1. Automated Waste Sorting
AI-powered robots and machines can accurately sort waste into recyclables, organics, and non-recyclables, improving recycling rates and reducing contamination in recycling streams.
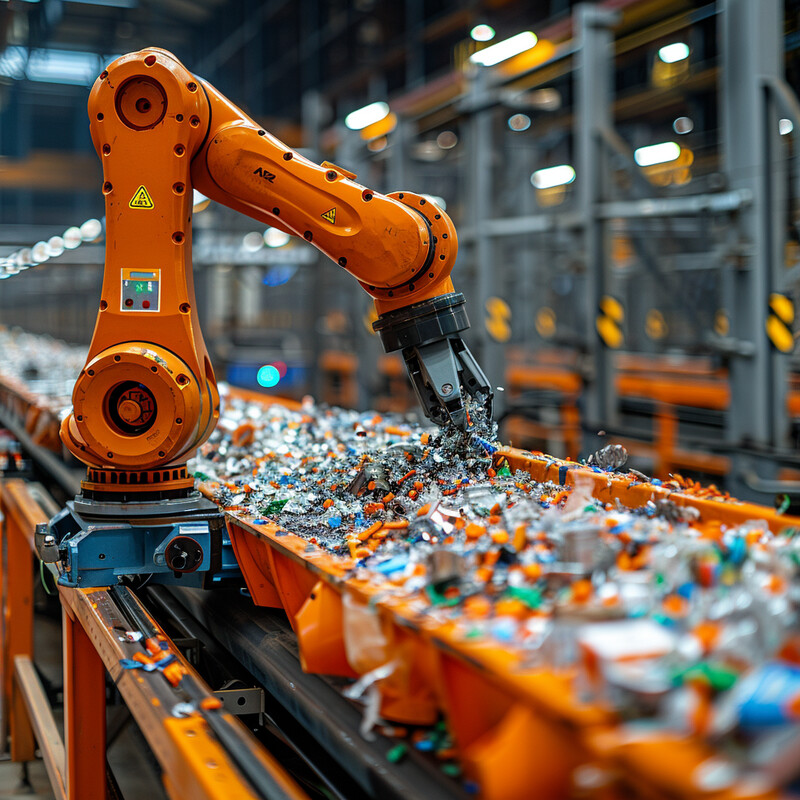
AI-powered robots and sophisticated sorting systems use machine vision and learning algorithms to identify and separate different types of waste materials. These systems can distinguish between various recyclables like plastics, metals, and paper with high accuracy. By automating the sorting process, AI reduces human error, increases the efficiency of recycling operations, and minimizes contamination in the recycling stream, leading to better quality recyclables.
2. Optimized Collection Routes
AI analyzes data from collection trucks and waste volumes to optimize collection routes, reducing fuel consumption and operational costs.
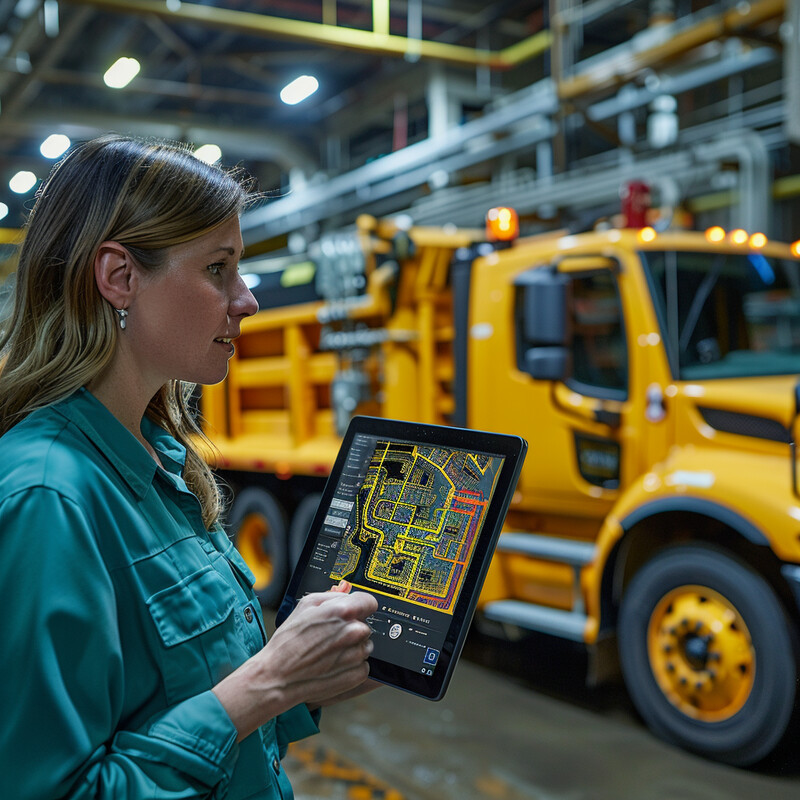
AI analyzes data from garbage collection routes, vehicle locations, traffic patterns, and bin fullness sensors to optimize the routes for waste collection trucks. This optimization not only reduces fuel consumption and emissions but also ensures that collections are timely and efficient, minimizing operational costs and environmental impact.
3. Predictive Maintenance for Equipment
AI uses data from sensors on waste management equipment to predict when maintenance is needed, preventing unexpected breakdowns and extending the life of the equipment.
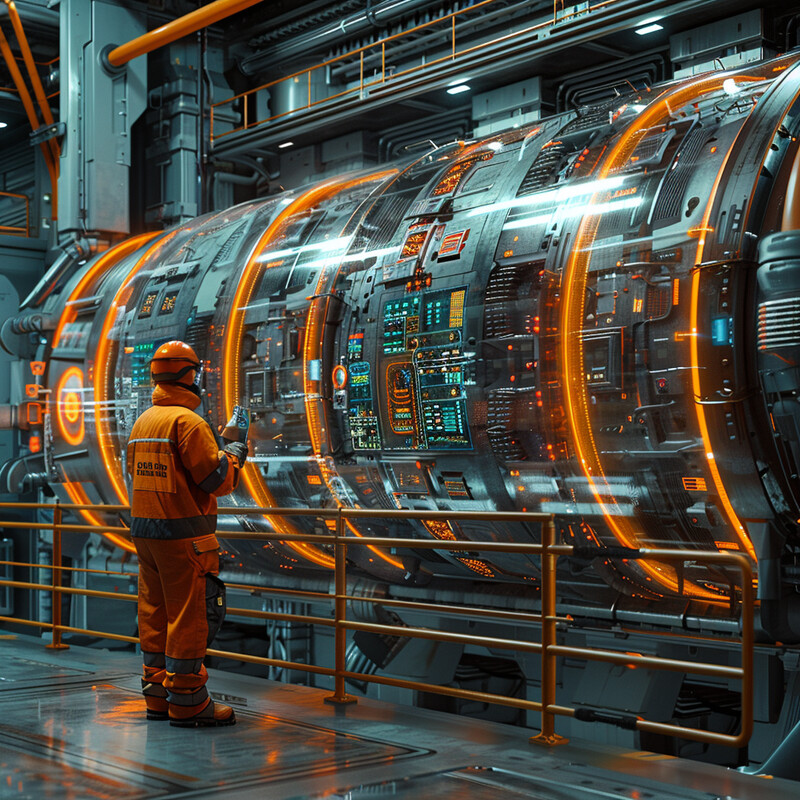
AI uses sensor data from waste management equipment to monitor their condition continuously. By applying predictive analytics, AI can forecast when and which components may need maintenance, scheduling interventions before failures occur. This proactive approach reduces downtime and extends the lifespan of equipment, enhancing overall operational reliability.
4. Dynamic Scheduling Systems
AI systems adjust waste collection schedules based on real-time data like bin fullness and historical waste generation patterns, enhancing service efficiency.
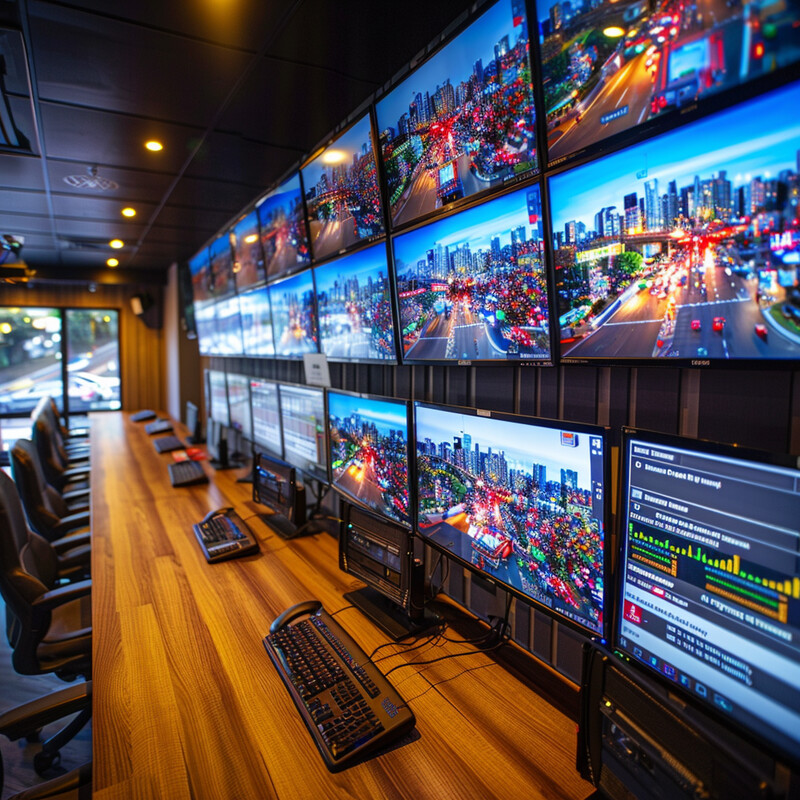
AI-driven dynamic scheduling systems adjust waste collection schedules based on real-time data such as bin fullness, historical waste generation patterns, and events that might increase waste production. This flexibility ensures that waste collection services are more responsive to actual needs, reducing unnecessary collections and optimizing resource use.
5. Enhanced Recycling Processes
AI enhances the capabilities of recycling facilities by identifying and categorizing different materials more accurately, leading to more efficient recycling and higher quality recycled products.
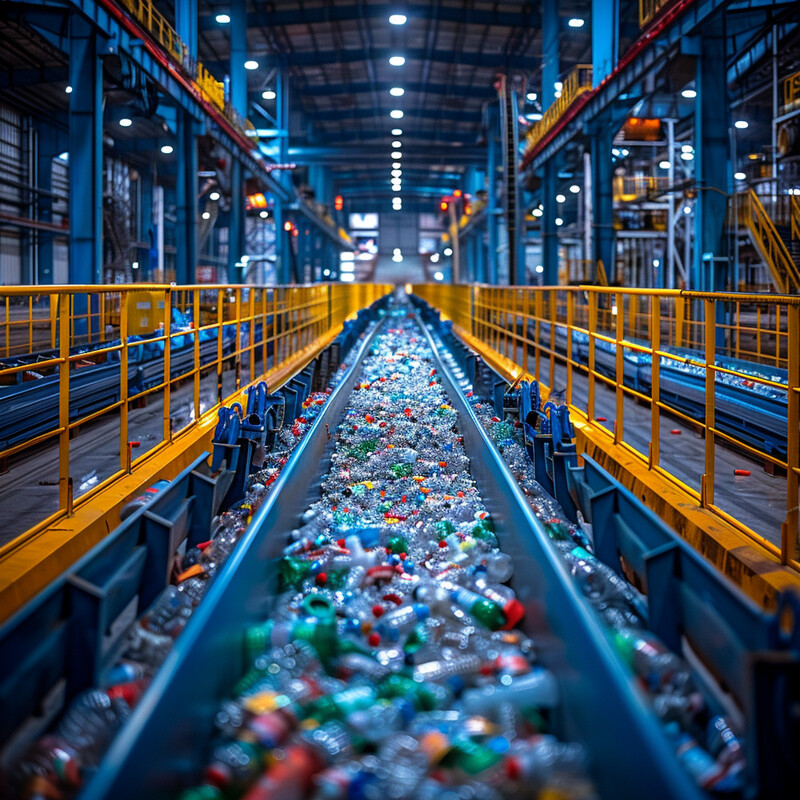
AI technologies improve recycling processes by more accurately identifying and categorizing materials through advanced sensing and imaging technologies. This precision allows for better separation of materials, improving the quality of recycled outputs and enabling more materials to be reused in new products.
6. Waste Volume Prediction
AI predicts waste generation trends based on factors such as seasonal changes, population growth, and consumption patterns, helping cities prepare for future waste management needs.
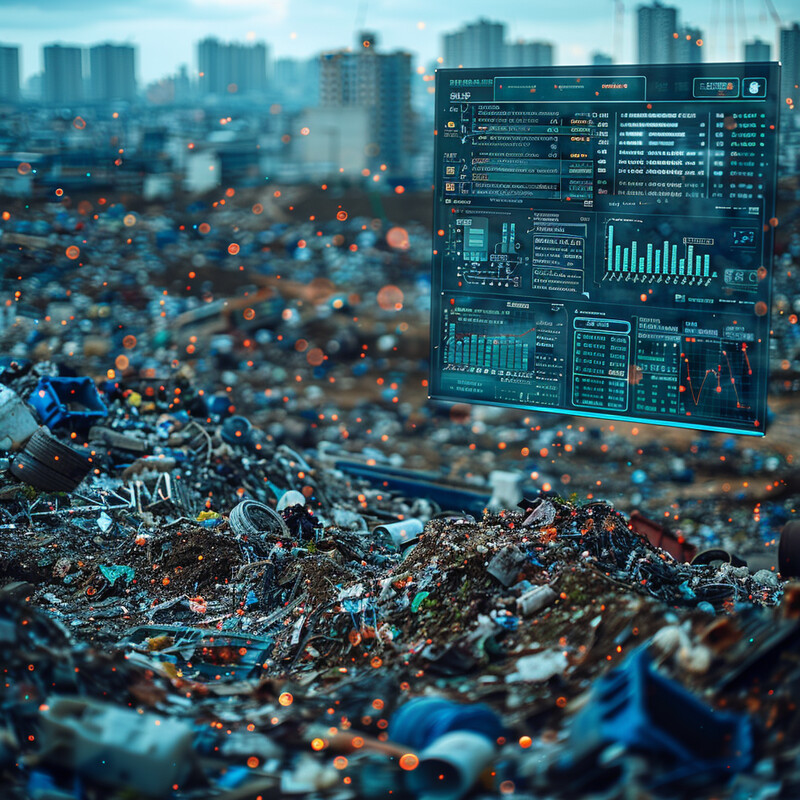
AI models predict future waste generation by analyzing trends from demographic data, consumption patterns, seasonal variations, and economic activity. Accurate predictions help waste management systems prepare adequately for future demands, optimizing resource allocation and infrastructure development to handle expected waste volumes.
7. Waste-to-Energy Optimization
AI optimizes waste-to-energy conversion processes by analyzing the energy content of waste streams and adjusting operational parameters for maximum efficiency.
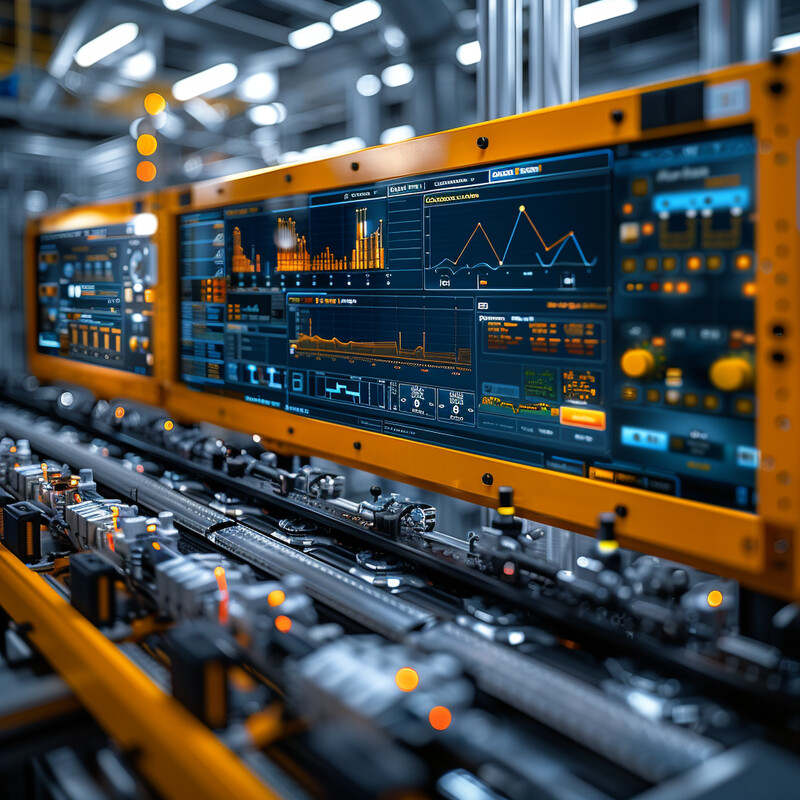
AI optimizes waste-to-energy plants by analyzing the calorific value of incoming waste streams and adjusting combustion parameters for maximum efficiency. This optimization ensures that the energy recovery from waste is maximized, contributing to sustainable energy generation and reducing landfill use.
8. Real-Time Monitoring Systems
AI provides real-time monitoring of waste management operations, offering insights into system performance and areas for improvement.
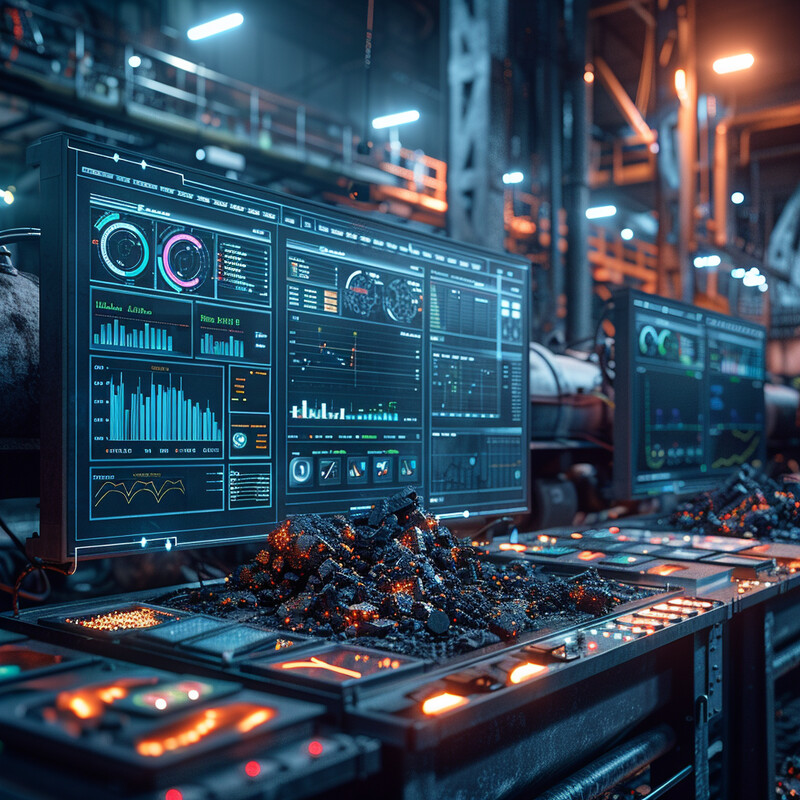
AI-enabled real-time monitoring systems provide continuous oversight of waste management operations. These systems track and analyze data across the waste management chain, from collection to processing, offering actionable insights that can lead to operational improvements and cost savings.
9. Public Engagement and Education
AI-driven platforms analyze public engagement data to tailor educational campaigns on recycling and waste reduction, increasing public awareness and participation.
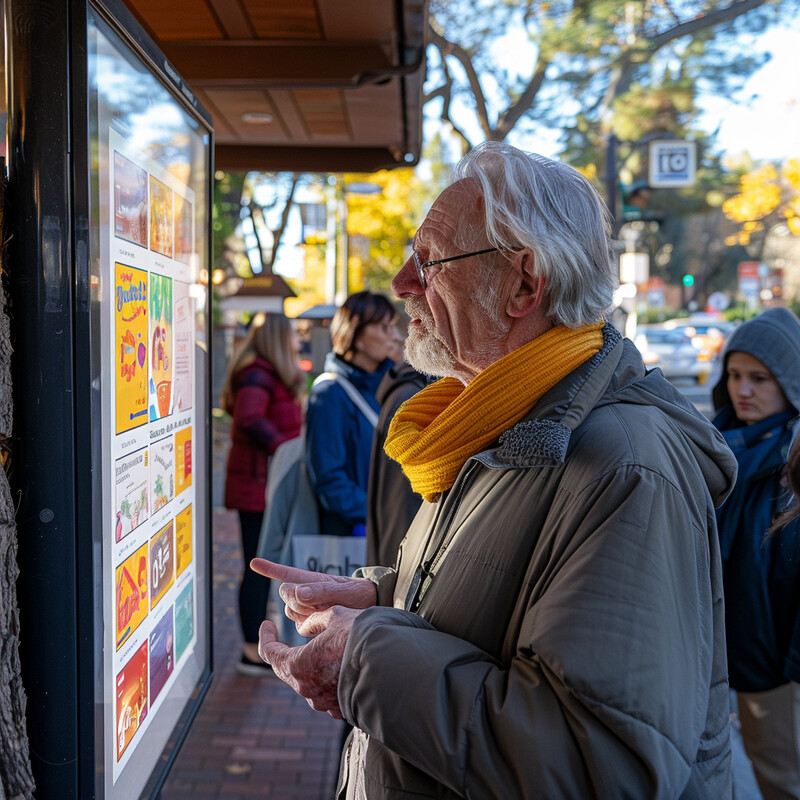
AI analyzes engagement and effectiveness of public education campaigns on waste management. By understanding which messages resonate with the community, AI can help tailor campaigns that effectively promote recycling and waste reduction, increasing public participation and compliance with waste segregation guidelines.
10. Regulatory Compliance Monitoring
AI monitors compliance with waste management regulations by tracking waste streams and ensuring that processes adhere to legal standards.
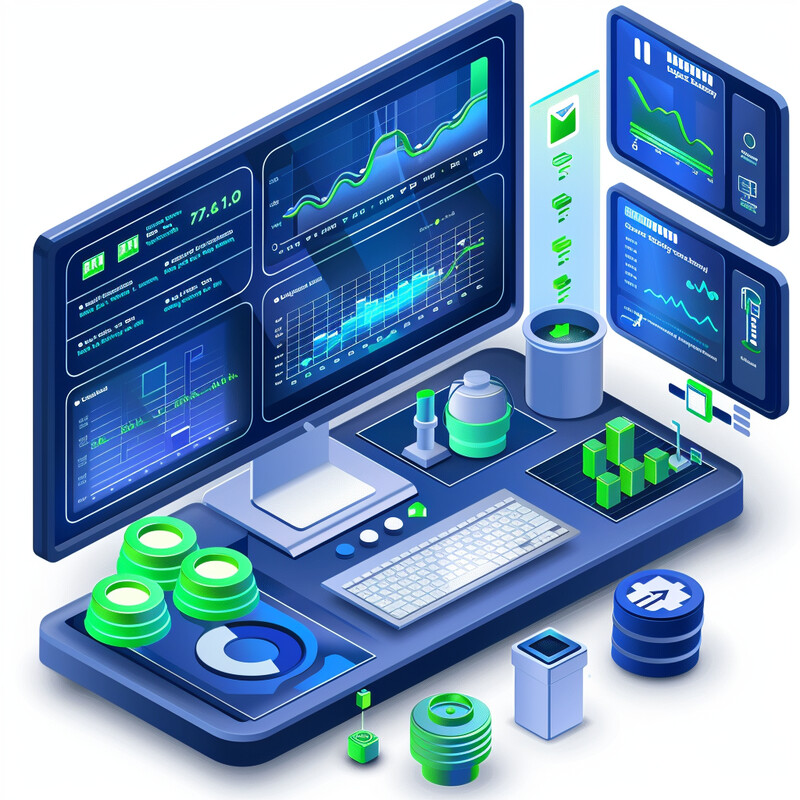
AI ensures that waste management practices adhere to regulatory standards by monitoring waste streams and processing operations. This monitoring helps identify deviations from compliance, allowing waste management providers to address issues promptly and maintain legal and environmental standards.