1. Demand Forecasting
AI is revolutionizing demand forecasting by leveraging machine learning models that can analyze vast datasets and detect complex patterns beyond human capability. Advanced algorithms (including deep learning and even transformer-based time series models) integrate diverse data sources – from historical sales and economic indicators to real-time weather or social media trends – to predict demand more accurately. These AI-driven systems continuously learn and adapt, allowing forecasts to update in real time as new data arrives. This leads to more agile planning: companies can respond faster to market changes, reducing stockouts and excess inventory. Overall, AI enables demand planning to be more data-driven and precise, which improves supply chain efficiency and profitability through better alignment of inventory with actual demand.
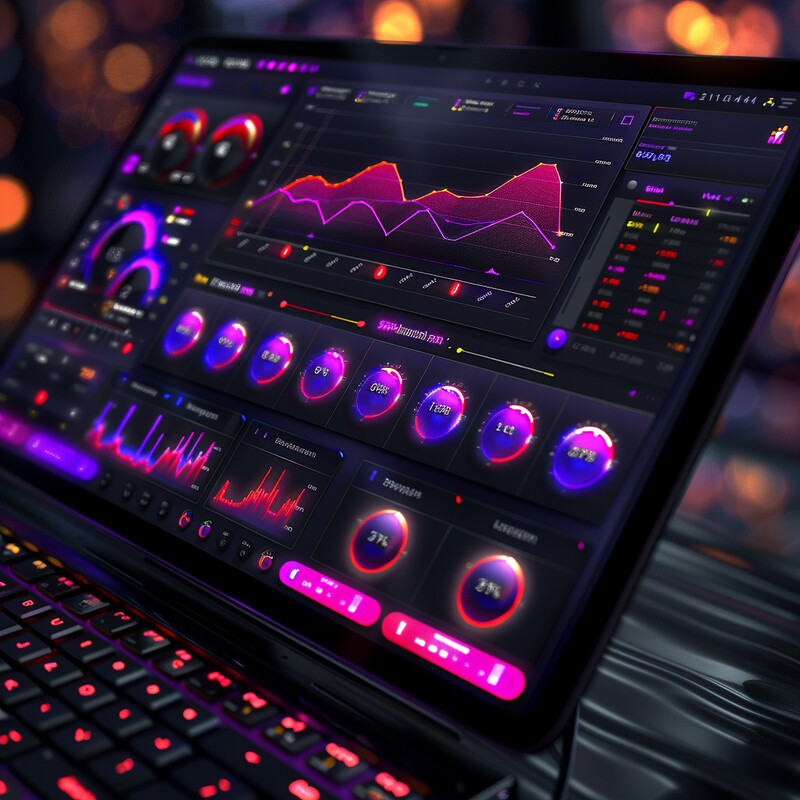
Research in 2024 found that companies adopting AI for demand forecasting achieved up to a 30% improvement in forecast accuracy compared to traditional methods. By using machine learning to incorporate external factors (like economic and consumer behavior data) that conventional tools might miss, AI-based forecasts significantly boost accuracy and responsiveness. For example, AI systems can automatically adjust forecasts when sensing shifts in real-time data, helping businesses optimize stock levels and reduce forecasting errors. This improvement not only cuts inventory costs but also enhances customer satisfaction by ensuring products are available when and where needed.
2. Supplier Selection and Management
AI is transforming how organizations identify, evaluate, and collaborate with suppliers. Machine learning models can analyze supplier performance data, financial stability indicators, and even news or social media sentiment to assess supplier risk and reliability. In supplier selection, AI-driven decision support tools help procurement teams weigh numerous criteria (price, quality, delivery times, compliance records) far more quickly and consistently than manual methods. Natural language processing (NLP) algorithms also assist by reviewing supplier documents and contracts to flag potential issues or opportunities. Once relationships are established, AI aids ongoing supplier management through automated performance scorecards and anomaly detection (e.g., flagging unusual lead time spikes or quality dips). By automating routine tasks like vendor screening and integrating diverse data for risk analysis, AI enables a more strategic and resilient approach to managing suppliers across the supply chain.
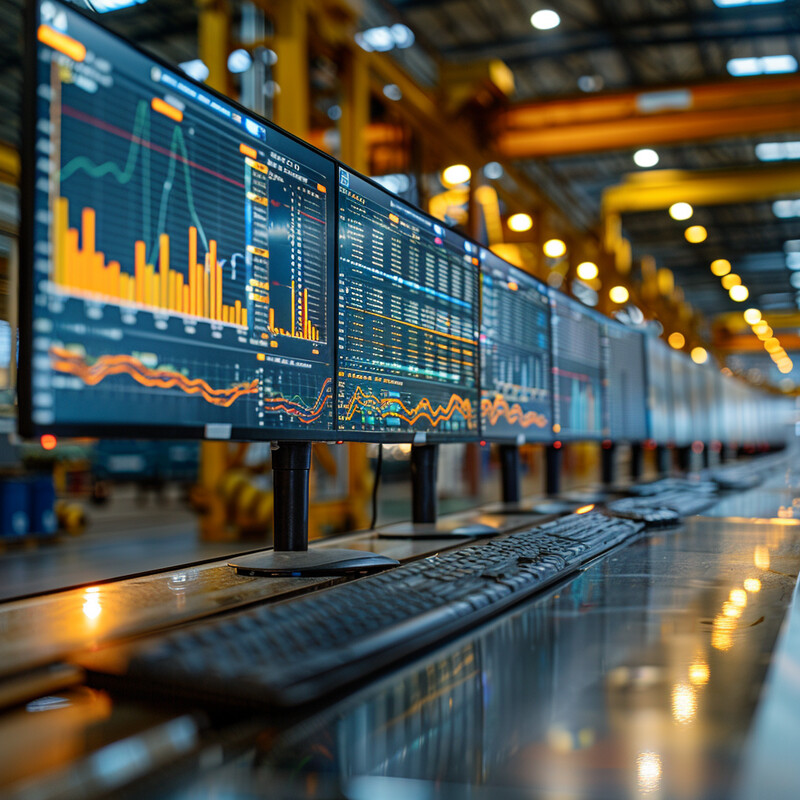
Adoption of AI in procurement is growing: in a 2024 survey of procurement leaders, 36% reported using AI for automated supplier selection and negotiation, and 52% were using AI tools for supplier risk management. These AI applications help teams efficiently evaluate suppliers and continuously monitor supplier performance or compliance risks. For instance, machine learning systems can automatically score suppliers on key performance metrics or predict risk events (like supply disruptions), allowing procurement managers to act proactively. The survey results indicate that many organizations are already leveraging AI to make supplier selection more data-driven and to identify at-risk suppliers early.
3. Route Optimization
AI technologies are elevating route optimization in logistics by processing real-time information and solving complex routing problems faster than ever. Modern route optimization tools use AI algorithms – often combining traditional operations research with machine learning – to calculate the most efficient delivery or travel routes. They consider factors like traffic patterns, weather conditions, fuel consumption, and delivery time windows simultaneously. Unlike static routing of the past, AI-powered systems can dynamically re-route vehicles in real time if there are disruptions (e.g., a sudden road closure or port delay). Some implementations use reinforcement learning, where AI “learns” optimal routing strategies over time from past trip data. The result is more efficient transportation: reduced mileage, lower fuel use, and faster delivery times. AI-driven route optimization is especially transformative in last-mile delivery, where it can sequence stops for delivery vans or autonomous robots in a way that minimizes delays and meets tight customer delivery expectations.
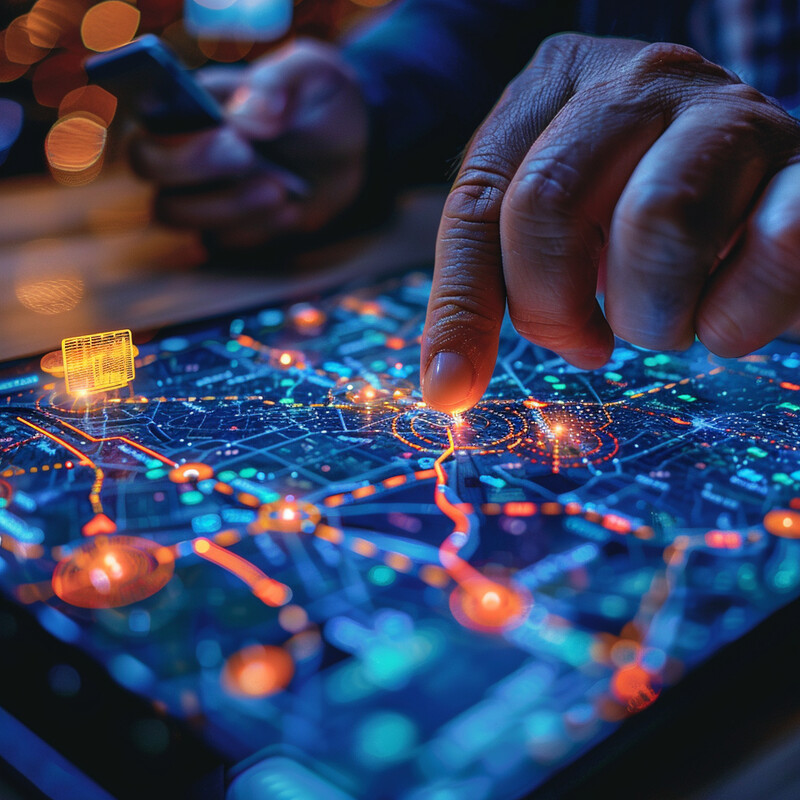
Logistics data from 2023 shows a rapid increase in AI-driven route planning. In the last-mile delivery sector, the use of AI for route optimization and related tasks jumped by 39% in 2023 compared to the prior year. Companies are investing in AI route optimization tools to cut down on delivery times and fuel costs. Indeed, industry reports (including DHL’s logistics analysis) indicate that AI-driven routing and predictive analytics can boost delivery efficiency by roughly 20%, through smarter scheduling and real-time adjustments. This means faster deliveries and fewer miles traveled, which not only reduces costs but also contributes to lower emissions.
4. Warehouse Management
AI is at the heart of the smart warehouse revolution. In modern warehouses, AI-driven systems manage inventory, optimize storage layout, and direct autonomous equipment to streamline operations. Machine learning algorithms analyze order patterns and inventory levels to predict future stock needs and ideal storage locations (“slotting”) for fast-moving items. Robotics powered by AI – such as autonomous mobile robots (AMRs) and robotic arms – are increasingly handling picking, packing, and palletizing. These robots use AI-based vision and control systems to navigate warehouse aisles safely and identify the correct items, significantly speeding up order fulfillment. AI also enables real-time inventory tracking: computer vision cameras and sensors, combined with image recognition algorithms, can count stock or detect empty shelf space with high accuracy. Furthermore, AI helps orchestrate labor and equipment by forecasting workloads and dynamically scheduling tasks (like replenishment or cycle counting) at optimal times. By reducing human error and automating repetitive tasks, AI-enhanced warehouses achieve greater accuracy in order fulfillment, higher throughput, and improved safety for workers who collaborate with intelligent machines.
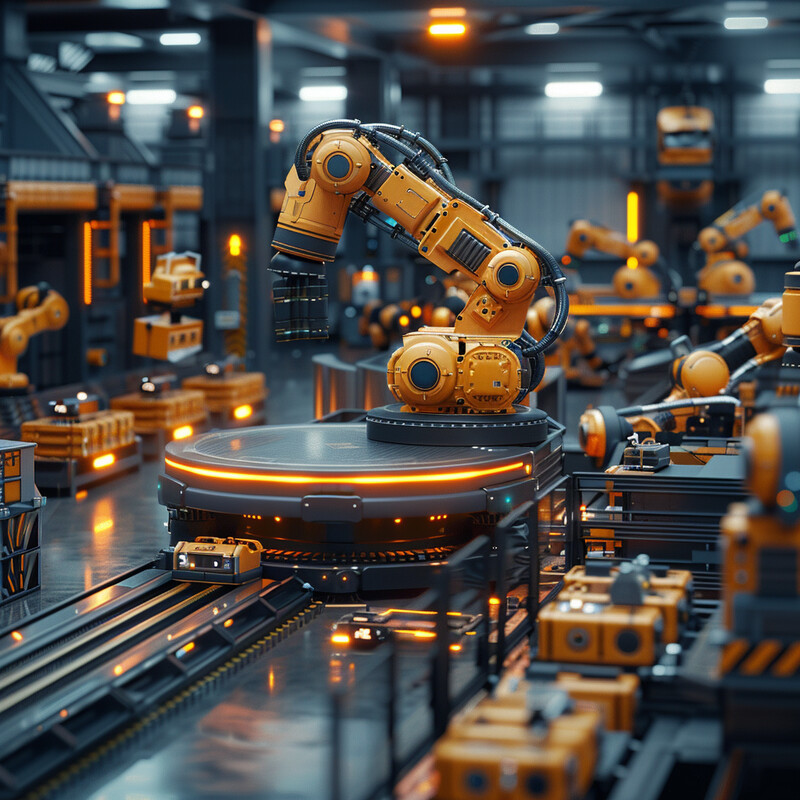
AI-powered automation dramatically improves warehouse accuracy and efficiency. Studies show that implementing robotic picking systems driven by AI can cut picking errors by up to 70%, significantly boosting productivity and order accuracy in fulfillment centers. For example, AI-guided robots equipped with sensors and vision can identify products and execute picks with precision, virtually eliminating the misplacement or mispicks that human workers might make when rushed. Such error reduction not only improves customer satisfaction (fewer incorrect orders) but also reduces waste and re-shipping costs. Additionally, AI-driven warehouse solutions often lead to faster operations — some facilities report 20–30% reductions in labor costs and quicker order cycle times after deploying AI robotics, as the technology handles repetitive tasks and allows human workers to focus on oversight and exception handling.
5. Predictive Maintenance
AI enables predictive maintenance by analyzing data from machines to predict equipment failures before they happen. In supply chain operations (factories, distribution centers, transport fleets), sensors on equipment continuously collect performance data – temperatures, vibrations, pressure readings, etc. Machine learning algorithms then process this “industrial IoT” data to learn patterns that precede malfunctions or wear-and-tear issues. Unlike traditional maintenance schedules (e.g., servicing a machine every 6 months regardless of condition), AI can identify the subtle signals of an upcoming failure and alert maintenance teams to intervene only when needed. Techniques like anomaly detection and predictive modeling mean that a system can warn, for example, that a conveyor motor has an 80% chance of failing in the next 10 days due to unusual vibration trends. With such foresight, companies can schedule repairs or part replacements during planned downtime, avoiding unexpected breakdowns. This AI-driven approach minimizes unplanned downtime, extends the lifespan of equipment, optimizes spare parts inventory, and reduces maintenance costs – all by shifting maintenance from reactive to proactive and data-driven.
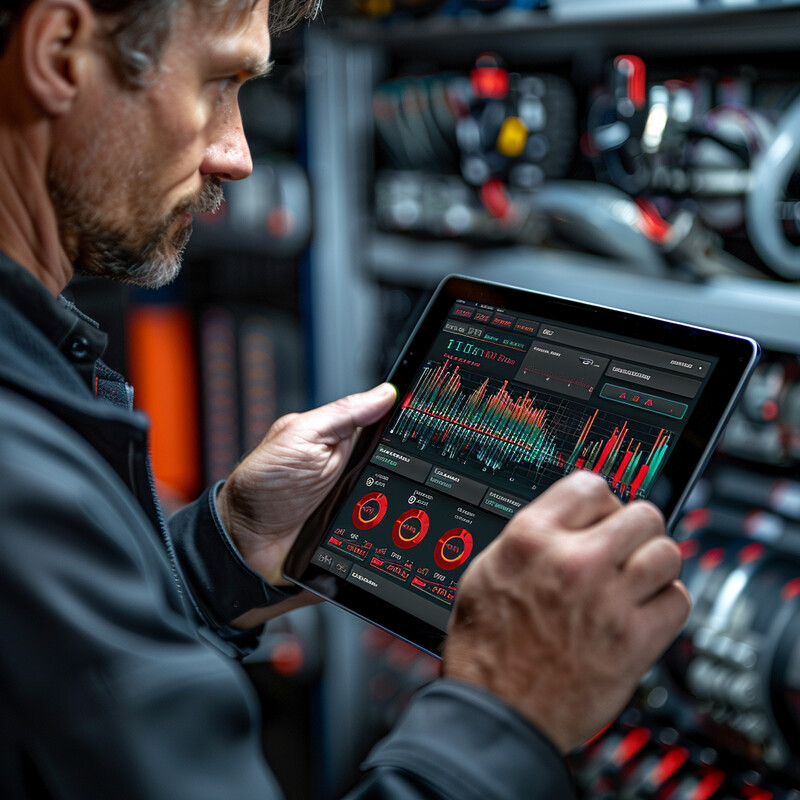
Manufacturers have rapidly embraced AI for maintenance: in 2023, about 70% of manufacturing companies were using AI-based predictive maintenance solutions to keep their equipment running smoothly. This high adoption reflects AI’s proven value in reducing unexpected breakdowns. Companies using machine learning to monitor machine health have reported substantially less unplanned downtime – studies by McKinsey and others note that AI-driven predictive maintenance can cut unplanned downtime by up to 50%. The widespread use of AI in this area means maintenance personnel get early warnings of issues (for instance, a production robot showing anomaly signals), allowing them to fix problems during scheduled maintenance windows. As a result, manufacturers improve their uptime and productivity, and they often see longer equipment life and lower maintenance costs due to the more precise targeting of maintenance activities.
6. Fraud Detection
AI has become a crucial tool for detecting and preventing fraud in supply chain activities and related financial transactions. Machine learning models excel at spotting anomalies in large datasets, which is key for fraud detection – they can flag unusual patterns in purchase orders, invoices, or payment records that might indicate procurement fraud, false billing, or cyber-attacks. For example, AI can automatically analyze thousands of transactions to find outliers, such as an abnormally high-priced invoice from a new vendor or a sudden spike in refund claims, and raise an alert for investigation. In addition, AI-driven fraud systems often use techniques like network analysis (graph algorithms) to uncover hidden relationships, identifying if a group of supposedly unrelated suppliers is actually controlled by the same entity (a red flag for collusion or shell companies). Because AI can process data in real time, it enables near-instantaneous fraud checks – stopping suspicious transactions before they go through. Over time, these systems learn from confirmed fraud cases, continuously improving their accuracy. By automating fraud surveillance and catching subtler patterns that humans might miss, AI helps companies significantly reduce losses due to fraud, ensure compliance, and maintain trust across their supplier and customer networks.
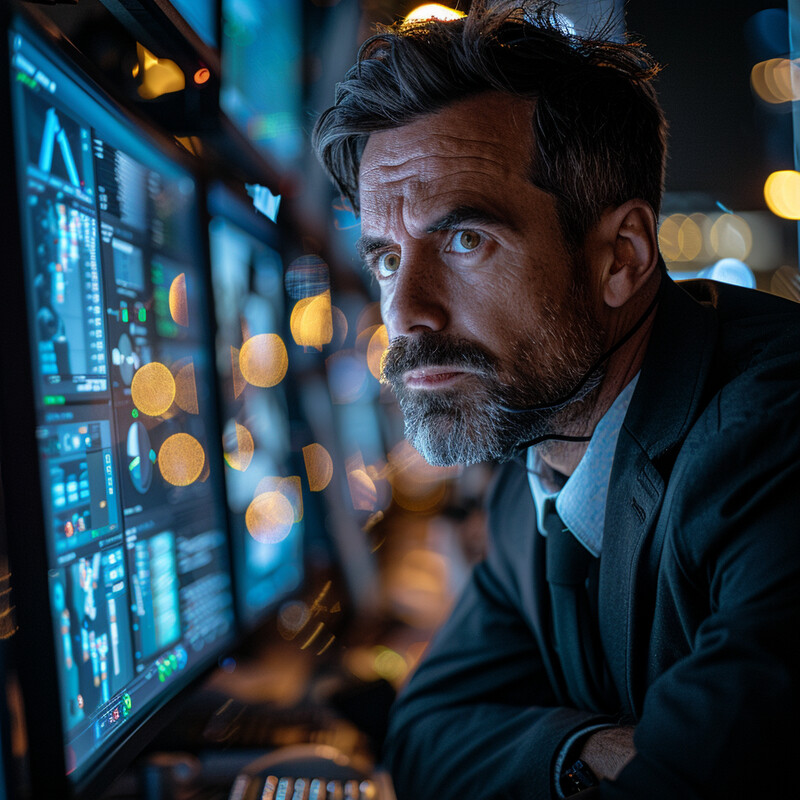
AI-driven systems vastly improve fraud detection efficacy. According to a recent market analysis, organizations that employ AI for fraud detection report a 98% success rate in identifying fraudulent activities in their operations. This extremely high detection rate underscores how effective modern AI algorithms have become at catching fraud that would previously slip through. The AI models can cross-verify data points (prices, quantities, vendor details, timing) across the supply chain and financial systems, quickly flagging inconsistencies. For instance, if a fraudulent invoice is submitted, an AI system can instantly compare it against historical norms and supplier profiles, and mark it as suspicious with near-perfect accuracy. Such success rates mean companies using AI are far more likely to intercept fraud attempts early, protecting their assets and reducing potential financial losses.
7. Customer Service Automation
AI is reshaping customer service in supply chain and logistics by enabling intelligent automation of customer interactions. Companies now deploy AI-powered chatbots and virtual assistants on their websites or messaging platforms to handle common customer inquiries around the clock. These AI chatbots use natural language processing to understand customer questions (e.g., “Where is my order?” or “I need to change my delivery address”) and provide instant responses or self-service solutions. For more complex issues, AI can triage and route queries to the appropriate human agent, along with context it has gathered, improving efficiency. Machine learning also personalizes service: AI can recognize a repeat customer and tailor responses based on their order history or preferences. Beyond text chat, AI voice assistants are being used on customer service phone lines, conversationally guiding callers or providing shipment tracking updates without human intervention. Importantly, AI doesn’t just work in isolation – many companies use AI to assist human support agents as well, for example by suggesting response prompts or pulling up relevant data instantly during a live call. By blending automation with human support, AI in customer service reduces wait times, provides 24/7 responsiveness, and frees up human agents to focus on more complex customer needs, thereby enhancing overall customer satisfaction.
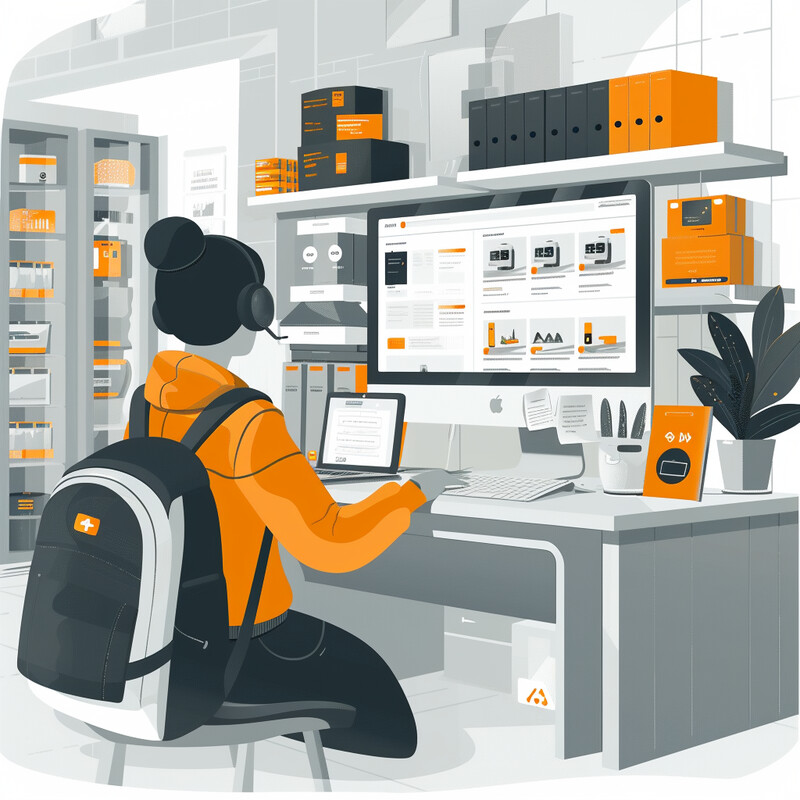
AI adoption in customer service has become mainstream. By 2025, an estimated 80% of companies will be using (or planning to use) AI-powered chatbots to handle at least part of their customer service operations (Gartner, 2023). This high adoption forecast reflects the trend already underway: many businesses have introduced conversational AI to answer FAQs, track orders, and initiate returns for customers automatically. Surveys of consumer behavior show a growing acceptance of these AI assistants – a majority of customers find that well-implemented chatbots are helpful for quick issues, and they appreciate immediate responses at any hour. On the business side, companies report that AI customer service bots can resolve a large volume of routine inquiries, typically cutting customer wait times dramatically. The result is that by mid-decade, utilizing AI in customer support is no longer seen as optional, but as a standard practice to improve service speed and consistency while controlling costs.
8. Risk Management
AI is becoming an indispensable ally in supply chain risk management by providing earlier detection of risks and deeper insights for mitigation. Supply chains face diverse risks – from supplier failures and demand fluctuations to geopolitical events and natural disasters – and AI helps monitor and analyze risk factors continuously. Machine learning models can sift through news feeds, social media, and market data to flag emerging risks (for example, an AI system might alert that a key supplier’s region is experiencing political unrest, potentially disrupting supply). AI-enabled risk platforms also use predictive analytics to model “what-if” scenarios: they can simulate how a hurricane or a sudden surge in demand would impact the supply chain, allowing companies to prepare contingency plans. For operational risks, AI analyzes data from across the supply chain (inventory levels, in-transit shipments, production status) to identify anomalies that could indicate a problem brewing, such as a production slowdown or transportation bottleneck. By quantifying probabilities and potential impacts of various risk events, AI assists managers in prioritizing their risk response efforts. Moreover, AI can optimize risk mitigation strategies – for instance, recommending how to reallocate inventory or switch sourcing if a risk is detected. In essence, AI augments human risk managers by processing far more data in real time than any team could, thereby enabling proactive and informed decisions to strengthen supply chain resilience.
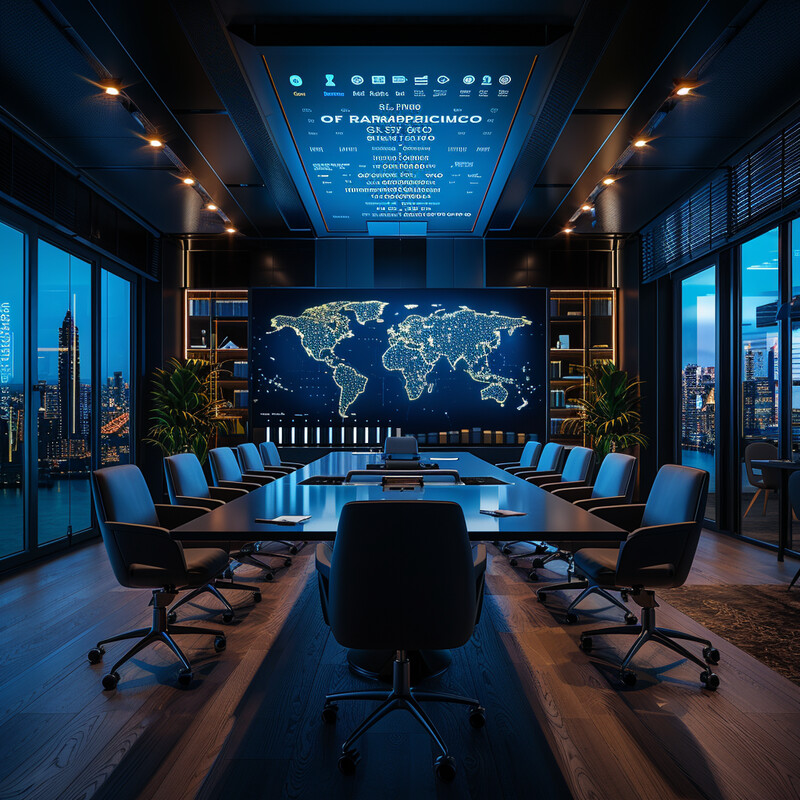
Many organizations are now turning to AI to bolster their risk management. A 2024 survey of risk and compliance professionals found that 62% of organizations either already use or plan to use AI in their risk management processes, while 38% admitted they have no current plans for AI in risk control. The majority with AI on the agenda are applying it in areas like risk forecasting, assessment, and scenario planning – for example, using generative AI to help anticipate future supply chain disruptions and model responses. This means most companies recognize that AI can greatly enhance their ability to predict and manage risks (such as supplier defaults or logistics delays) by providing data-driven early warnings. However, the survey also highlights that a significant minority are lagging in adoption, potentially missing out on the efficiency and foresight AI provides in risk management. As competitive pressure and the complexity of global risks grow, experts expect even more organizations will incorporate AI to avoid being left behind in managing supply chain uncertainties.
9. Sustainability Optimization
AI plays a pivotal role in making supply chains more sustainable by identifying inefficiencies and optimizing processes to reduce environmental impact. One key application is in energy management: AI systems can monitor energy usage across factories or warehouses and adjust settings (like heating/cooling or machine run times) to minimize waste without hindering operations. In transportation, AI route optimization (as discussed) not only cuts costs but also reduces fuel burn and emissions by finding shorter or smoother routes. AI is also used for demand planning and inventory optimization with sustainability in mind – by accurately forecasting demand, companies can avoid overproduction and excessive inventory, which in turn reduces waste and the resources used in producing, storing, and eventually disposing of unsold goods. Another emerging use is AI-driven product lifecycle analysis: algorithms assess sourcing options, production methods, and distribution models to suggest ways to lower a product’s carbon footprint (for instance, by choosing a supplier with cleaner energy or planning shipments to maximize container utilization). Furthermore, AI helps in recycling and circular economy initiatives, such as computer vision systems that improve sorting of recyclable materials in warehouses. By providing data-driven recommendations, AI enables companies to hit sustainability targets like lower greenhouse gas emissions and less waste generation while often simultaneously improving operational efficiency – creating a win-win for business and the environment.
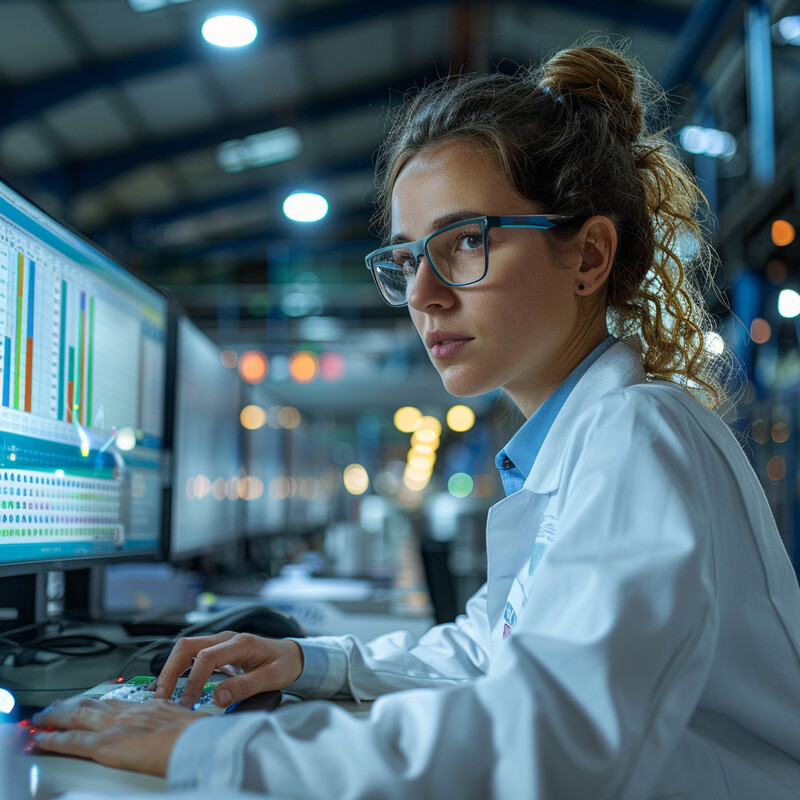
AI adoption correlates strongly with better sustainability outcomes. A global 2024 survey by Boston Consulting Group (BCG) found that companies using AI were 4.5 times more likely to achieve significant decarbonization benefits in their operations compared to companies not using AI. These benefits include major efficiency gains and reductions in emissions – for example, firms leveraging AI to optimize logistics and energy usage reported substantially larger cuts in carbon footprint and cost savings from energy efficiency. In practical terms, an AI-equipped supply chain might consolidate shipments and eliminate empty miles, or intelligently control manufacturing equipment to minimize energy waste, leading to measurable emissions reductions. The survey’s implication is clear: integrating AI into sustainability and carbon-reduction efforts greatly multiplies the chances of success, as AI helps pinpoint exactly where and how to cut resource usage while maintaining performance.
10. Real-Time Visibility
Real-time visibility in supply chains – the ability to know the status of orders, inventory, and shipments at any moment – has been greatly enhanced by AI technologies. Supply chain “control towers” now use AI to aggregate and analyze live data feeds from various sources: IoT sensors on trucks and containers, GPS trackers, ERP systems updates, and even weather or traffic APIs. AI algorithms sift through these streaming data to provide a unified, up-to-the-minute view of where goods are and to predict estimated arrival times or potential delays. If a disruption occurs (like a port congestion or a production line stoppage), AI can quickly alert managers and even suggest corrective actions, such as re-routing a shipment or expediting an alternative source. Machine learning improves these predictions over time; for example, by learning typical transit times and bottlenecks, the system can more accurately predict when a shipment will be late. Additionally, AI aids in exception management: rather than inundating users with raw data, it highlights anomalies like a shipment deviating from its route or an inventory level dropping unexpectedly. This real-time insight allows companies to respond faster – mitigating the impact of delays, informing customers proactively, and adjusting plans on the fly. In summary, AI-driven visibility tools create a transparent, responsive supply chain where information flows freely and decisions can be made with current, accurate data.
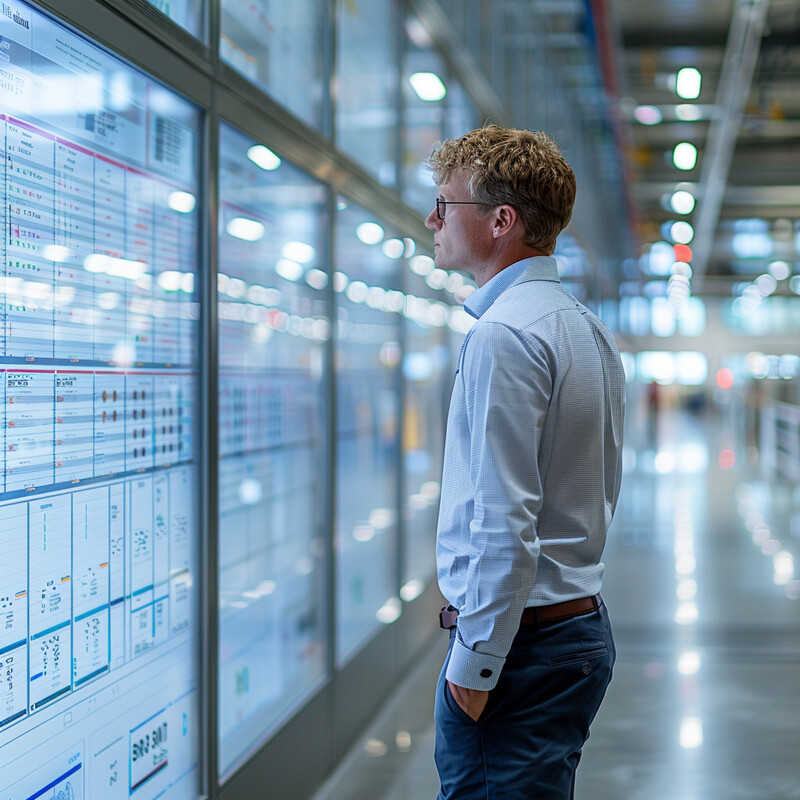
The majority of supply chain organizations are leveraging AI for end-to-end visibility. In 2024, roughly 68% of supply chain companies had implemented AI-powered traceability and real-time visibility tools, leading to an average 22% improvement in operational efficiency for those adopters. These AI systems gather data along the supply chain – scanning barcodes, tracking GPS signals, monitoring inventory sensors – and integrate it to give a live overview of operations. The result is fewer surprises: companies can see and address issues like delays or inventory shortages as they happen. The reported 22% efficiency gain comes from reductions in manual tracking effort, faster decision-making, and better coordination (for instance, warehouse teams know exactly when a delayed truck will arrive and can adjust labor accordingly). This statistic highlights that investing in AI for real-time visibility not only increases transparency but also has a tangible payoff in productivity and supply chain agility.