1. Automated Patient Triage
AI systems help in assessing patient symptoms through virtual consultations, prioritizing cases based on urgency and directing patients to the appropriate level of care.
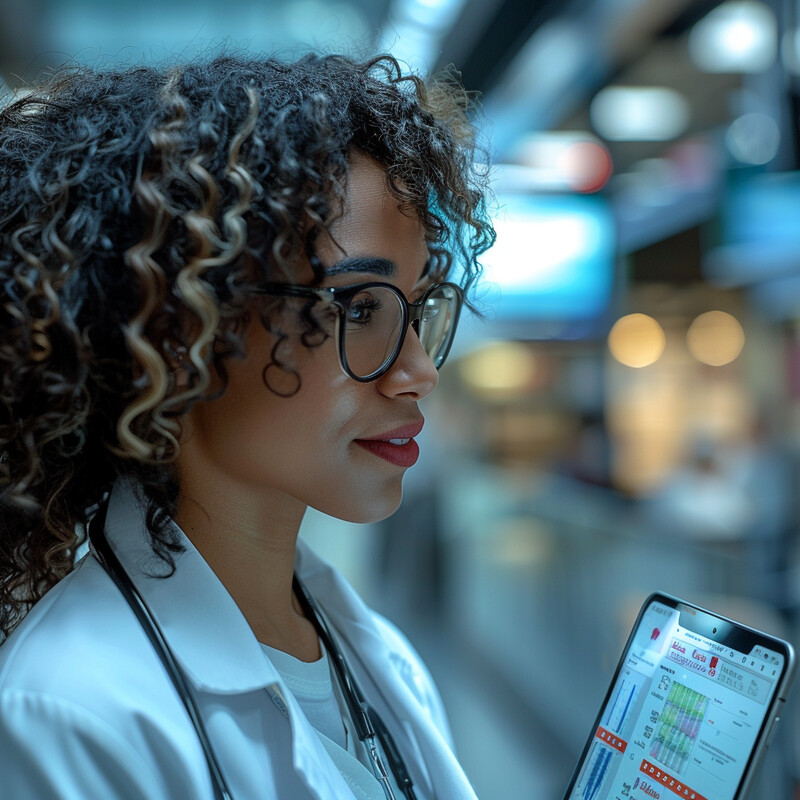
AI systems in telemedicine can efficiently handle initial patient consultations by assessing symptoms reported via online platforms. These AI-driven systems prioritize cases based on the severity and urgency of symptoms, ensuring that patients receive timely care while efficiently managing healthcare resources. This automation helps streamline the triage process, directing patients to the appropriate healthcare level without the need for immediate human intervention.
2. Diagnostic Support
AI enhances diagnostic accuracy by analyzing medical images, patient data, and historical health records, providing support to doctors in making more informed diagnostic decisions.
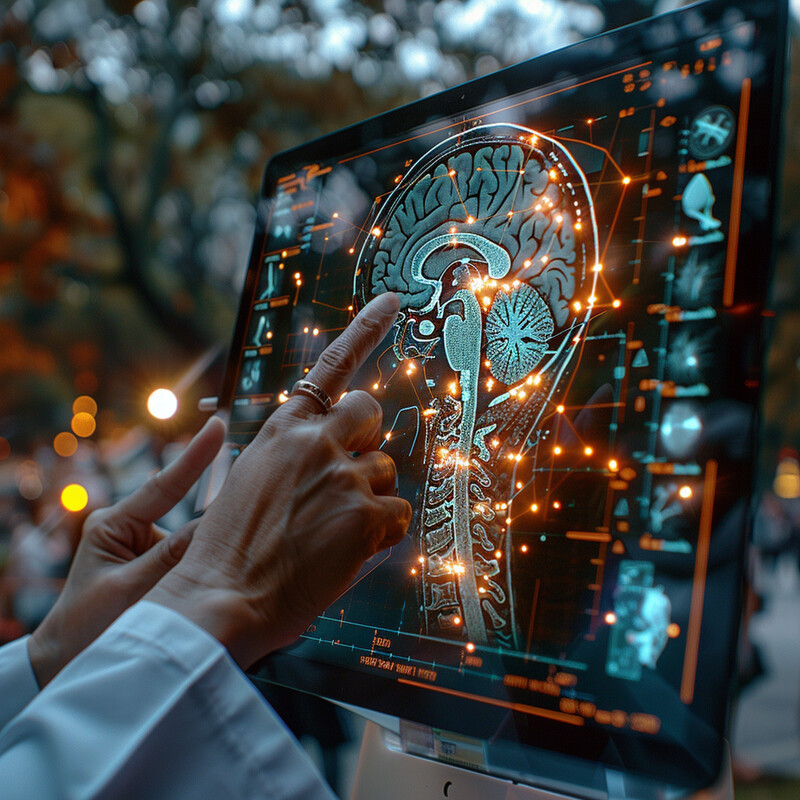
AI significantly supports diagnostics in telemedicine by analyzing medical images, patient histories, and real-time data during virtual consultations. Through deep learning and pattern recognition, AI can highlight areas of concern in medical images like X-rays or MRIs, aiding doctors in identifying subtle abnormalities that may not be immediately apparent, thus enhancing diagnostic accuracy and speed.
3. Personalized Treatment Plans
AI algorithms analyze patient data to recommend personalized treatment plans, taking into account individual health histories, genetic information, and lifestyle factors.
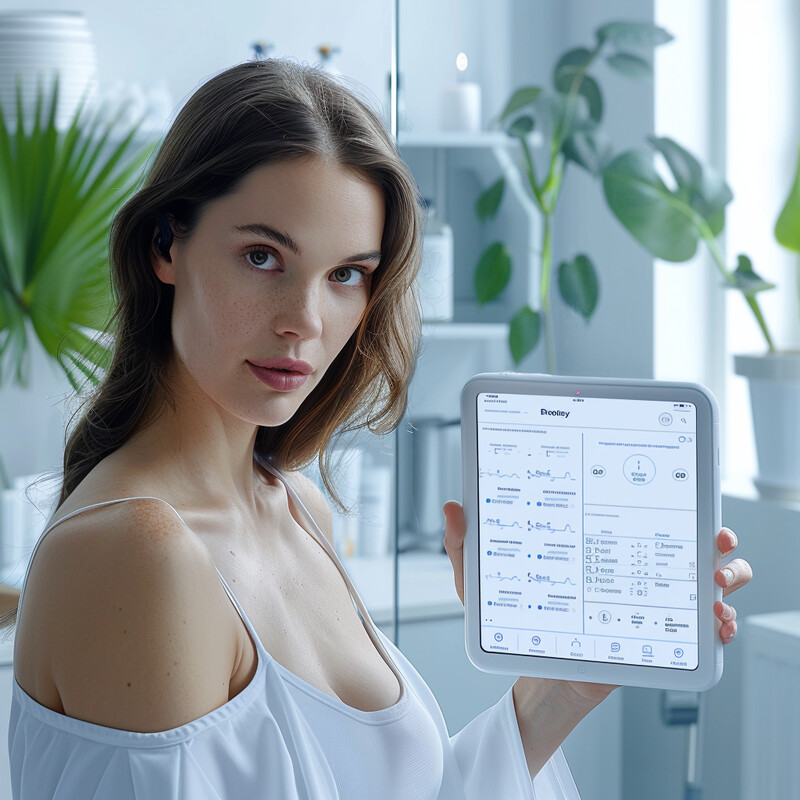
AI leverages data from a patient’s medical history, genetic information, and ongoing health data to tailor treatment plans specifically suited to individual needs. This personalization ensures that treatments are more effective, with adjustments made in real time based on patient responses and new health data collected through telemedicine platforms.
4. Medication Management
AI automates prescription management, helping to track medication schedules, monitor adherence, and alert patients when it’s time to take or refill their prescriptions.
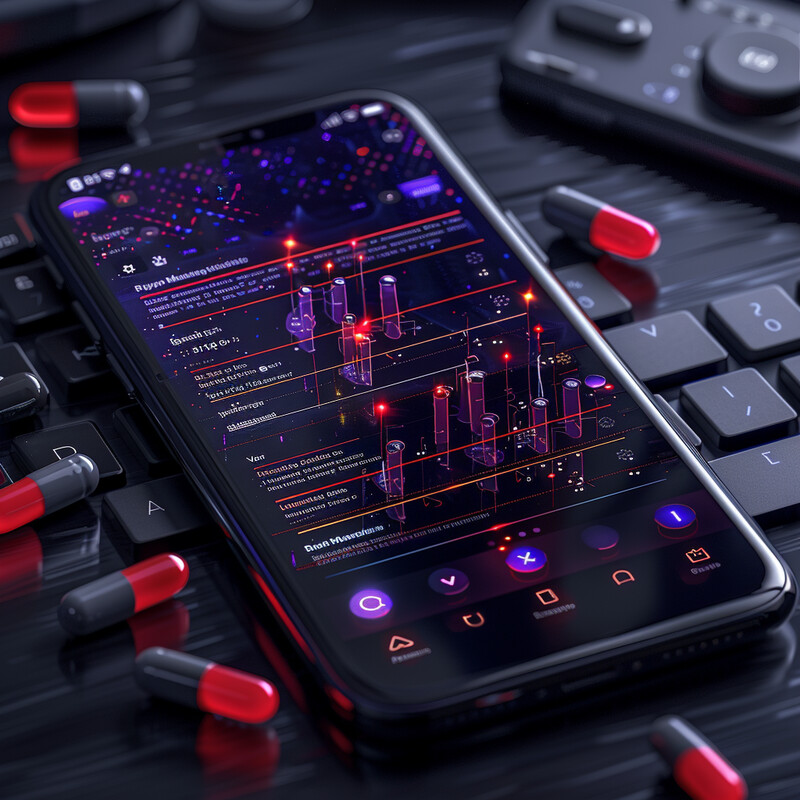
AI systems automate various aspects of medication management, including scheduling dosages, sending reminders to patients, and monitoring adherence through patient feedback and sensor data from connected devices. This technology is particularly beneficial for managing complex medication regimes, reducing the risk of errors and improving patient outcomes.
5. Mental Health Assessments
AI-powered tools assess mental health by analyzing speech patterns and writing during virtual consultations, helping to identify signs of mental health issues such as depression or anxiety.
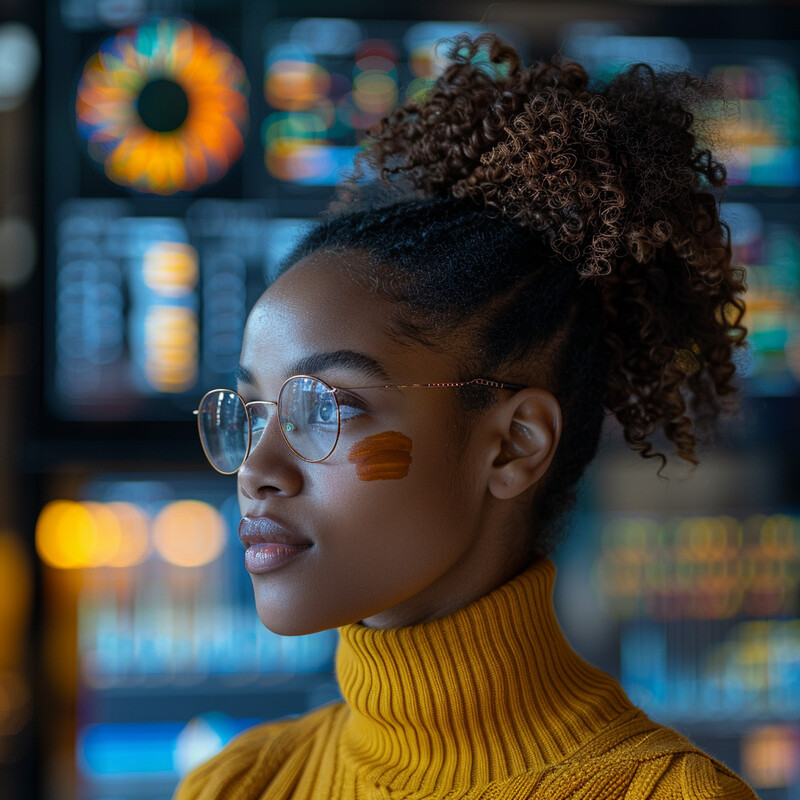
AI-powered tools in telemedicine use natural language processing to analyze patients' speech and writing patterns during virtual consultations. These tools can detect subtle cues that indicate mental health conditions such as depression or anxiety, enabling timely intervention and support from mental health professionals.
6. Remote Patient Monitoring
AI systems continuously monitor patient vitals through wearable devices, alerting healthcare providers if there are any abnormalities that require immediate attention.
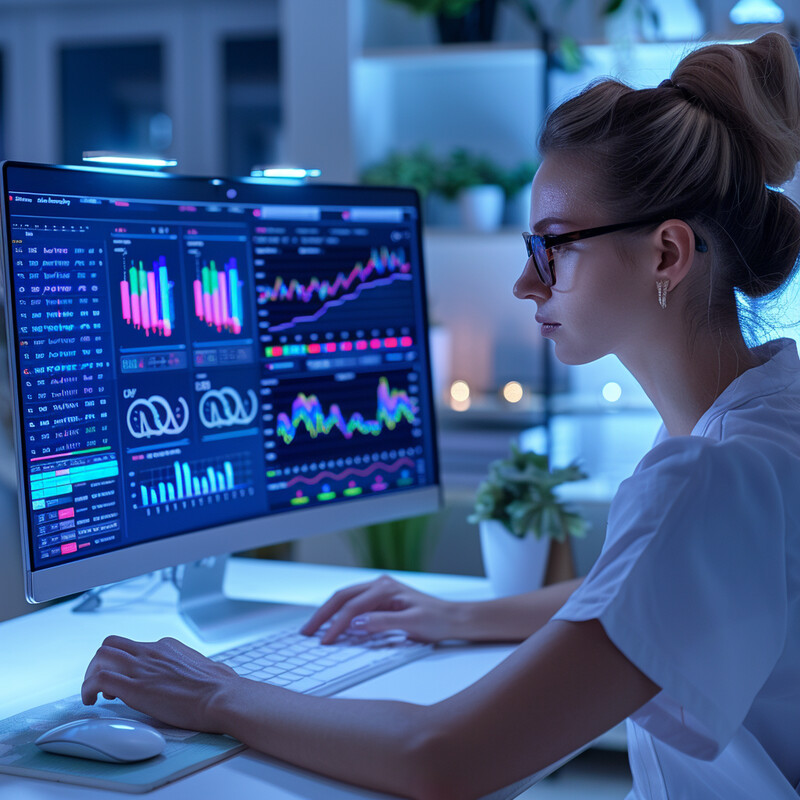
AI enhances remote patient monitoring by continuously analyzing data collected from wearable devices that track vitals like heart rate, blood pressure, and glucose levels. AI algorithms can detect deviations from normal ranges and immediately alert healthcare providers, facilitating prompt responses to potential health issues.
7. Chronic Disease Management
AI helps manage chronic conditions by providing regular assessments, personalized advice, and early warning signs of complications, all through remote interfaces.
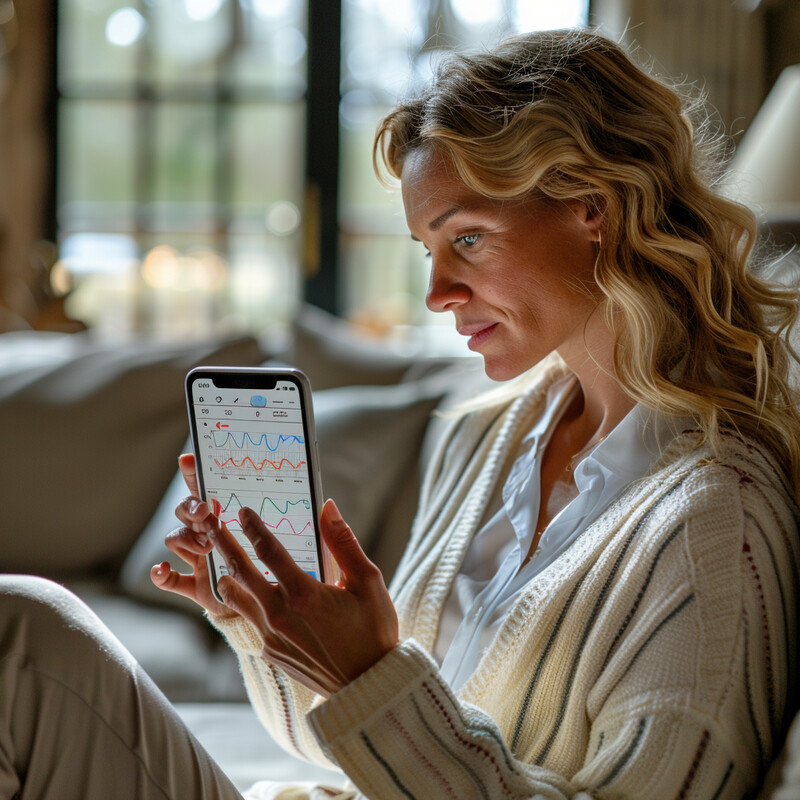
For patients with chronic diseases, AI provides comprehensive management tools through telemedicine platforms. These tools offer regular health assessments, monitor symptoms, and provide personalized feedback and alerts for managing conditions such as diabetes or heart disease, helping to prevent complications and improve quality of life.
8. Health Education and Engagement
AI delivers customized educational content and health maintenance reminders to patients, based on their specific conditions and treatment plans, to encourage active participation in their health management.
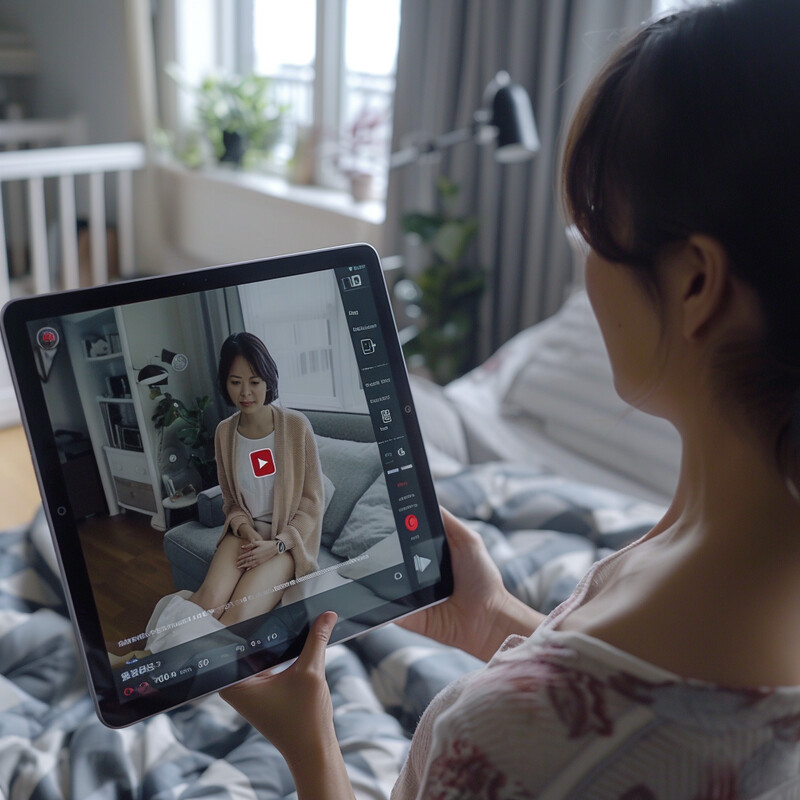
AI systems deliver tailored educational content directly to patients based on their specific health conditions and treatment plans. This personalized approach ensures that patients receive relevant information and are reminded of health maintenance activities, encouraging greater engagement and proactive management of their health.
9. Predictive Analytics
AI analyzes data across numerous patients to predict health trends and potential emergencies before they occur, enabling proactive rather than reactive care.
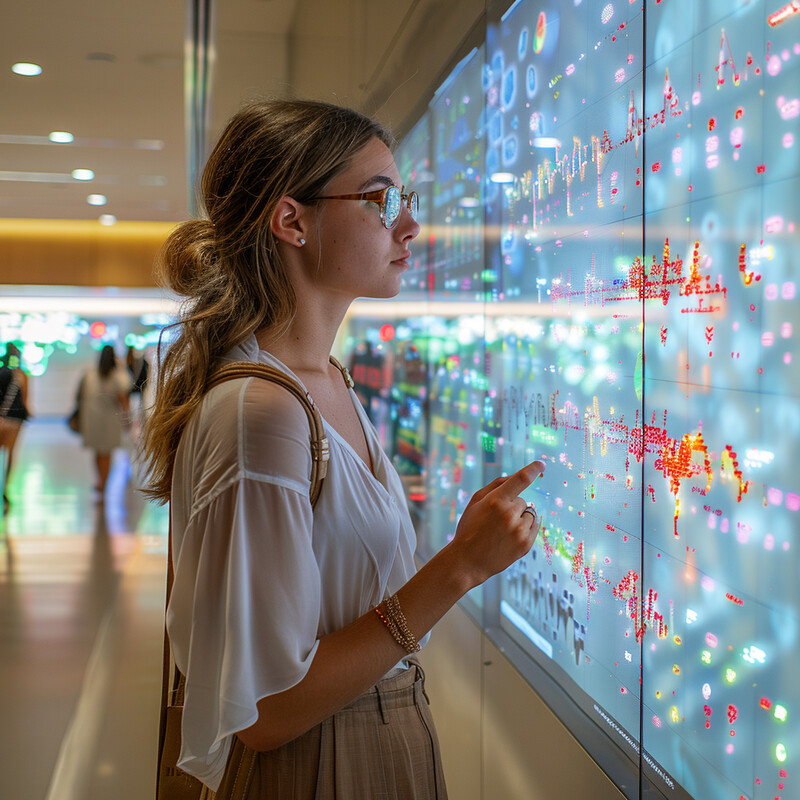
AI uses predictive analytics in telemedicine to analyze health trends across a population and predict potential health emergencies before they occur. This capability allows healthcare providers to offer preemptive advice and interventions, potentially preventing hospitalizations and managing health issues more effectively.
10. Language Translation Services
AI enhances communication between patients and healthcare providers who speak different languages, breaking down barriers in telemedicine consultations and expanding access to care.
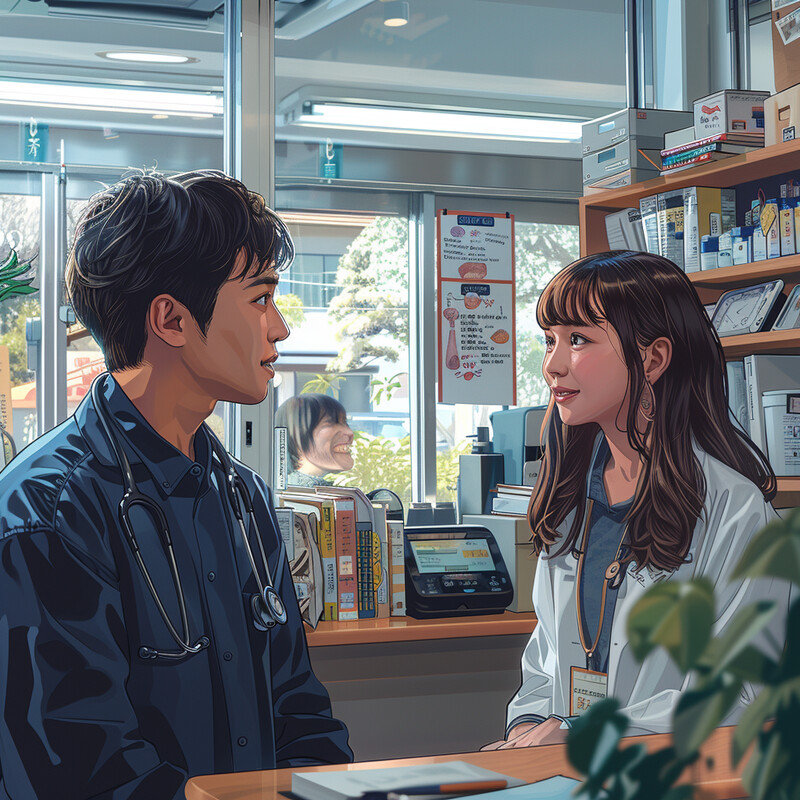
AI-powered language translation services in telemedicine break down language barriers, making healthcare more accessible to non-native speakers. These services translate conversations in real-time during virtual consultations, ensuring clear communication between patients and healthcare providers and expanding access to quality care.