1. Traffic Flow Optimization
AI algorithms analyze real-time traffic data to optimize traffic light timing and reduce congestion, improving the overall flow of traffic across urban networks.
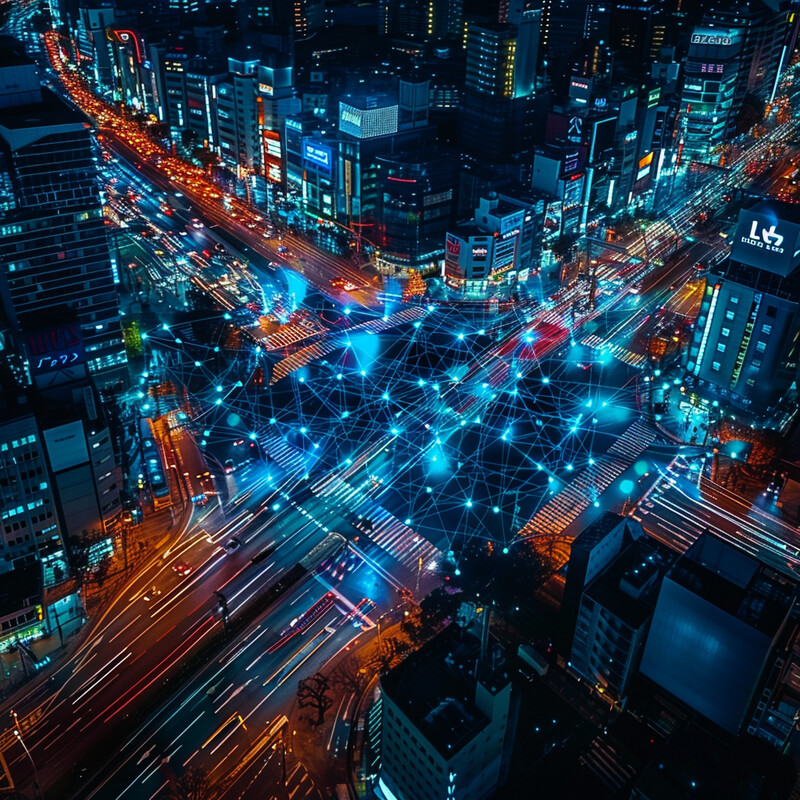
AI algorithms analyze real-time traffic data from cameras, sensors, and GPS devices to optimize the timing of traffic lights and signal phases. By adjusting traffic signals based on current traffic conditions, AI helps to reduce congestion, minimize stopping times, and improve the overall flow of vehicles through intersections and across urban networks, leading to a smoother and faster commute.
2. Incident Detection and Response
AI systems quickly detect accidents or road blockages and automatically adjust traffic signals or notify relevant authorities, minimizing traffic disruption and enhancing road safety.
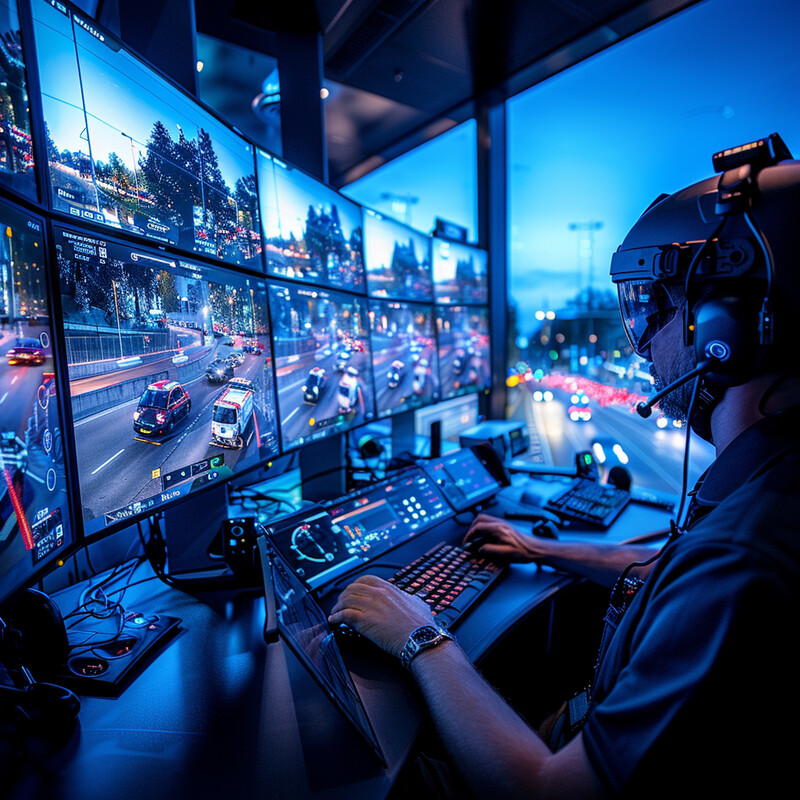
AI-powered systems quickly detect road incidents such as accidents or unexpected blockages by analyzing data from traffic cameras and sensors. Once an incident is detected, the AI system can automatically alert traffic management centers and emergency responders, and adjust traffic signals in real-time to redirect flow away from the incident, thereby enhancing road safety and minimizing congestion.
3. Predictive Traffic Modeling
AI uses historical data and current conditions to predict traffic patterns, helping city planners and drivers anticipate and avoid potential congestion.
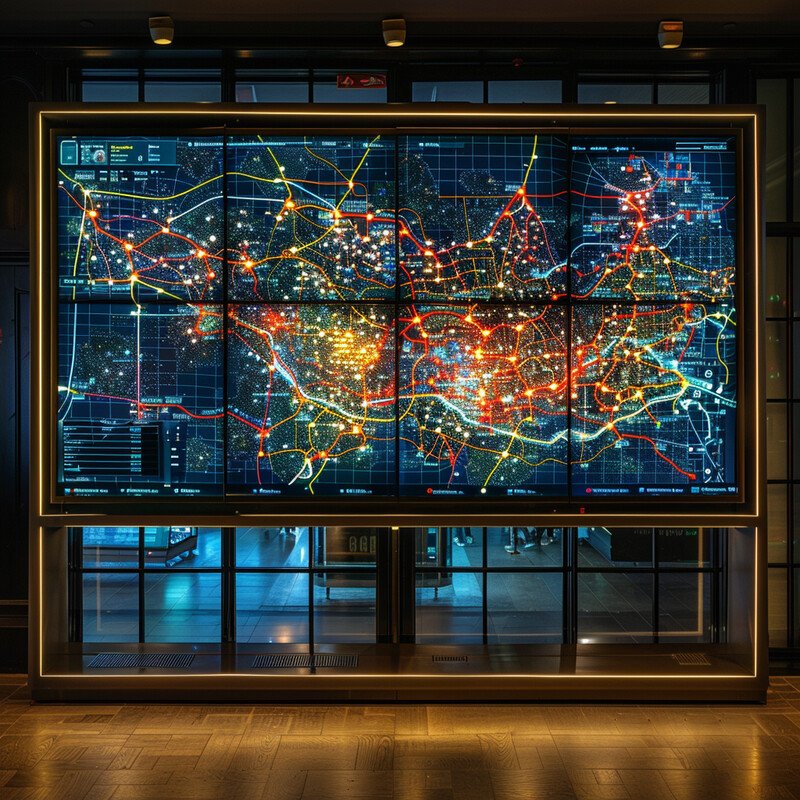
Using historical traffic data and patterns along with real-time inputs, AI models predict future traffic conditions. This predictive capability allows city planners and drivers to anticipate traffic volumes and speeds at different times and locations, aiding in better traffic management planning and providing commuters with insights to avoid potential congestion.
4. Public Transport Management
AI optimizes the schedules and routes of public transportation based on real-time demand and traffic conditions, enhancing the efficiency and reliability of public transit systems.
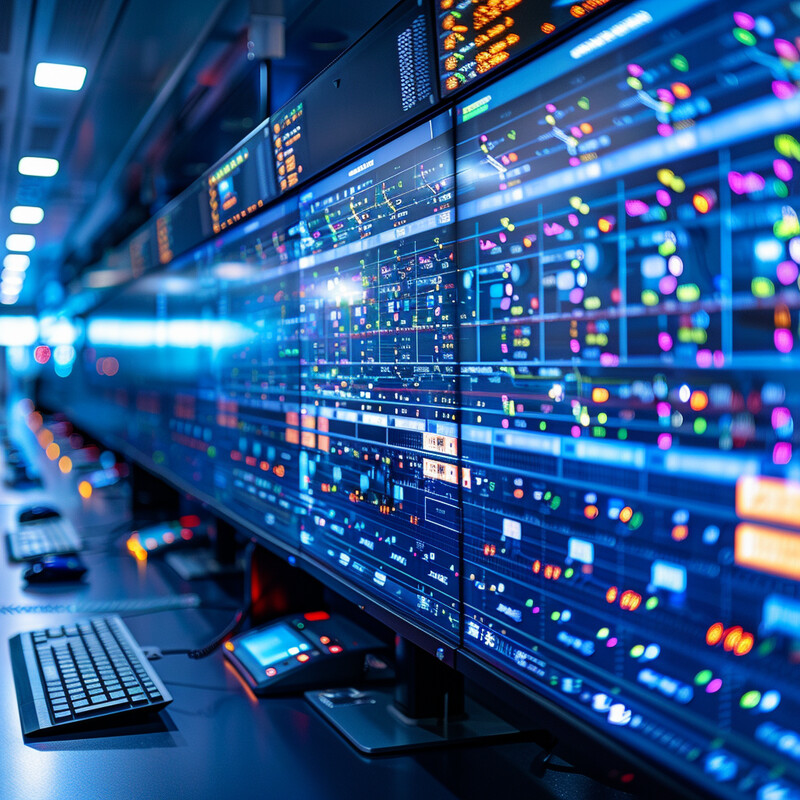
AI optimizes public transport by analyzing passenger data, traffic conditions, and vehicle availability to dynamically adjust routes and schedules. This ensures that buses, trams, and trains can respond in real-time to changes in passenger demand and traffic conditions, improving punctuality and service availability while reducing unnecessary wait times and journey durations.
5. Pedestrian Safety Improvements
AI-powered surveillance systems enhance pedestrian safety by identifying risky behaviors and potential collision points, enabling proactive measures to protect pedestrians.
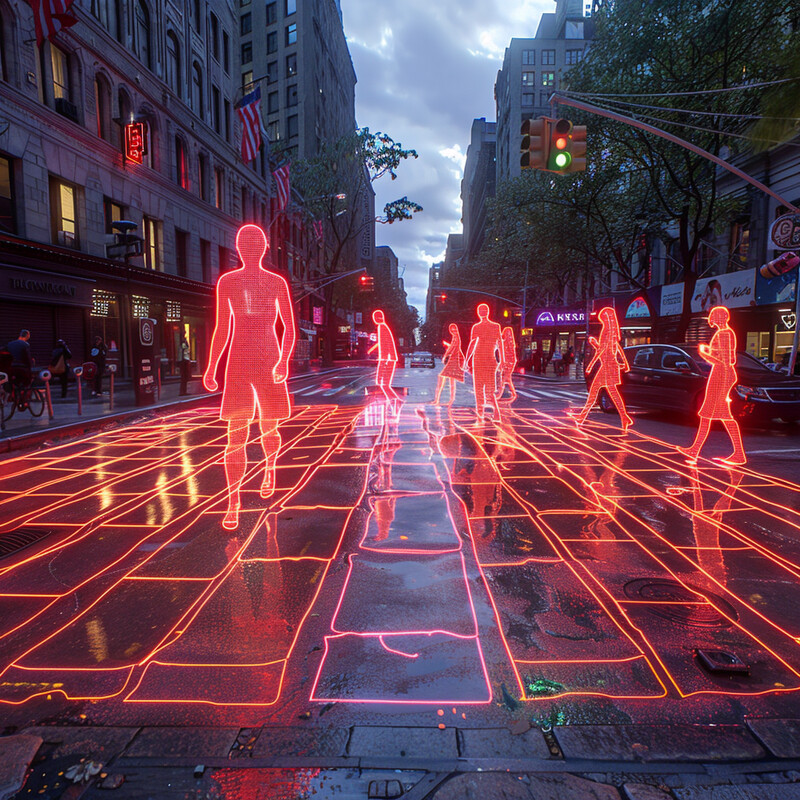
AI-enhanced surveillance systems monitor pedestrian movements and interactions with vehicular traffic using cameras and sensors. By identifying risky behaviors or potential hazards, AI systems can trigger immediate traffic control measures such as pedestrian alerts at crosswalks or adjusting walk times at traffic signals, significantly enhancing pedestrian safety.
6. Connected Vehicle Systems
AI facilitates communication between vehicles and traffic infrastructure (V2I) to provide drivers with real-time traffic updates and safety warnings.
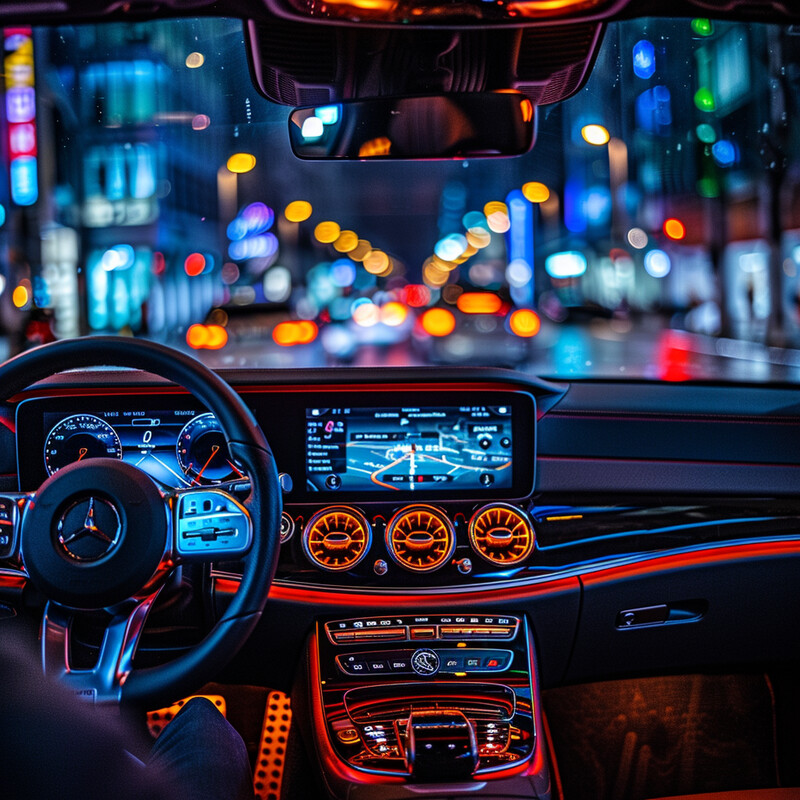
In connected vehicle systems, AI processes data exchanged between vehicles and traffic infrastructure (V2I communication) to provide real-time traffic updates, safety warnings, and route suggestions directly to vehicle dashboards. This integration helps drivers make informed decisions, enhancing traffic flow and road safety.
7. Parking Optimization
AI analyzes parking patterns and guides drivers to available parking spaces, reducing the time spent searching for parking and the associated traffic congestion.
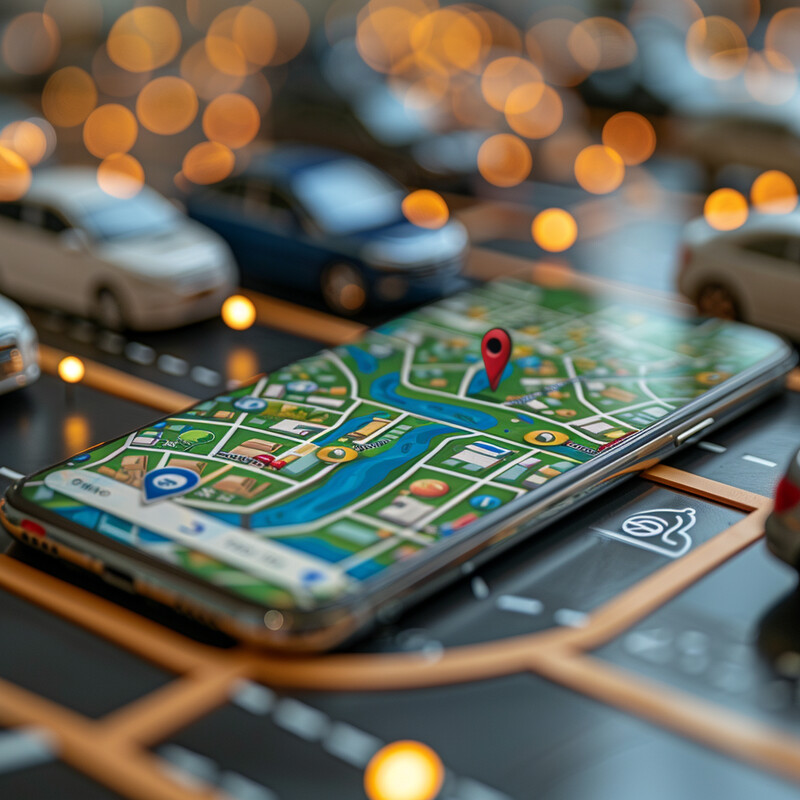
AI assists in parking management by analyzing data from parking lots and street parking spaces. It guides drivers to available parking spots via apps or in-car systems, reducing the time and fuel spent driving around looking for parking. This also decreases traffic congestion caused by vehicles circulating in search of parking.
8. Dynamic Toll Pricing
AI implements dynamic toll pricing based on real-time traffic conditions to manage road usage, encouraging drivers to travel at less congested times or use alternative routes.
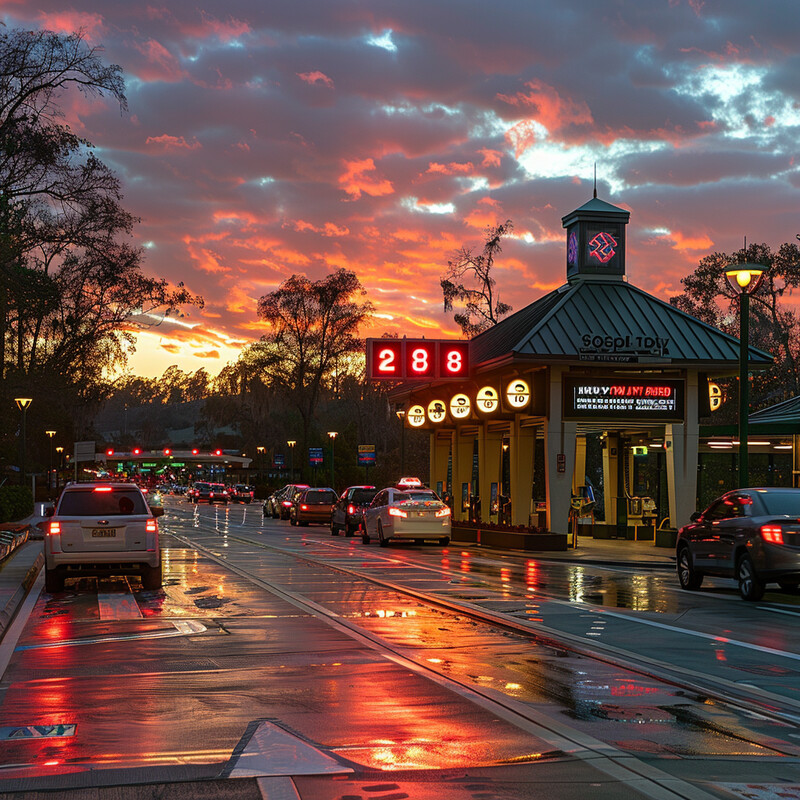
AI implements dynamic toll pricing strategies based on real-time traffic data. By adjusting toll rates during peak and off-peak hours, it encourages drivers to travel during less congested times or use alternative routes, helping to manage road usage and reduce traffic volume during busy periods.
9. Air Quality Monitoring
AI integrates with sensors to monitor and analyze traffic-related air pollution, providing data to support traffic control measures that mitigate environmental impact.
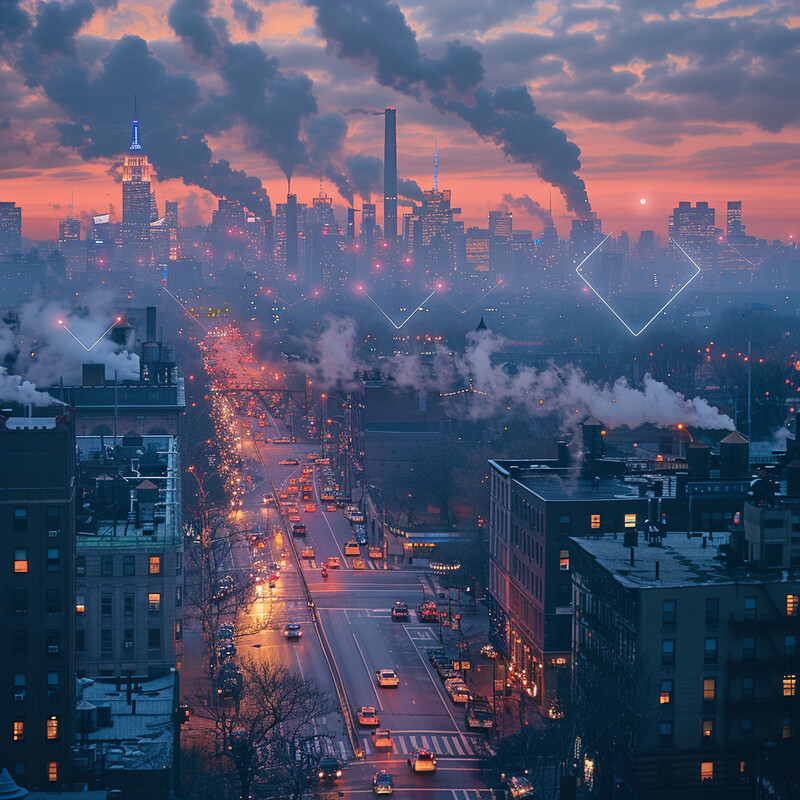
AI integrates with environmental sensors to monitor air quality in areas with heavy traffic. It analyzes emissions data to identify pollution hotspots and patterns, providing valuable insights that can inform traffic rerouting or control measures to improve air quality and public health.
10. Driver Behavior Analysis
AI analyzes the behavior of drivers to identify patterns that could lead to accidents or traffic jams, providing insights into necessary adjustments in traffic signals or road layouts.
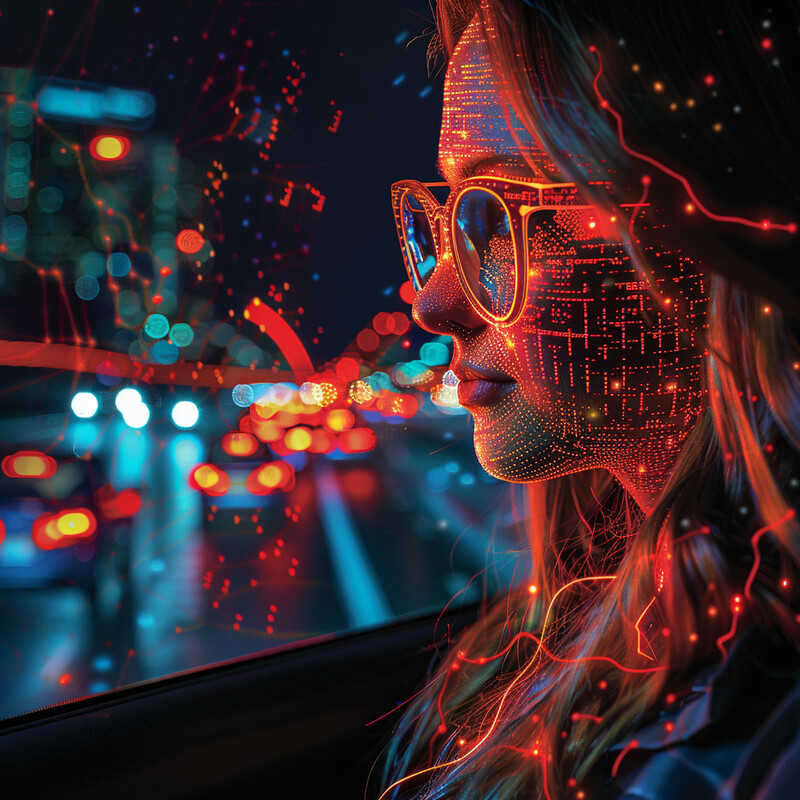
AI analyzes data on driver behavior to identify trends and habits that could lead to congestion or accidents, such as frequent abrupt stopping or speeding. This analysis can inform changes in traffic light timings, speed limits, or road layout to promote smoother traffic flow and reduce accident rates.