1. Player Performance Analysis
AI analyzes player movements and actions during games to provide detailed insights into their strengths and weaknesses, helping coaches tailor training programs.
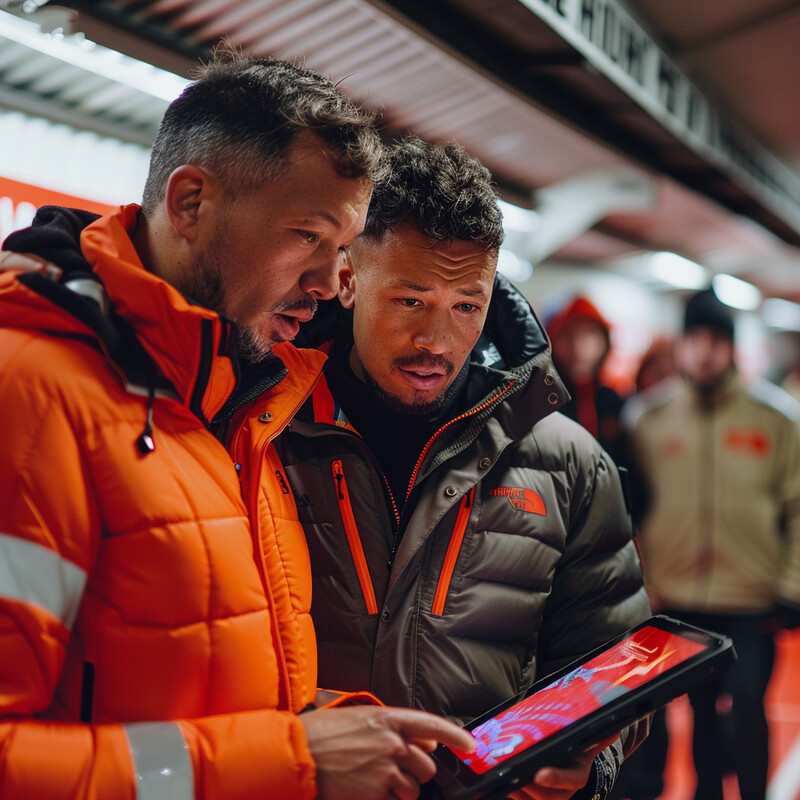
AI uses advanced algorithms to analyze video footage and sensor data from games and training sessions to evaluate player performance. This analysis includes tracking movements, assessing skill execution, and measuring physical output to identify strengths and weaknesses. The insights provided help coaches design personalized training programs that target specific areas for improvement, enhancing player performance over time.
2. Injury Prediction and Prevention
By monitoring players' health data and activity levels, AI can predict the risk of injuries and suggest preventative measures to minimize downtime.
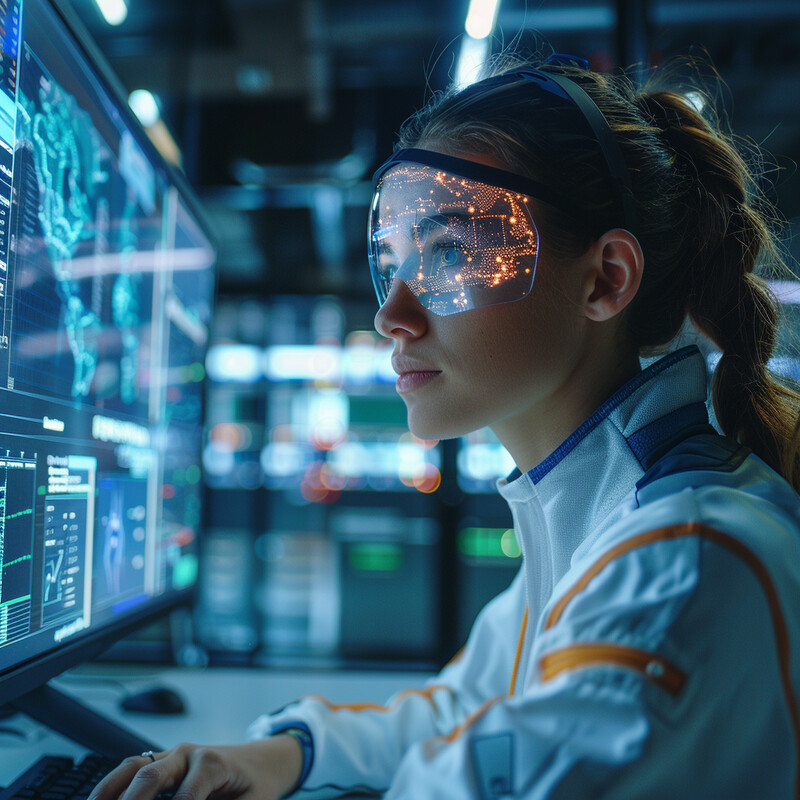
AI models predict potential injuries by analyzing data from wearable devices that monitor athletes' physical activities, stress levels, and fatigue. These models identify patterns that precede common injuries, allowing for preemptive interventions such as adjusting training loads or focusing on specific physical therapies, thereby reducing the risk of severe injuries and ensuring athletes maintain peak condition.
3. Game Strategy Development
AI models simulate different game strategies and outcomes based on historical performance data, enabling coaches to devise more effective game plans.
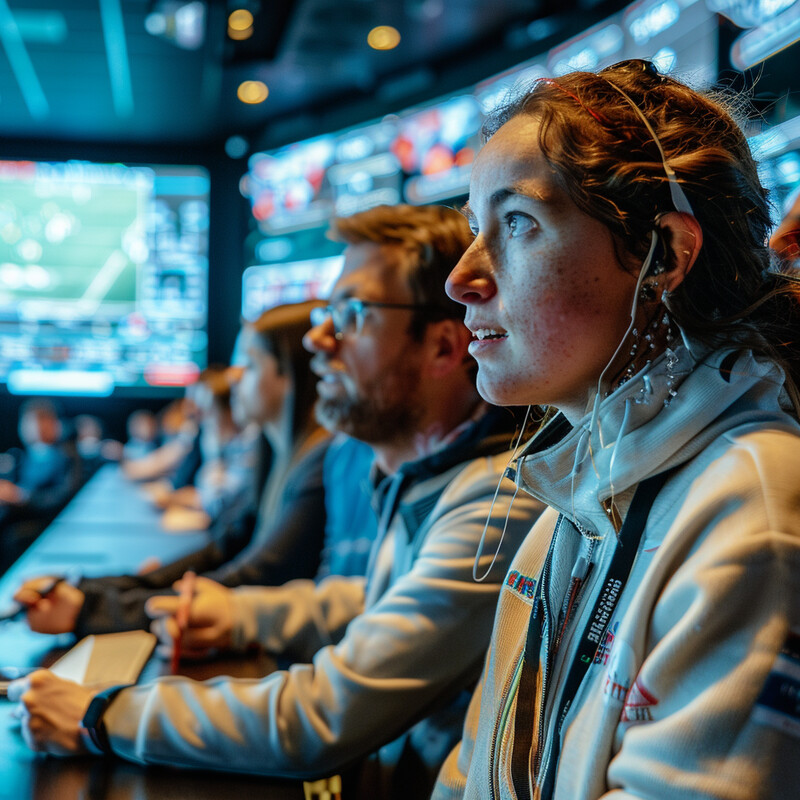
Using historical game data, AI simulates various game scenarios to predict outcomes based on different strategies. Coaches and analysts use these insights to refine their game plans, selecting strategies that maximize their team's strengths and exploit opponents' weaknesses. This strategic planning is crucial for preparing teams for critical matches and can significantly impact game outcomes.
4. Real-time Tactical Decisions
During games, AI can offer real-time tactical advice by analyzing the current state of play and comparing it with past games to suggest optimal tactical moves.
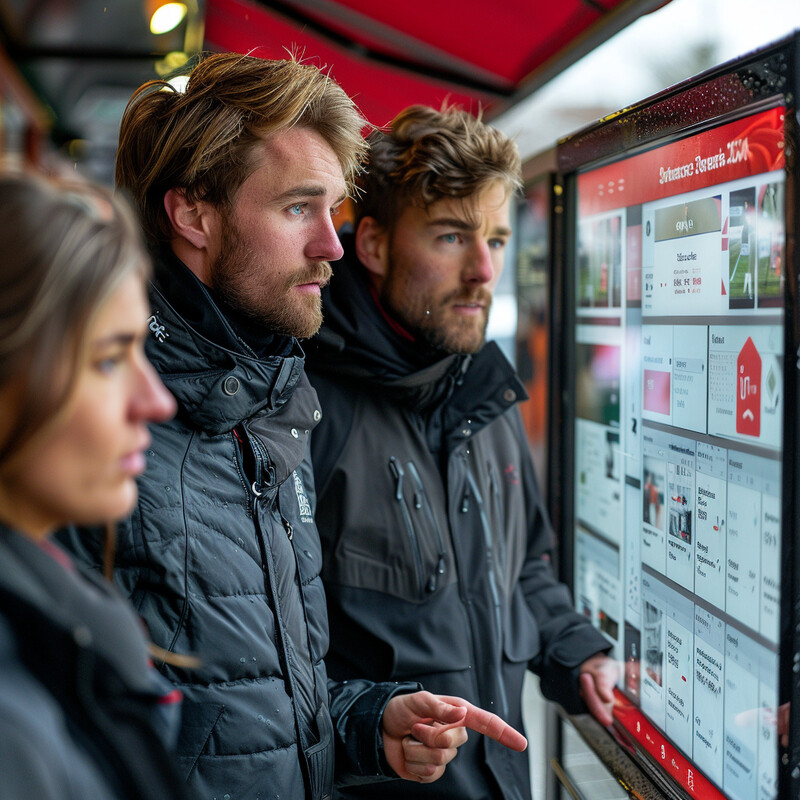
During live games, AI systems analyze the flow of play and provide real-time recommendations to coaches. These suggestions are based on the current game dynamics and statistical models developed from previous games, enabling coaches to make tactical adjustments on the fly, such as changing formations or player assignments to better counter the opponent's strategies.
5. Recruitment and Scouting
AI automates the analysis of potential recruits by evaluating their past performance data across various parameters, making the scouting process more efficient and objective.
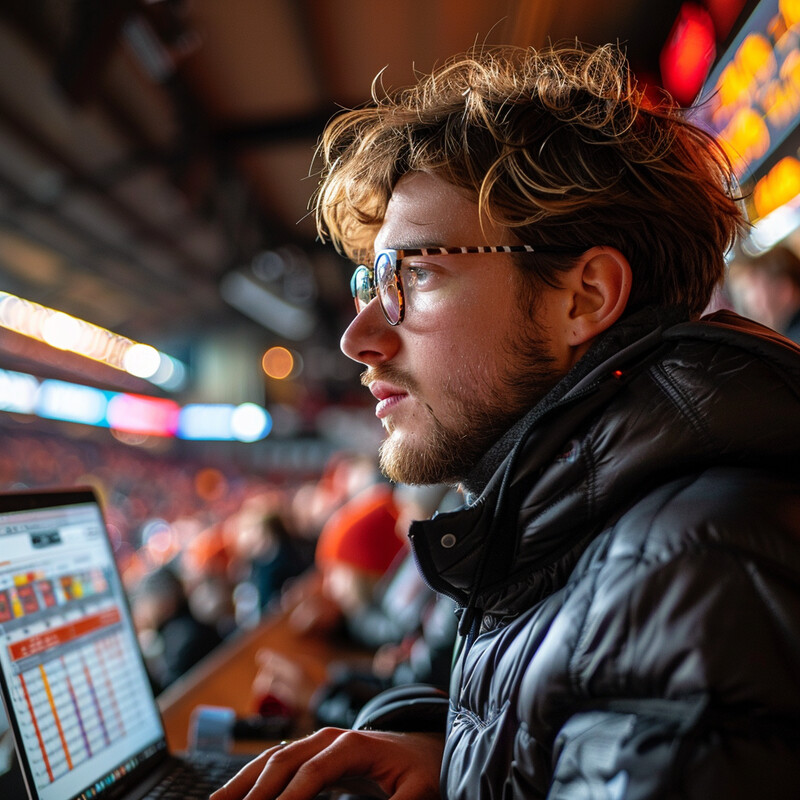
AI streamlines the scouting process by automatically evaluating vast amounts of data on potential recruits, including performance statistics, health records, and even psychometric data. This automation allows teams to quickly identify and assess talent that fits their strategic needs and cultural environment, making the recruitment process more efficient and data-driven.
6. Fan Engagement
AI enhances fan experiences by providing personalized content, predictive game analytics, and interactive platforms that engage fans before, during, and after the sports events.
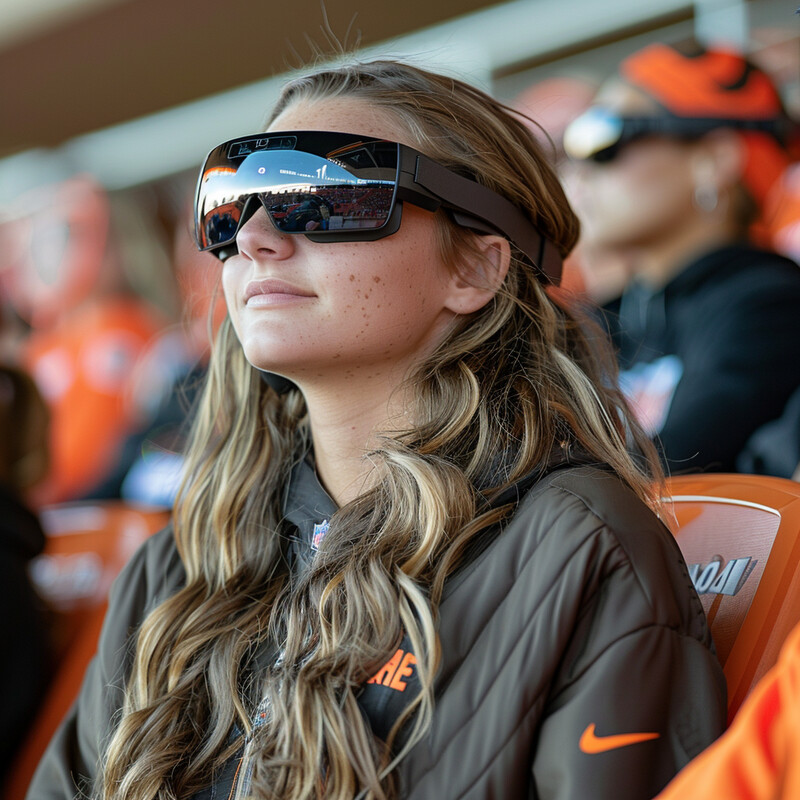
AI enhances the fan experience by personalizing content delivery based on individual preferences and behaviors. AI algorithms analyze fan data to tailor marketing messages, recommend merchandise, and even predict game outcomes, thereby increasing engagement and loyalty. Interactive AI features, such as chatbots or augmented reality apps, also enhance the game-day experience for fans.
7. Wearable Technology Integration
AI integrates with wearable technologies to track and analyze athletes' biometric and physiological data during training and matches, providing insights into their fitness levels and performance capabilities.
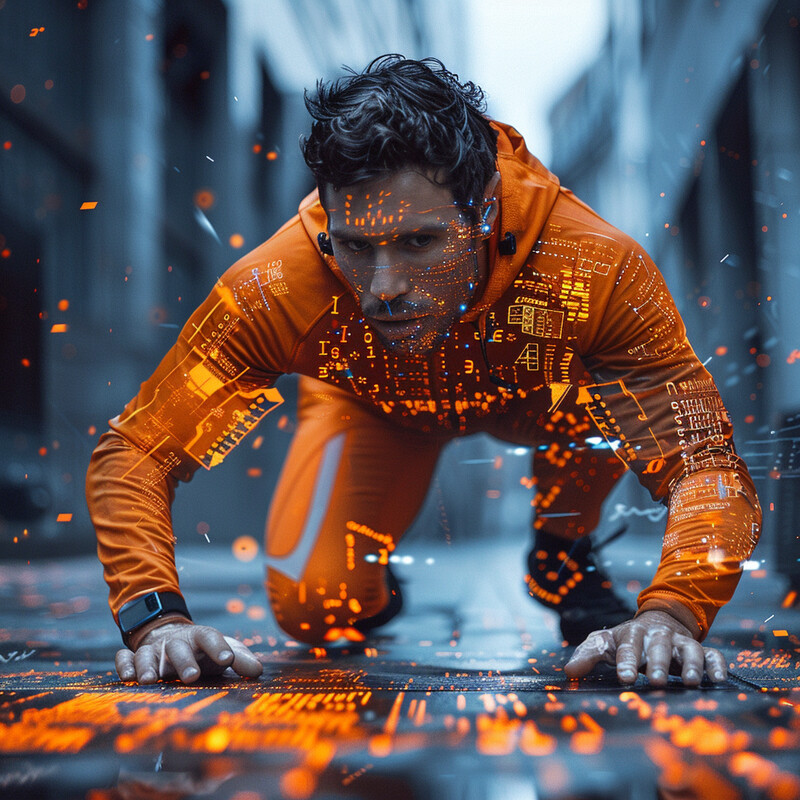
AI integrates with wearable technology to provide detailed analytics on athletes' physiological and biomechanical data during training and competition. This integration allows for precise monitoring of health metrics such as heart rate, oxygen levels, and muscle activity, which coaches and medical staff use to optimize performance and prevent overtraining.
8. Revenue Optimization
AI analyzes ticket sales, merchandise purchases, and viewership data to optimize pricing and marketing strategies for maximizing revenue.
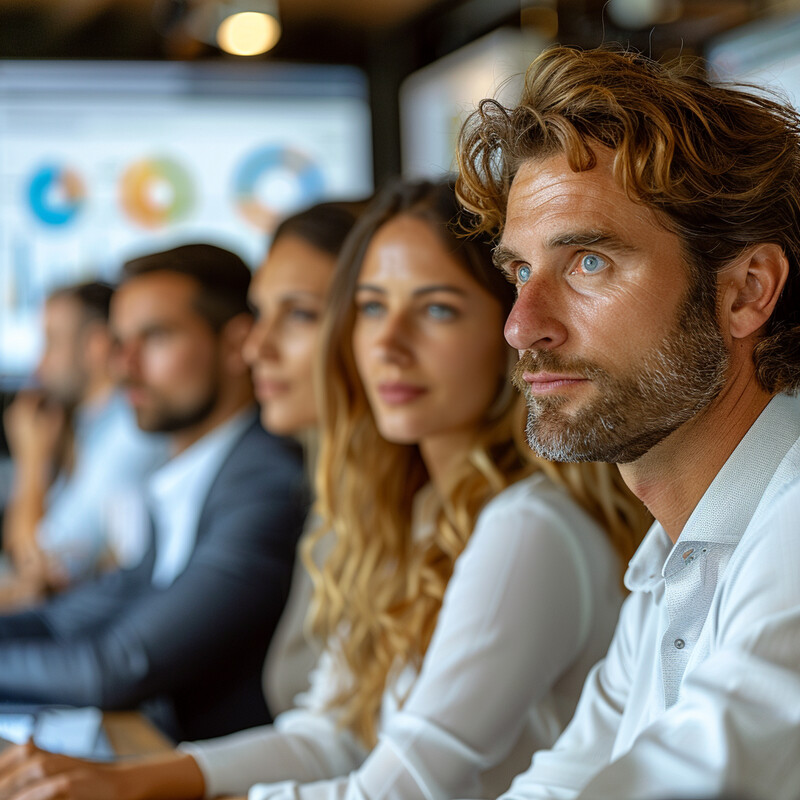
AI analyzes historical data on ticket sales, viewership, and merchandise purchases to optimize pricing strategies and marketing campaigns. By predicting fan behavior and preferences, AI helps sports organizations maximize revenue through dynamic pricing, targeted advertising, and strategic promotions.
9. Training Simulations and Virtual Reality
AI-powered simulations and VR environments allow athletes to practice in virtual settings that mimic real-game situations without the physical strain or risk of injury.
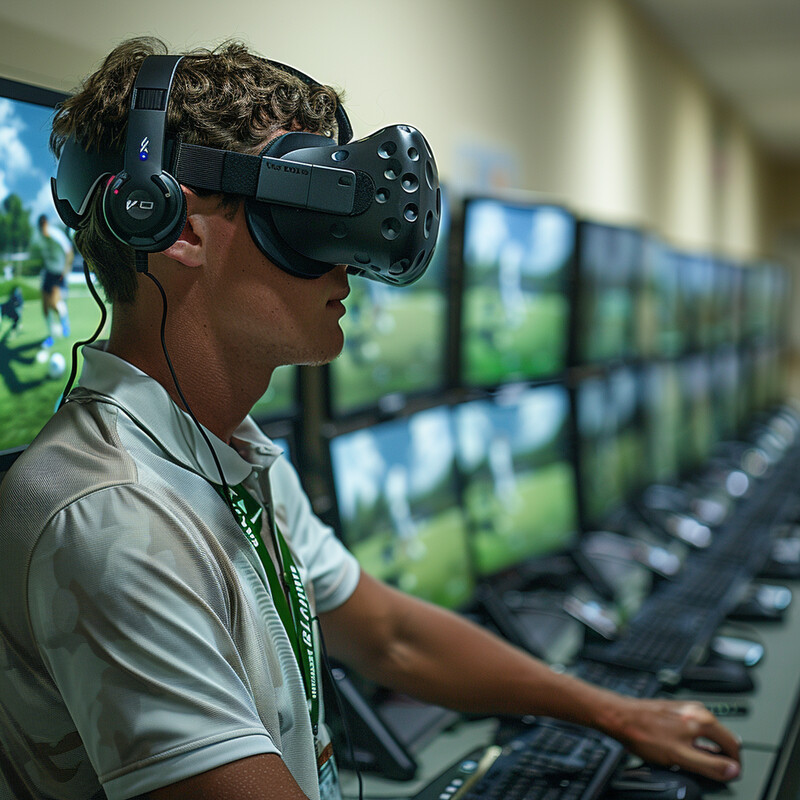
AI-powered virtual reality (VR) and simulation tools allow athletes to practice in immersive, controlled environments that replicate real-game conditions without the physical risks. These tools enable players to refine techniques and strategies in a virtual setting, providing valuable practice that is both effective and safe.
10. Competitive Analysis
AI tools analyze data from competitors to highlight trends, tactics, and performance metrics, giving teams a strategic advantage by understanding their opponents better.
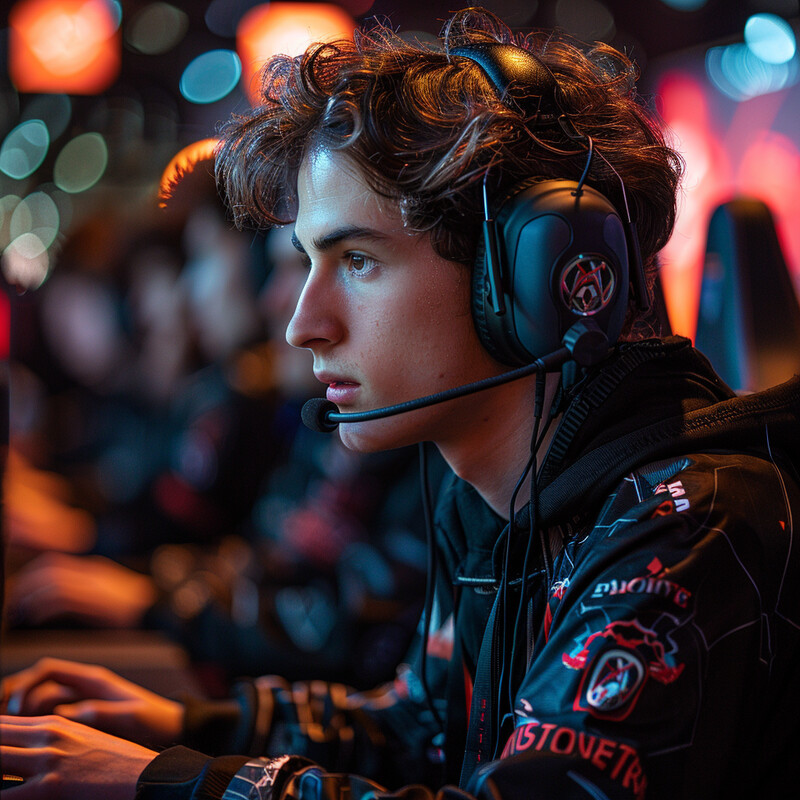
AI tools provide comprehensive analysis of competitors by aggregating data from various sources, including game footage, player statistics, and historical performance trends. This analysis helps teams gain insights into their opponents’ tactics, strengths, and potential weaknesses, offering a competitive edge in preparation and during actual gameplay.