activities responsibly, and maintain forest health. Accurate forest inventories support policies that balance economic interests with environmental stewardship, helping ensure that timber harvests remain sustainable and that forests continue to provide critical ecosystem services such as carbon sequestration, wildlife habitats, and soil stabilization.
11. Habitat and Biodiversity Assessment
Aerial images analyzed by AI can estimate tree heights, species composition, and biomass. This data assists in sustainable forest management, carbon accounting, and forestry operations planning.
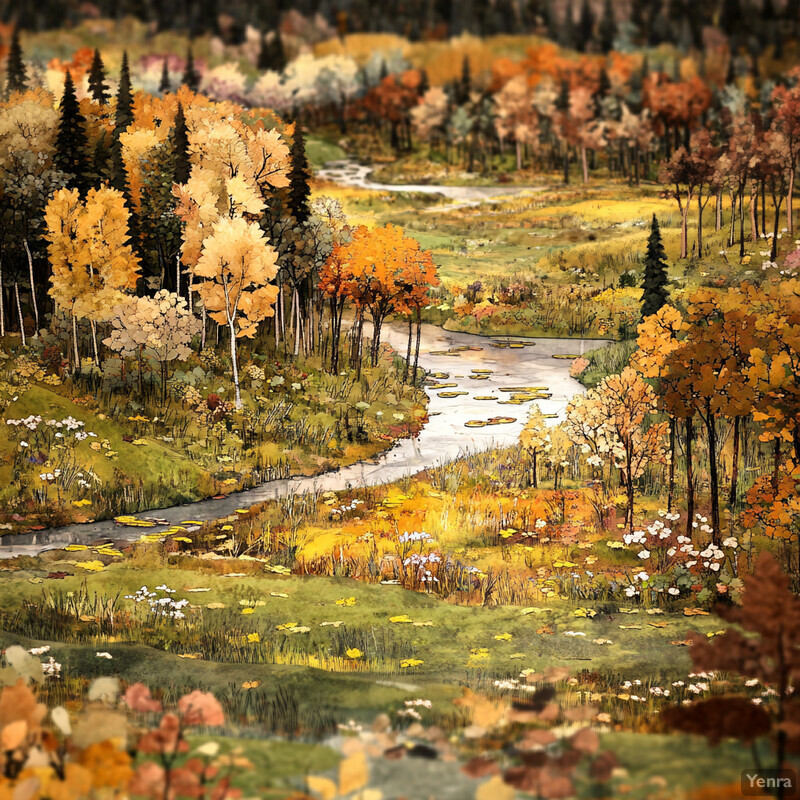
Conserving biodiversity requires detailed knowledge of habitat conditions and species distributions. AI-powered imagery analysis delineates critical habitat features—wetlands, riparian corridors, forest edges, and open grasslands. By correlating these data with known species requirements, land managers can identify and prioritize areas that need conservation or restoration efforts. Detailed habitat assessments enable proactive measures to protect endangered species, maintain ecological connectivity, and enhance overall biodiversity. In turn, these actions support climate resilience, ecosystem stability, and the protection of natural resources that communities rely on.
12. Precision Ranching and Livestock Tracking
Using imagery, AI can identify patterns in grazing areas, water availability, and forage quality. Ranchers and range managers can then optimize pasture rotation, animal distributions, and resource allocation to maintain healthy ecosystems and improve productivity.
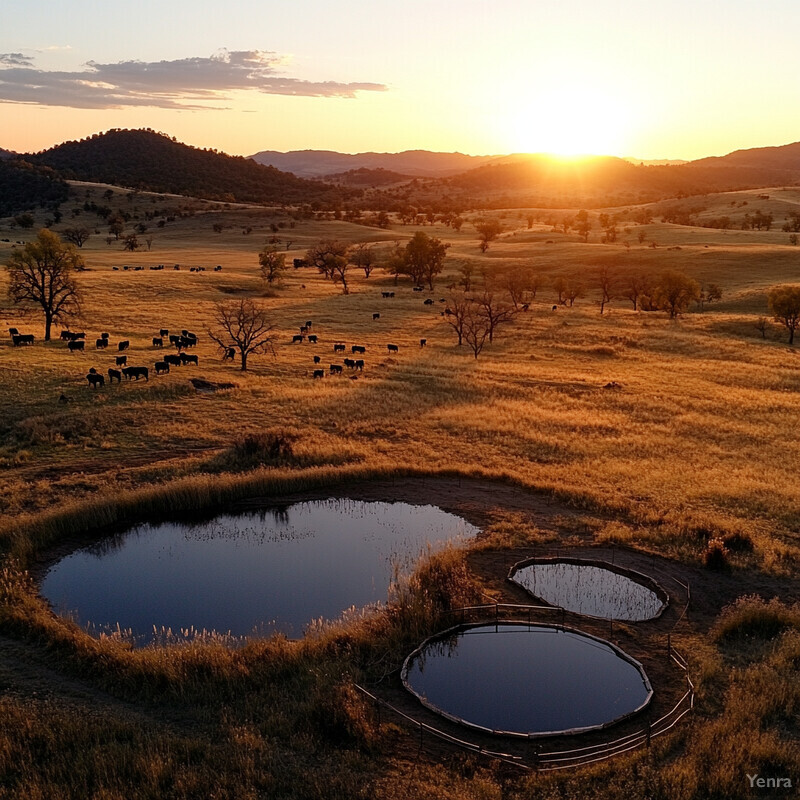
Sustainable pasture and rangeland management benefit from detailed, up-to-date information on forage quality and distribution. AI analyzes aerial imagery to assess vegetation density, classify forage types, and identify water sources. These insights help ranchers optimize grazing rotations, ensuring that livestock have access to adequate nutrition without overgrazing particular areas. By maintaining a balance between livestock needs and grassland health, ranchers reduce soil erosion, preserve plant biodiversity, and improve long-term productivity. AI-driven monitoring also supports decisions on fencing, supplemental feeding, and habitat improvements.
13. Infrastructure Damage Assessment Post-Disaster
After natural disasters, AI can rapidly evaluate damage to buildings, roads, and utility lines. This quick turnaround helps land managers and emergency responders prioritize recovery efforts and optimize resource deployments.
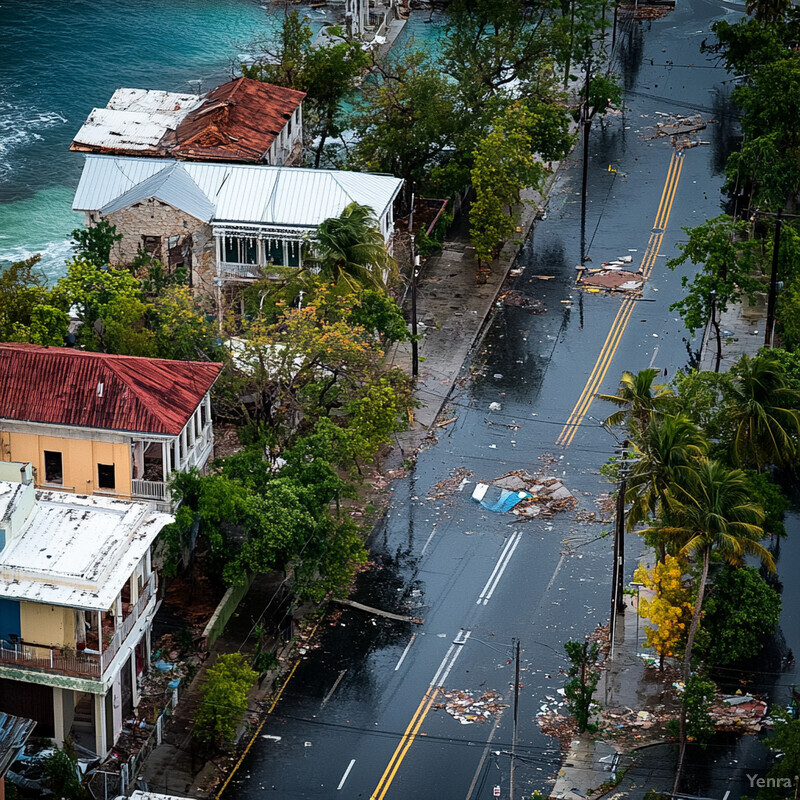
After hurricanes, earthquakes, floods, or wildfires, rapid infrastructure damage assessment is critical for effective emergency response. AI models can process post-disaster aerial imagery quickly to detect damaged buildings, compromised roads, downed power lines, and other hazards. By prioritizing the most affected areas, responders can streamline rescue operations, allocate resources efficiently, and plan rebuilding efforts more intelligently. This immediate situational awareness significantly reduces the time and cost associated with post-disaster recovery, helping communities return to normalcy as soon as possible.
14. Legal Compliance and Enforcement
AI-driven aerial surveys can verify whether land use activities comply with zoning regulations, conservation easements, or protected area guidelines. Automated alerts help ensure timely enforcement and promote responsible land stewardship.
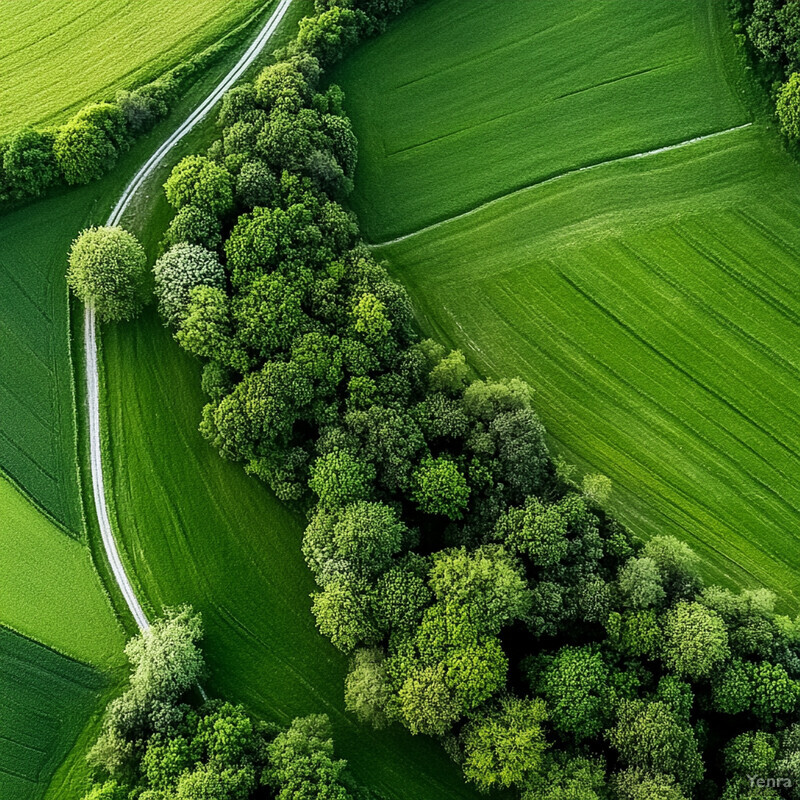
Regulations on land use, environmental protection, and zoning require continuous monitoring. AI-driven solutions provide automated checks on whether new construction projects infringe on protected zones, whether landowners are respecting conservation easements, or if agricultural expansions violate land-use plans. High-resolution imagery combined with AI detection can alert authorities to illegal logging, unauthorized mining, or encroachments into national parks. Timely enforcement backed by such technology not only upholds the law but ensures more responsible land stewardship and long-term sustainability.
15. Soil Erosion and Sedimentation Modeling
By examining landscape features, vegetation cover, and topography from imagery, AI models can predict areas prone to soil erosion. This foresight supports soil conservation measures, watershed management, and infrastructure protection.
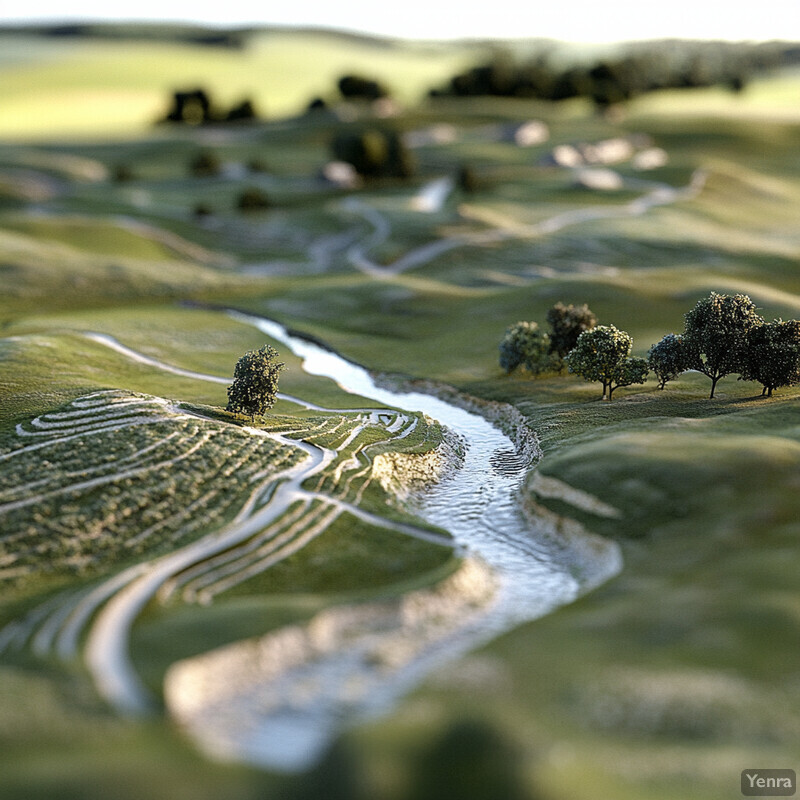
Protecting watersheds and agricultural productivity involves understanding where soil is most prone to erosion. AI models integrate aerial imagery with topographic, climatic, and vegetation data to identify at-risk areas. By spotting patterns—such as sparse vegetation cover on steep slopes—land managers can implement soil conservation measures, like planting cover crops or installing terracing. Reducing erosion helps maintain soil fertility, water quality, and the structural integrity of infrastructure like roads and dams. Over time, these interventions improve the resilience of both agricultural systems and natural ecosystems.
16. Cultural Heritage Site Protection
AI can detect subtle anomalies indicating the presence of archaeological features or cultural heritage sites. Land managers can use this insight to protect these sites during development, infrastructure projects, or conservation initiatives.
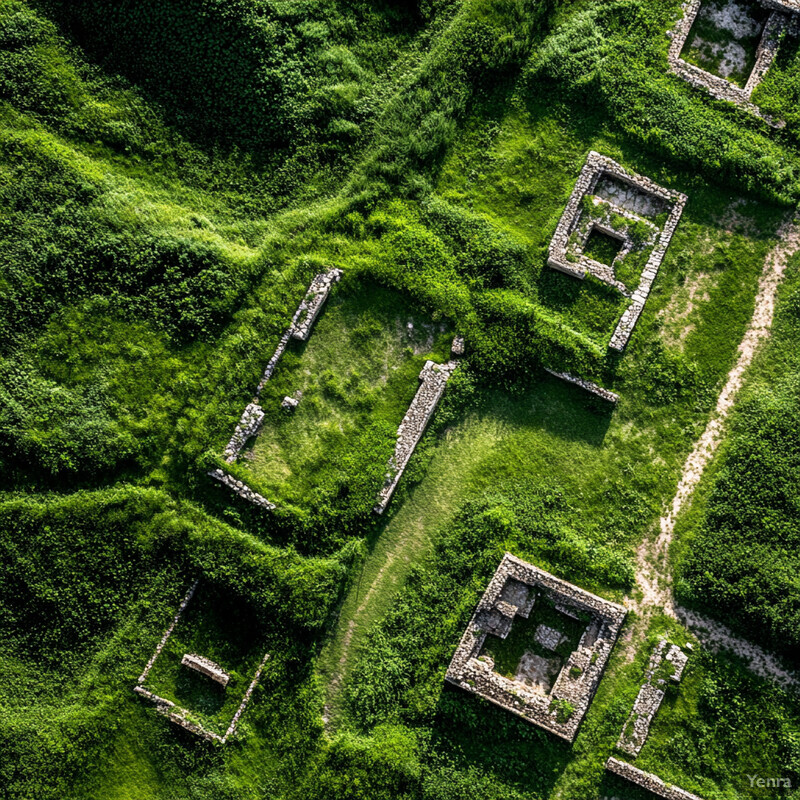
Preserving archaeological sites and cultural landmarks requires careful land use planning and constant monitoring. AI-enhanced imagery analysis can detect subtle indications of buried ruins, historical pathways, or architectural patterns that are not easily visible to the naked eye. With this early detection capability, land managers and archaeologists can protect these sites from inadvertent damage due to construction, agricultural expansion, or industrial activities. Safeguarding cultural heritage ensures that communities retain a connection to their past, while also fostering tourism, educational opportunities, and cultural identity preservation.
17. Carbon Sequestration Monitoring
Aerial imagery analyzed with AI can help quantify vegetation cover and biomass changes over time. Tracking these shifts assists land managers and policymakers in measuring carbon sequestration for climate mitigation efforts and carbon credit markets.
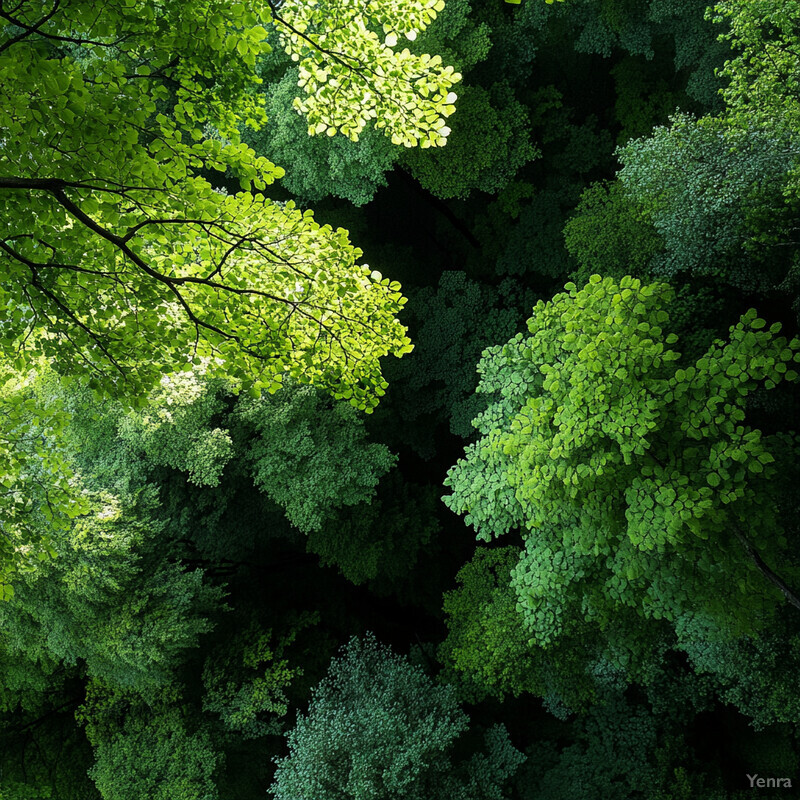
In the era of climate change, understanding and enhancing carbon sequestration in terrestrial ecosystems is paramount. AI helps estimate above-ground biomass and forest density from aerial imagery, tracking changes over time that correspond to carbon storage. Such monitoring guides reforestation, afforestation, and land management policies to increase carbon capture. By translating pixel-level vegetation data into actionable climate metrics, stakeholders can measure progress, optimize carbon credit schemes, and bolster global efforts to mitigate climate change.
18. Mine Reclamation and Environmental Compliance
In industrial areas, AI can evaluate the progress of mine site reclamation, vegetation regrowth, and remediation measures. Automated comparisons against compliance standards help ensure responsible land usage and environmental recovery.
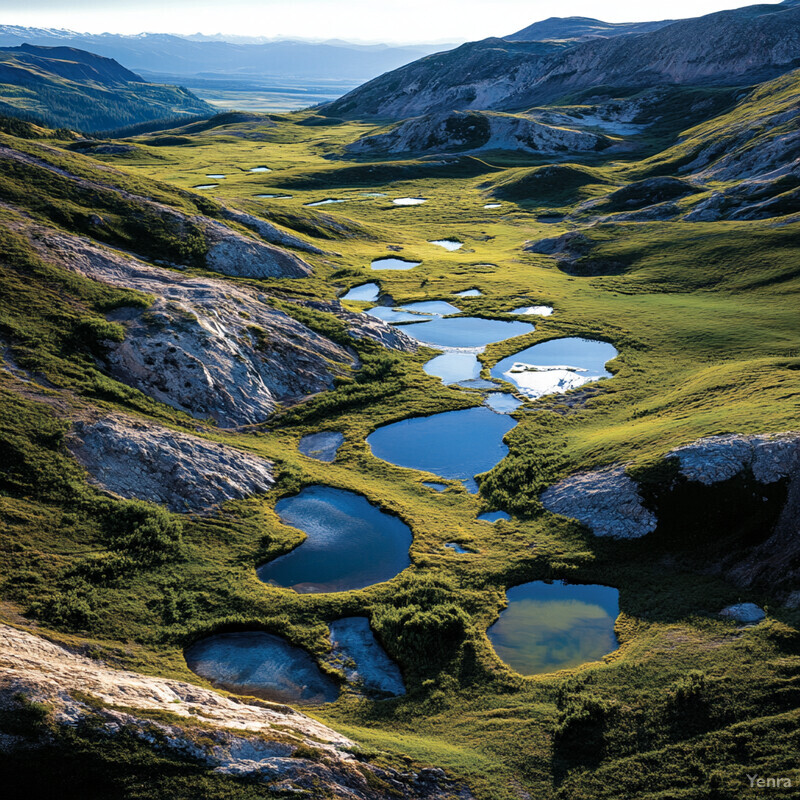
Extractive industries must follow strict environmental guidelines to restore landscapes after mining. AI-equipped aerial monitoring tools provide ongoing assessments of reclamation progress, vegetation reestablishment, and erosion control measures. By comparing before-and-after imagery, regulators and land managers confirm whether mining companies are meeting reclamation targets. This ensures that degraded landscapes are returned to functional ecosystems, helps maintain water quality, and supports habitat for local wildlife, ultimately rebuilding trust between communities, industry, and government entities.
19. 3D Terrain Modeling and Landscape Analysis
AI can turn aerial images into detailed Digital Elevation Models (DEMs) and 3D reconstructions. This helps land managers understand slope stability, watershed boundaries, and access routes, informing infrastructure development and erosion control.
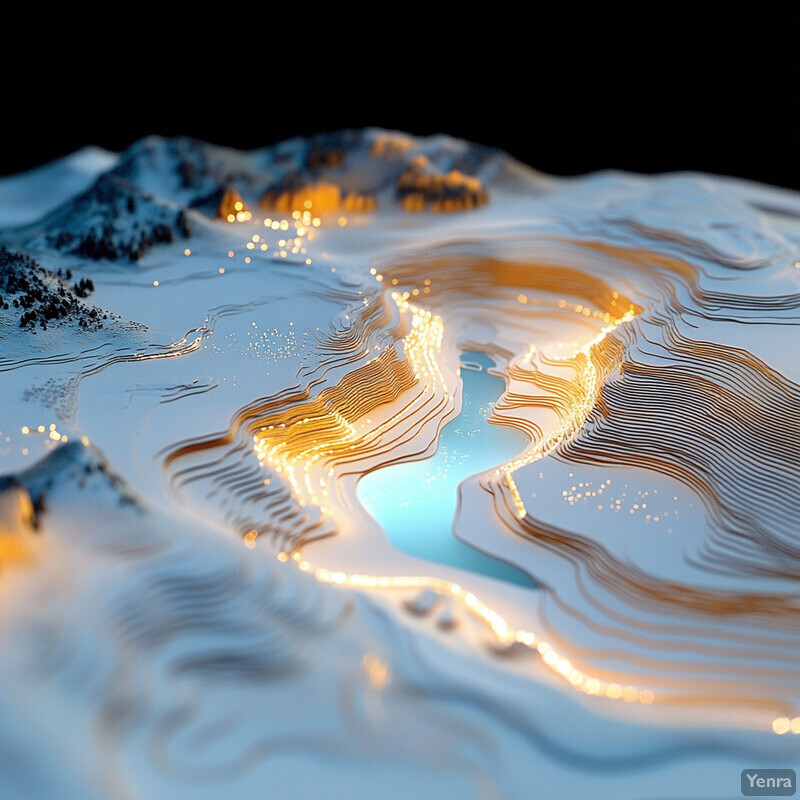
Converting flat, two-dimensional aerial imagery into three-dimensional landscape models is simplified by AI-driven photogrammetry and computer vision. These 3D models capture elevation changes, terrain roughness, and slope aspects, providing critical insights for infrastructure design, watershed management, and erosion control. Planners can visualize how a new dam might alter water flow, where a road should be built to minimize landslides, or how to route trails through a mountainous region safely. The rich detail of 3D analyses enables smarter decision-making, reducing long-term maintenance costs and environmental impacts.
20. Multi-Temporal and Seasonal Pattern Analysis
By aggregating and analyzing imagery across multiple seasons and years, AI can identify long-term trends in land use, vegetation cycles, and climate-induced changes. Such insights support strategic planning, policy formation, and sustainable land management practices.
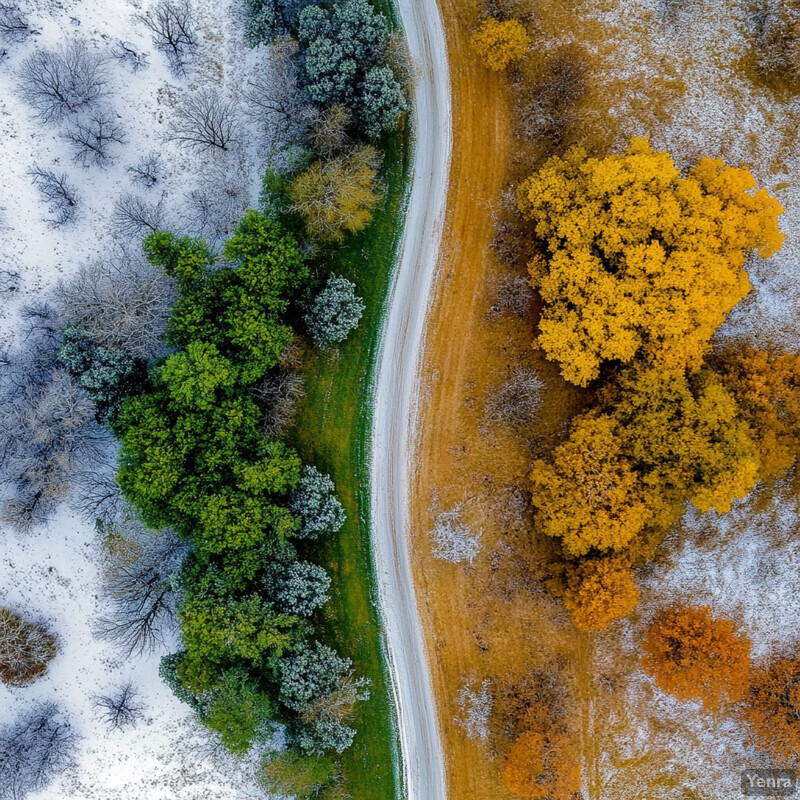
Over years, landscapes shift with changing climates, economic pressures, and policy decisions. AI can integrate long-term aerial image archives, analyzing seasonal and annual variations in vegetation growth, water availability, land cover transitions, and infrastructure development. By identifying these trends, land managers can anticipate future changes, adapt farming methods to shifting rainfall patterns, adjust conservation strategies in response to habitat loss, and plan infrastructure projects that remain resilient under evolving environmental conditions. This forward-looking perspective ensures that land management practices are not just reactive but also strategically aligned with long-term sustainability goals.