1. Predictive Analytics for Population Growth
AI models predict population growth and demographic changes, helping urban planners anticipate future demands for housing, schools, and infrastructure.
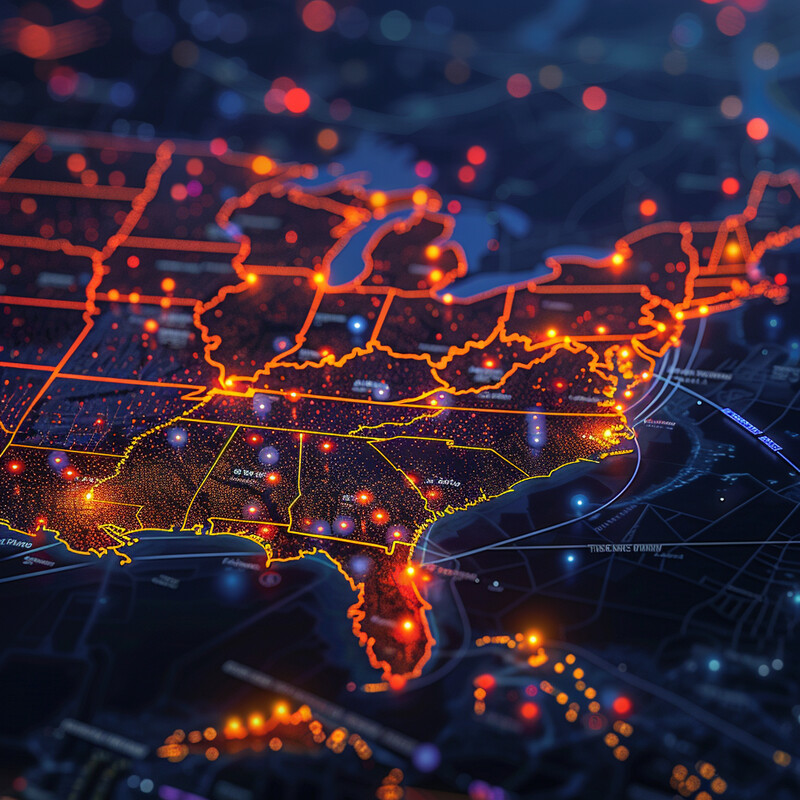
AI leverages demographic data, migration trends, and economic indicators to build predictive models that forecast population growth and shifts. Urban planners use these insights to plan for future housing, infrastructure, and services needs, ensuring cities can accommodate growth sustainably and efficiently.
2. Traffic Flow Optimization
AI analyzes traffic data to optimize road layouts, signal timings, and public transit routes, reducing congestion and improving city mobility.
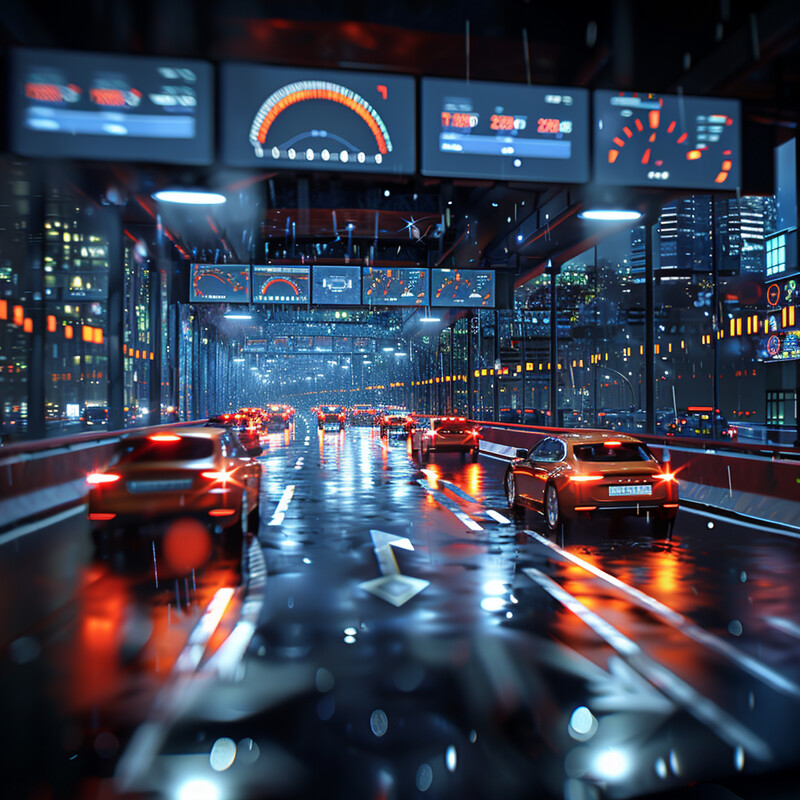
By analyzing traffic patterns, congestion points, and commuter behaviors, AI helps in designing more efficient road networks and improving traffic signal systems. This not only reduces traffic congestion but also enhances public transportation routes and schedules, leading to smoother mobility and decreased travel times.
3. Resource Allocation Efficiency
AI optimizes the allocation of limited urban resources such as water, energy, and emergency services based on real-time data and predictive models.
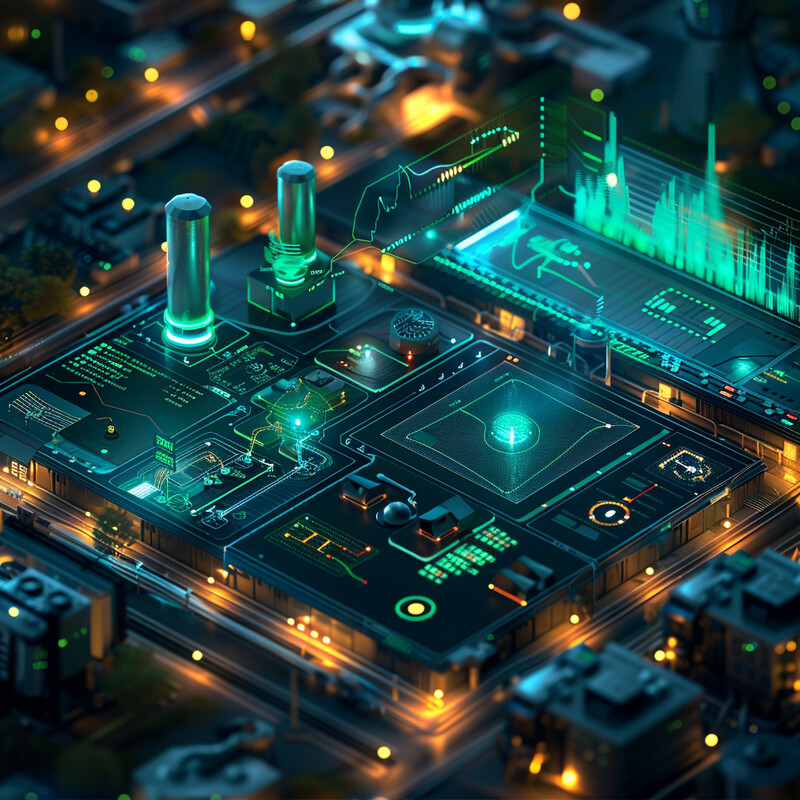
AI optimizes the distribution of urban resources like water, energy, and emergency services by analyzing usage patterns and predicting future demands. This ensures optimal resource allocation, reducing waste and ensuring that critical services are adequately provisioned across the city.
4. Environmental Impact Assessments
AI automates the assessment of potential environmental impacts for new urban projects, incorporating vast amounts of ecological data to ensure sustainable development.
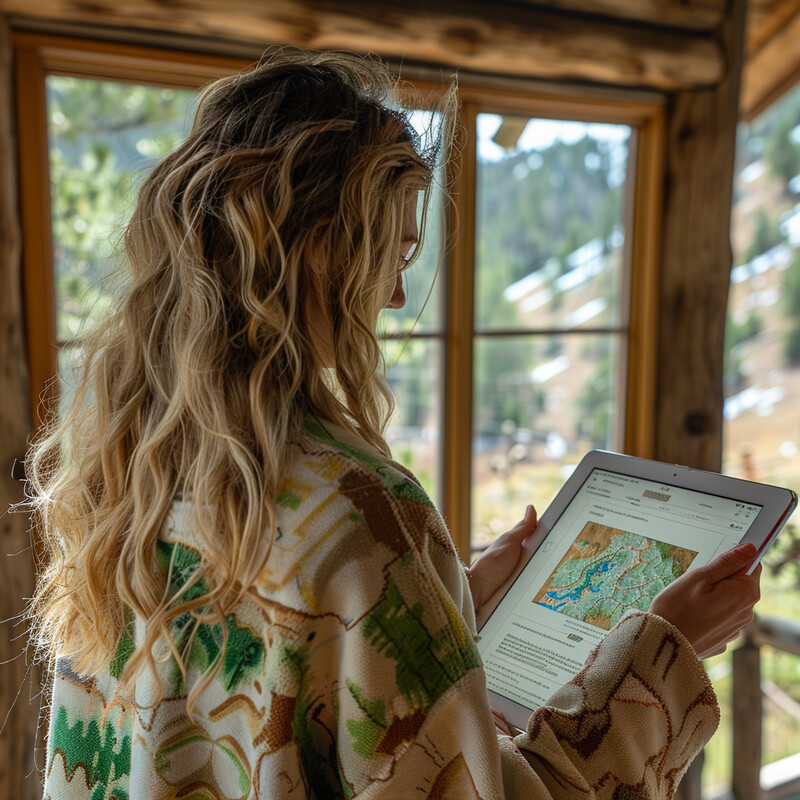
AI streamlines the process of environmental impact assessment for new urban developments by automatically analyzing large datasets related to air quality, water sources, wildlife populations, and more. This allows for faster and more accurate evaluations, helping to maintain sustainable development practices.
5. Public Safety and Security
AI enhances public safety by analyzing crime patterns and predicting potential hotspots, allowing for better placement of public safety resources and preventive measures.
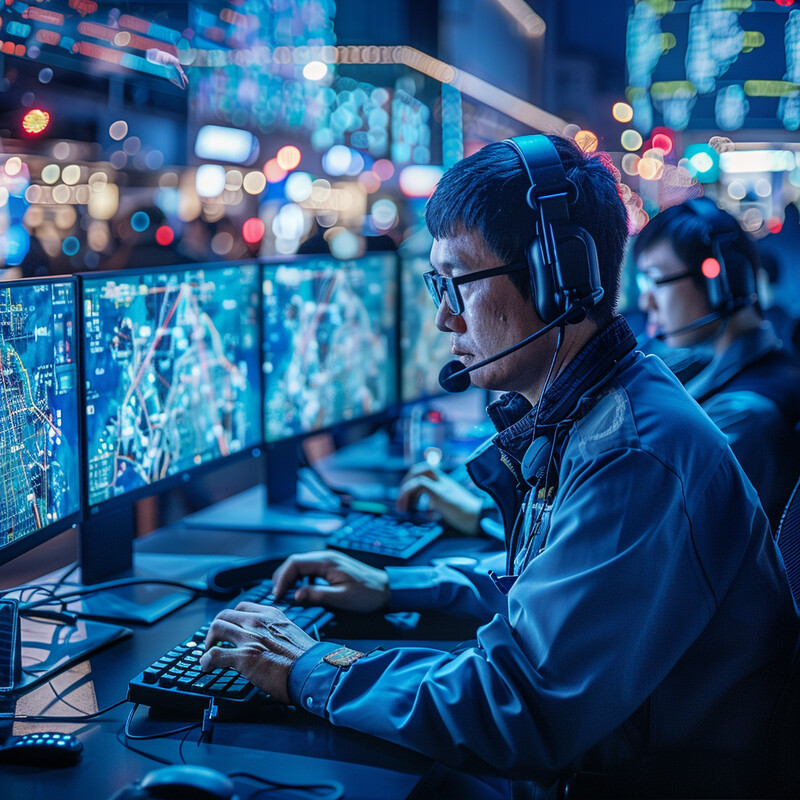
Utilizing crime statistics and predictive modeling, AI identifies potential crime hotspots and helps in strategizing preventive measures. This technology enables law enforcement to allocate resources more effectively, increasing public safety through targeted patrols and community engagement initiatives.
6. Economic Development Analysis
AI tools evaluate economic trends and simulate the impacts of various development projects, assisting in the planning of economic zones, commercial areas, and job creation initiatives.
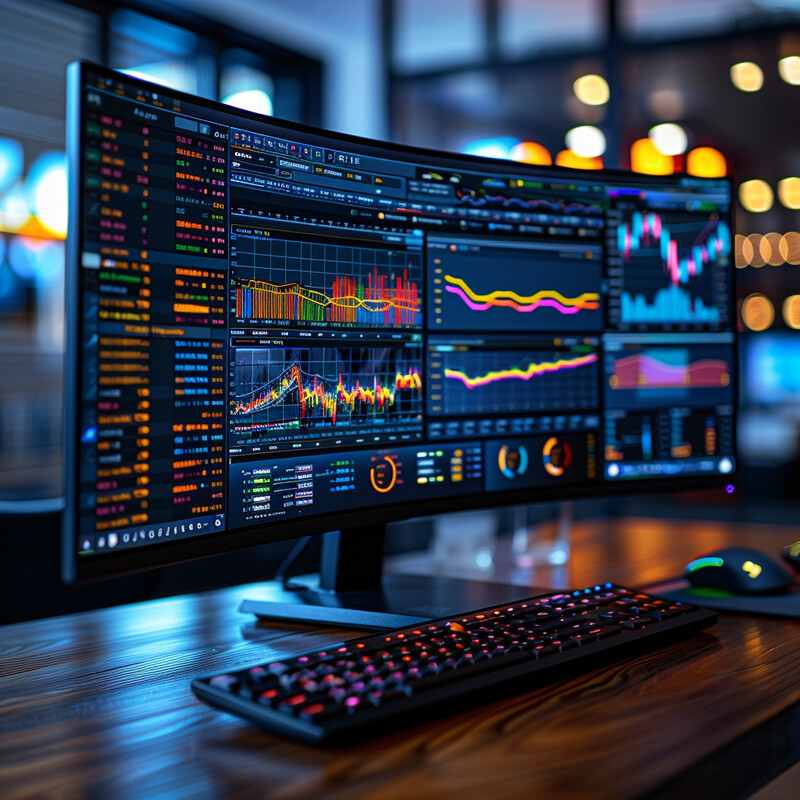
AI analyzes economic data to forecast the impacts of different urban development projects. This assists planners in deciding where to locate new commercial zones, industrial areas, and recreational facilities to stimulate economic growth and job creation effectively.
7. Smart Building and Zoning
AI helps in designing smart buildings and optimizing zoning regulations by analyzing data on land use, population density, and community needs, leading to more efficient urban layouts.
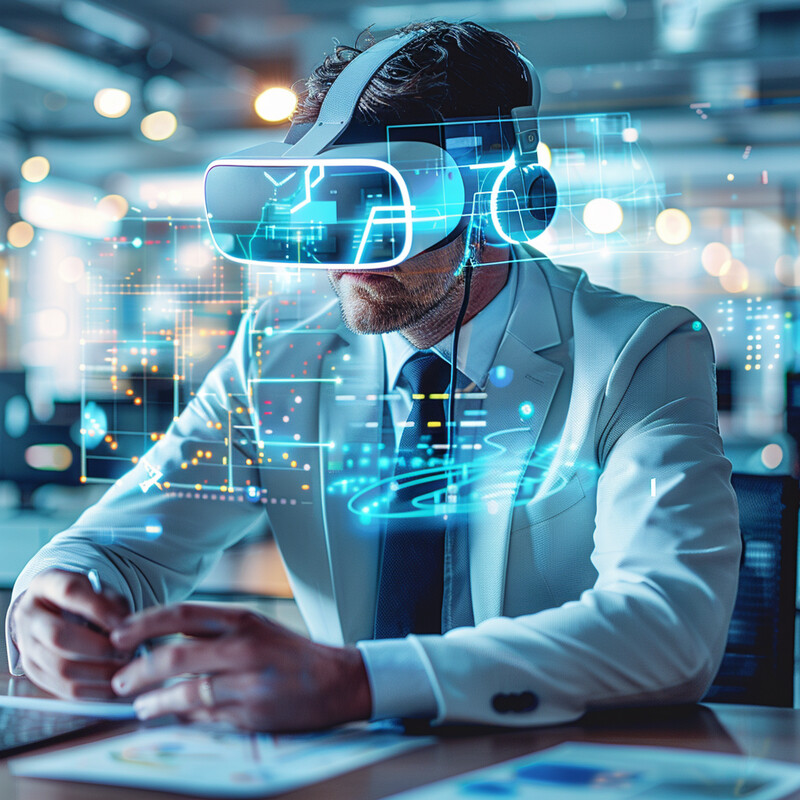
AI-driven tools analyze urban data such as land use, current infrastructure, and regulations to suggest optimal placements for new buildings and adjustments in zoning laws. This leads to smarter urban growth, enhanced property values, and better quality of life for residents.
8. Disaster Management and Response
AI models simulate disaster scenarios (like floods, earthquakes) to plan response strategies and infrastructure resilience, improving preparedness and reducing potential damage.
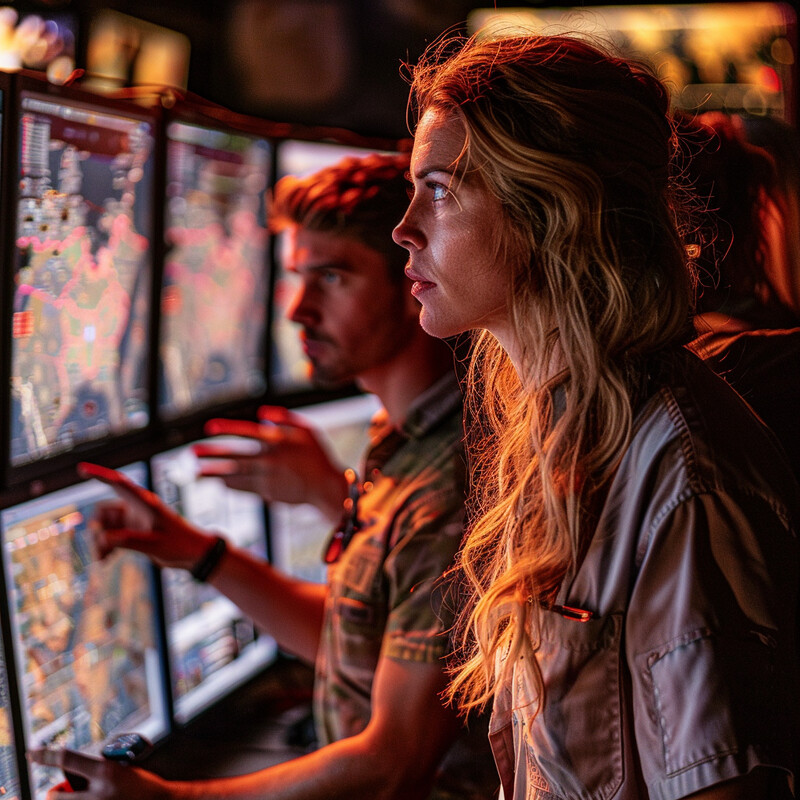
AI simulates various disaster scenarios to help urban planners design infrastructure that can withstand potential calamities like floods, earthquakes, or hurricanes. These models aid in developing effective emergency response strategies, significantly reducing the impact of disasters on urban populations.
9. Public Engagement and Feedback Analysis
AI analyzes feedback from the community gathered through social media, surveys, and public forums, providing insights into public opinions and needs, which can guide planning decisions.
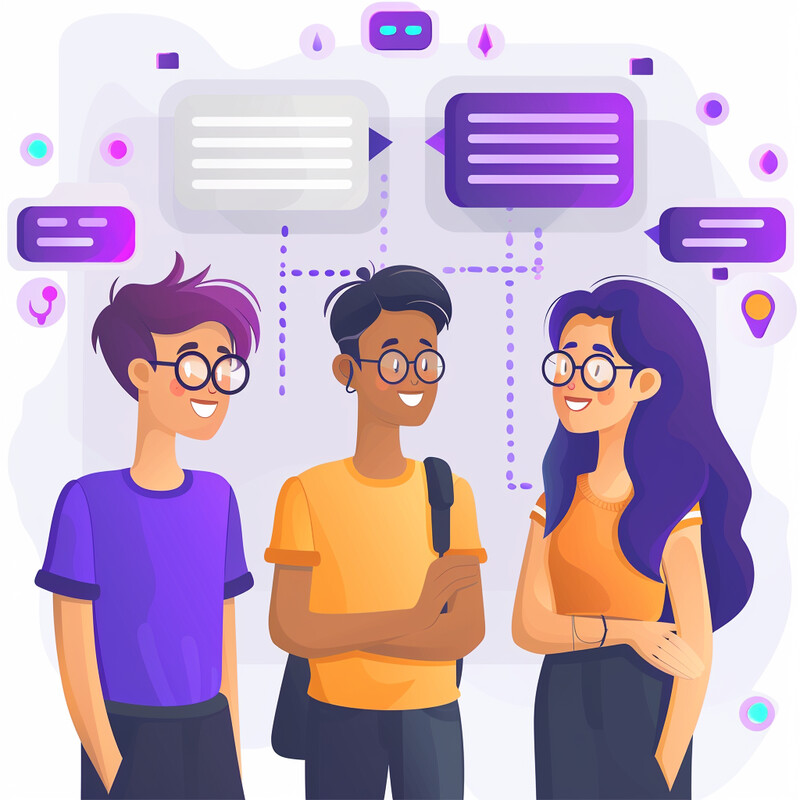
AI processes large volumes of public feedback from social media, surveys, and forums to gauge community needs and sentiments. This allows urban planners to align projects more closely with the preferences and requirements of the community, ensuring higher satisfaction and better utilization of public spaces.
10. Historical Data Preservation and Analysis
AI tools digitize and analyze historical urban data, allowing planners to consider historical trends and heritage preservation in their future urban strategies.
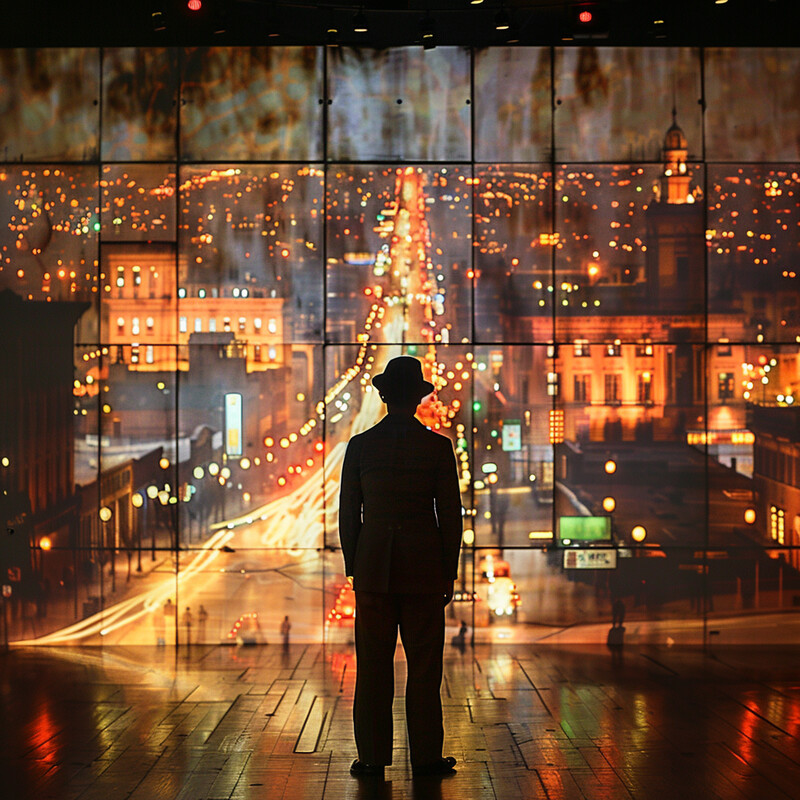
AI tools digitize and analyze historical data on urban development, preserving important cultural and architectural heritage. Planners use this information to ensure that new developments respect historical contexts and contribute positively to the city's historical narrative.