1. Improved Prediction Accuracy
AI is significantly boosting the accuracy of weather forecasts by learning complex patterns that traditional models may miss. Machine learning techniques can ingest decades of data to correct model biases and refine predictions at various scales. National weather agencies and tech companies alike have begun deploying AI-driven forecasting systems that often match or exceed the skill of conventional numerical models. This improvement means forecasters can better anticipate weather events and provide more reliable guidance. Ultimately, AI-enhanced forecasts reduce uncertainty, allowing communities and industries to make safer and more cost-effective decisions based on the weather.
AI algorithms analyze vast amounts of data from satellites, weather stations, and historical weather events to predict weather patterns more accurately.
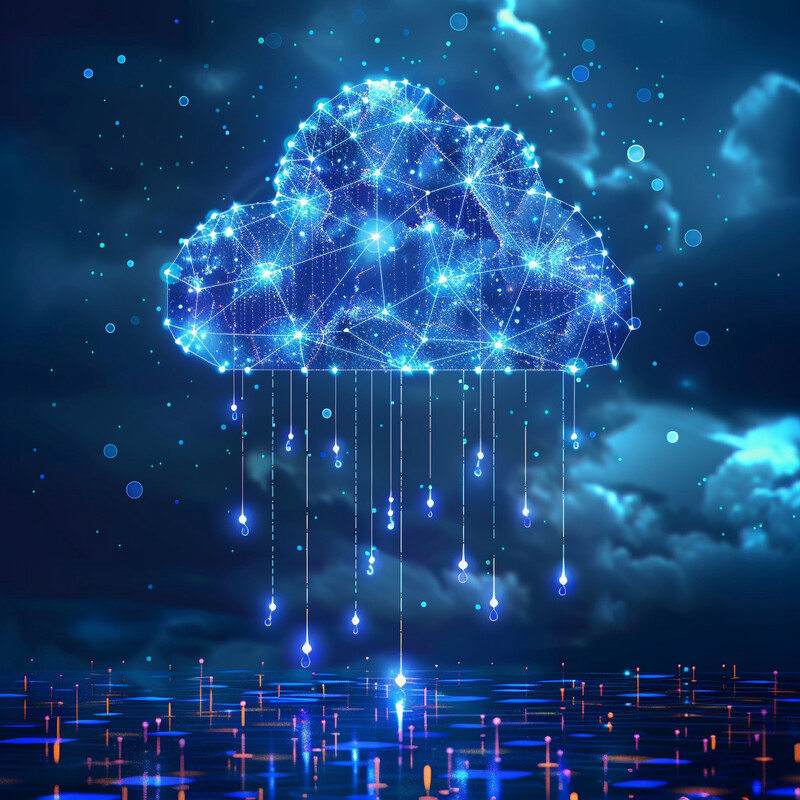
Early operational results show that AI-based forecasts can outperform standard models in key metrics. For example, the European Centre for Medium-Range Weather Forecasts reported that its new AI Forecasting System was about 20% more accurate for certain weather phenomena than its leading physics-based model. Likewise, a 2025 study introduced “GenCast,” an AI weather model that outperformed ECMWF’s own ensemble forecast on 97.2% of evaluated targets – including improved prediction of extreme weather and hurricane tracks. These machine-learning models learn from vast reanalysis datasets, enabling them to discern subtle atmospheric signals that improve forecast precision. By reducing errors, especially in challenging scenarios, AI-driven tools are pushing forecast accuracy to new heights and building trust in weather information.
AI significantly improves the accuracy of weather forecasts by analyzing massive datasets from satellites, weather stations, and historical weather records. Machine learning models identify patterns and trends that human forecasters might miss, allowing for more precise predictions of weather phenomena. This not only increases forecast reliability but also enhances the ability to predict rare or extreme weather events.
2. Real-time Data Processing
AI enables meteorologists to process incoming weather data and update forecasts in real time. Modern weather prediction involves an overwhelming volume of observations (from satellites, radars, ground sensors, etc.) that must be rapidly assimilated into forecast models. Machine learning algorithms can streamline this data flow by instantly recognizing patterns and anomalies, which speeds up the forecasting cycle. By using AI, meteorological services can provide updates and nowcasts (very short-term forecasts) almost as soon as new data arrives. This immediacy is crucial for time-sensitive decisions, like alerting the public to sudden storms or aviation hazards, and it marks a shift toward more responsive, continuously updated weather forecasts.
AI enables the processing of data in real-time, allowing meteorologists to update weather forecasts more frequently and with greater precision.
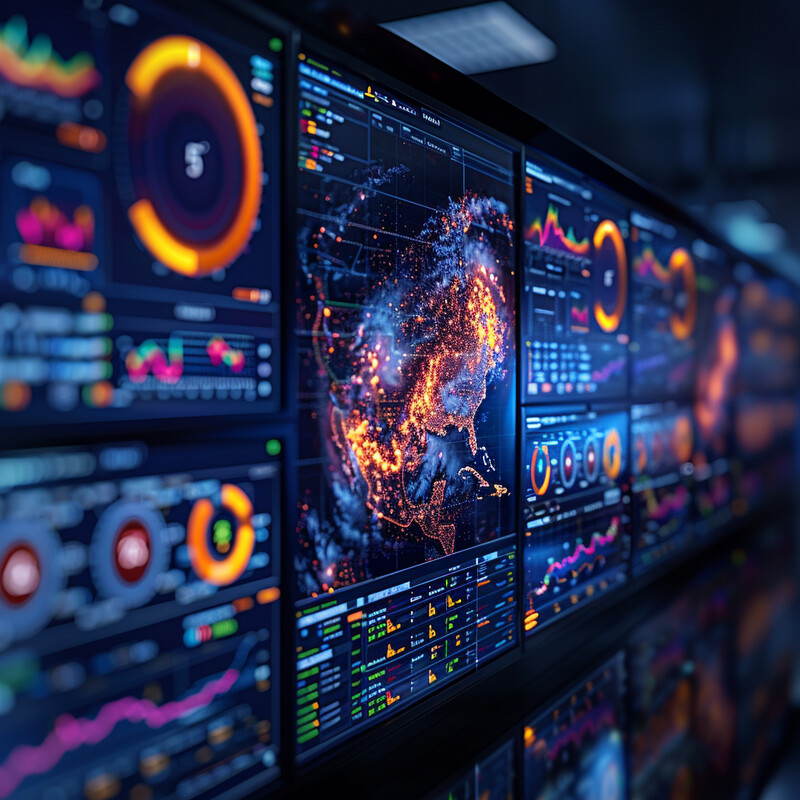
AI-driven nowcasting systems demonstrate how quickly forecasts can be generated from real-time data. Google researchers, for instance, developed a neural network that uses recent radar imagery to produce high-resolution precipitation forecasts with only 5–10 minutes of total latency. This machine-learning model generates 1-km scale rainfall predictions nearly instantaneously once trained, dramatically faster than traditional numerical models that might take an hour or more to run. Similarly, NOAA scientists are testing AI systems that can ingest fresh radar and satellite data and output local forecasts in under a minute. By shortening the time between observation and prediction, AI enables forecasters to issue timely updates – potentially providing critical minutes of lead time for fast-developing weather events.
AI excels in processing vast amounts of meteorological data in real-time. This capability allows for the continuous updating of weather models as new data arrives, leading to more accurate and timely weather forecasts. Real-time processing is crucial during fast-changing weather conditions, providing up-to-the-minute updates that are essential for emergency response and public safety.
3. Severe Weather Prediction
AI is improving the prediction of severe weather events – such as thunderstorms, tornadoes, and flash floods – by recognizing early signals and complex meteorological patterns that precede these dangers. Traditional forecasting of severe weather relies on expert interpretation of radar, satellite, and model data, but machine learning models can augment this by rapidly analyzing huge datasets (including historical storm events) to spot subtle precursors of severe outbreaks. The result is earlier and more confident warnings for events like severe thunderstorms, extreme rainfall, and tropical cyclones. By extending warning lead times and honing in on at-risk areas, AI-enhanced severe weather prediction helps emergency managers and the public act sooner to protect life and property.
AI helps identify signs of severe weather events like hurricanes, tornadoes, and blizzards earlier, giving communities more time to prepare and potentially saving lives.
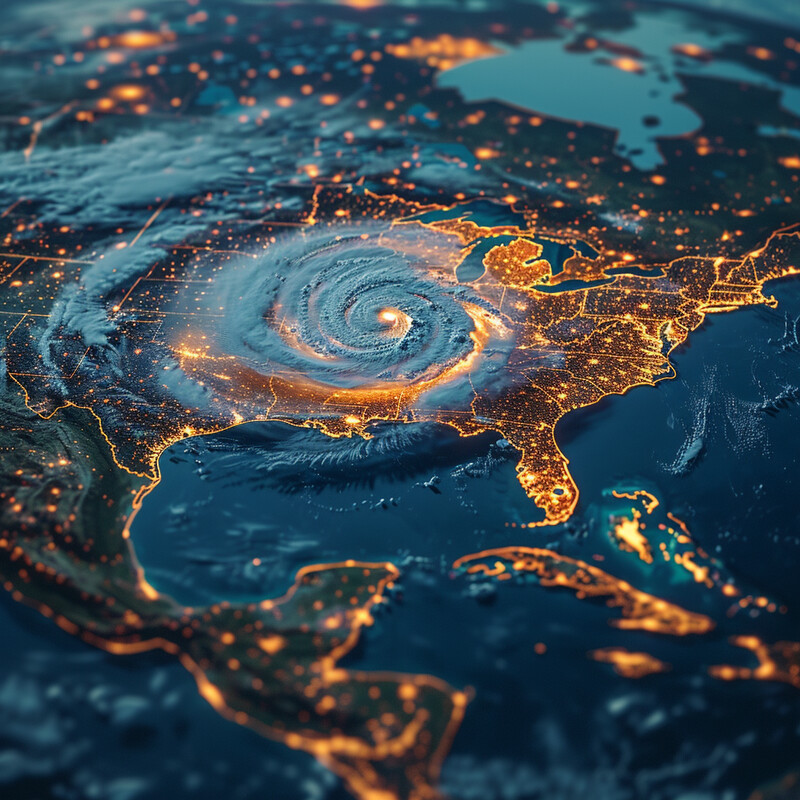
Experimental AI systems have shown promising gains in forecasting hazardous weather. NOAA’s National Severe Storms Laboratory, for example, developed an AI model called “WoFSCast” that predicts thunderstorm evolution up to 2 hours in advance, and the system generates a new forecast in less than one minute. This speed and foresight could lengthen tornado and hail warning lead times, giving communities additional minutes to seek shelter. In another study, researchers combined deep learning with physical modeling to improve extreme rainfall forecasts: their “NowcastNet” model outperformed several leading nowcast methods in about 70% of cases when predicting intense precipitation events, as verified by dozens of meteorologists in a blind test. These examples illustrate how AI can dissect storm dynamics more effectively, from pinpointing where a tornadic thunderstorm will travel to estimating how much rain a stalled storm will dump. By catching on to severe weather signals that humans or slower models might miss, AI systems enhance our ability to issue timely and accurate severe weather warnings.
AI enhances the prediction of severe weather events, such as hurricanes, tornadoes, and blizzards, by quickly analyzing environmental conditions that precede these events. Early detection through AI helps in issuing timely warnings to communities, potentially saving lives by giving people more time to take necessary precautions or evacuate if needed.
4. Climate Trend Analysis
Beyond short-term forecasts, AI is being applied to analyze climate trends and long-term weather patterns, improving our understanding and prediction of phenomena like El Niño, monsoons, and other climate oscillations. By sifting through extensive climate databases and model outputs, machine learning can identify subtle correlations and precursors that classical methods overlook. This is especially valuable for predicting slow-developing events (droughts, seasonal anomalies) and detecting signals of climate change in the weather record. AI tools are helping climate scientists extend prediction lead times for climate phenomena and reconstruct past climate extremes for which direct observations are scarce. Overall, integrating AI into climate analysis enhances our ability to anticipate significant shifts and extremes on seasonal to decadal scales, informing preparedness for climate-related risks.
AI analyzes long-term data to detect changes in climate patterns, aiding climate scientists in understanding and predicting global climate changes.
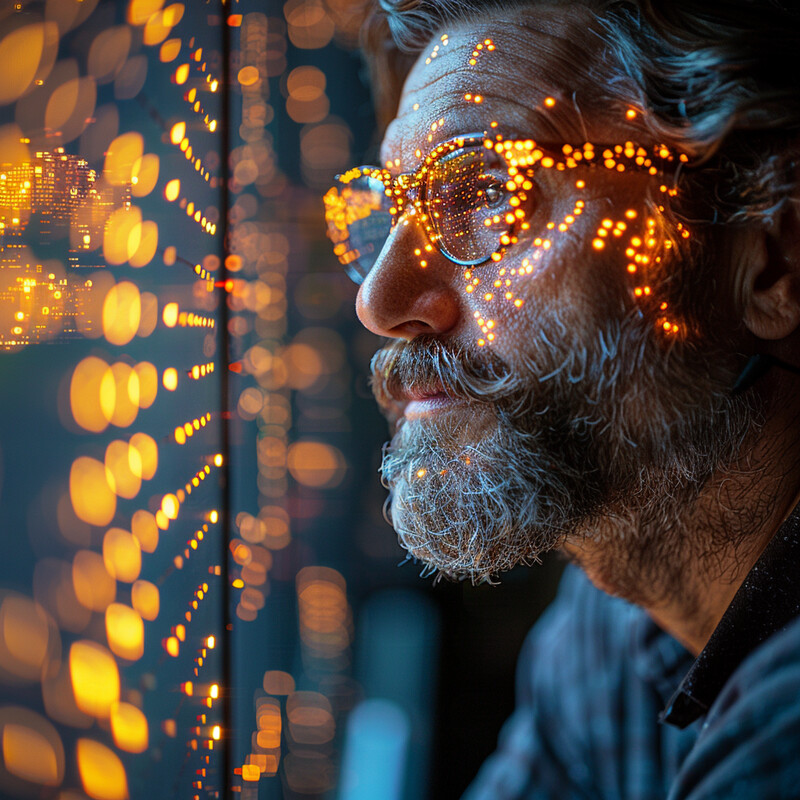
Recent research shows that AI can substantially improve forecasts of elusive climate patterns. In 2025, scientists reported a deep learning approach that successfully predicts the Atlantic Niño (an irregular Atlantic Ocean warming) up to several months in advance, something conventional climate models struggled to do. The AI model overcame longstanding biases in simulation and, for the first time, made it possible to forecast these Atlantic Niño events 2–3 months ahead with useful accuracy, according to the team. Climate experts hailed this result as a breakthrough, since earlier attempts using physics-based models had hit a dead end. By uncovering hidden links (for example, recognizing how conditions in the Pacific can serve as precursors for the Atlantic Niño), the AI provided a level of predictive insight that improves seasonal outlooks. This success with the Atlantic Niño mirrors progress in other areas – researchers are also using machine learning to analyze decades of climate data to better project trends in monsoons, Arctic sea-ice, and extreme events in a warming world.
AI algorithms process and analyze long-term environmental data to identify changes in climate trends. This analysis is vital for understanding global climate change, enabling scientists to make more accurate predictions about future conditions and helping policymakers make informed decisions about climate action.
5. Localized Weather Predictions
AI is enabling hyper-local weather forecasting, delivering predictions tailored to very specific locations – down to the city block or individual neighborhood. Traditional forecast models typically operate on larger regional grids, but machine learning can downscale outputs and incorporate local data (like topography and urban effects) to refine forecasts for microclimates. This means weather information can be customized for a small town, a rural valley, or even a single city intersection, accounting for factors like local terrain or coastlines that influence conditions. Hyper-local forecasts powered by AI benefit sectors such as agriculture, renewable energy, and city management, where knowing block-by-block weather (e.g. which street will flood or where the wind will gust most) is critically important. By providing granular detail that general models miss, AI-driven localized predictions help communities better prepare for and respond to weather on their doorstep.
AI can provide hyper-localized weather forecasts, down to individual neighborhoods or streets, which is crucial for precise agricultural planning, event planning, and disaster management.
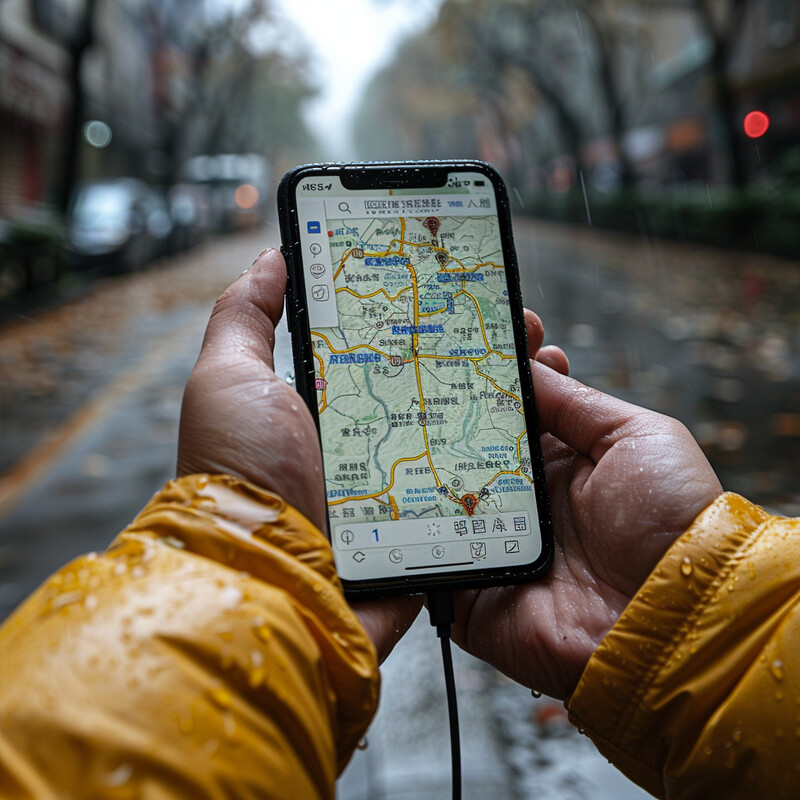
New AI weather services are delivering super-granular forecasts that would have been impractical before. One notable example is in Hong Kong, where researchers and a tech startup developed a system to predict rainfall and flooding at the individual street level. Their approach takes high-resolution rainfall forecasts and then uses machine learning to simulate flood inundation on every street in the city in under 3 minutes. Because the AI can run so quickly, these hyper-local flood forecasts can be updated in near real time and issued hours in advance of approaching storms. In practice, this means a resident could get a warning that “Main Street will likely flood by 3 PM,” rather than a more general city-wide alert. Insurers in the region are partnering in this effort to send targeted alerts – for instance, texting a car owner to move their vehicle from a specific garage that is forecast to flood. This kind of street-by-street prediction and notification was beyond the reach of traditional methods. Thanks to AI, hyper-local forecasts are now becoming a reality, empowering more proactive responses at the neighborhood level.
AI provides detailed, hyper-localized weather forecasts that can drill down to specific neighborhoods or even streets. This granularity is particularly beneficial for agricultural planning, urban planning, and managing local events, allowing for precise strategies to mitigate adverse weather impacts.
6. Integration with IoT Devices
The rise of Internet of Things (IoT) devices is providing a new wealth of weather data, and AI is crucial for integrating these unconventional data streams into forecasting. Millions of IoT sensors – from smartphone barometers and car windshield wipers to smart home weather stations and even signals from cellular towers – can act as micro weather observatories. AI algorithms can intelligently quality-control and assimilate this crowd-sourced data, which is often noisy and uneven, to enhance weather models. By doing so, previously data-sparse areas (such as rural regions or parts of the ocean) gain additional observations that improve local forecast accuracy. This blending of IoT data and AI effectively expands our weather monitoring network dramatically. It leads to more detailed initial conditions for models and enables hyper-local nowcasts (for example, detecting a sudden pressure drop from phones in a city that might indicate a developing storm). In short, AI + IoT is transforming everyday objects into valuable weather sensors and making forecasts more precise and personalized.
AI integrates data from numerous IoT sensors deployed across various locations, enhancing the granularity and accuracy of weather data collection.
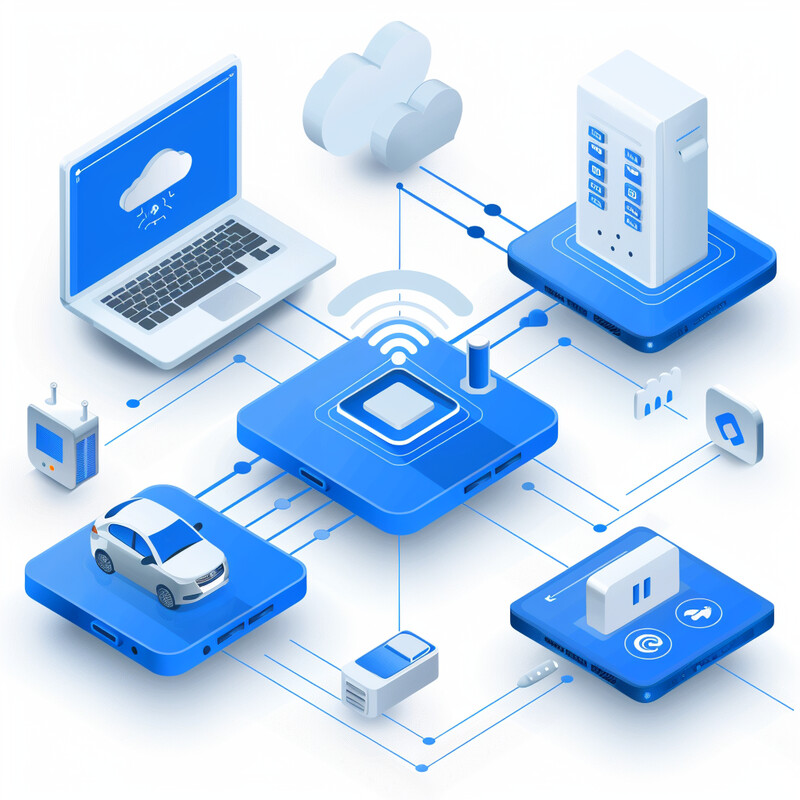
Major forecast providers have begun leveraging IoT data via AI to enrich their services. For instance, IBM’s The Weather Company uses a platform that analyzes data from IoT devices, mobile sensors, and even citizen observers to generate hyper-local forecasts. This means inputs like personal weather stations in backyards, pressure readings from smartphones, and car sensor data are automatically processed by AI to adjust and refine local weather predictions. Such a system can detect, say, a network of home rain gauges indicating higher rainfall in one part of a city and immediately incorporate that into updated forecasts for that neighborhood. The integration is already benefiting various industries: agriculture gets on-farm weather specifics, logistics companies receive route-specific hazard alerts, and energy utilities can forecast demand with neighborhood-level precision. By tapping into the IoT, forecasting systems augmented with AI cover microclimates that traditional instruments might miss, leading to more responsive and finely tuned weather updates for end-users.
By integrating with IoT devices scattered across various locations, AI enhances the collection and analysis of environmental data. Sensors can monitor everything from temperature to humidity, providing a denser, more comprehensive data network. This integration leads to better model accuracy and, consequently, more reliable weather predictions.
7. Enhanced Visualization Tools
AI is improving how meteorologists visualize and interpret weather data, leading to more effective analysis and communication. Complex model outputs and massive datasets can be difficult for humans to parse, but machine learning can highlight the most important features automatically. For example, AI image recognition techniques are being applied to weather maps and satellite images to identify patterns like fronts, storm cells, or jet stream positions and display them for forecasters. Instead of manually drawing boundaries or poring over multivariate charts, meteorologists can use AI-annotated maps that immediately show critical features (e.g. an algorithm drawing the probable location of cold and warm fronts on a map). Additionally, AI is enabling new forms of visualization such as augmented reality weather graphics and custom maps that adjust to a user’s needs. By turning raw data into clear visuals – whether for an expert or a public audience – AI helps ensure that key weather insights are not overlooked and are conveyed in an intuitive way.
AI develops sophisticated visualization tools that allow meteorologists and the public to see weather changes in real-time, making the data more accessible and understandable.
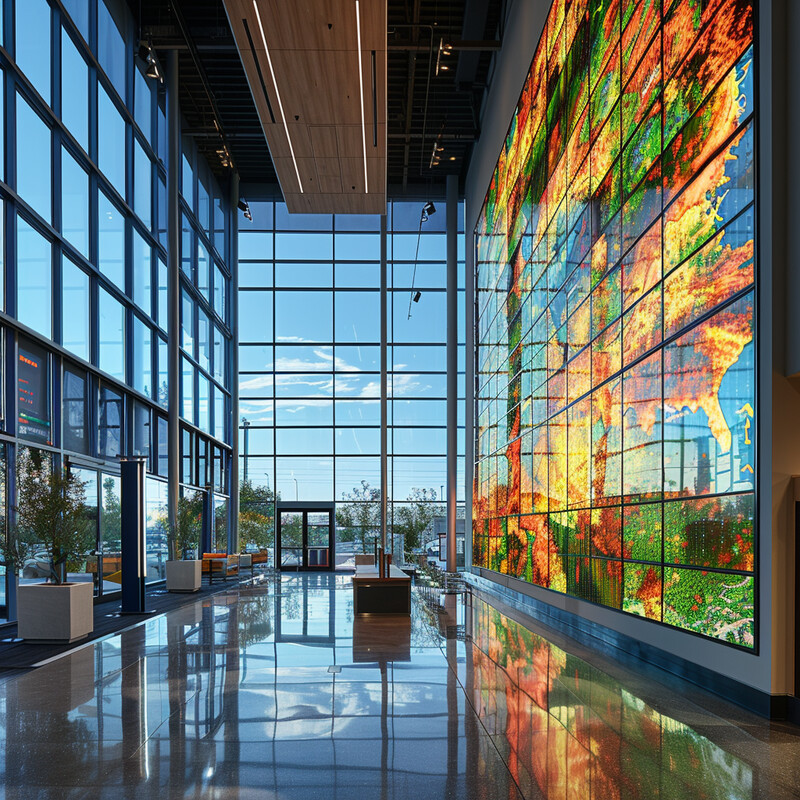
An example of AI-aided visualization is the automation of surface weather front analysis. Traditionally, identifying frontal boundaries (the lines between air masses) on weather maps has been a manual, time-consuming task for meteorologists. Now, an AI model called “FrontFinder” uses a neural network to instantly detect and classify fronts (cold, warm, stationary, and occluded fronts, as well as drylines) from atmospheric data. This tool is so effective that it’s being used in operations at NOAA’s Weather Prediction Center to assist human forecasters. FrontFinder can take in model output or reanalysis data and output a map with all the frontal lines drawn in the correct locations, essentially automating a routine analysis step. Forecasters then see an annotated map highlighting features of interest, which improves their efficiency and consistency. Beyond fronts, similar AI-driven visualization aids are emerging – from algorithms that track storm cell development on radar imagery (and outline those cells for easier monitoring) to AI that generates 3D hazard displays for pilots. These tools act like an extra set of eyes, guiding meteorologists to the most pertinent details and enhancing the clarity of weather presentations for decision-makers.
AI-powered visualization tools transform complex meteorological data into user-friendly, dynamic visual formats that are easy to understand. These tools are invaluable for both meteorologists, who can more effectively communicate weather changes, and the general public, enhancing awareness and preparedness.
8. Optimized Atmospheric Modeling
AI is revolutionizing the efficiency of atmospheric modeling, making weather predictions faster and less resource-intensive without sacrificing accuracy. Traditional numerical weather prediction (NWP) requires solving complex physics equations on supercomputers, which can take hours for each forecast run and consume vast computational resources. Machine learning offers a shortcut: once trained on historical data, an AI model can emulate the behavior of the atmosphere much more quickly than running a full physics simulation. This means forecasts can be produced in minutes or seconds, and with far less computing power. Such improvements not only cut costs and energy use (which is significant for large modeling centers) but also allow for more frequent updates and the possibility of running many forecast scenarios in parallel. Furthermore, AI models can be tuned to capture small-scale processes that standard models struggle with, effectively boosting resolution without the usual computational penalty. Overall, by optimizing the modeling process, AI is enabling a new generation of forecasting systems that are both high-speed and high-fidelity.
AI optimizes models that simulate atmospheric conditions, improving the prediction of weather phenomena by understanding complex interactions in the atmosphere.
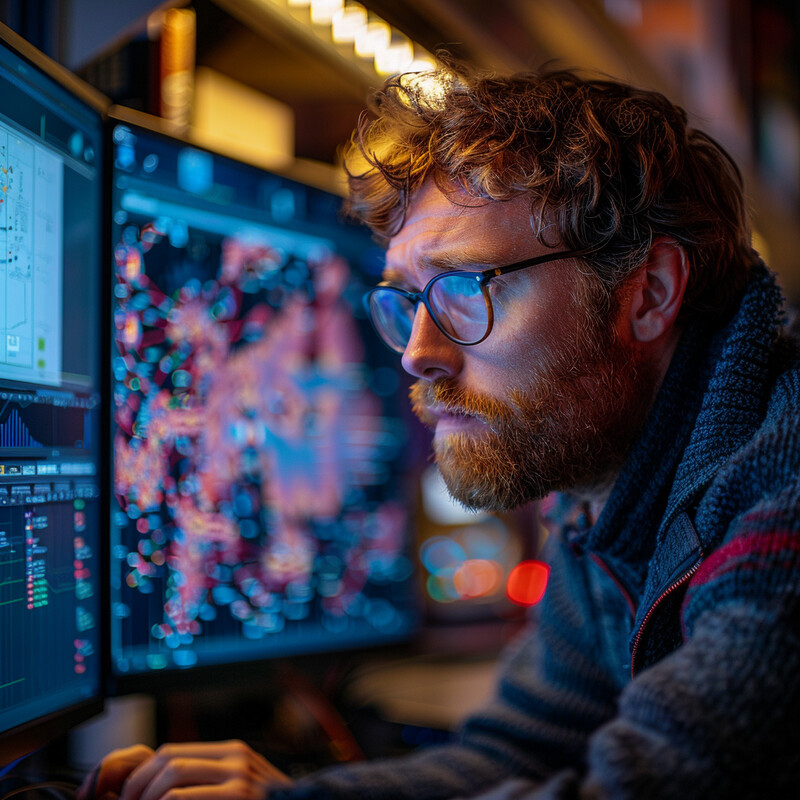
The efficiency gains from AI-based modeling are dramatic. The ECMWF’s first AI-enhanced global forecasting system demonstrated it could generate forecasts using about 1,000 times less computational energy than the traditional approach, all while maintaining or improving accuracy. In practice, what would have required a massive supercomputer can potentially be done on a much smaller machine or cloud setup when using AI. Similarly, Google DeepMind’s recent AI weather model (“GraphCast/GenCast”) was able to produce a 15-day worldwide forecast in roughly 8 minutes, compared to the hours of computing usually needed for that range. Despite its speed, this AI model didn’t compromise on skill – it actually matched or outperformed one of the world’s best numerical models in predicting key weather variables. These examples show that AI can drastically streamline number-crunching: forecast updates that once ran twice per day might be able to run hourly, and developing countries with limited supercomputing access could run sophisticated models on ordinary hardware. By optimizing calculations, AI-driven modeling is paving the way for more accessible and rapid weather forecasting, which is especially crucial when quick turnarounds are needed (such as in emergency weather situations).
AI optimizes atmospheric models that simulate air movements, temperature changes, and other atmospheric phenomena. Improved modeling helps meteorologists better understand and predict weather conditions by accounting for the myriad interactions that occur in the atmosphere.
9. Personalized Weather Updates
AI is making weather information more personalized than ever, tailoring forecasts and alerts to individual users’ needs and preferences. Instead of one-size-fits-all forecasts, AI allows weather communication to adapt on the fly – whether that means translating technical data into a user’s preferred language, focusing on specific weather parameters someone cares about (e.g. pollen count for an allergy sufferer), or delivering forecasts via the medium that user finds most useful (text, voice, chatbot, etc.). For example, AI-powered apps can learn that a given user is interested in morning running conditions, and then push a custom notification like “Ideal running weather at 6 AM tomorrow.” Personalization also involves making forecasts more accessible: using natural language generation to write location-specific forecast narratives or employing AI to automatically translate and disseminate warnings in multiple languages. By leveraging user data and machine learning, weather services can provide information that feels curated—helping people make better decisions based on forecasts that truly matter to them.
AI tailors weather updates and alerts to individual preferences and needs, such as specific alerts for pollen levels, air quality, or UV index, enhancing daily life management.
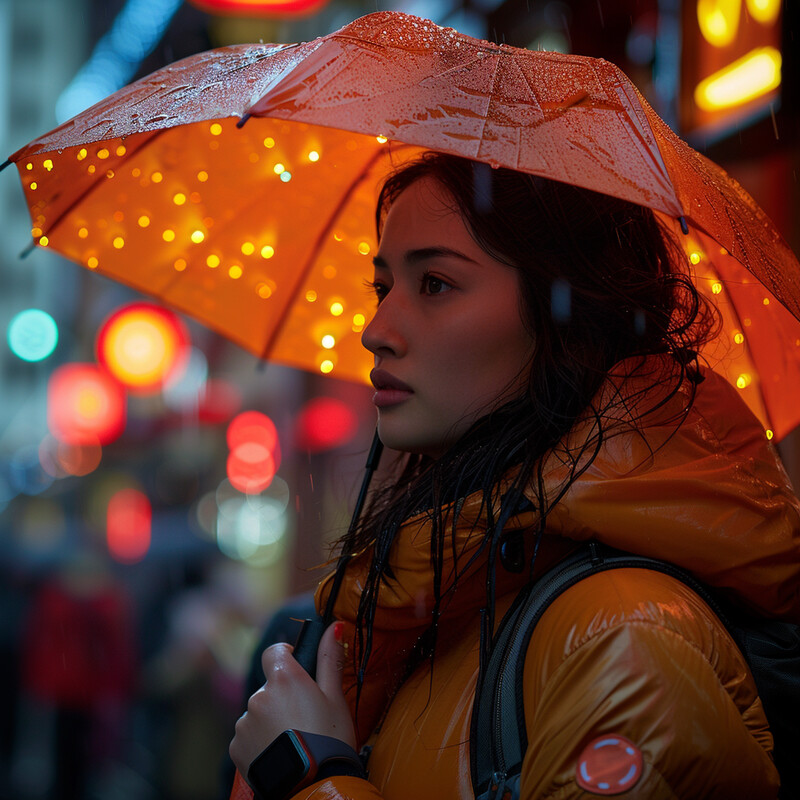
The U.S. National Weather Service has started to harness AI for tailoring forecast communications. In late 2023, NOAA began using an AI system to automatically translate weather forecasts into other languages (including Spanish and Simplified Chinese), ensuring that non-English-speaking communities receive timely and accurate warnings in their native tongue. This is a form of personalization at scale – adapting the content of forecasts to meet linguistic needs of different user groups. On the private sector side, consumer research underscores the demand for customized weather content: surveys found that roughly 69% of people are willing to share their ZIP code in order to get forecasts specific to their precise location. In response, companies have introduced AI-driven personal weather assistants (like smartphone apps and smart speakers) that can deliver hyper-local and user-specific updates. These systems might use a person’s exact GPS location, routine, or interests to filter weather data – for instance, sending an alert if rain is expected during the time of one’s usual commute, or providing farmers with crop-specific weather advisories. By using AI to interpret both user data and weather data, personalized forecast services make weather information more relevant and actionable for everyone.
AI tailors weather updates and alerts to the specific needs and preferences of individuals, taking into account factors like location, health concerns (e.g., pollen allergies), and personal schedules. This customization makes weather information more directly relevant and useful, aiding daily activity planning.
10. Automation of Routine Meteorological Tasks
Graham, E. (2025, April 11). NOAA incorporates AI for translations and tailored forecasting. Nextgov/FCW. / The Weather Company. (2023). Leveraging AI for hyperlocal weather content and forecasting (Blog post – SmithGeiger study cited).
AI automates the analysis and reporting of standard meteorological data, freeing up experts to focus on more complex weather analysis and public communication.
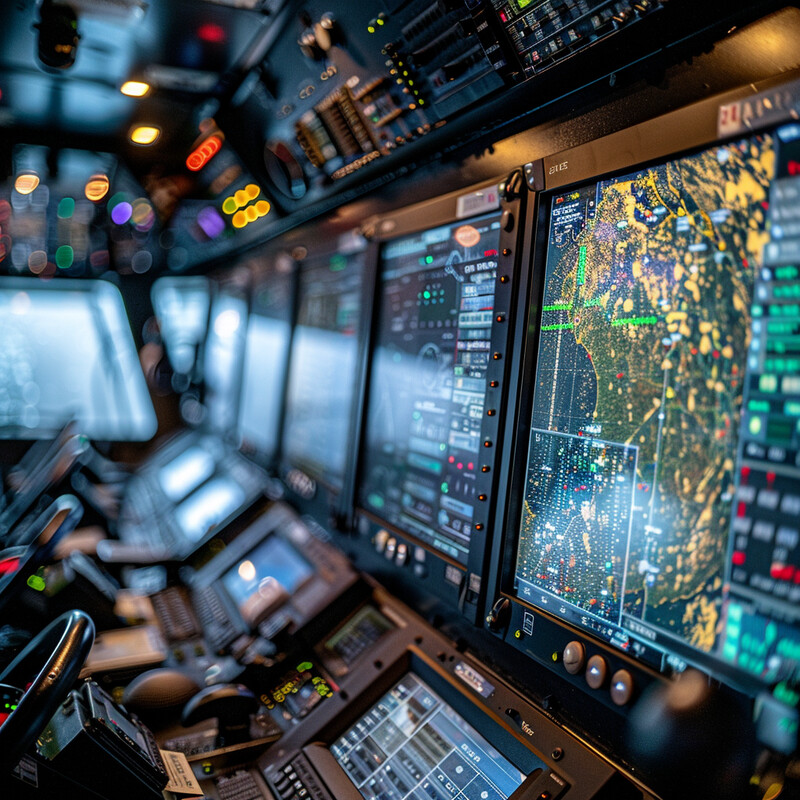
AI automates the routine tasks of collecting, analyzing, and reporting meteorological data. This frees up meteorologists to concentrate on more complex analysis and improves the efficiency and accuracy of weather reporting, ensuring that human expertise is used where it is most needed.