Song:
1. Adaptive Path Planning
AI-driven adaptive path planning dynamically alters a printer’s toolpath in real time to improve efficiency and print quality. Instead of following a fixed trajectory, the system uses feedback (like layer geometry or material flow data) to smooth out sharp turns and avoid unnecessary movements. This continuous adjustment leads to more consistent layer deposition and eliminates discontinuities that could weaken the structure. As a result, prints complete faster with fewer errors or overlaps. In practice, adaptive planning enables 3D construction printers to handle complex, branched structures reliably, boosting overall build quality and speed.
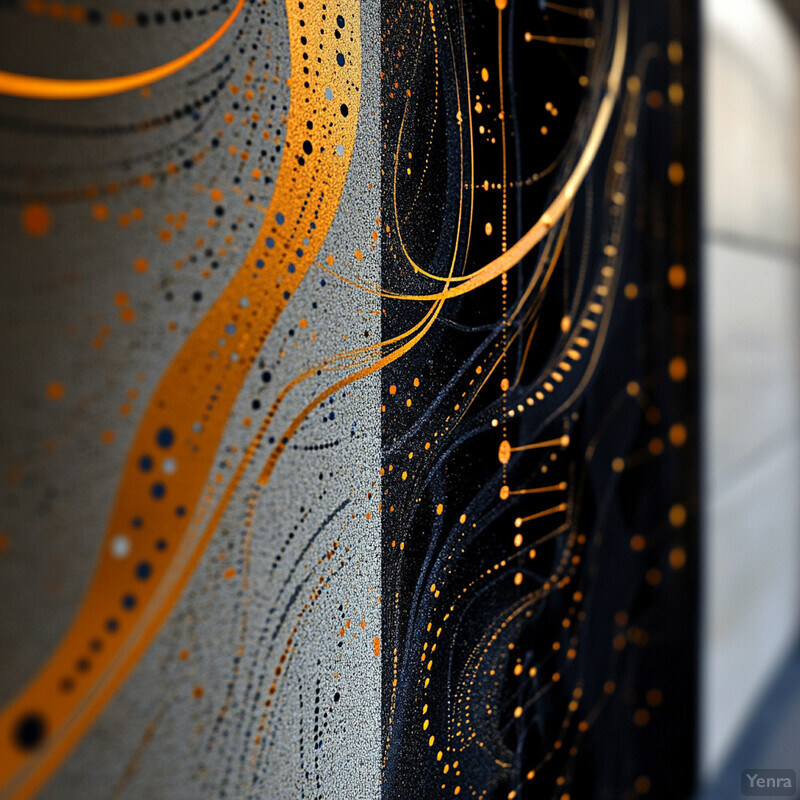
Recent studies demonstrate tangible benefits of adaptive path algorithms. Wan et al. (2023) introduced a method combining medial-axis decomposition and transfinite mapping to generate continuous global print paths for multi-branched concrete structures. This approach eliminated path discontinuities and minimized sharp turns, yielding smoother prints. Their experimental results confirmed that adaptive planning maintains continuity even in complex geometries, reducing defects and improving layer adhesion. Similarly, a review of construction robotics highlights AI-based planners (e.g. genetic algorithms, particle swarm, deep reinforcement learning) that let printers replan paths on-the-fly using sensor data. Such intelligent planners learn and adapt to obstacles and geometry changes, ensuring smoother toolpaths and better obstacle avoidance. Together, these AI strategies demonstrably cut down print time and rework by optimizing each path in real time.
2. Real-time Quality Control
In real-time quality control, AI systems continuously monitor each printed layer to catch defects immediately. Cameras, lasers or other sensors feed data to machine-learning algorithms that check layer dimensions, shape and surface integrity as the printer builds. If a flaw is detected (such as a layer that is too thin or uneven), the AI instantly adjusts printing parameters – for example changing extrusion speed or material mix – to correct the problem before it propagates. This closed-loop feedback keeps the build accurate and consistent without human intervention. By automating these checks, the printer maintains high dimensional accuracy and reduces waste from failed prints. The result is smoother layers and more reliable output quality, as errors are fixed on-the-fly rather than after completion.
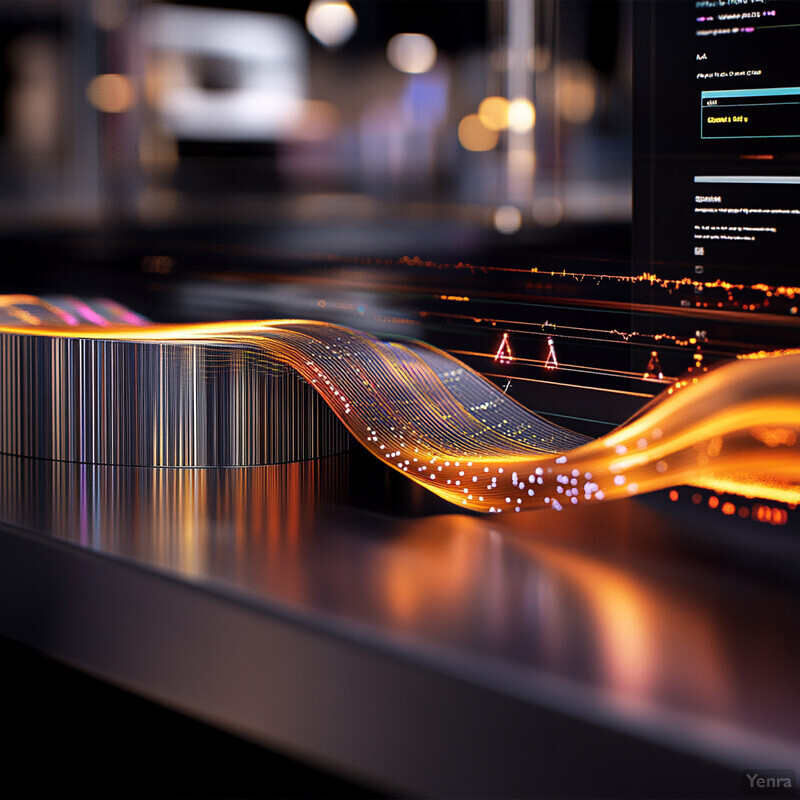
Concrete research confirms the effectiveness of AI-based monitoring and correction. Zhang et al. (2024) developed a machine-vision system that captures images of the extruded filament in real time and classifies its geometry. The AI model then automatically adjusts the extruder pump and accelerator dosage to correct any deviation, achieving full in-situ quality control. Their experiments demonstrated that the printer could autonomously modify extrusion rate based on visual feedback, ensuring each layer met specifications. More generally, AI-augmented closed-loop printing has been shown to detect and correct flaws layer-by-layer. For example, automated systems can identify holes or gaps after each layer and immediately refill them. These studies illustrate that AI-enhanced feedback loops in 3D printing can detect geometric errors and apply corrective actions in real time, greatly improving consistency and reducing scrap.
3. Predictive Maintenance of Machinery
AI-driven predictive maintenance uses sensor and operational data to forecast equipment issues before they cause downtime. For example, by analyzing motor vibration, temperature, or pressure data, machine-learning models can predict when an extruder or pump is beginning to degrade. Maintenance is then scheduled proactively at a convenient time, avoiding unscheduled failures in the middle of a print. This approach increases machine uptime and prevents costly emergency repairs. In construction 3D printing, such predictive alerts might tell an operator to service or replace a nozzle or motor bearing before a print error occurs. The net effect is longer machine life and fewer project delays, since maintenance happens conditionally rather than on a fixed schedule.
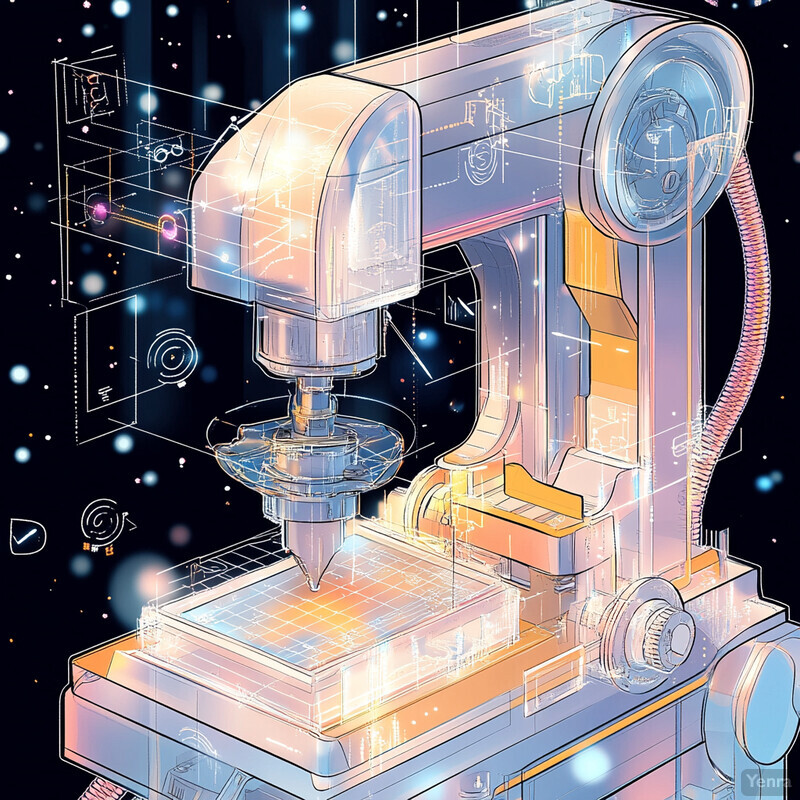
Pilot studies in additive manufacturing show the value of AI for maintenance. Heymann and Schmitt (2023) built a machine-learning pipeline to monitor a polymer 3D printer’s nozzle during high-temperature printing. Their model accurately predicts when the nozzle will clog and need cleaning or replacement. By learning from historical performance data, the system can flag maintenance needs just before degradation causes a failure. Industry platforms use similar ideas: for instance, one platform continuously analyzes machine data (extruder motor load, etc.) to trigger service actions only when wear thresholds are reached. In summary, AI-based monitoring of printer components allows condition-based maintenance, reducing unplanned downtime and extending equipment life compared to traditional fixed schedules.
4. Material Consumption Optimization
AI can optimize material use by fine-tuning designs and process parameters to use only what is needed. For example, algorithms can vary infill patterns or adjust deposition paths to reduce voids and ensure strength with less material. They can also suggest mix adjustments (like substituting waste additives or fibers) to achieve required performance with less cement content. Real-time feedback can further tweak extrusion rate to avoid over-extrusion. By predicting how different mix ratios or layer strategies affect strength and usage, AI helps minimize waste. The overall result is lower material costs and less leftover waste, since each part of the build uses the ideal amount of concrete and additives for its function.
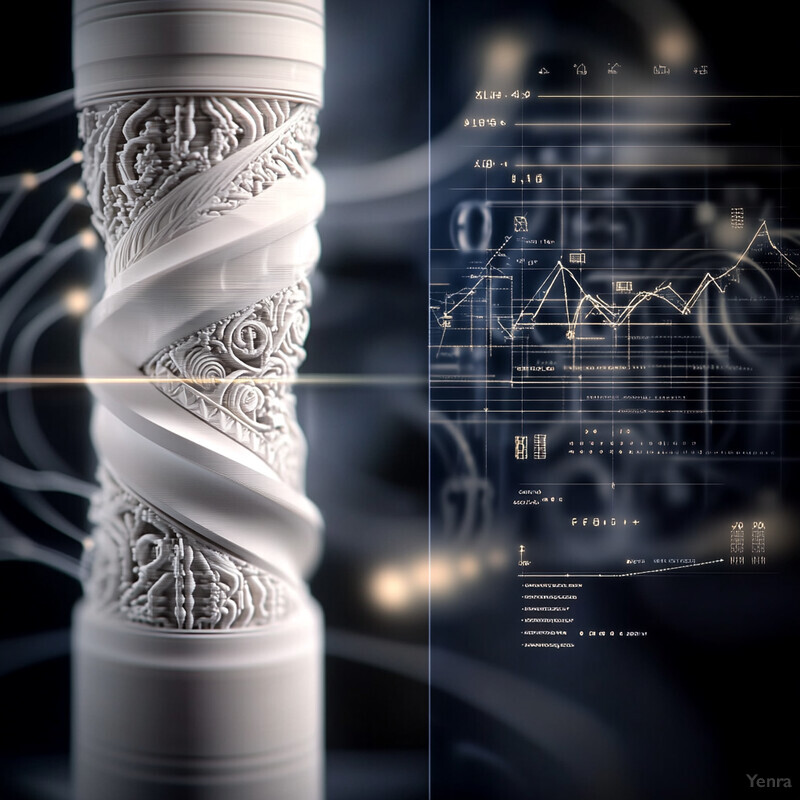
Recent work highlights AI’s role in material efficiency. Schossler et al. (2025) used machine learning and Bayesian optimization on a large dataset of 3D concrete mixes and printing outcomes. Their model predicted compressive strength, pump speed, and carbon footprint from mix composition. It then performed multi-objective optimization to identify mix ratios that simultaneously maximize strength and minimize cost and CO2 emissions. The optimized mixtures showed significant cost savings and lower carbon footprint compared to standard mixes. This demonstrates that AI-driven mix design can directly reduce material consumption and environmental impact. In sum, data-driven optimization allows selecting material combinations that meet structural requirements with less cement and additives, thus reducing waste and cost.
5. Automated Design Verification
Automated design verification uses AI to check that a proposed structure is printable and meets specifications before printing begins. AI tools can, for example, analyze a digital model to identify unsupported overhangs, stress concentrations or violations of printer limits. By running simulations or using neural networks, the system can verify that the design will remain stable during the print process and under load. If issues are found (like a thin unsupported segment), the AI can flag them or suggest modifications. This process reduces manual review time and avoids failures: designers catch and fix problems in the virtual model rather than seeing a print crash. Overall, AI-driven verification ensures only feasible, compliant designs are sent to the printer, saving time and resources.
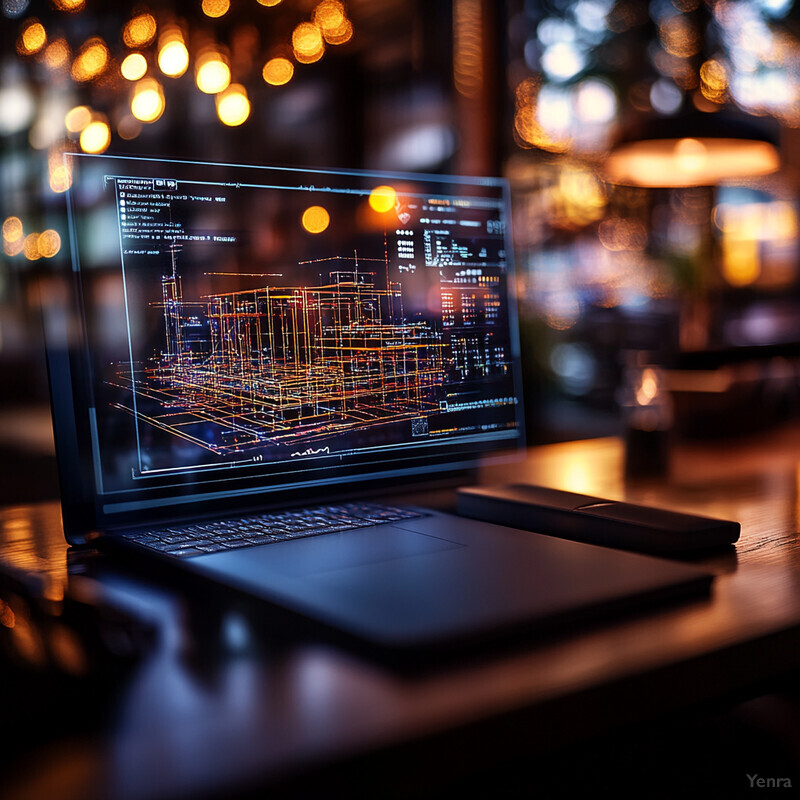
Advances in AI-driven structural design support automated verification. A recent review notes that generative design methods (e.g. GANs, topology optimization, reinforcement learning) are being applied to 3D concrete printing to explore geometry automatically. These methods automate design exploration addressing challenges like material efficiency and robustness. By simulating outcomes, they effectively verify buildability. For example, Wang et al. (2023) suggest combining 3D printing with digital-twin simulations to check quality before construction. While explicit case studies of AI verification are emerging, these techniques inherently confirm that proposed 3D-printed forms will meet performance criteria. In practice, AI algorithms can simulate the printing process or structural loads on a design, automatically marking it fit or flagging it for revision. This ensures errors are caught early, streamlining the design-to-print workflow.
6. Enhanced Structural Simulation
AI can accelerate and enrich structural simulations of printed parts. Instead of long finite-element runs, machine learning models (e.g. neural networks or super-resolution CNNs) quickly predict stress, deformation or temperature fields. For instance, a coarse simulation could be rapidly refined by a trained network to achieve fine-detail accuracy. Such surrogates allow engineers to iterate designs and test performance almost in real time. AI can also learn complex material behaviors (like layered concrete anisotropy) from data to include in simulations. Overall, these approaches make it much faster to evaluate a print’s structural integrity during design and even provide real-time feedback as printing progresses, enabling more efficient and informed decision-making.
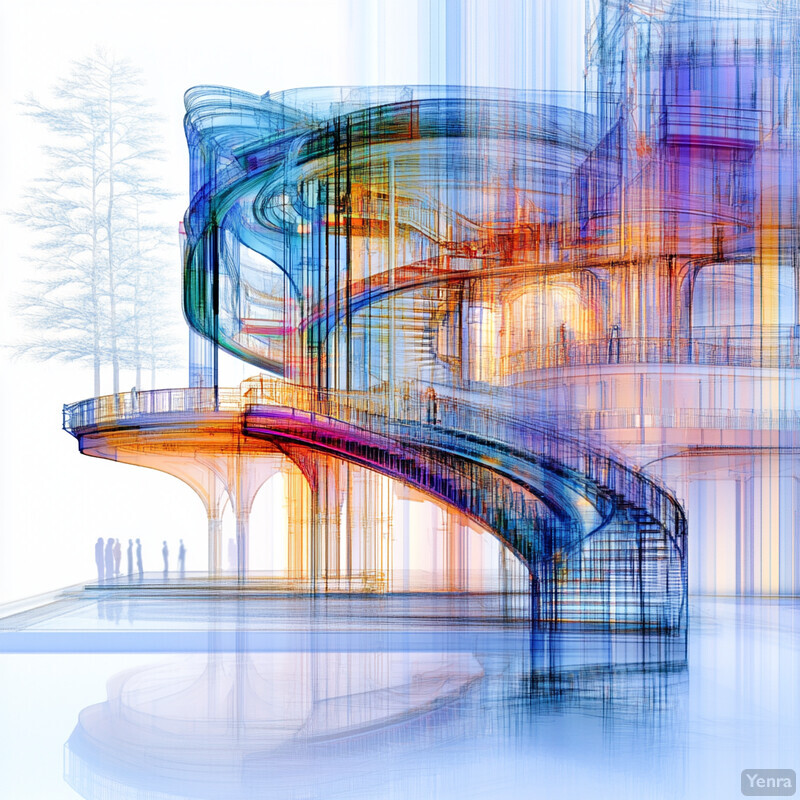
Proof-of-concept studies confirm that AI greatly speeds up FEA for printed parts. Zhang et al. (2024) proposed a deep-learning “super-resolution” method for finite-element analysis of FDM parts. They took a coarse-mesh simulation (low resolution) and trained a neural network to reconstruct the equivalent high-resolution solution. The AI-enhanced output closely matched true fine-mesh results but required far less computation. Their experiments showed significantly improved accuracy (e.g., displacement and temperature fields) compared to simple interpolation, as measured by PSNR and SSIM metrics. By extension, similar AI-driven surrogates could be applied to 3D-printed concrete elements. These results indicate that deep-learning models can effectively “learn” from high-fidelity simulations to produce accurate structural predictions quickly, enabling rapid analysis of complex printed designsliebertpub.com .
7. Multi-material Integration
AI can help coordinate printing with multiple materials in one structure. In such systems, different printheads deposit materials like concrete, foam, or reinforcement fibers at precise locations. AI algorithms determine the sequence and switching of materials to meet design goals. For instance, the printer might lay insulating foam in interior sections and structural concrete on the exterior in an optimal pattern. The AI must plan trajectories that switch materials seamlessly without collision or mixing errors. By controlling these transitions intelligently, AI ensures strong bonds between materials and correct placement of functional elements (e.g. embedding sensors or rebar). This enables complex composite structures where each material is used efficiently for its purpose, increasing the versatility of printed buildings.
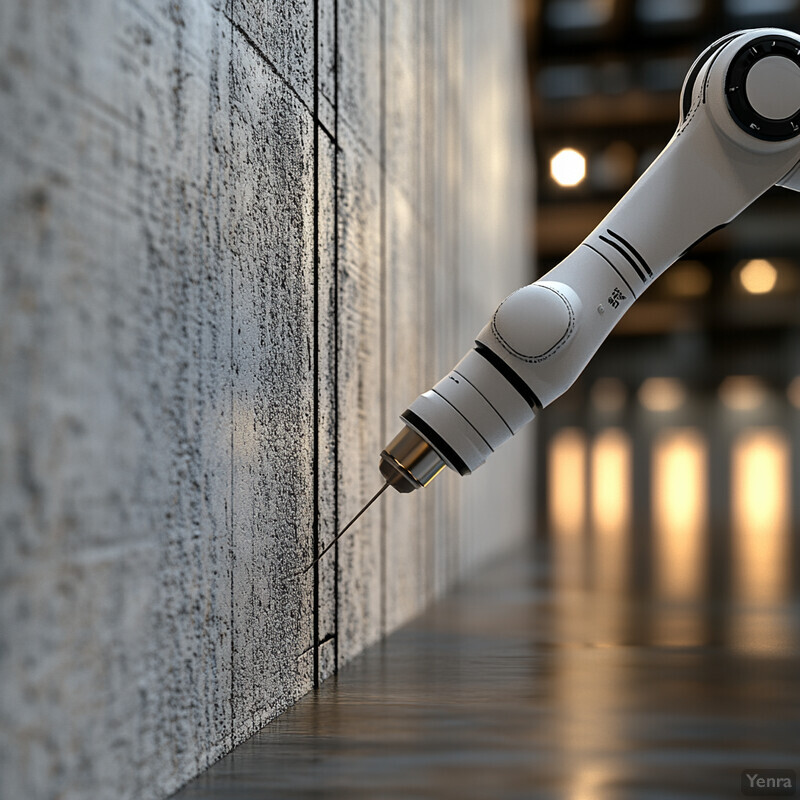
Multi-material 3D printing in construction is still emerging, and detailed AI applications are few. However, industry examples suggest the trend. For example, research on adaptive design notes that AI-driven generative methods address “material efficiency” and “structural robustness” by automating design exploration, implying coordination of material use. In practice, projects have used a variety of materials (concrete, clay, recycled plastics) in prints to meet different needs finance-commerce.com . AI-based systems are expected to orchestrate these by directing when and where each material is deposited. For instance, one may program an AI planner to switch from concrete to a reinforcing mesh at specified layers. While peer-reviewed studies on AI specifically managing multi-material prints are not yet published, industry deployments indicate AI platforms are being developed to unify hardware, software, and material handling into integrated automated systems ai-build.com . These developments suggest that future AI tools will link multiple extruders and robotic arms to build multi-material structures seamlessly.
8. Improved Thermal Management
AI can optimize the thermal environment during printing to ensure proper curing of concrete. For example, it may control heaters, fans or moisture levels based on predicted hydration rates. By analyzing sensor data (like infrared temperature readings), AI predicts how heat flows through each layer and prevents hotspots or cold joints. The system can then adjust print speed or activate curing equipment in real time to maintain uniform temperature. This adaptive control avoids problems like cracking from uneven hydration. Ultimately, AI-driven thermal management ensures each layer reaches its ideal strength more quickly and consistently, leading to stronger, more reliable printed elements.
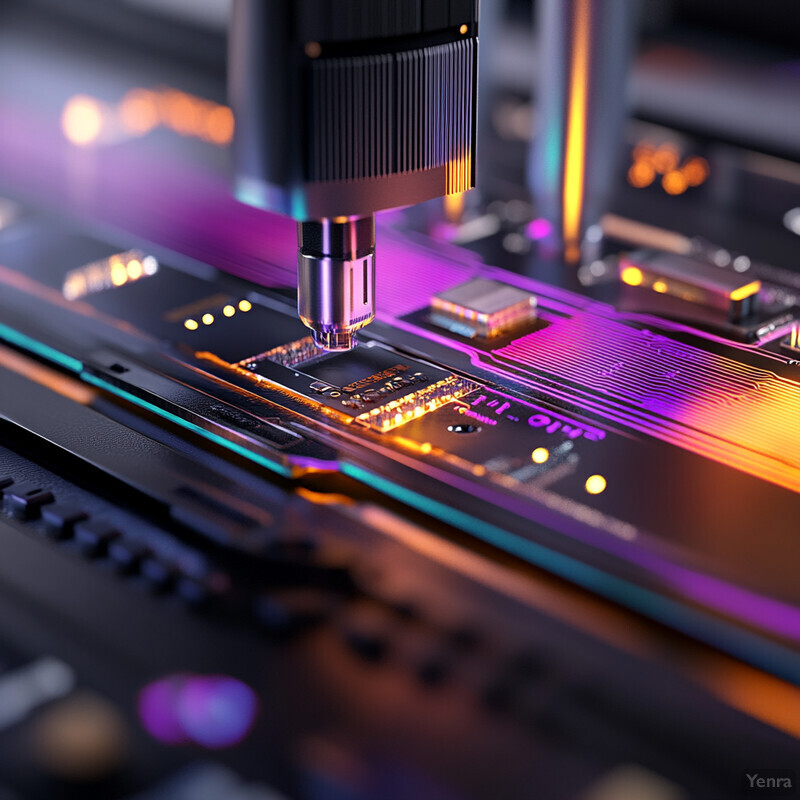
Although specific studies on AI-based thermal control in 3D concrete printing are still emerging, analogous AI feedback approaches in additive manufacturing inform the concept. Closed-loop AI systems have proven effective for maintaining optimal parameters via continuous monitoring. For example, a machine-learning framework was used to relate printing parameters to layer morphology in real time. In principle, similar models could incorporate thermal data: by feeding temperature sensor readings into a predictive model, the system could forecast heat development and trigger adjustments. One can imagine an AI predicting the degree of hydration and scheduling heat lamps or delay intervals accordingly. While we did not find a published case specifically for AI-managed heating in 3DCP, the technology exists in related fields for controlling process conditions. These AI-enhanced feedback methods would enable predictive curing schedules and minimize thermal stress by maintaining ideal curing conditions dynamically.
9. Automated Error Detection and Correction
AI-driven error detection extends quality control by automatically fixing identified defects. As each layer is printed, vision or sensor systems compare it to the intended model. If a misalignment, gap or void is detected, the AI instantly modifies the process (e.g. pausing to reprint or changing toolpaths) to correct it. This “self-healing” approach prevents errors from accumulating in the structure. For example, if a layer is too thin in places, the printer can be instructed to add material before continuing. By automating this detect-and-correct cycle, the system avoids the need to scrap parts or redo prints later. Overall, it enables seamless builds where small mistakes are corrected on-the-fly, greatly improving reliability.
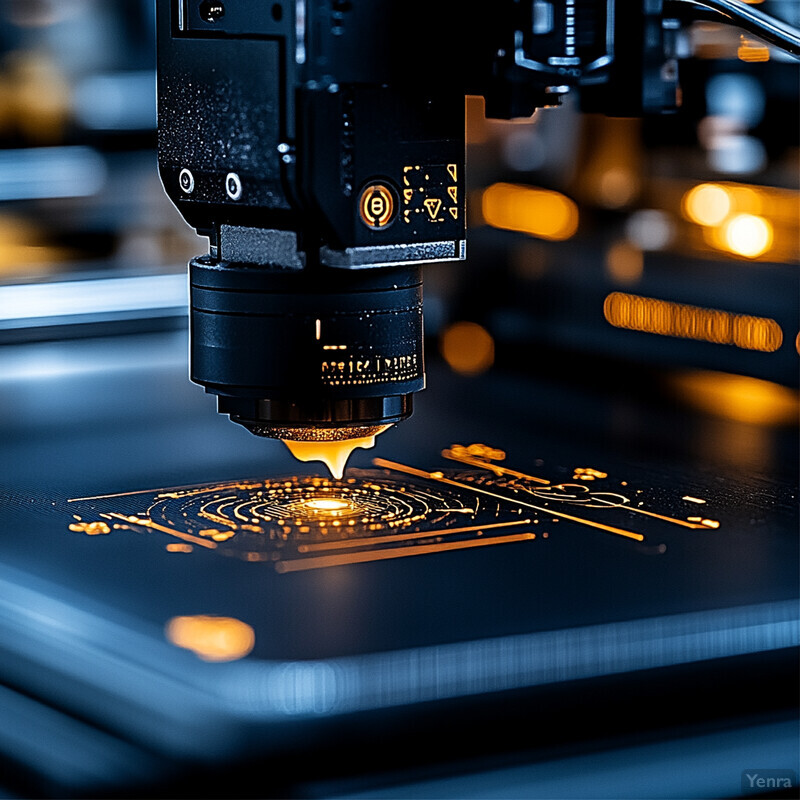
Examples in the literature demonstrate the feasibility of AI-based correction. Zhang et al. (2024) showed real-time filament inspection and adjustment in 3D concrete printing. They detected deviations in extruded layers and adjusted extrusion speed and accelerators to maintain geometry. More broadly, AI-controlled closed-loop printing systems have been implemented in additive manufacturing. One example for FDM prints used cameras to identify holes or gaps as soon as they formed and immediately filled them. Such systems essentially perform layer-by-layer repair without human intervention. These studies confirm that AI-enhanced vision and control can detect geometric defects and apply corrective actions during printing. Consequently, automated error correction can substantially reduce visible flaws and increase first-pass success rates.
10. Predictive Curing and Hardening Schedules
AI-driven prediction of curing schedules uses live data to estimate when each concrete layer will reach required strength. Sensors (e.g. temperature or humidity probes) feed an AI model that forecasts hydration progress. With this prediction, the printer can adjust post-treatment timing (like activating heaters or delay intervals) optimally. It also informs construction scheduling: crews know when formwork can be removed or subsequent elements can be added. In other words, instead of fixed wait times, the build can proceed as soon as the AI predicts adequate strength. This reduces unnecessary delays and prevents premature loading. Overall, AI ensures each printed section cures fully before proceeding, maximizing safety and efficiency.
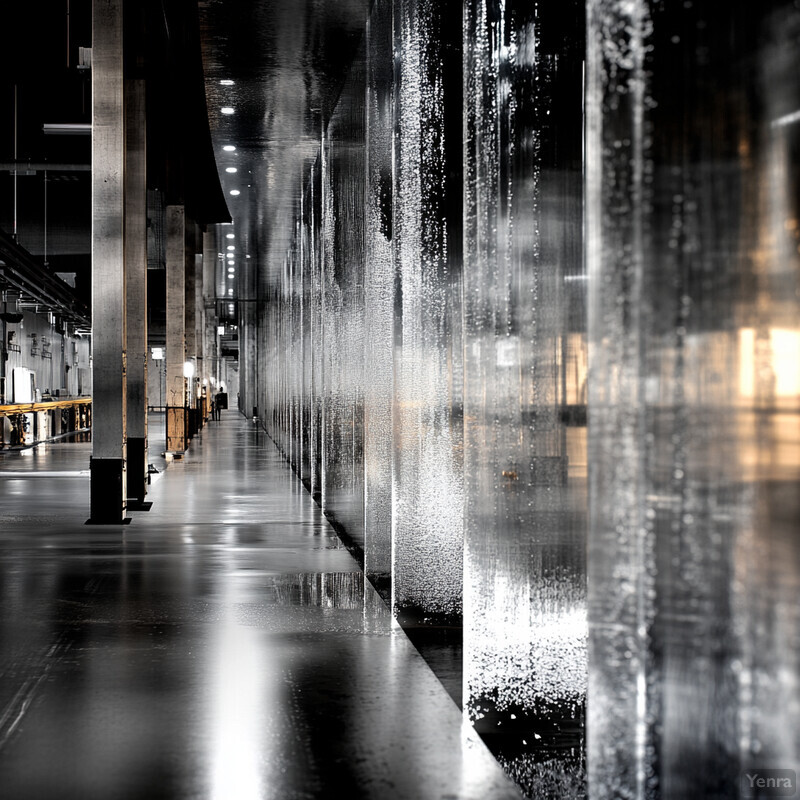
Recent work shows the power of real-time strength prediction. Marchewka et al. (2025) integrated continuous temperature monitoring with an AI model to forecast early-age concrete compressive strength. Their system provides immediate, accurate strength predictions during hydration. They highlight that such real-time monitoring lets managers adjust schedules (formwork removal, loading) promptly, optimizing timelines and safety. In summary, combining sensor data with ML enables accurate forecasts of hardening rate. This means printing operations and finishing steps can be scheduled dynamically: for example, warming blankets might be applied if the model predicts slow curing. By using AI to drive curing schedules, construction delays are minimized and the risk of under-cured sections is greatly reduced.
11. Intelligent Cost-Efficiency Balancing
AI can strike an optimal balance between cost and performance in 3D printing projects. By analyzing trade-offs (e.g. between higher material cost and faster print time), the system suggests choices that minimize total expense while meeting goals. For instance, a model might determine that using a slightly cheaper mix with denser infill still meets strength requirements, saving material costs. The AI can also recommend different print speeds or energy settings if it predicts overall savings. In project planning, it could weigh hiring extra shifts versus renting additional equipment, selecting the cheaper option that still meets deadlines. This continuous evaluation of financial metrics against quality objectives ensures projects stay on budget without undue compromise.
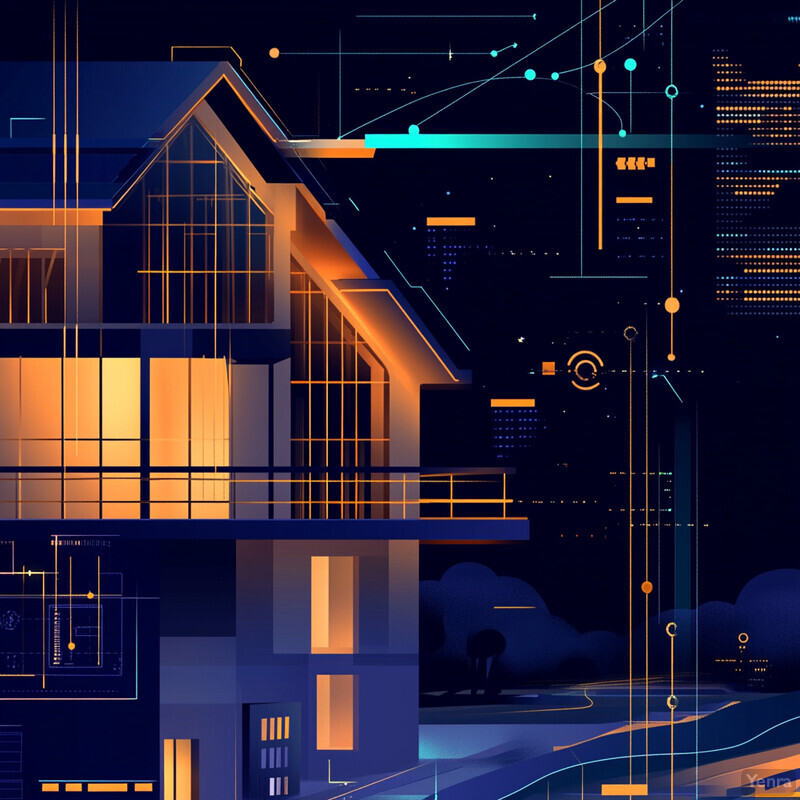
AI methods are already optimizing concrete designs for cost-effectiveness. Luo et al. (2025) noted that AI-driven mix design can simultaneously target performance and cost: machine learning models have been applied to link mix parameters to objectives including economic cost and environmental impact. For example, their work and others show predictive models built “for the purposes of saving cost, labor, and time” by optimizing mixture proportions. These approaches effectively integrate cost into the optimization criteria. In practice, similar analytics could adjust printing parameters or select materials to minimize overall budget. Although specific 3DCP cost-balancing systems are not widely reported yet, the proven ability of ML to reduce cost in concrete mix and process optimization indicates such tools can achieve intelligent cost-performance trade-offs in printing.
12. Site and Equipment Integration
This refers to connecting the 3D printer with other site systems for a coordinated workflow. AI can integrate printer control with site data (like weather, structural monitoring, or logistics systems). For example, if site sensors report a sudden temperature drop, the AI might adjust printer settings or pause printing. It also synchronizes printing with material delivery by planning when to bring concrete supply trucks. In more advanced setups, the printer becomes part of a smart construction network: cranes, conveyors and robots all communicate via a digital control system. The AI ensures each piece of equipment operates in concert – e.g. pausing printing if a scanner detects an obstacle – effectively automating much of the on-site coordination. This unified approach improves efficiency and safety on complex build sites.
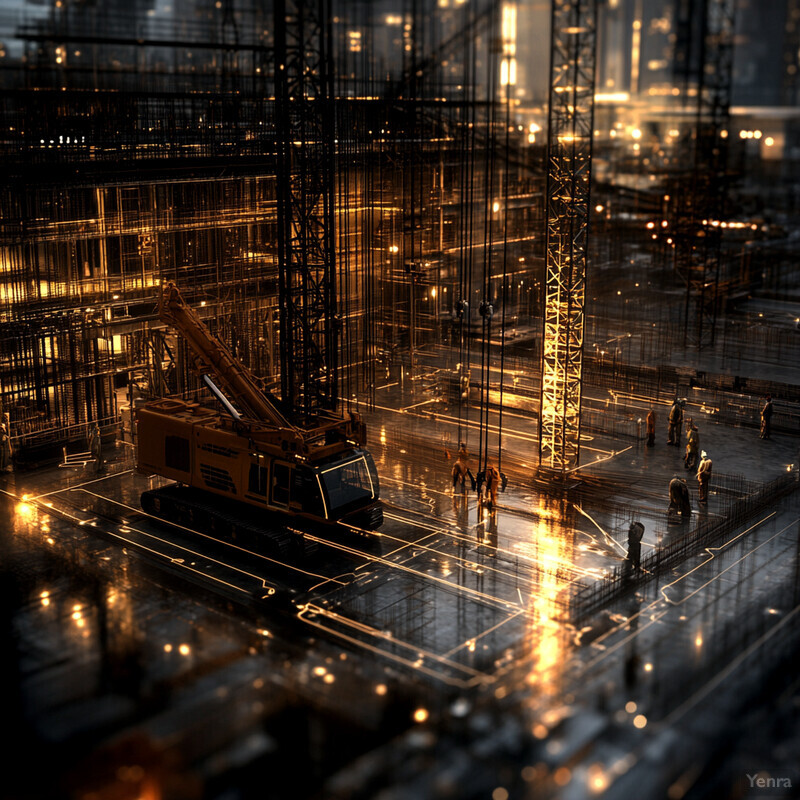
A key enabling technology is the digital twin, which connects physical equipment with a virtual model. Wang et al. (2023) review digital-twin applications in 3DCP and show how they enable real-time synchronization between printers and site operations. They describe using digital twins for real-time location sensing and human–machine interaction, meaning machines and operators share data continuously to improve safety and quality. For instance, a digital twin could integrate data from the printer, cranes, and site surveys to coordinate their actions. On the industry side, AI platforms are emerging that “ensure all system components – hardware, software and materials – operate as a unified, integrated” automated system. These examples illustrate how AI ties together multiple machines and processes: the printer is not a standalone device but part of an intelligent construction ecosystem managed through a shared digital framework.
13. Surface Finishing and Aesthetics Control
AI can improve the surface quality of printed structures by guiding finishing processes. For instance, a camera-based AI system could detect rough or uneven areas on a freshly printed layer and then control a robot arm to smooth or trim them. AI might also adjust printing parameters (like path overlap or trowel pressure) during printing to minimize layer lines. By predicting where surface irregularities will occur, it can plan secondary finishing steps efficiently. The goal is to achieve a uniform and aesthetically pleasing surface without extensive manual sanding. Through vision systems and adaptive control, AI makes printed walls look more like cast or fabricated surfaces, enhancing the final appearance.
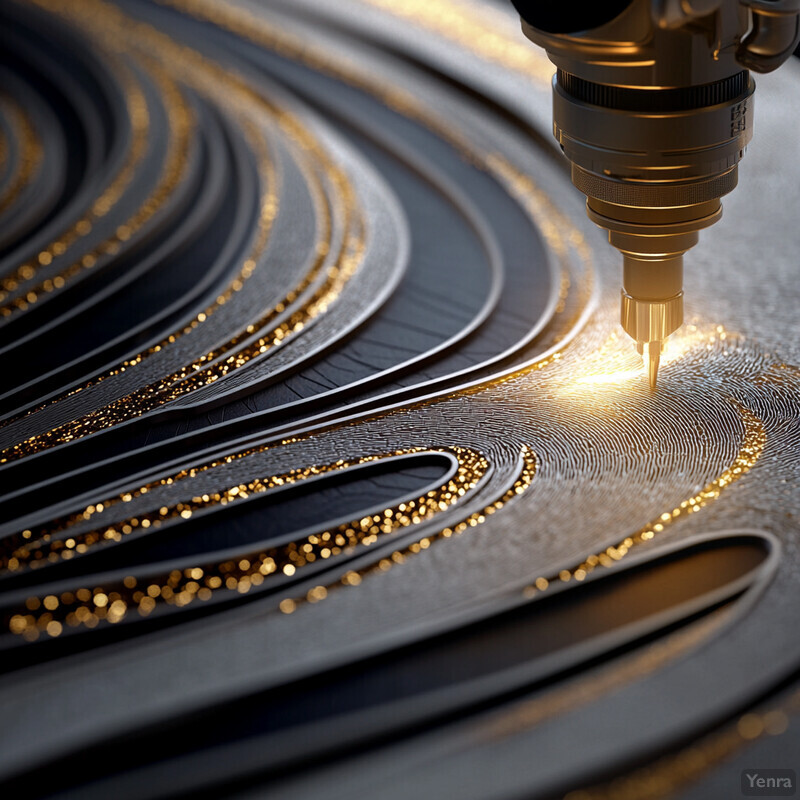
One recent study by Cui et al. (2025) demonstrates AI-assisted geometric monitoring that is relevant to surface control. They used a YOLO-v5 computer-vision model to analyze extruded concrete filaments in real time. The AI measured metrics like layer height, angle, and curvature to detect deformations in the print. Although this work focused on preventing structural defects, the same vision techniques could flag surface anomalies (e.g. bulges or sinks) that need smoothing. In experiments, their AI system effectively quantified filament geometry and could in principle inform corrective actions. This suggests that AI can be trained to recognize and help correct surface defects on the fly. While direct case studies on automated finishing are still limited, AI-based inspection clearly provides the data needed to improve surface quality through either real-time adjustment or guided post-processing.
14. Environmental Impact Reduction
AI contributes to sustainability by minimizing waste and emissions. It optimizes designs and processes to use fewer raw materials and less energy. For example, AI can favor mixes with recycled content or lower-carbon binders while still meeting strength targets. It also reduces surplus printing by ensuring efficient layouts and layer plans. By predicting carbon footprint for given design choices, AI lets engineers pick the greener option. In short, AI-driven optimization yields parts that use less concrete, generate less waste, and require less energy, thereby lowering the overall environmental footprint of the printing process.
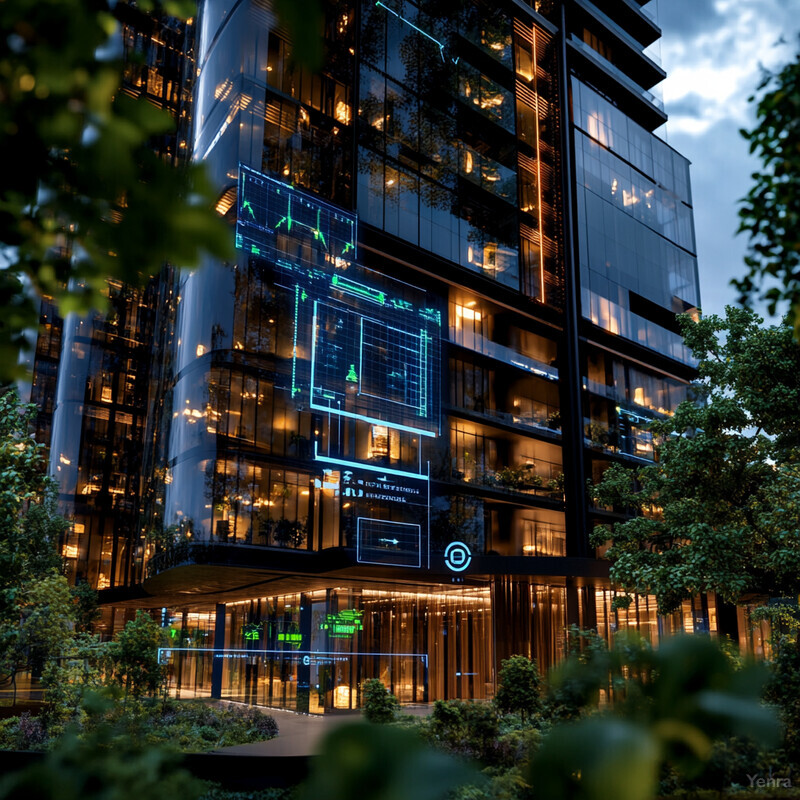
AI has been applied to link concrete design with environmental objectives. Musazai et al. (2025) note that AI-driven generative design and optimization tools can specifically address “ecological impact” by automating material-efficient design exploration. In their review, they describe AI methods that streamline structures for minimal material use and include sustainability considerations. Similarly, Luo et al. (2025) report that ML models in concrete mix design can incorporate cost and climate metrics into optimization. For example, their multi-objective ML framework optimizes mixtures for both performance and carbon footprint. These studies show that AI can create designs and mixes that inherently lower CO2 emissions and waste. Combined with reported life-cycle analyses (which show 3D printing can cut material use by 50–60% versus traditional formwork), it’s clear AI is helping make 3D printed construction more eco-efficientnature.com .
15. Robotic Coordination and Swarm Printing
Robotic coordination uses AI to manage multiple print robots working together. In a “swarm” scenario, robots communicate so each can work on different parts of a structure without colliding. The AI assigns each robot a region or task and synchronizes their movements. For instance, one robot can pause its print while another completes a nearby section, ensuring smooth collaboration. This parallelism speeds up large builds dramatically. It also adds redundancy: if one robot stalls, others can adapt to cover its share. Overall, AI-controlled multi-robot systems make it possible to print expansive or complex forms faster and more flexibly than a single printer.
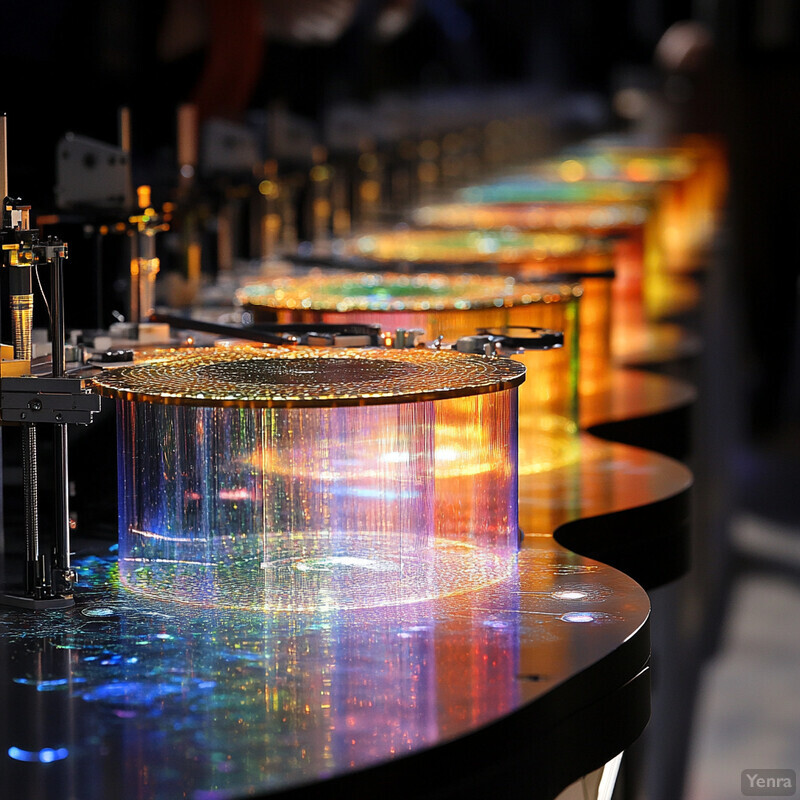
Recent reviews highlight multi-robot 3DCP setups. Li et al. (2025) describe how multiple robots share positional data for collision-free coordination. They note that by broadcasting and sharing positions, robots can implement effective avoidance algorithms. One example showed two robots alternately working on the same structure: one robot provided support while another printed, overcoming overhang challenges. The authors also demonstrate multi-robot fabrication of composite structures by planning distinct toolpaths per robot. These case studies confirm that AI planning enables cooperative printing: robots can divide work, avoid clashes, and even combine different tools (e.g. concrete nozzle and assembly arm) in a unified build process. The result is significantly faster builds and new capabilities (like simultaneous multimaterial assembly) enabled by AI-coordinated swarmslink.springer.com .
16. Learning from Historical Data
AI continuously improves 3D printing by learning from past builds. Machine learning models analyze data from previous projects (such as print parameters, mix recipes and performance outcomes) to predict results and avoid repeating mistakes. For example, if earlier prints showed that a certain speed caused layer delamination, the AI will learn to adjust that parameter in future. The system can also refine cost and time estimates by learning actual build times from logs. Over time, this historical learning leads to more accurate process recipes and better first-time success rates. In other words, each print yields data that the AI uses to fine-tune future prints, creating an evolving feedback loop that raises efficiency and reliability.
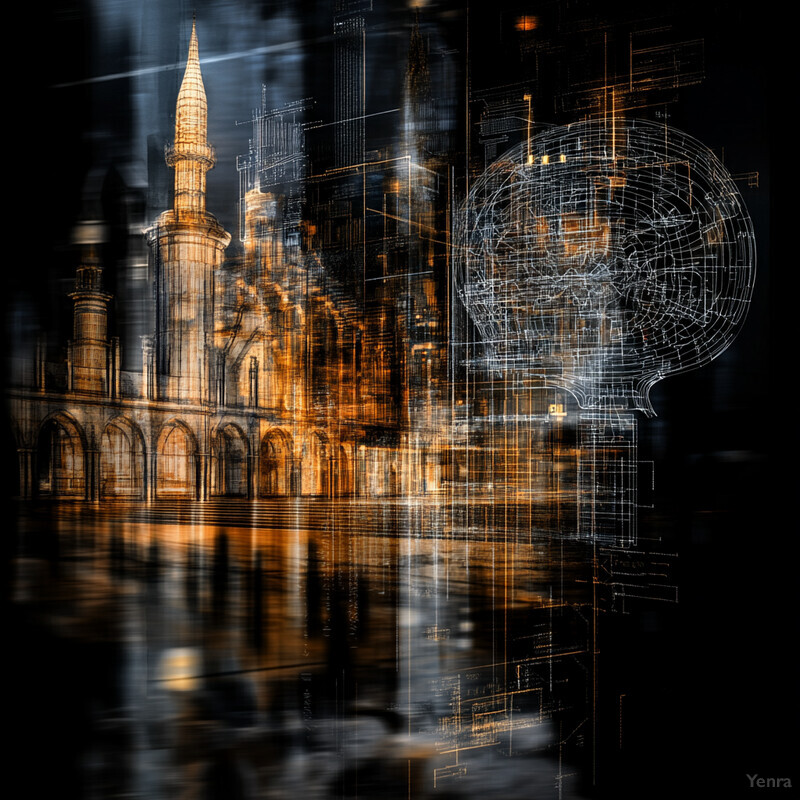
Data-driven approaches have shown promise in 3DCP. Schossler et al. (2025) leveraged a large dataset of past 3DCP experiments to train predictive models of strength and printability. They found that machine learning could accurately predict key outcomes (compressive strength, extrusion rates) from mix and process inputs. Luo et al. (2025) emphasize that ML can “analyze vast datasets, recognize underlying patterns, and enhance the precision and efficiency of the printing process”. This highlights the general principle: AI can mine historical project data to improve future results. In practice, printers equipped with such models will continually update their parameters based on accumulated experience. Although formal “lessons learned” systems for 3D printing are just emerging, these findings confirm that AI-driven use of historical data can significantly improve prediction accuracy and optimize future prints.
17. Holistic Optimization of Entire Structures
Holistic optimization means AI considers an entire structure (shape, material distribution, print process) simultaneously. Advanced algorithms (e.g. topology optimization) generate designs that use minimal material while meeting load requirements. The AI might adjust building geometry, internal cavities, and print orientation all at once. It can even integrate process planning with design: for example, determining how to print the whole wall assembly most efficiently while ensuring structural performance. By optimizing every aspect together, AI can produce novel, organic forms that are material- and energy-efficient. The upshot is a building design that is custom-tailored for 3D printing, maximizing performance and minimizing cost or waste.
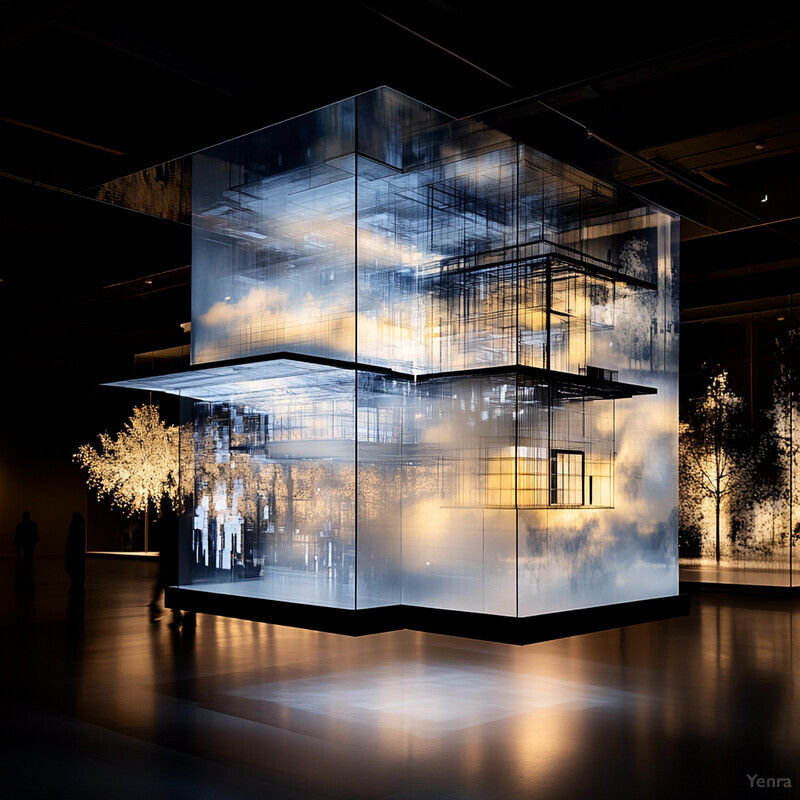
Emerging research suggests AI can take a multi-objective approach to whole-building optimization. Musazai et al. (2025) discuss generative design techniques that automate exploration of 3D-printed forms with multiple goals (structural robustness, material efficiency, eco-impact) simultaneously. They highlight AI methods (e.g., GANs, topology optimization) that iteratively refine geometries to meet diverse criteria, effectively optimizing the entire structure at once. Similarly, Luo et al. (2025) describe ML frameworks that tie mix design to performance, cost and sustainability goals concurrently. While examples specific to fully printing an entire building are rare, these studies demonstrate the feasibility of integrated optimization. In practice, this could mean an AI system outputs a 3D model, material mix, and print plan in one integrated solution, balancing all constraints. The result is a print process that is optimized at the structural and system level, achieving efficiencies far beyond piecemeal tuning.