1. Real-time Contaminant Detection
Artificial Intelligence enables water systems to detect contaminants in real time, providing much faster alerts than traditional lab tests. Machine learning algorithms can continuously analyze data from sensors (e.g., for chemicals or microbes) and recognize abnormal patterns that indicate pollution or unsafe water. This immediacy helps utilities and regulators respond to contamination events (like chemical spills or pathogen intrusions) before they spread widely. AI-driven detection is also sensitive, catching subtle changes in water chemistry or biology that humans might overlook. By shortening the time between contamination and detection, AI-enhanced monitoring protects consumers and ecosystems through prompt intervention.
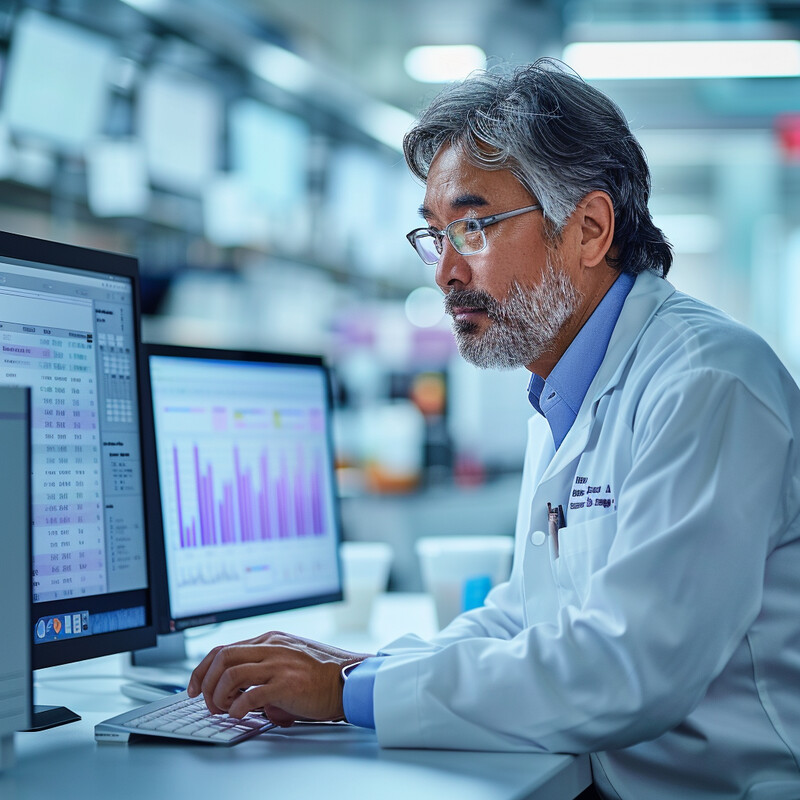
Recent research demonstrates the power of AI sensors for rapid contamination detection. For example, a 2024 study at King Saud University developed advanced optical sensors coupled with machine learning that can rapidly distinguish clean vs. contaminated water and even detect harmful bacteria like E. coli based on spectral patterns. Such AI-enabled sensors can analyze water quality instantly under UV/IR light and correctly identify contamination status, a task that previously required slower, manual lab assays. These real-time detection systems underscore how AI is improving both the speed and accuracy of water quality alerts in drinking water and wastewater systems.
2. Predictive Maintenance for Water Infrastructure
AI is transforming maintenance of water infrastructure from reactive to proactive. Using machine learning on data from pipelines, pumps, and treatment equipment, utilities can predict failures or leaks before they happen. This means water utilities can fix or replace aging pipes and components on a schedule informed by risk rather than just by age. Such predictive maintenance minimizes catastrophic breaks (which cause water loss and service outages) and reduces costs by addressing issues early. In practice, AI models factor in pressure readings, flow anomalies, vibration data, and historical break records to identify which assets are most likely to fail soon. The result is a smarter asset management strategy that extends the life of infrastructure and prevents water quality incidents (like contamination from pipe breaks or sewage overflows) through early intervention.
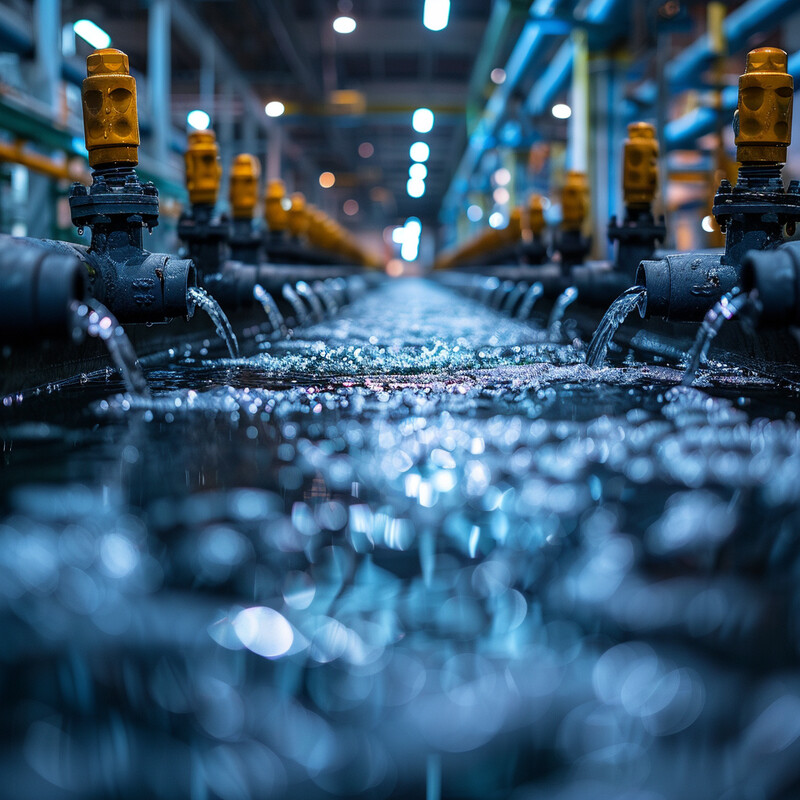
The scope of the U.S. water infrastructure challenge is immense – more than $1 trillion is needed over the next 25 years to upgrade aging drinking water systems. To tackle this, utilities are deploying AI tools to prioritize repairs. In 2024, for instance, a new AI-based solution used diverse data (utility records, public data, sensor outputs) to identify which pipe segments were most likely to fail and which still had useful life, helping target replacements efficiently. By pinpointing high-risk pipes in advance, such predictive AI maintenance can reduce the estimated 6 billion gallons of treated water lost daily in the U.S. from leaky pipes and avert costly emergency bursts. This data-driven approach keeps water quality higher and service more reliable by preventing infrastructure-related contamination events.
3. Optimization of Treatment Processes
AI is used within water and wastewater treatment plants to optimize their processes for both efficiency and effectiveness. Advanced control systems powered by AI can adjust treatment parameters (like chemical dosing, aeration levels, filtration rates) in real time based on incoming water quality data. This leads to more consistent output water quality and often significant savings in energy and chemical use. For example, in wastewater treatment, aeration systems (major energy consumers) can be dynamically controlled by AI to meet oxygen demand without over-aerating, maintaining pollutant removal while cutting energy waste. Similarly, AI can help drinking water plants dose coagulants or disinfectants precisely as needed, avoiding over-treatment and harmful by-products. Overall, AI-driven optimization keeps treatment processes stable against changing conditions (like spikes in pollutant levels or flow) and ensures compliance with water quality standards at lower cost.
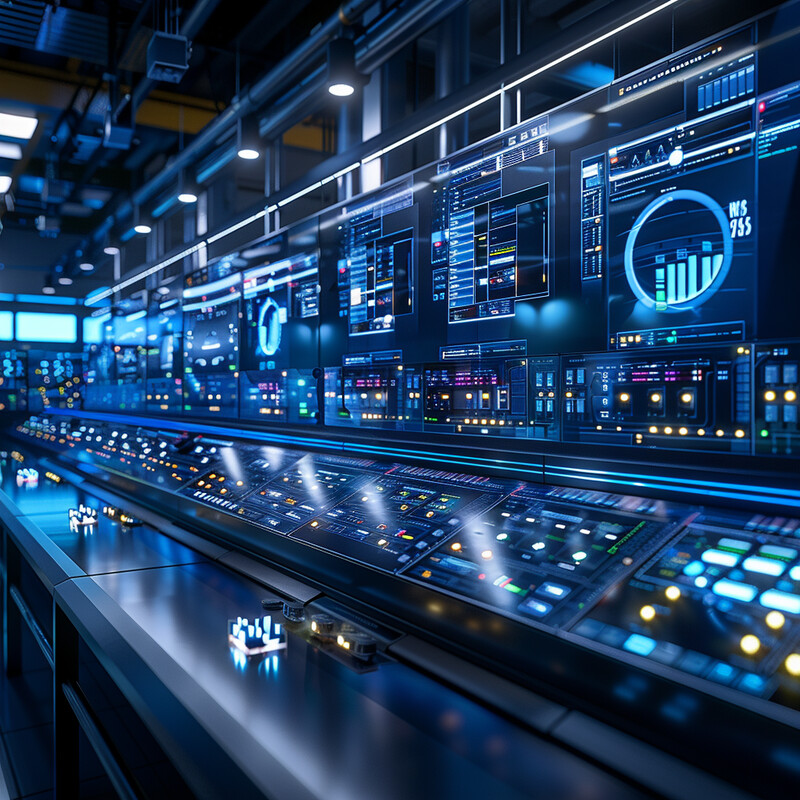
The impact on efficiency can be dramatic. A North America industry study found that using AI to optimize aeration in wastewater facilities can cut energy consumption by 30–50% while actually improving treatment performance. In practice, AI algorithms analyze historical and real-time data (e.g. flow rates, pollutant concentrations, weather) to predict the optimal aeration needed and adjust blowers accordingly. These systems respond to conditions continuously, something human operators or static controls cannot do as finely. The result reported in pilot implementations is a large reduction in electricity use (aeration often accounts for ~60% of a plant’s energy) and very stable effluent quality. This evidence from 2024 underscores how AI optimizes treatment processes, yielding both economic and environmental benefits without compromising water quality.
4. Toxicity Analysis and Prediction
AI is improving our ability to analyze and predict the toxicity of water pollutants, especially complex mixtures of contaminants that are hard to assess with standard tests. Machine learning models can be trained on ecotoxicological data (such as how different chemicals affect aquatic organisms) and then used to predict the toxicity of untested water samples or new chemical compounds. This is valuable for identifying hidden dangers in water: for instance, AI can infer if a combination of runoff chemicals might together be harmful, even if each single pollutant was below regulatory limits. By analyzing patterns in large toxicology datasets, AI models help regulators prioritize which contaminants or mixtures pose the greatest risk to ecosystems and human health. In essence, AI augments traditional toxicity testing by providing rapid, data-driven risk assessments, reducing the need for extensive animal testing and enabling more preventive water quality management.
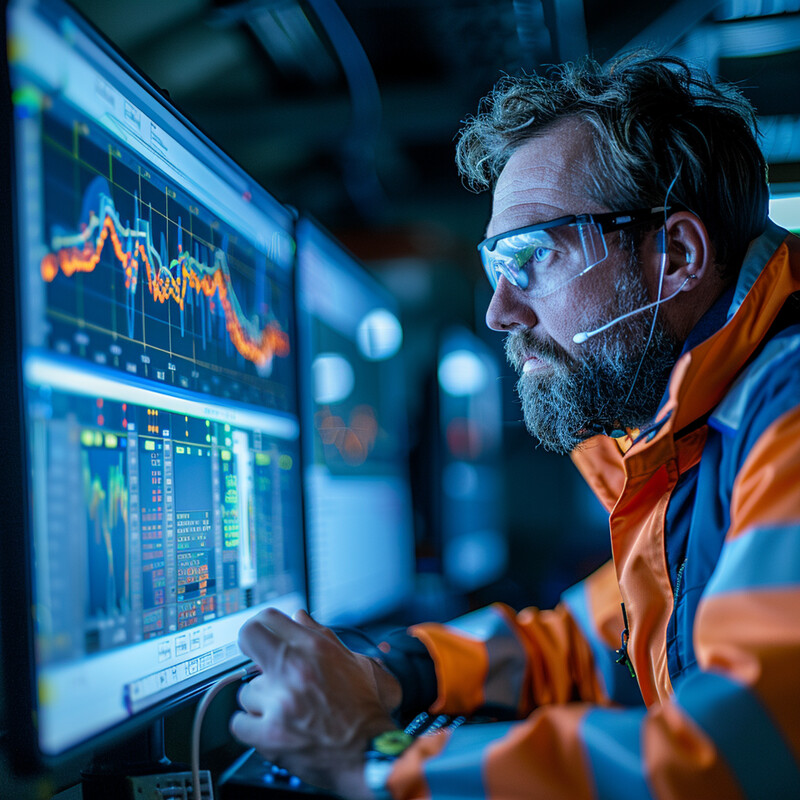
Researchers are already seeing success using AI for toxicity prediction. In late 2024, a University of Birmingham-led team applied AI to find **“hidden hazards” from chemical mixtures in river water that would evade one-at-a-time chemical tests. By monitoring gene expression changes in water fleas (Daphnia) and using machine learning, they could identify which combinations of pollutants were causing toxic effects. This AI-driven approach revealed that certain low-level chemical mixtures worked together to stress aquatic organisms, information that traditional methods missed. Similarly, on the chemical side, Swiss scientists trained AI models on 26,000 data points of fish toxicity and showed the models could predict a chemical’s toxicity to fish within normal biological variance ranges. Such results highlight how AI can accurately predict toxicity outcomes, flag dangerous pollutant synergies, and guide water quality standards to account for complex real-world contaminant mixtures.
5. Trend Analysis for Long-term Water Quality
AI excels at finding patterns and trends in large water quality datasets collected over years or decades. By sifting through historical monitoring data, climate information, land use records, and more, AI can reveal long-term trends – for example, gradual changes in nutrient levels, increasing frequency of algal blooms, or impacts of policy interventions on water quality. This helps scientists and policymakers understand whether water conditions are improving or deteriorating and why. Unlike basic statistical methods, AI (especially techniques like neural networks or time-series models) can capture nonlinear relationships and subtle signals in the data, such as periodic cycles or regime shifts. In practice, this means AI-driven trend analysis can improve forecasts of future water quality under various scenarios (e.g., climate change or urban growth) and inform long-range water resource planning. It essentially turns the wealth of past data into actionable insight about future conditions.
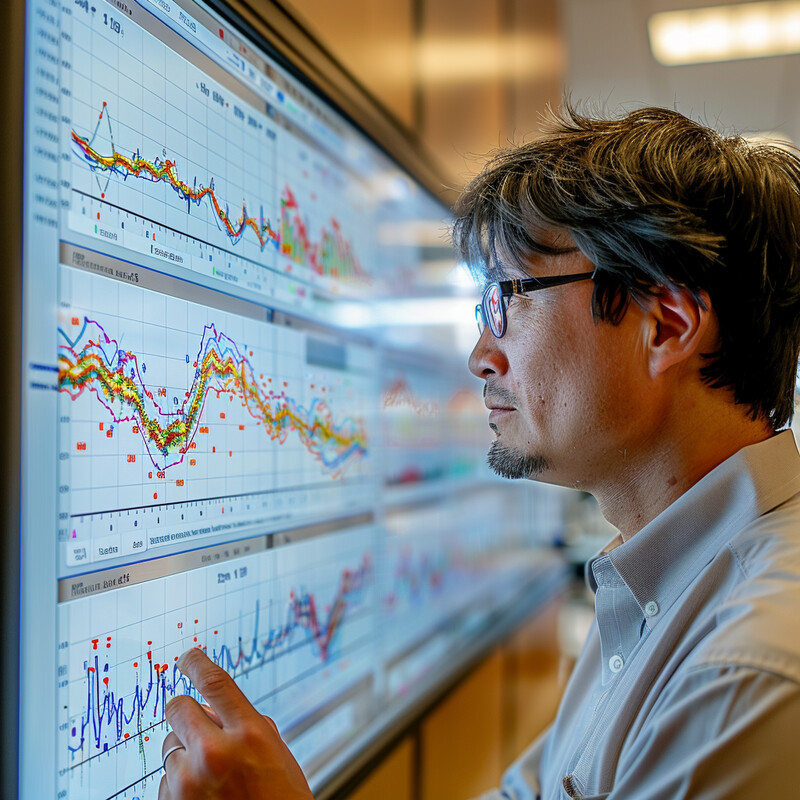
One cutting-edge example is the development of a national-scale water quality forecasting system in the United States. In 2025, University of Vermont researchers announced a tool that integrates the federal National Water Model with real-time sensor feeds using AI to forecast water quality across the country. Tested on New York City’s water supply, the system was able to predict turbidity (water clarity) in reservoirs in advance, giving managers early warning of when storms might cause sediment spikes. This achievement – the first-ever application of NOAA’s National Water Model for water quality – creates “a pathway towards a national capacity to forecast water quality”. It shows how AI-driven analysis of long-term, large-scale data (continental hydrologic models plus years of sensor observations) can spot emerging trends and provide forecasts with enough lead time for preventive action, a significant upgrade to long-term water quality management in the U.S.
6. Automated Sampling and Analysis
AI is enabling automated systems – like robots, drones, and smart sensors – to handle the collection and analysis of water samples with minimal human intervention. This advancement means water quality can be monitored in dangerous or hard-to-reach locations (such as flood zones, industrial outfalls, or remote rivers) safely and frequently. Autonomous boats and aerial drones equipped with AI can navigate to sampling sites, collect water, and even do on-board analysis or preservation for lab tests. By programming these devices with AI algorithms, they can decide when and where to sample (for instance, triggering extra samples when an anomaly is detected). Automated analysis can include on-site testing for certain parameters or rapid DNA tests for pathogens, with AI interpreting the results instantly. The overall effect is a more continuous and expansive monitoring network that can catch pollution events or changes without waiting for a person to take a sample. It also standardizes sampling procedures, improving data quality by reducing human error.
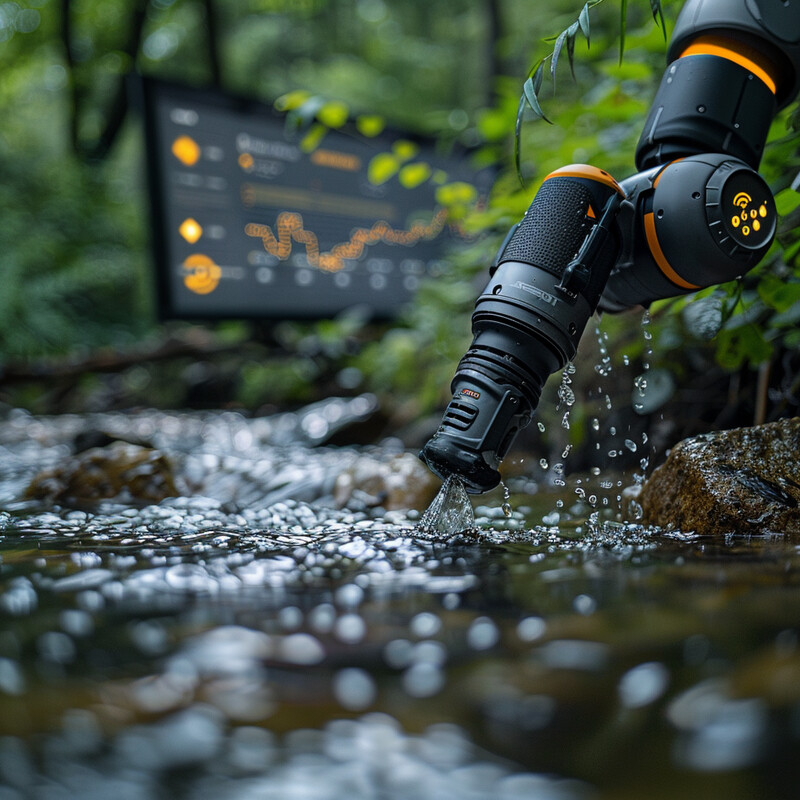
The U.S. Geological Survey has demonstrated the benefits of robotic water sampling for public health monitoring. Their 2024 field trials with a “robotic environmental DNA (eDNA) sampler” found it captured and processed water samples as reliably as human technicians, while operating autonomously at high frequency. These AI-guided samplers can be left in streams to collect samples hourly or daily – far more often than is practical with manual methods – and can work in hazardous conditions (e.g., floodwaters or sites with toxic contamination) that would endanger people. In Hong Kong, five autonomous monitoring boats have been used since 2017 to gather reservoir water quality data; the fleet can survey the entire 12 km² reservoir in one day instead of “a few days” required by traditional vessels, greatly improving monitoring efficiency. These examples show how AI and automation greatly expand our sampling reach and frequency, leading to earlier detection of issues and more robust water quality datasets.
7. Data Integration from Multiple Sources
AI facilitates the integration of water quality data from many disparate sources into a cohesive analytical picture. Water quality information comes in various forms – sensor readings (for temperature, pH, etc.), laboratory test results, satellite imagery, rainfall and flow data, reports from field inspectors, and more – often stored in incompatible formats. Machine learning and data fusion techniques can merge these streams and harmonize them, allowing analysis of all relevant factors together. This multi-source integration is crucial for understanding complex water issues; for example, linking upstream land use and weather patterns with downstream water quality requires combining remote sensing, weather data, and in-stream measurements. AI tools can handle the volume and variety of such datasets, cleaning and standardizing them (sometimes using common frameworks like WaterML for sensor data). By breaking down data silos, AI gives water managers a 360-degree view – one can simultaneously see, for instance, how a spike in turbidity correlates with satellite-detected soil erosion and a change in river flow. Ultimately, this leads to more accurate analyses and models, since integrated data provides context that single-source data lacks.
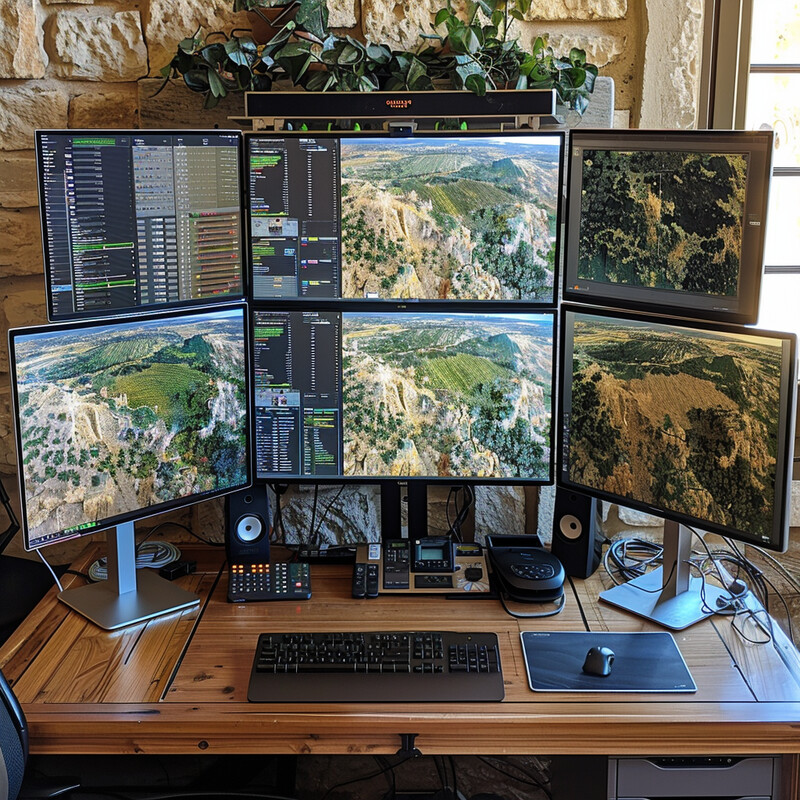
A prominent U.S. initiative underscored the power of integrating diverse data for water forecasts. In 2025, scientists combined a continental hydrologic model with real-time sensor inputs and AI, effectively creating a unified platform to predict water quality events. This system leverages multiple data sources – the National Water Model’s simulated flows, weather data, and NYC’s extensive network of water sensors – fused via AI to forecast issues like high turbidity. The success of this approach in New York’s Ashokan Reservoir (which supplies millions of people) shows how integrating model data with on-the-ground observations can improve reliability of water quality predictions. More generally, water agencies are adopting standards (e.g., Sensor Observation Service protocols) and AI-driven data pipelines so that groundwater well data, river gauges, satellite images, and treatment plant outputs can all be analyzed together. The result is more meaningful analysis and early detection of water quality trends that only emerge when datasets are combined.
8. Effluent Quality Management
AI is helping wastewater treatment plants and industrial facilities better manage the quality of effluent (discharged water) to ensure it meets environmental standards. Through advanced monitoring and control, AI can adjust treatment operations in real time to keep pollutants in the effluent within permitted limits. Key discharge parameters like nutrient concentrations, pH, turbidity, or chemical residues can be continuously predicted by AI models, which then fine-tune the treatment process (e.g., aeration intensity, chemical dosing or membrane filtration rates) to prevent deviations. This predictive control reduces the occurrence of permit violations and environmental harm to receiving waters. Additionally, AI can prioritize responses to abnormal situations – for example, if a sudden load of pollutants is detected entering a wastewater plant, the AI system can temporarily ramp up certain treatment stages or divert flow as needed. By stabilizing effluent quality and reacting swiftly to changes, AI not only helps compliance but can also optimize resource use (not overtreating when not needed, thus saving energy). Overall, effluent management becomes more reliable and efficient with AI oversight, resulting in cleaner water released to rivers, lakes, or oceans.
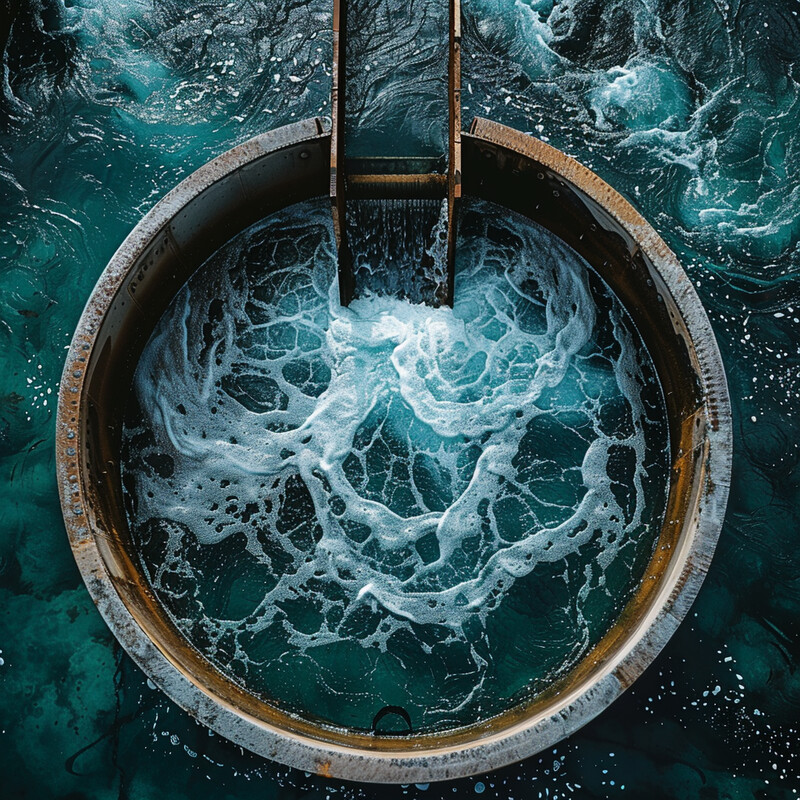
Studies show that AI-based control strategies can significantly improve effluent consistency. Researchers in 2024 developed a hybrid AI model for wastewater treatment that accurately predicted effluent quality and enabled more precise control of the process – leading to better compliance with standards. By processing real-time sensor data, the model could anticipate the levels of key pollutants in the outgoing water and adjust operations proactively. Water utilities are already moving in this direction: Suez, a major utility, reports using AI to turn “millions of data [points] into concrete action,” with systems that classify the severity of incoming pollution events and recommend real-time adjustments in treatment (like tweaking aeration or dosing) to stabilize the effluent. These AI-guided interventions ensure that even when incoming wastewater quality fluctuates, the plant’s discharge remains within safe limits. Early results include reduced chemical usage and more consistent removal of nutrients and contaminants, demonstrating AI’s value in protecting downstream water quality.
9. Remote Sensing and Aerial Surveillance
AI greatly enhances water quality monitoring via remote sensing – that is, using aerial or satellite platforms to observe water bodies. Traditionally, interpreting images for features like algal blooms, sediment plumes, or pollution slicks required expert analysis, but now computer vision algorithms (often deep learning) can automatically detect these phenomena in real-time imagery. Drones and aircraft equipped with cameras or hyperspectral sensors, guided by AI, can patrol watersheds and identify problems such as illegal discharges, algae outbreaks, or sewage leaks from the air. Satellite data (e.g., from Landsat, Sentinel, or NOAA satellites) combined with AI allows large-scale monitoring of water color and clarity, indicating water quality over vast areas (lakes, coastlines) without physical sampling. This aerial surveillance means earlier discovery of issues – for example, spotting a developing harmful algal bloom in a reservoir days before toxins reach dangerous levels, or detecting a chemical spill spreading in a river. It also covers inaccessible terrain (dense wetlands or conflict zones) where ground monitoring is sparse. By automating image analysis, AI ensures that these remote observations are continuous and objective, providing a powerful complement to in-situ monitoring.
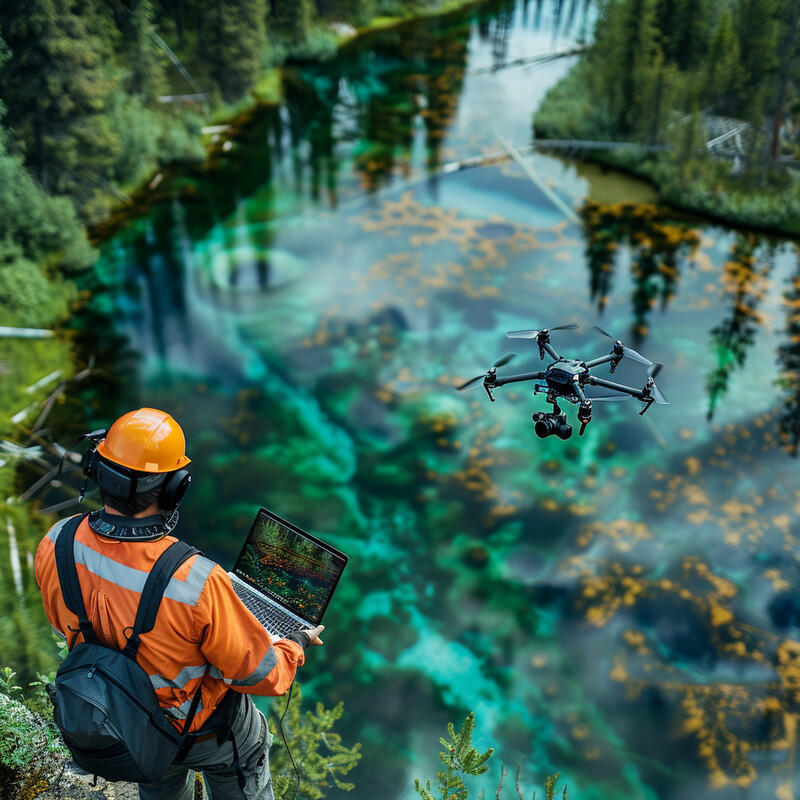
Cutting-edge projects have shown how effective AI-powered aerial monitoring can be. In 2024, a team at University of Iowa unveiled a vision-based AI system using cameras and drones to perform real-time water pollution surveillance, without human operators. The system’s deep learning model (YOLOv5) was trained to recognize telltale visual signs of water contamination – from algal blooms and synthetic foam to oil spills and floating trash – and it could detect these across local water bodies instantly. Uniquely, they also integrated a language model to generate context (explaining the type and likely cause of the pollution) for authorities. This AI surveillance setup can alert water managers the moment, say, an algal bloom appears in a lake, enabling rapid public advisories. Similarly, NASA’s open-source CyAN/CyFi project in 2024 applied machine learning to high-resolution satellite images to pinpoint high-risk algae concentrations in smaller lakes that previous low-res imagery would miss. These examples from the U.S. and abroad illustrate AI’s growing role in remote sensing for water quality – catching problems early over large areas and guiding interventions (like targeted cleanups or treatment adjustments) with advanced notice.
10. Public Health Analytics
AI is increasingly used to draw connections between water quality data and public health, helping predict and prevent water-related health crises. By analyzing patterns in drinking water quality, wastewater, and disease outbreak data, AI can serve as an early warning system for health risks. For example, sudden changes in sewage microbiology detected by AI might signal a community outbreak of a gastrointestinal illness even before patients visit clinics. Likewise, AI can correlate years of water contamination records (like lead or nitrates) with health outcomes in a population to identify communities at risk of chronic exposure. This approach enables public health officials to respond proactively – issuing boil-water advisories, mobilizing vaccination or medical resources, or remediating contamination sources – rather than reacting after illnesses occur. In essence, AI-driven public health analytics turns environmental monitoring into actionable health intelligence, ensuring that trends like emerging pathogens, toxic algal blooms, or chemical spills are flagged in time to protect the public. This fusion of environmental science and epidemiology through AI improves our ability to safeguard drinking water supplies and prevent waterborne disease.
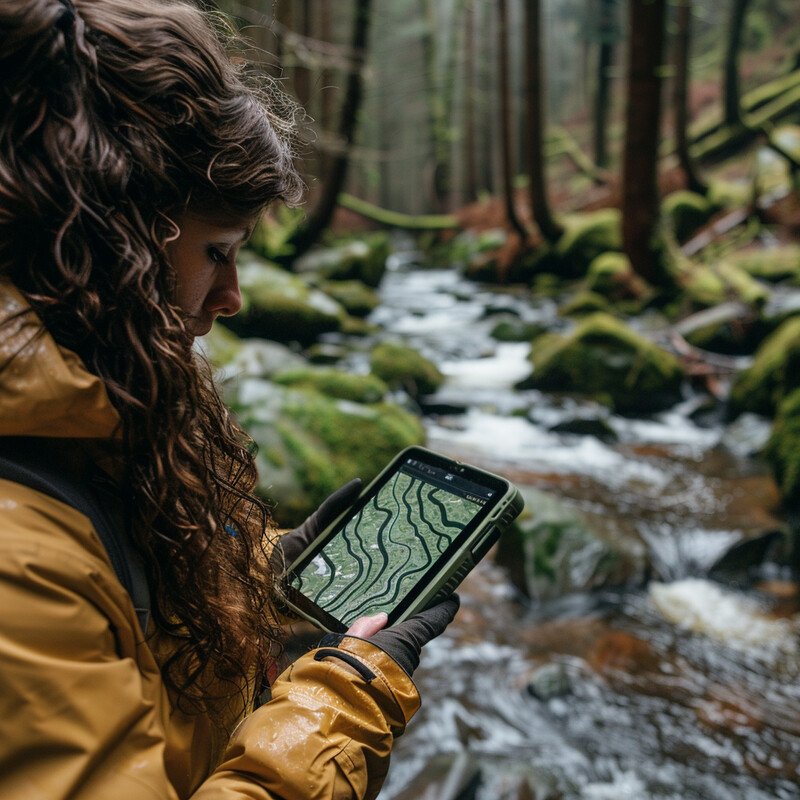
The impact of merging water and health data is evident in modern surveillance initiatives. A striking example is the use of wastewater analysis to predict disease outbreaks: a 2024 study in China developed an AI model that estimated human monkeypox (mpox) cases from sewage virus levels with remarkable precision (capturing ~87% of the variance in actual case numbers). By robotically sampling wastewater and quantifying viral DNA, then using AI, the system provided an early indicator of mpox spread – effectively forecasting case surges ahead of clinical reporting. In the U.S., recognition of wastewater’s value for public health led the CDC in 2024 to expand its National Wastewater Surveillance System (NWSS) to track not just COVID-19 but also influenza A, RSV, and even avian flu as part of routine monitoring. These data streams are increasingly analyzed with machine learning to spot infection spikes. Public health departments have noted that wastewater-based detection often outpaces traditional case reporting, giving a critical head start in responding to threats. By intelligently mining water quality data, whether chemical or biological, AI is helping predict dangers like disease outbreaks or drinking water contamination, thereby supporting interventions that protect community health.