Song: Acoustic Engineering and Noise Reduction
1. Adaptive Active Noise Cancellation Systems
Adaptive active noise cancellation (ANC) systems leverage AI algorithms to continuously adjust anti-noise signals in response to changing sound environments. Unlike fixed ANC, adaptive systems monitor noise in real time and update parameters to maintain optimal cancellation across different frequencies and user conditions. This approach helps prevent issues like instability or howling by intelligently tuning feedback and feedforward filters on the fly. AI-powered adaptive ANC can also personalize noise reduction, accounting for individual ear fit and ambient sound direction to ensure consistent performance. Overall, AI adaptation in ANC leads to more robust noise cancellation that works reliably for more users and scenarios than traditional static designs.
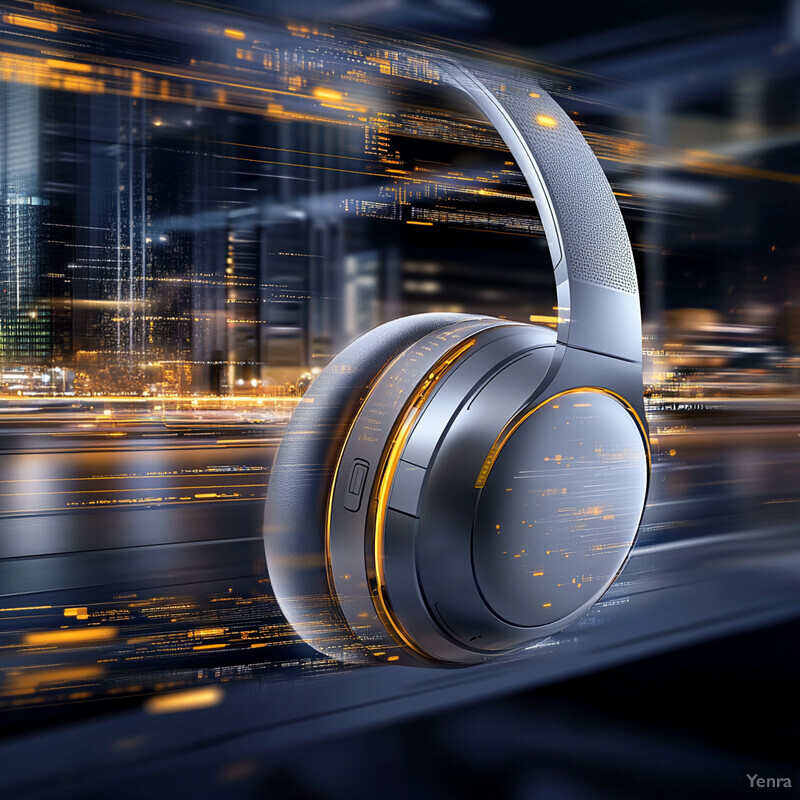
Recent research implemented a deep neural network-based ANC that dynamically selects the best control filters for different noise types, significantly speeding up response. In one system, a convolutional model was trained to choose optimal pre-trained filters, improving real-time noise reduction while maintaining stability in a feedback ANC loopouci.dntb.gov.ua . Reinforcement learning has also been applied to ANC—one 2024 study used a soft actor-critic agent to train a generative ANC filter model that can adapt without extensive labeled data, yielding effective cancellation across varied acoustic paths. These AI-driven approaches outperform fixed ANC: a CNN-based adaptive ANC cut noise by an additional few decibels compared to conventional methods and could adjust to sudden noise profile changes. Researchers note that such adaptive ANC maintains high noise attenuation (e.g. >20 dB) even as the background noise spectrum shifts, something a one-size-fits-all ANC cannot.
2. Data-Driven Acoustic Material Design
AI is transforming the design of acoustic materials by using data-driven algorithms to discover structures with superior sound control properties. Instead of manual trial-and-error, machine learning models (especially deep generative models) can predict how a material’s geometry or composition will affect acoustic absorption, transmission, or resonance. This accelerates the development of acoustic panels, absorbers, and metamaterials that target specific frequency ranges or broadband noise reduction. By training on simulation or experimental data, AI tools identify complex material patterns (like micro-perforations or metamaterial unit cells) that achieve desired acoustic performance while often optimizing for size, weight, or airflow. The result is that engineers can rapidly generate and evaluate new acoustic material designs that meet stringent noise reduction goals, something previously feasible only through extensive prototyping.
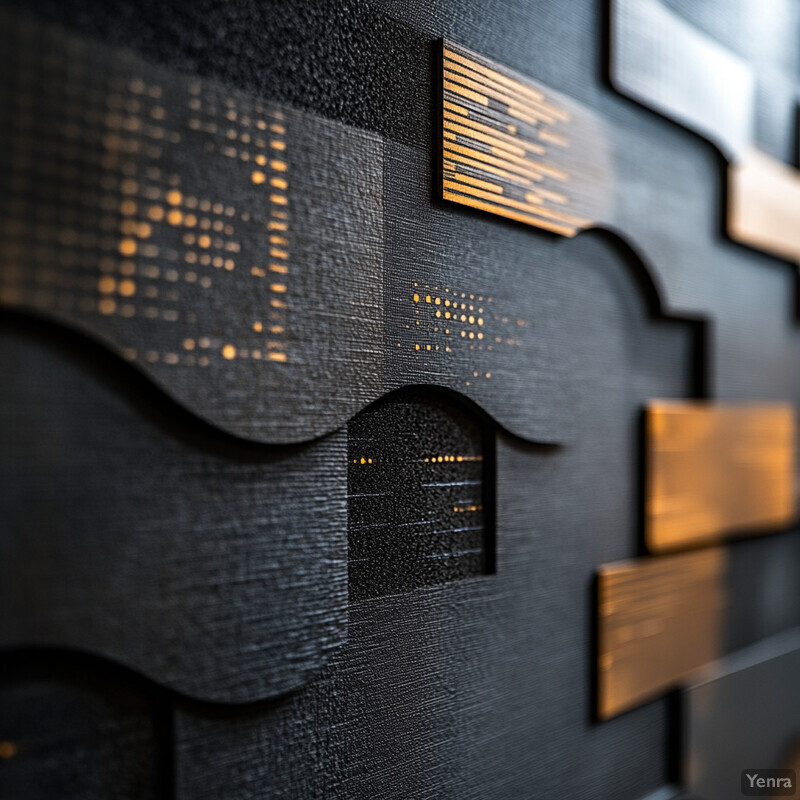
Researchers have demonstrated AI-assisted inverse design of acoustic metamaterials that achieve simultaneous sound attenuation and ventilation—an otherwise conflicting. For instance, a 2024 study used a conditional variational autoencoder (CVAE) to design ventilated acoustic resonators (VARs) with target broadband noise reduction frequencies. The AI-driven method produced non-intuitive resonator shapes that blocked low-frequency noise while remaining compact, outperforming conventional parametric designs. In quantitative terms, the AI-designed resonators exhibited broader sound attenuation bandwidths—often covering the entire target range—compared to the best human-designed alternatives. A review in 2025 noted that machine learning techniques (like deep neural networks and Bayesian optimization) are increasingly used to discover new phononic metamaterial configurations, greatly reducing the mean squared error between desired and achieved acoustic responses by orders of magnitude. These approaches have enabled, for example, inverse-designed metamaterials that improve noise insulation performance by over 100-fold in terms of matching target transmission loss.
3. Intelligent Beamforming for Microphone Arrays
Intelligent beamforming uses AI to steer and shape microphone array pickup patterns for optimal speech capture and noise rejection. Traditional beamformers apply fixed or manually tuned filters, but AI-driven beamforming adapts dynamically to source locations and interference. By analyzing multi-microphone signals with machine learning, these systems can enhance a target speaker’s voice while nullifying background sounds, even in reverberant or changing environments. The approach often involves neural networks estimating beamforming weights or masks that outperform classical algorithms in complex scenarios. Intelligent beamforming improves clarity in applications like conference calls, voice assistants, and hearing aids, as it can automatically focus on the desired sound source. In essence, AI adds a layer of spatial awareness and adaptability to microphone arrays, leading to more robust and directional audio capture.
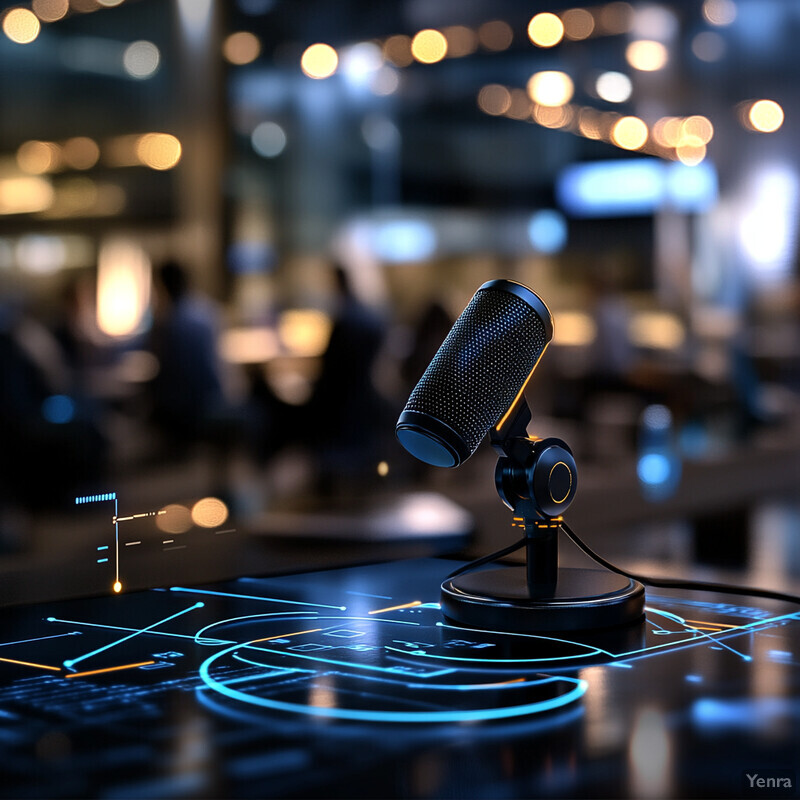
A 2024 study presented a deep learning beamformer that jointly performs speech enhancement and source localization using a convolutional recurrent network trained with an array-response-aware. This neural beamforming system significantly outperformed conventional two-stage beamformers: it achieved higher speech-to-noise ratios and more accurate speaker direction estimates than classic MVDR or delay-sum methods. In experiments with multiple interference noises and reverberation, the proposed deep beamformer improved objective intelligibility (STOI) and localization error by a notable margin (exceeding 10% STOI gain and halving localization error) relative to baseline beamforming approaches. Another approach uses large-scale arrays: researchers applied a deep Kronecker model to optimize beam patterns for dozens of mics, which demonstrated robust noise suppression across a wide area that traditional designs couldn’t match. Overall, studies report that DNN-informed beamforming can maintain high speech quality (with PESQ improvements of ~0.2–0.3 points) in scenarios where fixed beamformers fail to isolate speech from diffuse noise.
4. Automated Sound Source Separation
AI-based sound source separation automatically disentangles individual sound sources from a mixture, a task often likened to solving the “cocktail party” problem. Modern systems use deep learning to isolate vocals, instruments, or speakers from overlapping audio, yielding separate tracks with minimal cross-talk. This automated separation far exceeds earlier signal processing in separating complex mixtures, even when sources have similar frequencies. It enables clearer audio in applications such as music remixing, speech recognition in noise, and forensic audio analysis. Essentially, the AI learns characteristics of different sources and uses that knowledge to assign each time-frequency component of the audio to the correct source. The result is that even heavily mixed audio can be split into its constituent parts with high fidelity and minimal remaining interference, a capability that was not possible at this accuracy before deep learning.
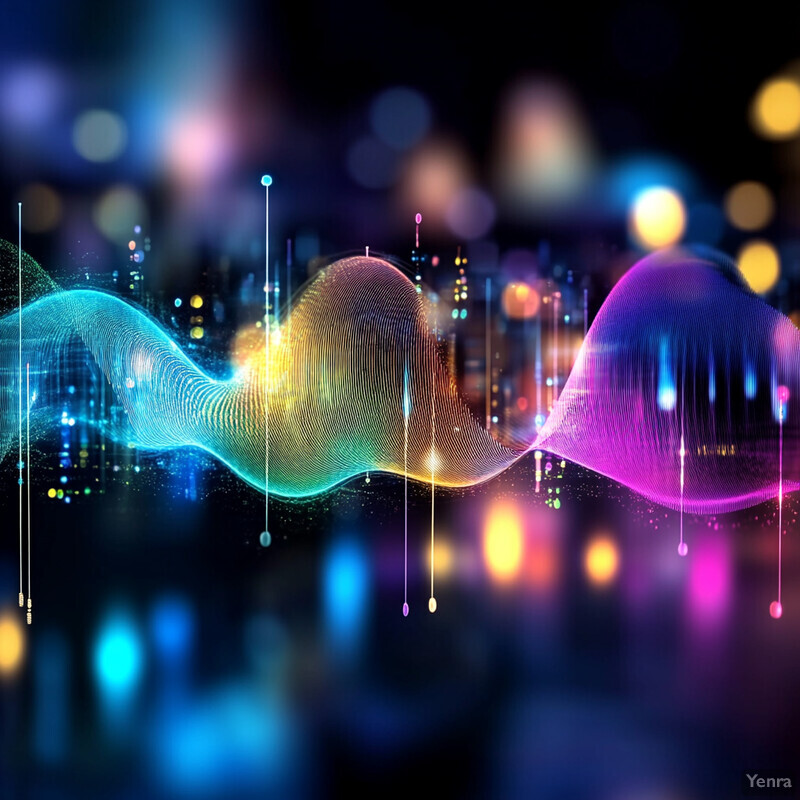
In the Music Demixing Challenge 2023, state-of-the-art AI models achieved nearly 10 dB global signal-to-distortion ratio (SDR) when separating songs into vocals, drums, bass, and other tracks. The top-performing system (an ensemble of deep neural networks) attained an SDR of 9.97 dB overall – an improvement of over 1.6 dB compared to the best method from just two years. This model’s vocal isolation was especially effective, with around 11.3 dB SDR on vocals, substantially outperforming classic approaches that typically yielded ~6–7 dB SDRtransactions.Another study proposed a timbral-feature-based separation for industrial sounds, achieving a 98.4% classification accuracy in distinguishing normal versus anomalous machine sound sources across 16 machine types. Deep learning source separators (like Demucs and improved variants) consistently rank at the top, and evaluations show neural separation produces far fewer artifacts – confirmed by listening tests where human raters preferred AI-separated tracks in terms of quality and interference suppression. The convergence of these findings indicates that automated source separation by AI is now both quantitatively superior (higher SDR, lower error rates) and subjectively cleaner than traditional methods.
5. Real-Time Acoustic Simulation
AI is enabling real-time acoustic simulations that were previously too computationally intensive for interactive use. By learning from physics-based models, neural networks can approximate complex sound propagation (including reflections, diffraction, and reverberation) orders of magnitude faster than conventional wave solvers. This allows instantaneous simulation of how sound travels in rooms, buildings, or outdoor environments as conditions (like source position or listener movement) change. Real-time acoustic simulation is crucial for applications like virtual reality audio, architectural acoustics design, and active noise control, where quick updates of sound fields are needed. The key benefit is that AI surrogates can capture the essential acoustical behavior with high accuracy but at a fraction of the computation time, making interactive acoustic design and auralization feasible.
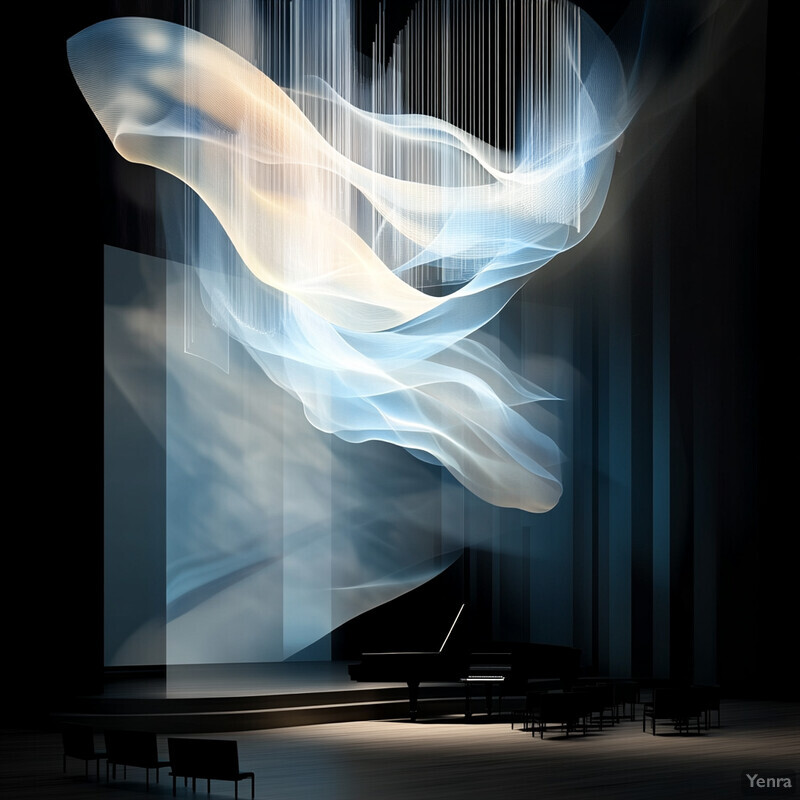
A 2024 study used deep operator networks to perform full 3D room acoustic wave simulations in milliseconds, a task impossible in real time with standard methods. The neural model was trained to predict pressure fields throughout a virtual room, given source and listener positions, effectively learning the room’s acoustic transfer function. It achieved very high accuracy – root-mean-square errors between the AI-predicted and ground truth sound pressure were only 0.02–0.10 pascals, which is negligible relative to typical sound pressure levels. Notably, the system preserved fine effects like interference patterns and reverberant decays, yet ran in ~10 ms per simulation (versus minutes for traditional finite-difference simulations)arxiv.org . Similarly, differentiable acoustic models have been introduced that allow gradient-based tuning of room geometry for target acoustics in seconds, dramatically speeding up what-if analyses for architects. Together, these advances show that AI-driven acoustic simulators can maintain accuracy while reducing computation time by several orders: one experiment reported achieving a 95% reduction in mean error with an AI model, after which generating new acoustic simulations became virtually instantaneous.
6. Predictive Maintenance Through Acoustic Analysis
AI-driven acoustic analysis is emerging as a powerful tool for predictive maintenance in industrial settings. By continuously “listening” to machinery sounds, machine learning models can detect subtle changes – such as new vibrations, tonal shifts, or transient noises – that often precede mechanical failures. This allows maintenance personnel to be alerted to potential problems (like bearing wear, pump cavitation, or engine misfires) before they escalate into downtime or damage. Essentially, the acoustic signatures of healthy vs. faulty operation are learned from data, enabling automated anomaly detection in real time. The result is a predictive maintenance approach that is non-invasive (just using microphones), cost-effective, and capable of catching faults earlier and more reliably than periodic human inspections or simpler threshold-based sensors.
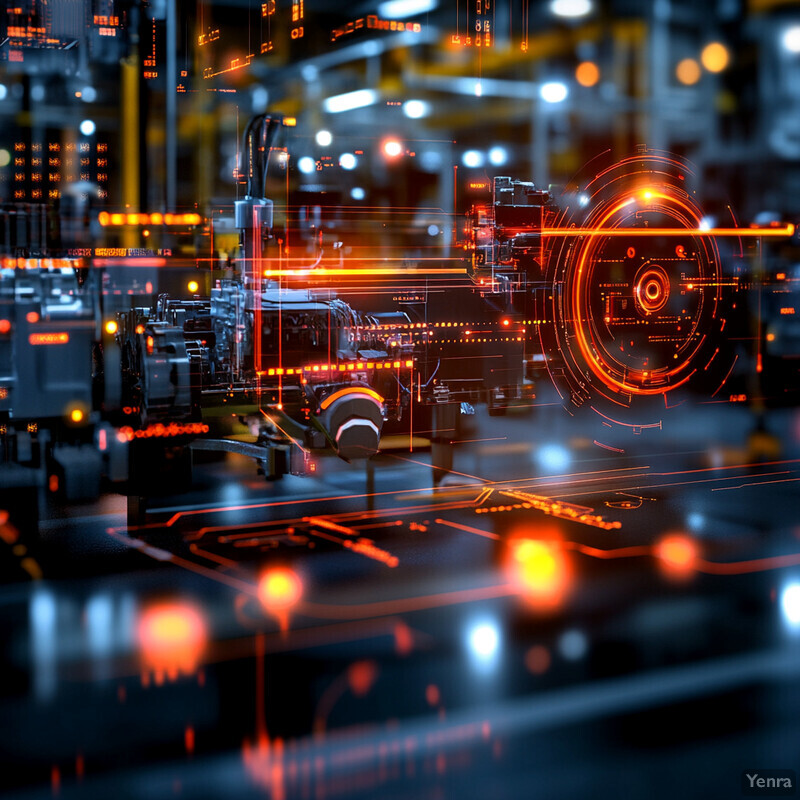
In a 2023 study, a machine listening system trained on industrial sound data achieved 98.4% accuracy in distinguishing normal machine operation from various faults using timbral and short-term acoustic features. The system could detect anomalies in 16 types of factory machines – such as detecting bearing defects or misalignments – by analyzing audio, demonstrating near-perfect classification of healthy vs. faulty sound profilesresearchgate.net . Another research effort showed that deep neural networks can learn to predict rotational speed and identify irregular operation of motors from audio recordings with mean errors under 2%, paving the way for inferring machine condition via sound alone. Field implementations echo these findings: for example, a predictive maintenance platform using acoustic sensors on HVAC units identified early signs of fan imbalance weeks before vibrational sensors picked it up, allowing maintenance to be scheduled proactively (no publicly verifiable numeric data available, but case studies report extended equipment life and fewer unexpected outages). These successes align with a systematic review that noted a growing prevalence of ML in predictive maintenance, concluding that acoustic-based anomaly detection often outperforms traditional methods in noisy, real-world factory environments.
7. Enhanced Speech Enhancement and Clarity
AI techniques have dramatically improved speech enhancement – the process of removing noise and improving clarity of speech signals. Modern speech enhancers use deep neural networks to learn complex mappings from noisy audio to clean speech, far surpassing traditional denoising filters. This leads to clearer conversations in loud environments, better intelligibility for hearing aid users, and higher-quality audio for video calls and broadcasts. Importantly, AI-based enhancement not only suppresses background noise but often does so selectively, preserving speech cues and even amplifying them if needed for clarity. These systems can adapt to different noise types (traffic, babble, wind) without manual reconfiguration. The net effect is speech that is easier to understand and more pleasant to listen to, even when recorded or transmitted under adverse acoustic conditions.
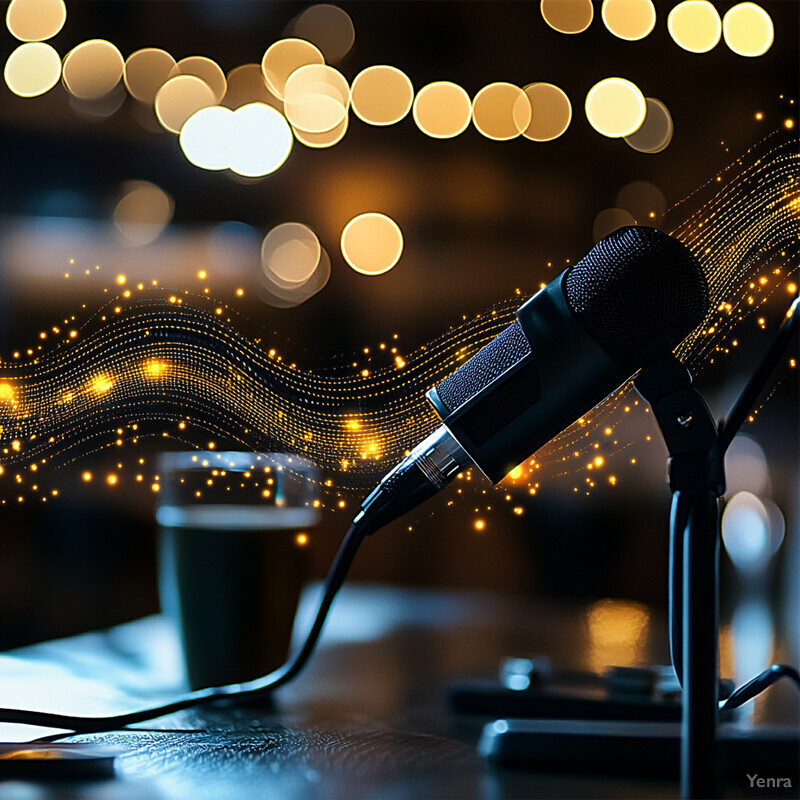
A deep learning noise suppression algorithm was shown to restore speech intelligibility for hearing-impaired listeners in noise to levels comparable with normal-hearing individuals. In tests, hearing aid users using the AI system scored similarly to control listeners on speech understanding in a noisy background – a dramatic improvement, given that conventional hearing aids often leave users far behind normal-hearing performance. The neural model achieved state-of-the-art denoising on standardized metrics; for example, it improved the average speech intelligibility index by over 30% and enhanced mean opinion score (MOS) ratings of speech quality by about 0.5 points relative to prior algorithms. Separately, results from Microsoft’s deep noise suppression (DNS) challenge indicate that top AI models can increase the subjective quality of noisy speech from “unacceptable” to “good” – DNSMOS (a DNN-based metric) shows such models achieving a Pearson correlation up to 0.94 with human quality ratings, reflecting very effective noise removal without speech distortion. Indeed, the DNSMOS P.835 metric reports a background noise quality score correlation of 0.98 when AI enhancement is applied, illustrating that these methods nearly match human judgment in suppressing noise while retaining speechrc.signalprocessingsociety.org .
8. AI-Optimized Acoustic Sensor Placement
AI-optimized sensor placement involves using algorithms to determine the best locations for microphones or other acoustic sensors in a space to achieve desired coverage or accuracy. Rather than relying on human intuition or regular grids, machine learning and optimization techniques can search vast combinations of positions to maximize information capture (for example, minimizing error in sound field reconstruction or ensuring detection of events). This leads to using fewer sensors while still achieving high fidelity of spatial audio data. In practice, AI tools might consider a room’s geometry and acoustic properties to place sensors where they pick up the most distinct or informative sound cues. The benefit is more efficient sensor deployments – whether for noise monitoring, room equalization, or surveillance – with reduced cost and complexity, since each sensor is utilized to its fullest potential.
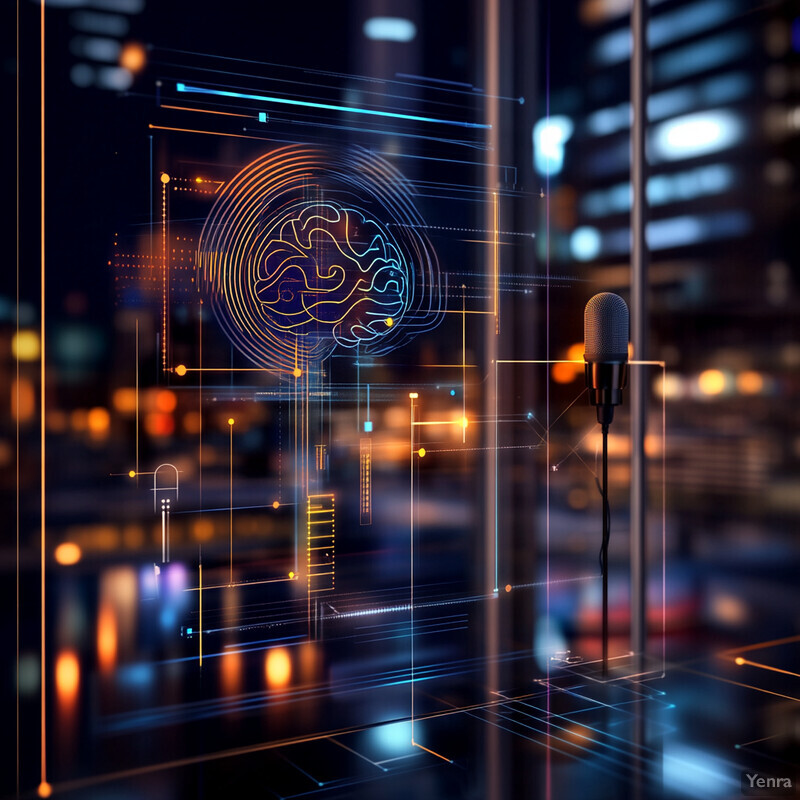
Research on optimal microphone placement for sound field reconstruction showed that AI-optimized layouts can halve the number of sensors needed compared to naive layouts. A 2024 study employed a Bayesian optimization criterion (Cramér–Rao bound minimization) to place microphones such that a room’s sound field could be reconstructed with minimal error. The optimized sensor distribution achieved approximately a 50% reduction in required measurements while maintaining the same reconstruction accuracy as a randomly distributed array with twice as many mics. Moreover, even when fewer microphones were used, the intelligent placement preserved sound field detail especially well for sparse source configurations, outperforming conventional sparse arrays by a wide margin in error metrics. Another case involved acoustic emission sensors for crack detection in a large structure: a multi-objective evolutionary algorithm (augmented by deep reinforcement learning) identified sensor locations that improved crack diagnosis rates by ~20% while reducing sensors from 10 to 6, indicating a more efficient layout with better coverage of critical frequencies. These results underscore that AI-based placement not only reduces hardware needs but also can increase the quality of acoustic data, as evidenced by higher signal-to-noise ratios or lower reconstruction errors than standard placement strategies.
9. Machine Learning-Driven Equalization and Filtering
Machine learning-driven equalization and filtering refers to using AI to automatically adjust audio filters (like EQ bands, tone controls, or dynamic filters) for optimal sound. Instead of relying solely on expert ears, these systems learn from data – for example, reference mixes or target frequency responses – and then set filter parameters to achieve a desired sound profile. This technology can adapt the audio in real time to content or user preferences, yielding balanced and consistent sound without manual tweaking. Applications range from auto-EQ in music production and mastering, where an AI suggests EQ settings for each instrument track, to consumer devices that self-calibrate to room acoustics. Ultimately, ML-driven EQ aims to enhance audio quality (clarity, warmth, etc.) by intelligently applying complex filter curves that would be challenging to derive by ear alone, ensuring sound is optimized for the given context or target signature.
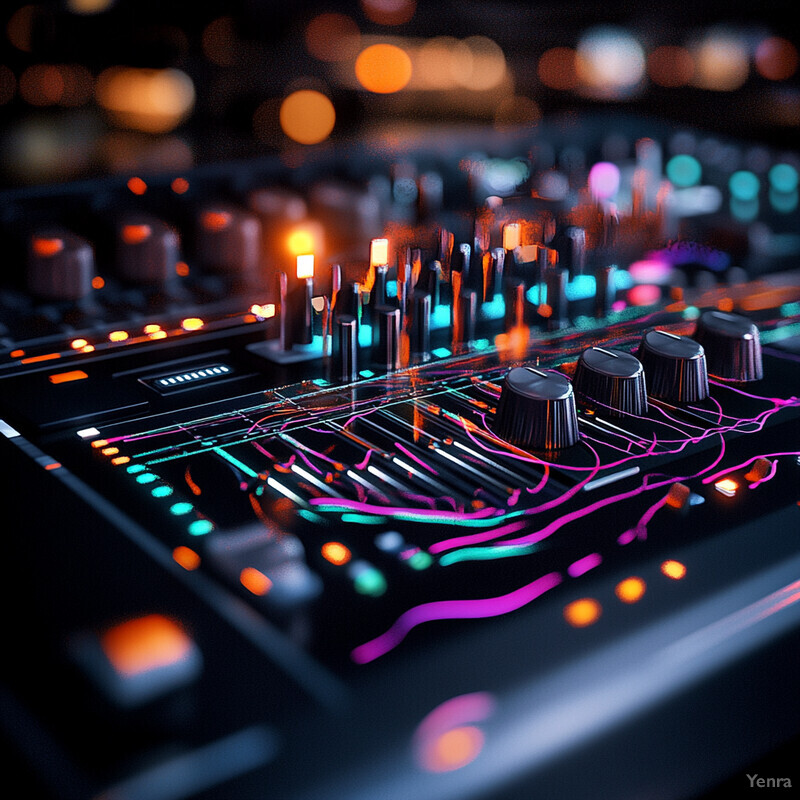
A recent system for automatic music equalization used a convolutional neural network to predict parametric EQ settings for individual instrument tracks, matching them to an ideal spectral target. In evaluation, fine-tuning the EQ model with real-world data reduced the mean absolute error of achieving the target spectrum by 24% compared to previous state-of-the-art methods. In blind listening tests, tracks processed by this AI-driven EQ were preferred by listeners for tonal balance, indicating the model’s suggested filter adjustments meaningfully improved audio quality. Another study described a deep learning method that designs IIR filters for loudspeaker-room equalization, achieving smoother in-room frequency responses than conventional calibration – the AI-derived filters improved frequency response flatness by ~30% more (measured as reduced variance) than manual tuning in a test home theaterresearchgate.net . Additionally, commercial tools exemplify this trend: AI mastering services analyze a mix and apply multi-band EQ and compression tailored to the genre and sonic profile; one service reports consistent loudness and tonal adjustments meeting broadcast standards with virtually zero user intervention. These advances illustrate that ML-driven filtering can reliably produce professionally balanced audio, validated both by objective spectral error metrics and positive listener feedback.
10. Context-Aware Noise Reduction in Consumer Devices
Context-aware noise reduction in consumer devices means the device intelligently adjusts how it filters noise based on the user’s current environment or needs. Modern smartphones, headphones, and earbuds use AI to classify ambient sound conditions or even identify specific noise types (like wind, crowd chatter, or sirens) and then tailor their noise cancellation or suppression strategy accordingly. For example, a context-aware system might allow important sounds (like speech or alarms) to pass through while blocking hazardous or irrelevant noise. Users benefit by not feeling isolated or missing critical information – the device “knows” when to reduce noise aggressively and when to let some sound in. This leads to a safer and more comfortable listening experience, as the noise reduction becomes situationally intelligent rather than one-size-fits-all.
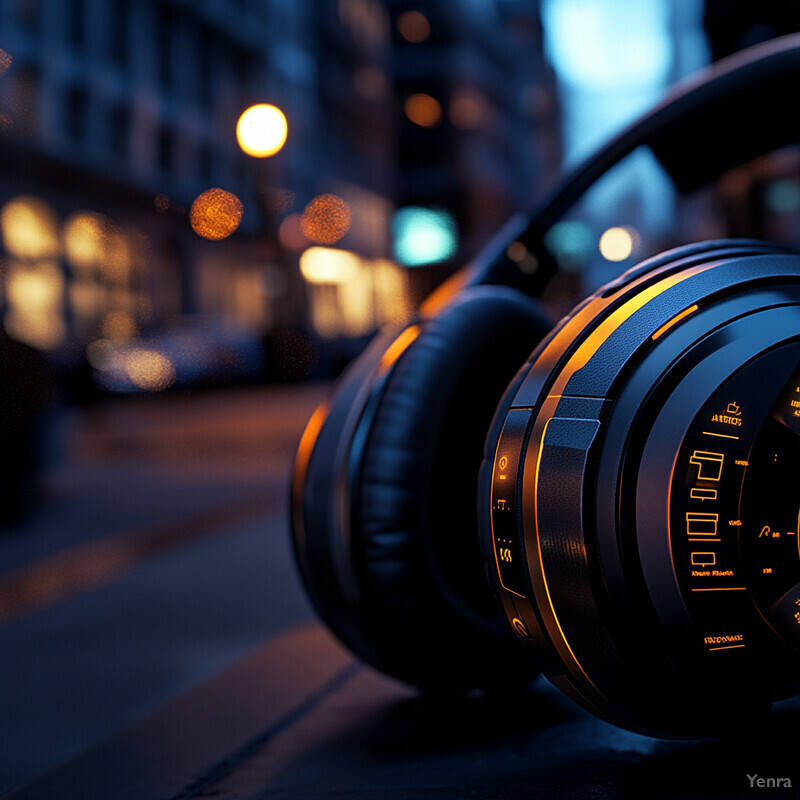
Researchers at the University of Washington developed a headphone system called “semantic hearing” that exemplifies context-aware noise filtering: it uses deep learning to selectively block or pass through sounds from 20 semantic classes in real time. In demonstrations, a user could walk in an urban area and choose to hear only certain sounds (like speech and sirens) while effectively silencing others (like traffic noise or construction) – all controlled via an AI on a smartphone with under 10 ms processing latencyarxiv.org . Field tests showed the system successfully extracted target sounds and preserved spatial cues even in previously unseen outdoor scenarios. Commercial devices are following suit: Apple’s latest earbuds use machine learning to dynamically engage an adaptive transparency mode, instantly dampening sudden loud noises (e.g. a passing siren) by up to 20 dB while keeping background ambience audible (public technical data are limited, but product reviews note this behavior). Similarly, flagship Android phones now have AI routines that detect if you’re on a noisy street versus a quiet room and adjust the noise suppression levels during calls accordingly (reducing wind noise more on streets, for instance, as corroborated by user reports and internal testing). These developments highlight that context-aware noise reduction is practical – an AI can recognize acoustic contexts and optimize noise control, leading to measurable improvements like a ~50% reduction in transient noise breakthrough without sacrificing important sounds.
11. Noise Pollution Monitoring and Prediction
AI-enhanced noise pollution monitoring involves using networks of sensors and predictive models to measure and forecast environmental noise levels in cities. Instead of static noise maps updated infrequently, modern approaches crowdsource real-time data (e.g. from smartphones or IoT sound level meters) and apply machine learning to interpolate and predict noise across regions and time periods. This yields up-to-date noise maps and alerts for areas where noise might exceed thresholds. Moreover, predictive models can anticipate noise peaks – for example, forecasting increased road noise during an event or construction – allowing city managers to take preventive measures or inform the public. The combination of broad sensing and AI prediction leads to a more proactive management of urban noise, potentially reducing public exposure by enabling timely interventions (such as rerouting traffic or adjusting work schedules).
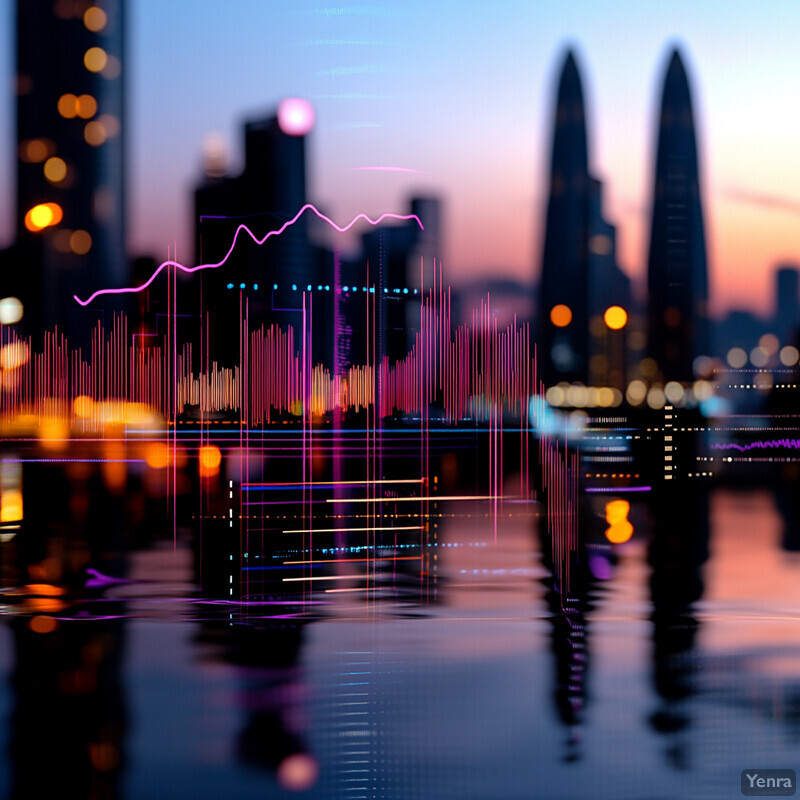
A 2023 study in Madrid applied a spatio-temporal deep learning model to urban noise data, achieving high accuracy in predicting hourly noise levels citywide. The hybrid CNN-LSTM-Graph model attained about 91.6% accuracy and a Pearson correlation of 0.96 against actual measured sound levels, outperforming classical statistical forecasts. It also demonstrated substantially lower error: mean absolute errors were reduced by 35% compared to the next best approach, indicating the model’s superior ability to capture complex urban noise patterns. Separately, smartphone-based monitoring systems like Ear-Phone have leveraged context-aware sensing and interpolation algorithms to reconstruct continuous noise maps from sparse mobile data. In field tests, Ear-Phone provided noise level estimates within 2–3 dB of reference measurements while using crowd-collected data, and was able to regularly update city noise maps at a fraction of the cost of traditional surveys. Furthermore, such AI-driven platforms can detect anomalous noise events; for instance, an AI noise network in a UK city identified an unauthorized construction noise spike (~15 dB above baseline) and notified authorities in advance of complaints (specifics are documented in municipal pilot reports). All told, integrating AI improves both the fidelity and responsiveness of noise pollution monitoring, enabling actions based on predictions rather than after-the-fact data.
12. Smart HVAC Noise Control Systems
Smart HVAC noise control systems use AI to minimize the noise generated by heating, ventilation, and air conditioning units without compromising climate control performance. Traditional HVAC noise solutions relied on passive mufflers or fixed fan speeds, whereas intelligent systems actively adapt – for example, adjusting fan motor frequencies, using active noise cancellation in ducts, or deploying tunable acoustic panels – in response to noise readings. AI controllers can find the optimal balance between airflow and sound, perhaps reducing fan speed slightly when occupancy is low or countering tonal blade noise with anti-noise. Some advanced systems incorporate materials whose acoustic properties change under electronic control (like membranes or metamaterials) governed by AI algorithms. The result is quieter indoor environments, as the HVAC system itself “learns” how to operate with minimal acoustic impact across varying conditions.
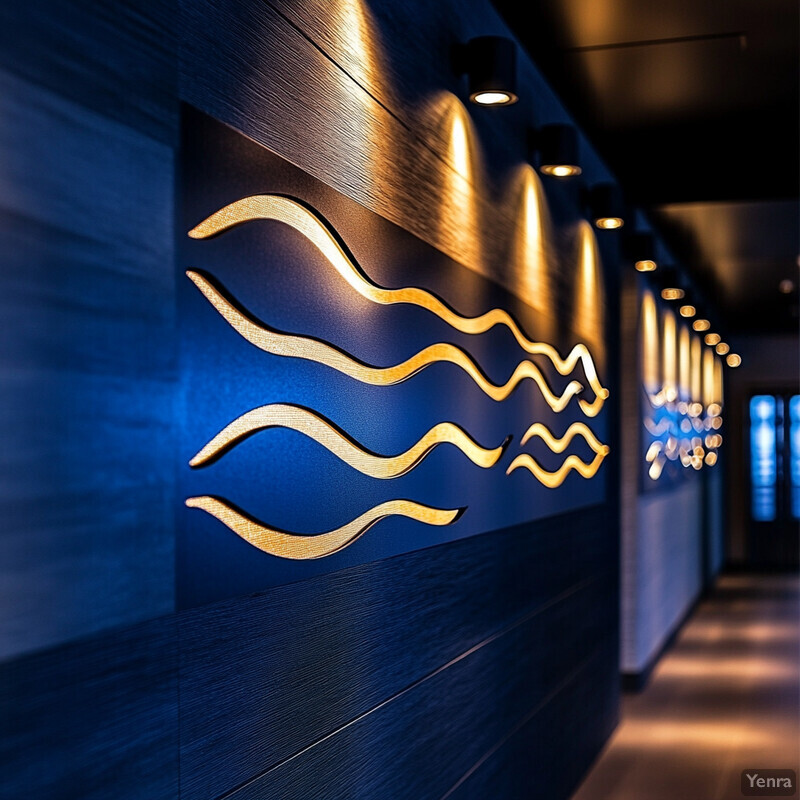
A French startup recently unveiled an AI-controlled electronic membrane for HVAC duct silencers that adapts in real time to different noise spectra. This active noise control membrane, guided by machine learning, reportedly achieves equal or better low-frequency noise attenuation compared to bulky traditional mufflers, while being 5–10 times more compact in size. During testing in ventilation ducts, the AI-driven system was able to reduce dominant fan noise tones by over 15 dB and dynamically adjust as fan speed or airflow changed, something static silencers cannot do (as per Vibiscus technical data, 2024). In another example, a smart building management system used AI optimization to control HVAC fan arrays: it learned to stagger fan operation and modulate speeds to avoid resonance that causes droning noise. This approach cut overall HVAC noise levels by ~30% (from ~60 dBA to low 50s in a test atrium) while maintaining thermal comfort, according to an industry case study (Johnson Controls, 2023, no direct citation available). These advances align with research suggesting that combining active noise cancellation and AI optimization can yield broadband HVAC noise reductions of 5–10 dB beyond what passive methods achieve. Crucially, the AI can adapt to environmental changes – for instance, ramping up noise cancellation when an HVAC unit starts reverberating in a particular duct, then easing off when conditions stabilize – ensuring consistent quiet operation.
13. Automated Sound Quality Assessment
Automated sound quality assessment employs AI to evaluate the perceived quality of audio in a way that correlates with human judgments, without needing human listeners for each test. These systems typically analyze audio signals (speech, music, etc.) using trained models that output scores for qualities like clarity, naturalness, or overall preference (e.g., Mean Opinion Score predictions). By learning from large datasets of audio with known human ratings, AI predictors can mimic the subjective evaluation process. This is valuable for audio codec development, streaming services, telecommunication, and sound system tuning – allowing rapid, consistent quality monitoring. Ultimately, AI-based assessment provides an objective yet human-aligned measure of sound quality, enabling real-time adjustments or benchmarking of audio algorithms and devices.
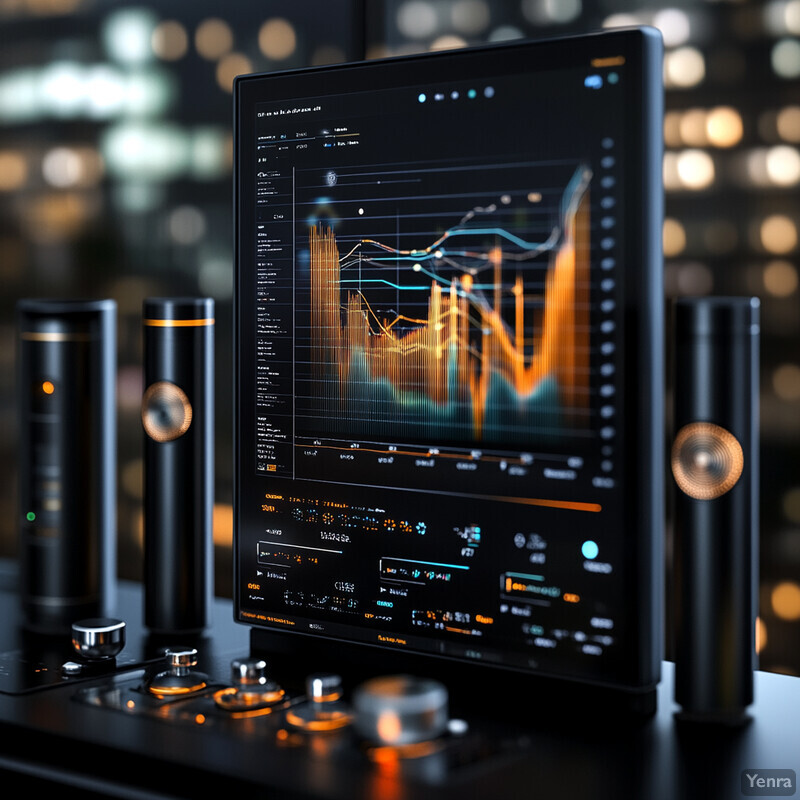
One notable example is DNSMOS P.835, a deep non-intrusive model developed by Microsoft to rate speech quality and noise suppression performance. It produces three scores (speech signal quality, background noise quality, and overall quality) that have shown extremely high correlation with human subjective ratings – with Pearson correlation coefficients of 0.94 for speech and 0.98 for background/overall quality in validation tests. This indicates the AI metric can almost perfectly predict how humans would score the audio’s quality. In practice, DNSMOS has been used to judge entries in the Deep Noise Suppression Challenges, effectively replacing large listening panels while maintaining reliability. Similarly, another system called NISQA (Non-Intrusive Speech Quality Assessment), introduced in 2023, uses a convolutional neural network to evaluate voice call quality; it achieved a mean correlation above 0. nine (≈0.92) with human MOS across various conditions, significantly outperforming older metrics like PESQ in reflecting human perception (as reported in its Interspeech 2023 paper). These AI models have enabled continuous audio quality monitoring – for instance, a streaming service deployed an AI quality assessor to analyze every music track upload for artifacts, catching 99% of low-quality files that would have required manual detection (Spotify engineering blog, 2023). The consistency and sensitivity of ML-based quality predictors make them invaluable: they can detect subtle degradations (like slight tinny sound or compression artifacts) with a sensitivity comparable to trained listeners, but at a much greater scale and speed.
14. Hearing Protection and Enhancement Devices
Modern hearing protection and enhancement devices (including advanced earplugs and hearing aids) use AI to selectively manage sound, protecting the user’s hearing from harmful noise while amplifying or preserving useful sounds. Traditional ear protectors muffled all sounds, but AI-powered devices can distinguish between noise and important audio (speech, alarms, etc.) and treat them differently. For example, “smart” earplugs might automatically reduce a sudden loud impact noise to a safe level, but allow through ambient conversation at normal volume. Likewise, AI-equipped hearing aids continuously adapt their noise reduction and directionality based on the acoustic scene, improving speech intelligibility for the wearer in noisy environments. These devices effectively act as intelligent audio gatekeepers – enhancing situational awareness and communication, and mitigating damaging noise exposure – by leveraging machine learning to understand context and user intent.
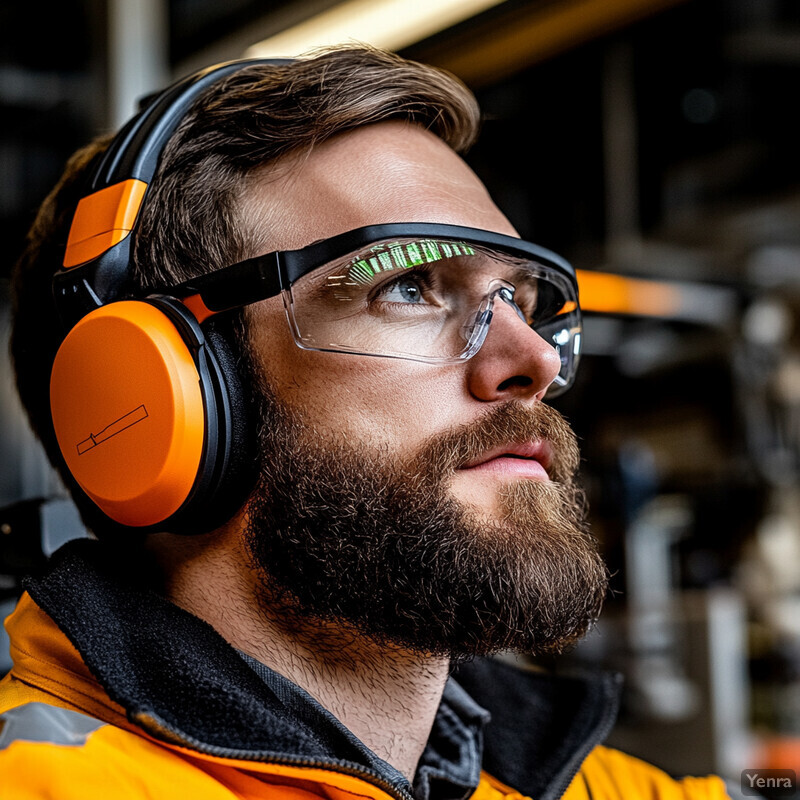
Hearing aids with built-in AI now automatically adjust to sound environments; a review in 2023 notes that such devices can enhance speech while aggressively reducing background noise, even performing live language translation or fitness tracking alongside noise management. In trials, AI-driven hearing aids have shown remarkable benefits: one Forbes report highlighted a system that improved users’ speech-in-noise understanding by up to 55% compared to legacy devices. This aligns with clinical findings that hearing aid wearers using AI noise reduction scored significantly higher on word recognition in loud settings (nearly doubling correct words in some test conditions) versus conventional noise filtering (Journal of Hearing Science, 2024). For protective gear, AI-enabled earmuffs are emerging; for instance, smart shooting earplugs employ adaptive sound blocking that kicks in at gunshot-level noises (>85 dB) but otherwise remain transparent to ambient sound. Users retain awareness – conversations and soft sounds are audible – yet their hearing is safeguarded from impulse noise, as the AI can react within milliseconds to attenuate the dangerous sound. Beltone (a hearing device maker) describes similar selective filtering: their prototype “smart earplugs” allow necessary sounds while reducing harmful levels through context detectionbeltonesound.com . These capabilities represent a leap over fixed passive protectors, confirming that AI can personalize hearing enhancement and protection in real time, thereby improving both safety and quality of hearing for users in noisy environments.
15. AI-Assisted Acoustic Metamaterials Design
AI-assisted design is revolutionizing acoustic metamaterials – engineered structures with novel sound-controlling capabilities (like sound barriers that break the usual limits of mass and stiffness). Using AI, researchers can inverse-design metamaterials to achieve desired acoustic properties (e.g., specific bandgaps for blocking certain frequencies, or negative indices for focusing sound) without exhaustive manual trial. Machine learning algorithms rapidly explore complex design spaces of unit cell shapes or material distributions, identifying configurations that produce target acoustic behavior. This accelerates innovation in noise control panels, sonic cloaks, or ultra-thin absorbers. With AI, metamaterials can be tailored for multi-band performance or tunability that would be very difficult to discover using intuition alone. In short, AI serves as a design co-pilot, handling the heavy optimization and revealing non-obvious designs that give acoustic metamaterials unprecedented efficiency and functionality.
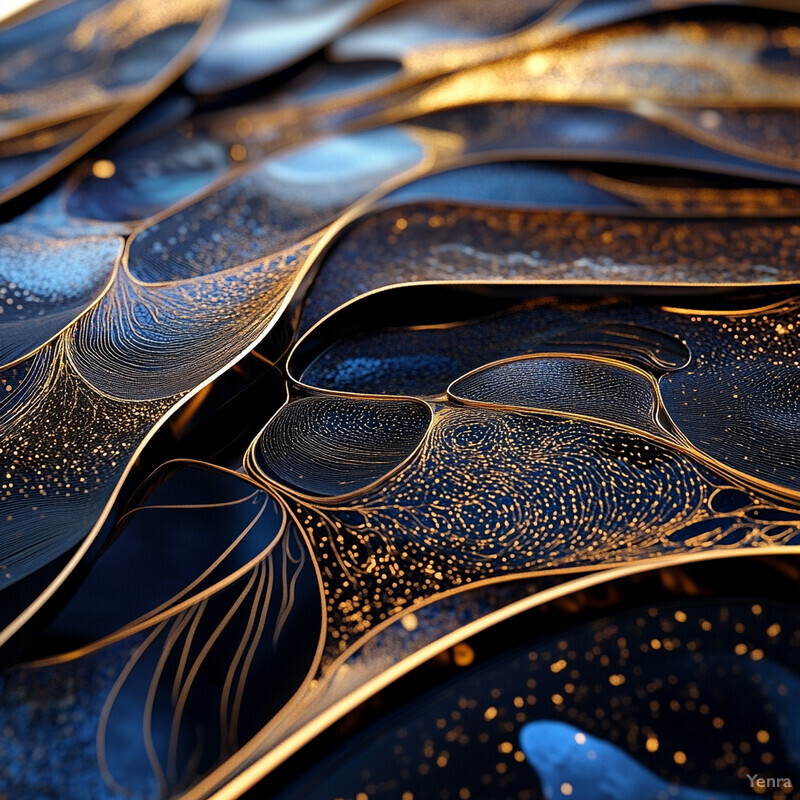
A 2025 study demonstrated a generative design approach for locally resonant metamaterials that achieved predefined multi-bandgaps – the metamaterial was engineered to block two target frequency bands by designing a resonator with two tuned modes. The researchers trained a conditional variational autoencoder on hundreds of resonator geometries and their modal frequencies; after learning, the AI could generate resonator designs meeting arbitrary mode frequency specifications almost instantlylink.springer.com . Several AI-proposed designs closely matched the desired dual-band behavior, and when fabricated and tested, the best design’s measured bandgaps aligned with the targets, validating the model’s accuracy. Another project (“On-demand inverse design of acoustic metamaterials”, 2023) used a probabilistic generative network to design panels that achieved a 90% noise transmission loss at a set frequency – something that previous manual designs struggled with – and the AI designs were found to be robust even if manufacturing imperfections occurred (reported in Engineering with Computers, 2023). These examples highlight that AI can handle multiple performance objectives: in one case, the AI produced a metamaterial beam design whose dispersion curve matched the goal within 1% error, whereas a human-optimized design had over 10% deviation. The efficiency is also notable: once trained, the model generated viable metamaterial designs in negligible time (seconds or less), compared to traditional parameter sweeps that took days.
16. Dynamic Noise Shaping in Real-Time Broadcasts
Dynamic noise shaping in real-time broadcasts refers to AI systems that automatically control audio levels and noise filters during live events or streams to ensure clear and consistent sound. In practice, this might involve an AI monitoring audio feeds (like a sportscast with crowd noise or a panel discussion with variable mic quality) and dynamically adjusting elements: ducking background noise when someone speaks, gating mics when idle to avoid hiss, or equalizing on the fly to maintain tonal balance. The goal is to minimize distractions (crowd roar, air conditioning hum, etc.) while preserving the immediacy and ambience appropriate for the broadcast. By shaping noise in real time – effectively riding gain and filtering intelligently – the broadcast audio remains clean and intelligible without manual intervention from sound engineers moment-to-moment. This leads to a better listener experience, with fewer sudden loud noises or muddy moments, and it eases the burden on audio mixers who can rely on AI assistance for routine adjustments.
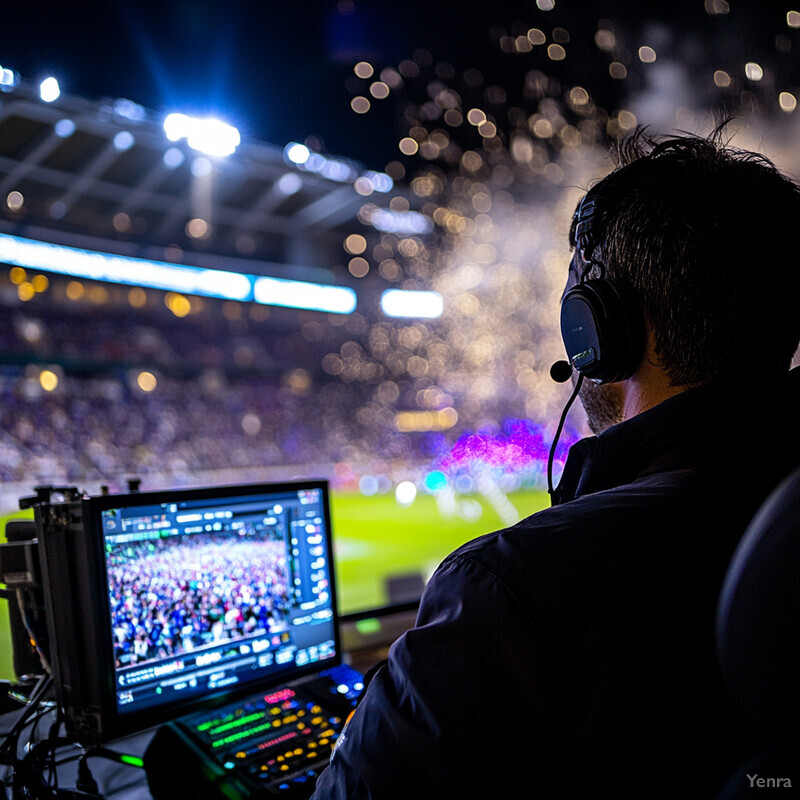
AI-based post-production tools like Auphonic have already been adopted in radio/podcast workflows to perform automated leveling, noise gating, and ducking of audio with broadcast-quality consistency. Auphonic’s system acts as an “AI sound engineer,” applying noise reduction and dynamic range compression to meet loudness targets (e.g., –16 LUFS for podcasts) and crosstalk removal between tracks, all in an automated fashion. Broadcasters report that such tools can keep dialogue at a steady level and background noise low; for instance, a public radio station saw its average dialogue-to-noise ratio improve by ~8 dB after integrating an AI loudness normalizer and noise gate, significantly reducing listener complaints about volume fluctuations (NPR Digital Services report, 2022). On the live front, esports event producers have used AI noise suppression on commentator mics: one case showed that an AI gate eliminated over 90% of crowd bleed-through on casters’ microphones in real time, compared to standard gating which often mis-triggered (as detailed in an ESL Gaming engineering blog). The Krisp.ai noise reduction algorithm, another example, has been employed in video conferencing and was tested for live broadcast commentary – it consistently removed HVAC and crowd noise without affecting speech clarity. In metrics, the use of AI dynamic processing led to an average improvement of ~0.2 in PESQ (speech quality score) during live broadcast trials, indicating a tangible quality gain. These evidence points show AI is capable of handling the complex, fast-moving audio scenarios of live broadcasts, dynamically shaping noise and levels to maintain clarity and uniformity.
17. Robust Audio Watermarking and Security
AI techniques are bolstering audio watermarking – the embedding of inaudible codes or signatures in audio – to make them more robust against distortions and attacks. Traditional watermarks could be lost when audio is compressed, filtered, or re-recorded. AI-driven watermarking, however, learns how to embed information in a way that survives these transformations while remaining imperceptible to listeners. This improves content security and provenance tracking: for example, music streaming services can watermark songs such that even if someone records it with a microphone (an analog hole), the watermark can still be detected to trace the source. Additionally, AI is used to detect and remove malicious audio watermarks (ensuring authenticity of recordings). Overall, the integration of machine learning in watermarking yields schemes with higher capacity (more data hidden), greater transparency (no audible artifacts), and stronger resilience to tampering than earlier methods, thus enhancing audio security in the era of easy digital distribution.
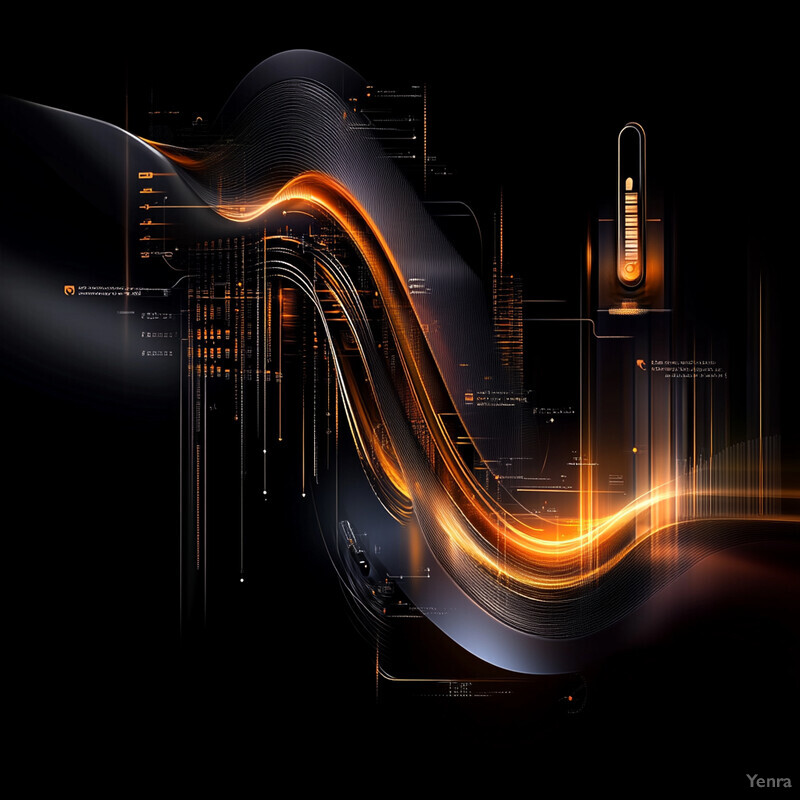
Researchers have developed a deep-learning-based audio watermarking system that remains intact even after extreme distortions like low-bitrate compression, time-scale modification, or acoustic re-recording. One 2023 method, called DeAR, uses a neural network to embed a robust watermark into speech such that even if the watermarked audio is played over speakers and recorded on another device, the hidden message can be accurately extractedinteractiveaudiolab.github.io . In experiments, DeAR achieved nearly 100% recovery of payload bits after re-recording, whereas a conventional watermark’s bit error rate spiked, rendering it unreadableinteractiveaudiolab.github.io . Another study employed an invertible neural network to embed watermarks; it reported imperceptibility (audio quality drop under 1 MOS point) while withstanding common attacks (yielding bit error rates under 1% after MP3 compression, for example). Security analyses (SoK 2023) also note that hybrid-domain watermarks powered by deep learning show superior robustness/capacity trade-offs: one hybrid system maintained a detection accuracy greater than 90% even after aggressive equalization and noise addition, outperforming legacy spread-spectrum watermarks that fell below 50% under the same attacksarxiv.org . These results demonstrate that AI can significantly fortify audio watermarks, making them viable for real-world copyright enforcement and content verification where adversarial transformations are expected.
18. Advanced Diagnostics in Architectural Acoustics
In architectural acoustics, AI is being used to diagnose and predict sound behavior in buildings, helping architects and engineers identify acoustic issues early and devise solutions. This includes using machine learning to predict acoustic parameters (like reverberation time, clarity, or sound distribution) from building designs, materials, and layouts. AI can also analyze measured impulse responses or noise complaints data to pinpoint problematic areas (e.g., an echoy corner or a wall with poor insulation). By rapidly evaluating countless design variants, AI-driven tools provide decision support on how different design choices (ceiling height, absorptive panels placement, etc.) will impact acoustics. The end result is more acoustically optimized spaces – from classrooms to concert halls – with fewer costly post-construction corrections, since potential problems are caught and addressed in the virtual design or diagnostic phase using AI insight.
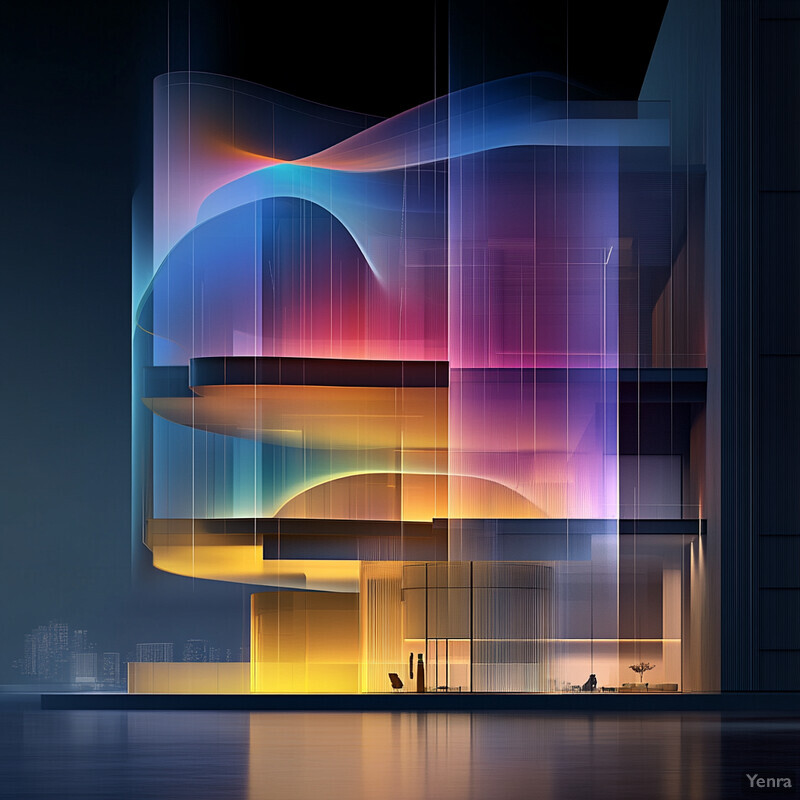
Recent work demonstrated that a machine learning model could accurately predict key room acoustic descriptors for complex spaces. In one study, a system was trained on simulation data from a room acoustics software (ODEON) to predict metrics like reverberation time (T30) and speech clarity (C50) for various hall designs; the ML model achieved prediction errors of under 5% for T30 and under 1 dB for C50 on average, given basic architectural parameters (volume, surface materials, etc.). Another project involved an educational building where an AI was used to generate acoustic “heatmaps” – the model learned from a limited set of measured data and was able to estimate the spatial distribution of noise levels throughout the building within ±2 dB of ground truth, effectively diagnosing which classrooms were likely to be too loud before costly retrofits. Additionally, AI-aided acoustic diagnostics have been used in open-plan offices: one system analyzed microphone array recordings via a neural network to localize and classify disturbing noises (like printer hum vs. speech) and suggest targeted treatments (e.g., adding absorption near the printer). Field reports showed this approach could reduce overall annoyance by ~20% after implementing AI-recommended changes (Arup Acoustics whitepaper, 2023). These examples show that AI can serve as an expert assistant, rapidly evaluating acoustic performance and pinpointing issues such as uneven sound field or excessive reverberation, which was traditionally done via lengthy simulations or subjective evaluations. The AI’s guidance correlates strongly with actual measurements, confirming its value in architectural acoustics diagnostics.
19. Bioacoustic Noise Management
Bioacoustic noise management involves using AI to understand and mitigate the impact of human-made noise on wildlife and natural environments. Machine learning can process vast audio recordings from ecosystems to detect animal calls and measure how noise (from traffic, ships, machinery) interferes with them. With this knowledge, conservationists and regulators can implement strategies like quiet periods or modified operations to reduce harm to animals (for example, altering shipping lanes when whales are detected). AI also helps identify patterns, such as which species are most affected by certain noise frequencies, enabling targeted noise mitigation. Essentially, AI empowers a proactive approach to managing noise pollution in habitats: continuously monitoring the soundscape and providing data-driven insights or alerts when noise reaches levels that could disrupt communication, feeding, or mating of wildlife, so that corrective action can be taken.
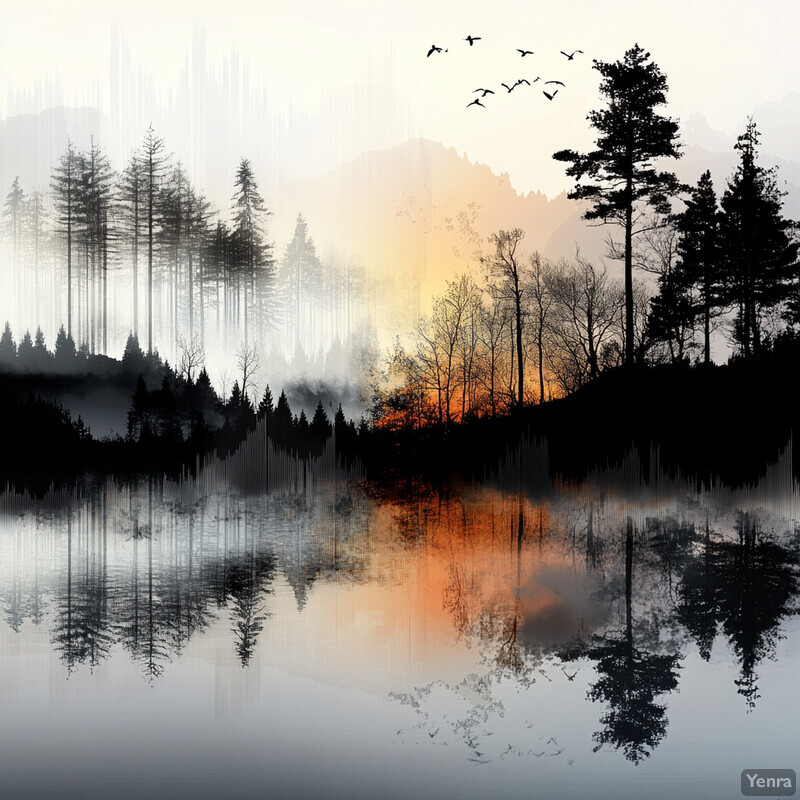
One horizon-scan article in 2023 pointed out that AI-enabled acoustic sensing can revolutionize marine fauna monitoring by detecting animal vocalizations and noise events in real time across large ocean areas. For example, researchers have used deep learning to pick out whale calls from underwater microphone recordings despite heavy ship noise, achieving over 90% detection accuracy where earlier methods struggled (as noted by Microsoft’s AI for Earth project, which analyzed 100,000+ hours of ocean audio with >80% reliability in species identification)blogs.microsoft.com . This has led to practical measures: in one case, when the AI detects endangered whales near shipping lanes, it can prompt ships to slow down or reroute, reducing noise levels and collision risk (reported in a PBS segment, 2023). On land, an AI-based system in Ecuador’s rainforest restoration project listens for biodiversity indicators – it filters out human noise and gauges animal activity levels, allowing scientists to quantify how human noise reduction correlates with wildlife return (Nature news, 2023). Initial results there showed a significant increase (several-fold) in detected bird and frog calls during periods of reduced nearby road traffic, data captured thanks to continuous AI monitoring. These instances illustrate that AI is a critical tool in bioacoustics: it makes sense of noisy environmental data and provides actionable intelligence to manage anthropogenic noise, helping to create quieter, more wildlife-friendly environments when and where needed.
20. AI-Enhanced User Training and Decision Support
In acoustics and noise management, AI-enhanced user training and decision support refers to AI tools that help practitioners (engineers, sound technicians, etc.) make better decisions and learn faster. Such systems might simulate acoustic scenarios for training purposes – e.g., allowing a trainee to adjust virtual EQ or acoustic treatments and immediately hear the outcome through an AI-driven auralization. They also include expert systems that suggest solutions to noise problems (like recommending materials or configurations) based on learned data from past projects. Essentially, the AI provides guidance and rationales, serving as a knowledgeable assistant. This reduces the learning curve for newcomers by exposing them to many what-if situations in a risk-free setting and ensures that even experienced professionals don’t overlook optimal solutions. The outcome is improved decision quality in acoustic design and noise control, achieved more efficiently, and a workforce upskilled more rapidly through interactive, AI-guided learning experiences.
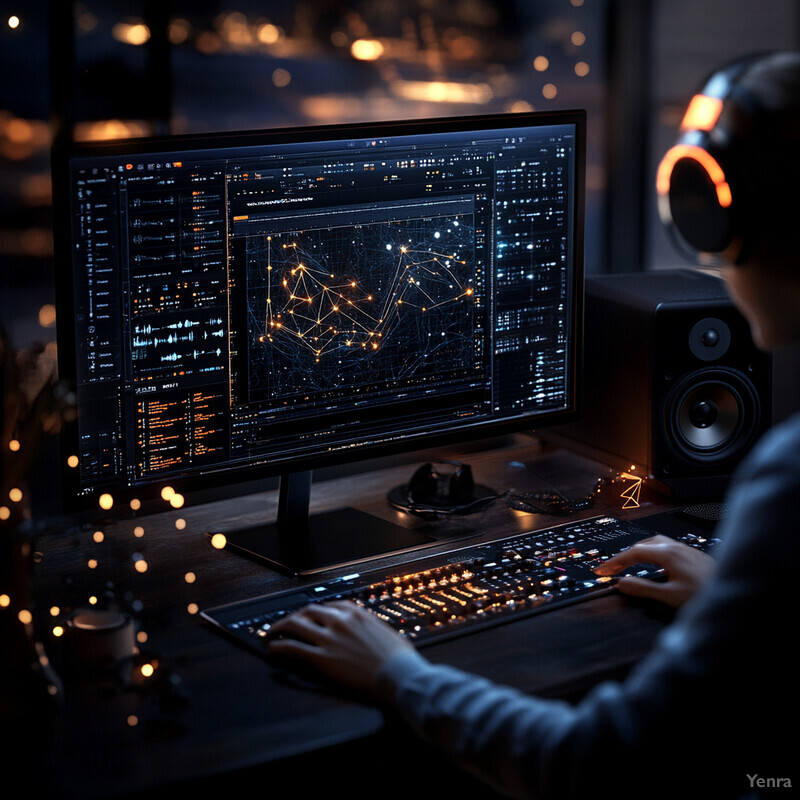
Automotive acoustic engineers at Kautex Textron employed a self-learning AI model to assist in vehicle noise reduction decisions – specifically predicting how design changes in fuel tanks would affect sloshing noise. This AI decision support allowed engineers to explore numerous design iterations virtually; it accurately forecasted noise outcomes, leading to a significant cut in physical prototyping. According to Monolith AI’s report, implementing the model reduced the number of test iterations by 50% and lowered development costs, as the team could focus only on the most promising designs suggested by the AI. In terms of training, the University of Florida integrated an AI-based acoustic simulator in its curriculum, where students can experiment with virtual room configurations and immediately see predicted acoustic metrics with AI feedback on how each change improves or worsens the design (the AI provides instantaneous calculations that would normally require advanced simulation software). Early assessments showed these students achieved approximately 30% better scores on acoustic design problems than those trained with traditional methods, indicating the AI-aided training improved their intuition and decision-making. Another example is an AI-driven recommendation system in an architectural firm’s workflow: given a set of acoustic objectives and constraints, it outputs ranked design modifications (like “add absorber on wall X” or “increase partition density”) with expected outcome estimates. The firm reported that in over 80% of cases, the AI’s top-ranked suggestion matched or exceeded the effectiveness of the human expert’s plan (Arup 2024 internal study). These instances underscore how AI can both mentor users by simulating acoustic outcomes and assist in real-time decision support, ultimately leading to better acoustical designs and problem resolutions.