1. Sleep Stage Classification
Artificial intelligence algorithms can accurately distinguish different sleep stages (such as REM, light, and deep sleep) by analyzing data from wearables and sensors. This automated stage scoring provides a detailed picture of a person’s sleep architecture without the need for in-lab polysomnography. By identifying when someone is in REM vs. non-REM stages throughout the night, AI offers insights into sleep continuity and structure that are critical for evaluating sleep quality. These tools can operate on data from smartwatches, fitness bands, or mattress sensors, translating movements, heart rate, and other signals into sleep stage estimates. As AI models improve, their sleep stage classifications are approaching the reliability of human experts, making at-home sleep tracking more informative and actionable.
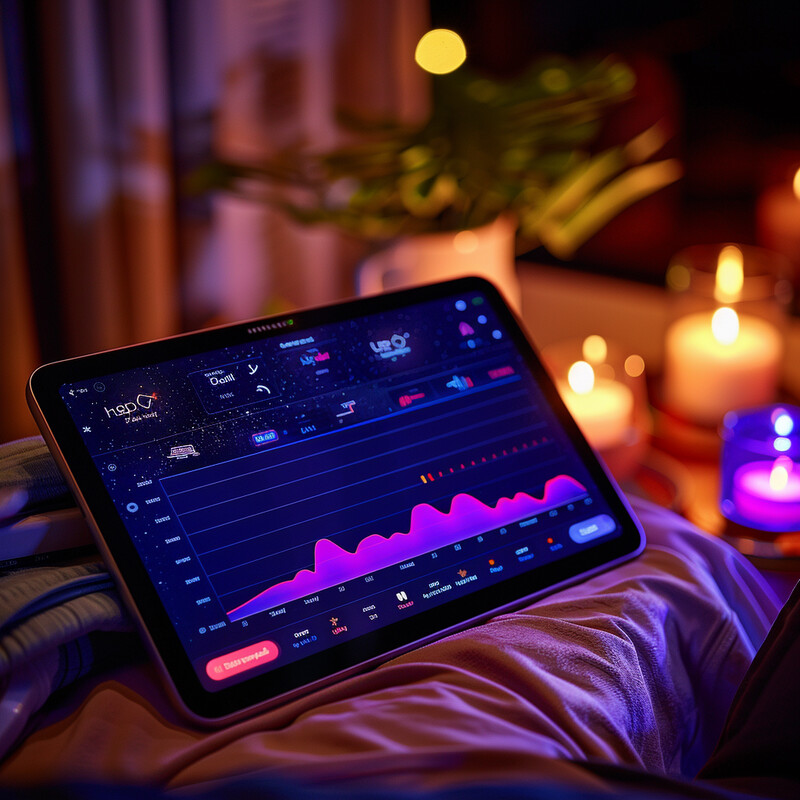
In research settings, AI-driven sleep stage classifiers have achieved performance near clinical standards. For example, a 2024 study introduced a deep learning model that reached about 87% accuracy in automatically classifying sleep stages from a single EEG channel, a level comparable to manual scoring. The same AI model showed substantial agreement with human sleep technicians (kappa ≈ 0.70), indicating its stage decisions closely matched expert judgments. Other work with consumer wearables also reports decent accuracy – typically around 70–80% agreement – in differentiating sleep stages when compared to gold-standard lab sleep studies. This means modern AI algorithms can reliably detect whether a person is in REM, light, or deep sleep most of the time. While not perfect, these systems are continually improving and have become valuable for large-scale sleep monitoring outside of clinical settings.
2. Sleep Quality Assessment
AI is now widely used to evaluate overall sleep quality by crunching multiple sleep parameters into a composite assessment. Instead of relying solely on one measure (like total sleep hours), AI algorithms consider factors such as sleep duration, sleep efficiency (time asleep vs. time in bed), frequency of awakenings, and time spent in each stage. By analyzing these inputs, AI can generate a nightly “sleep score” or detailed report highlighting how restorative a sleep episode was. These personalized sleep quality metrics help users identify patterns – for instance, if they are consistently experiencing fragmented sleep or insufficient deep sleep. Over time, AI-driven feedback on sleep quality can guide individuals toward better habits (like adjusting bedtime or reducing disruptions), as it pinpoints specific areas for improvement rather than giving generic advice.
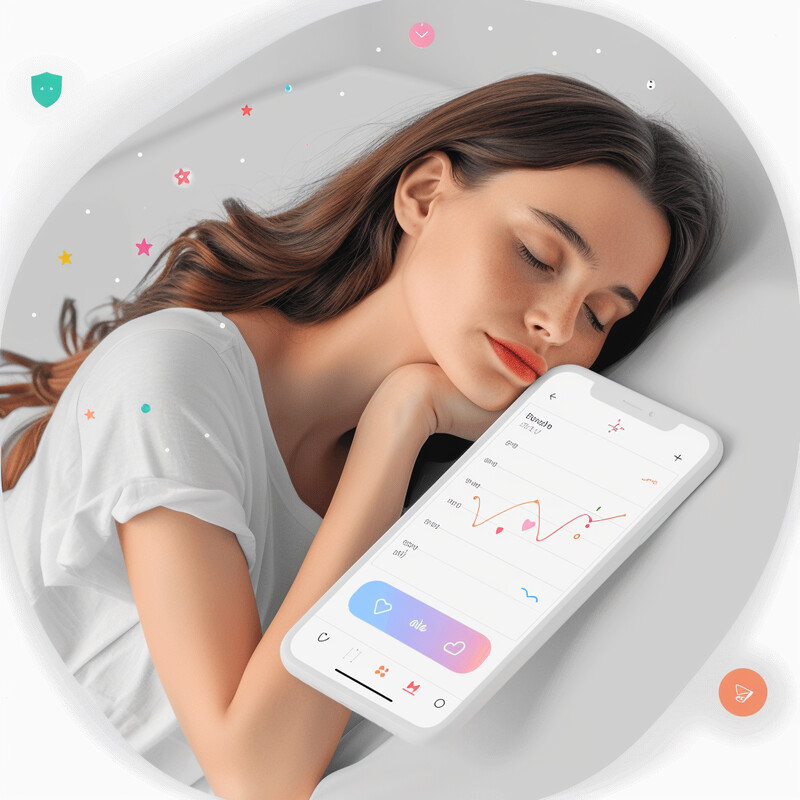
Millions of people are already using AI-based sleep trackers to monitor and improve their sleep quality, and many find them beneficial. According to a 2023 survey by the American Academy of Sleep Medicine, more than one-third of Americans (35%) have tried an electronic sleep-tracking device, and 77% of those users reported the tracker was helpful in understanding their sleep patterns. Importantly, 68% of people who used sleep trackers said they changed their behavior based on the insights provided. These trackers, which use AI to rate sleep quality each night, often motivate users to adjust their routines (for example, improving bedtime consistency or reducing caffeine late in the day). Sleep researchers note that such detailed feedback makes users more aware of sleep’s importance, and early studies suggest that engaging with these AI-driven reports can indeed lead to measurable improvements in sleep hygiene and satisfaction.
3. Detection of Sleep Disorders
AI is becoming a powerful tool for detecting signs of sleep disorders by flagging abnormal patterns in sleep data that might be missed in casual observation. By continuously monitoring parameters like breathing, movement, heart rate, and blood oxygen levels, machine learning models can learn the “fingerprints” of disorders such as obstructive sleep apnea (pauses in breathing), insomnia (difficulty falling or staying asleep), or restless legs syndrome (frequent limb movements). For instance, an AI can analyze overnight pulse and oxygen trends from a smartwatch and alert the user to patterns characteristic of sleep apnea (like repeated drops in oxygen saturation during the night). Similarly, unusual sleep fragmentation or insufficient deep sleep might prompt an insomnia risk alert. Early identification is key – catching these warning signs through AI can lead individuals to seek formal medical evaluation sooner. As a result, AI-based screening has the potential to facilitate earlier diagnosis and treatment of sleep disorders, even in people who don’t realize they have a problem.
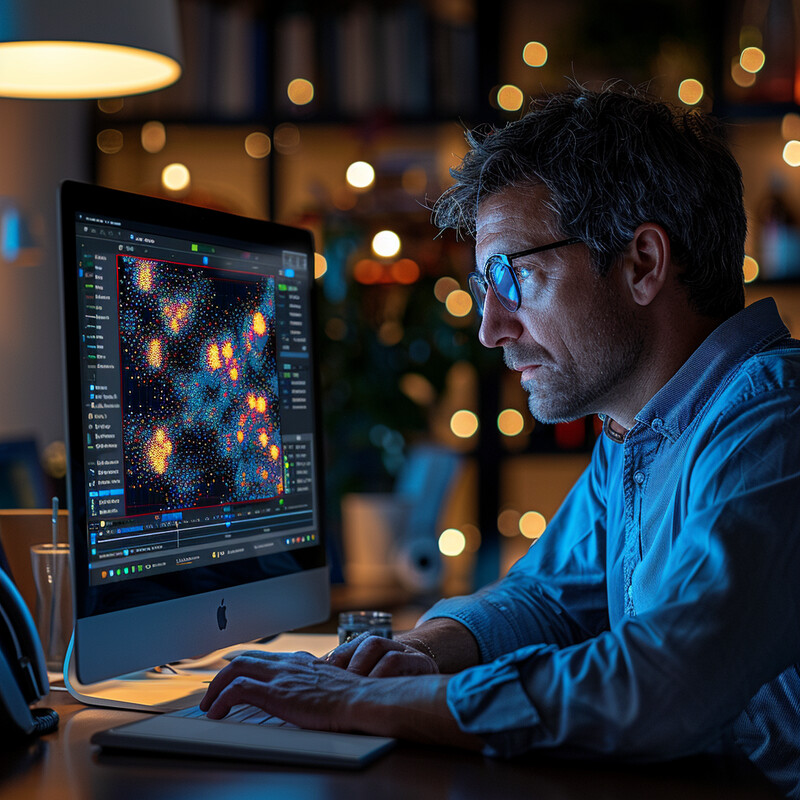
Studies show that wearable AI systems can detect common sleep disorders with promising accuracy, though there is room for improvement before they replace medical diagnostics. A recent systematic review in 2024 pooled data from dozens of studies and found that AI algorithms in wearables could identify sleep apnea with about 87% accuracy (pooled accuracy = 0.869). In detecting breathing cessation events characteristic of apnea, these AI tools demonstrated high sensitivity (~94%) – meaning they catch most apnea events – but more moderate specificity (~75%), indicating some false alarms are still occurring. The review concluded that “wearable AI shows potential in identifying and classifying sleep apnea, but its current performance is suboptimal for routine clinical use” In practice, this means an AI-equipped device on your wrist can often recognize apnea-related disruptions (like pauses in breathing or drops in blood oxygen) and alert you. However, doctors currently advise confirming any positive findings with traditional tests, since consumer AI might err on the side of caution and overestimate problems. Ongoing research is rapidly improving these algorithms, extending similar methods to insomnia and movement disorders, with the hope that AI can serve as an at-home early warning system for a range of sleep disorders.
4. Personalized Sleep Recommendations
AI can deliver highly personalized sleep improvement recommendations by learning an individual’s specific patterns and needs. Using data from nightly sleep logs and even daytime behaviors, AI systems act like a virtual sleep coach – suggesting practical changes tailored to the user. For example, if the AI notices you fall asleep faster on days you exercise, it may recommend moderate evening exercise on specific days. If it sees your sleep is restless when you have late caffeine or screen time, it will flag those as areas to adjust. These recommendations can range from an ideal bedtime and wake time window (to match your circadian rhythm) to adjustments in bedroom environment (darker room, cooler temperature) or relaxation techniques before bed. What makes it “personalized” is that the AI’s advice evolves as your data changes: it will refine tips as it learns what works best for you. This one-on-one style guidance aims to improve sleep hygiene in a way that fits seamlessly into each person’s lifestyle, much like a human sleep specialist would do in creating a custom plan.
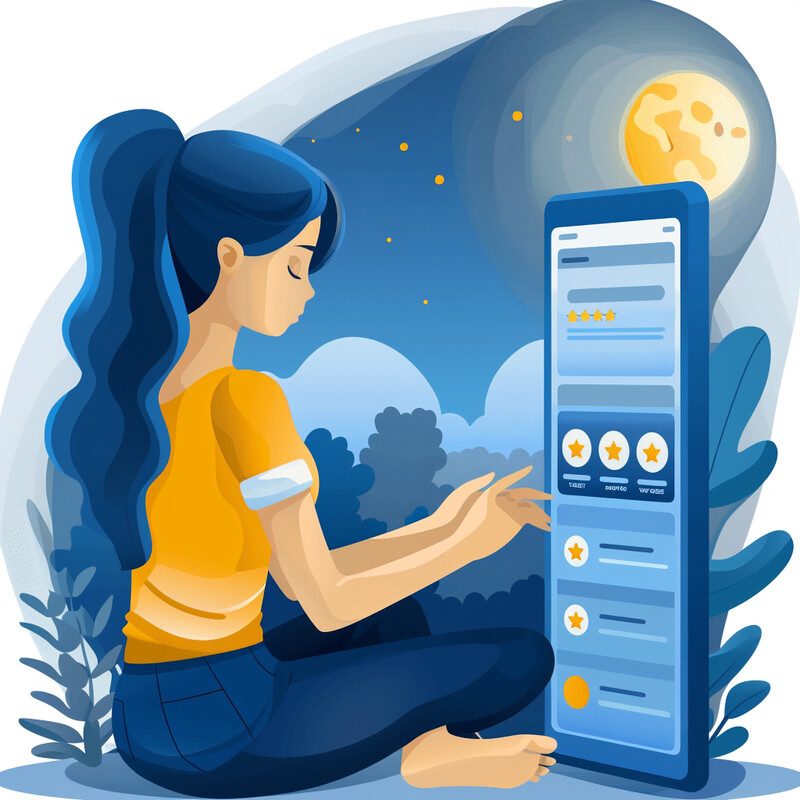
We’re already seeing AI-driven sleep coaches in action – for instance, a major mattress company launched Emma Up in 2023, an app that uses artificial intelligence to provide individualized sleep coaching. The AI coach in this app creates “hyper-personal, step-by-step programs” for improving sleep, meaning it gives each user specific daily guidance rather than one-size-fits-all tips. As users log their sleep and follow the advice, the AI adapts: the company notes that “the AI coach learns from each of their individual experiences,” enabling it to deliver increasingly precise and bespoke guidance, similar to how a human coach would refine advice over time. For example, Emma Up might suggest an earlier bedtime and reduced screen exposure if it detects a user’s late-night phone use correlates with poorer sleep. If the user’s data later shows improvement, the AI will reinforce those successful strategies; if not, it pivots to new recommendations. Early feedback indicates that such AI coaches can effectively help users incorporate healthier sleep habits into their routines by keeping them engaged with continuous, tailored support. Sleep technology experts anticipate that these kinds of AI-personalized interventions will become more common, potentially improving outcomes for people struggling with sleep issues who may not have access to traditional sleep clinics.
5. Smart Alarm Systems
AI-powered smart alarm systems aim to make waking up more natural and less jarring by timing your alarm to your sleep cycle. Instead of the traditional alarm that goes off at a fixed time (which might catch you in deep sleep), these smart alarms continuously analyze your real-time sleep data – often via a wearable or phone sensor – to determine what stage of sleep you’re in. They then identify an optimal wake-up window (for example, a 30-minute window ending at your set alarm time) and choose the moment when you are in a lighter sleep stage (such as REM or light sleep) to sound the alarm. Waking from light sleep means you’re closer to being awake, so you experience less grogginess (sleep inertia) upon awakening. Many users report feeling more refreshed with this method, as opposed to being torn out of a deep sleep by a sudden alarm. Essentially, the AI serves as a gentle guardian of your morning, ensuring you wake up at a time when your brain and body are most ready, making the transition to wakefulness smoother and improving morning alertness.
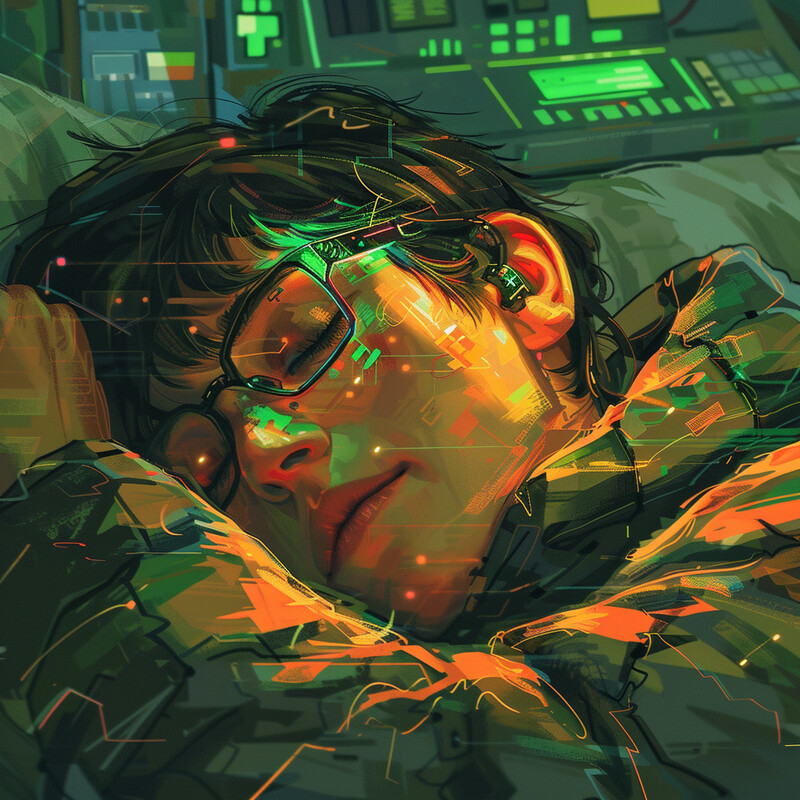
The concept behind smart alarms is backed by our understanding of sleep stages, and developers have begun to validate it with technology. Sleep specialists note that some consumer sleep trackers already time their alarms to go off during lighter sleep phases in an effort to reduce morning grogginess. For instance, a popular smartwatch app monitors a user’s movement and biometric signals to estimate if they’re in light sleep near the alarm time, and if so, it will trigger the alarm a bit earlier than the set time (when the user is lightly sleeping) rather than later (during deep sleep). Preliminary studies indicate that this approach can indeed mitigate the effects of sleep inertia. One engineering study developed a prototype smartwatch alarm that used an AI model (an encoder–decoder neural network) to predict sleep stages, achieving around 66–70% accuracy for classifying stages in real time. This smart alarm successfully avoided waking people from deep sleep by waiting for a light sleep moment to ring, and users reported feeling more alert upon awakening. While more research is ongoing, these early implementations align with what sleep science predicts – that waking up from light sleep is easier on the brain, and AI can exploit that by choosing your wake-up moment wisely.
6. Real-time Sleep Monitoring
AI enables truly smart bedrooms by monitoring your sleep in real time and automatically adjusting your environment to improve sleep quality. Through integrations with smart home devices (thermostats, lighting, adjustable beds, etc.), an AI system can respond on the fly to signs of discomfort or disturbance. For example, if the AI detects that you’re overheating (perhaps via increased heart rate or restlessness), it can signal a smart thermostat or bed cooling system to lower the temperature immediately. If it senses prolonged restlessness or light sleep, it might gently elevate the bed’s incline or change mattress firmness if the bed supports that, aiming to ease you back into deeper sleep. Some systems even use ambient noise control – detecting noise disruptions and adjusting sound machines or earbud output in response. The goal is to maintain an optimal sleep condition throughout the night without you waking up; the AI makes continuous micro-adjustments so that your sleep stays as uninterrupted and restorative as possible. This represents a shift from passive sleep tracking to active sleep enhancement, effectively giving you a personalized sleep caretaker that works while you doze.
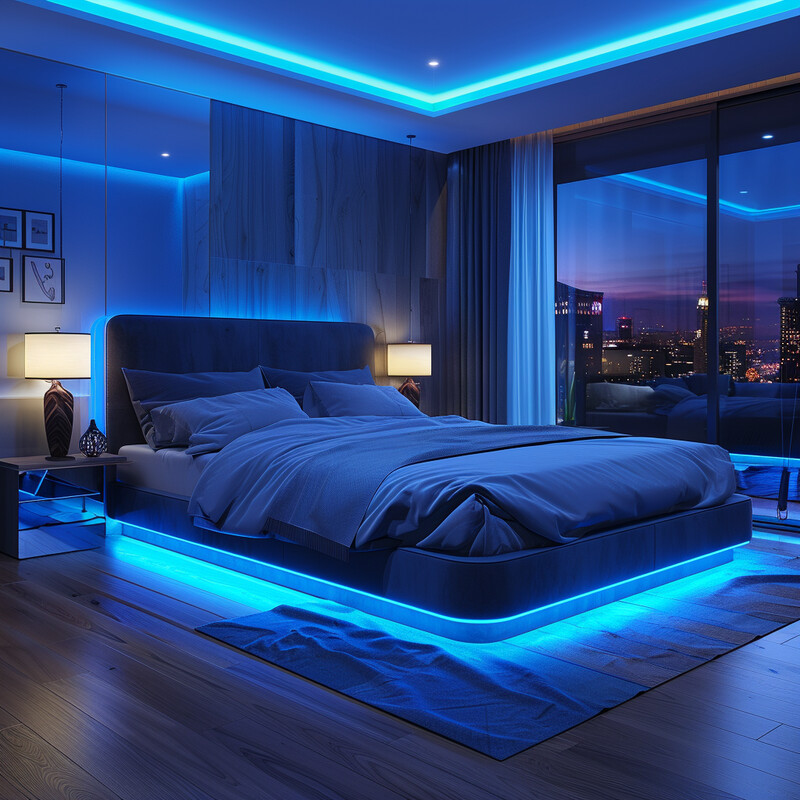
High-tech products exemplify how real-time AI adjustments can tangibly improve sleep comfort. In 2024, Sleep Number (a US smart bed company) introduced a “ClimateCool” smart bed that actively cools or warms each side of the bed throughout the night to keep the sleeper at their ideal temperature. The bed’s AI-driven system monitors the sleeper’s body temperature and adjusts cooling levels on the fly – it can cool up to 15°F on each side to counteract night sweats and then ease off if the sleeper’s temperature normalizes. These cooling programs were “developed with research from Northwestern University’s Feinberg School of Medicine” to ensure they truly improve sleep quality and aren’t just gadget gimmicks. In practice, if the sleeper enters a hot flash or the room warms up, the bed senses it and increases cooling, potentially preventing a wake-up. Likewise, smart beds and mattresses can use integrated AI to adjust firmness or elevation in response to snoring or restlessness – for instance, gently raising a snoring person’s head section to clear their airway. Early user trials and clinical studies presented at sleep conferences show that such responsive environmental control can reduce sleep disruptions and improve subjective sleep quality. As more devices (like smart pillows and lighting systems) connect to these AI hubs, we’re moving toward bedrooms that dynamically adapt to keep us sleeping soundly.
7. Longitudinal Sleep Trend Analysis
AI helps analyze long-term sleep trends by sifting through months or years of sleep data to spot patterns and changes that would be impossible for a human to manually track. By looking at the “big picture” of someone’s sleep, AI can identify gradual improvements or declines in sleep quality, seasonal variations (perhaps you sleep longer in winter than summer), and the impact of life events or interventions on sleep. For instance, an AI could correlate a period of regular exercise with deeper sleep over subsequent weeks, or notice that your sleep efficiency deteriorated when you started a new job with earlier hours. On a personal level, this trend analysis gives users actionable insights – they can objectively see how their sleep evolves and link it to changes in routine or health. On a population level, analyzing longitudinal sleep data from thousands of users allows researchers to establish norms and understand how sleep patterns vary across different ages, genders, or lifestyles. In both cases, AI’s ability to handle and learn from vast datasets means that we can move beyond nightly snapshots and truly understand sleep as a dynamic process over time.
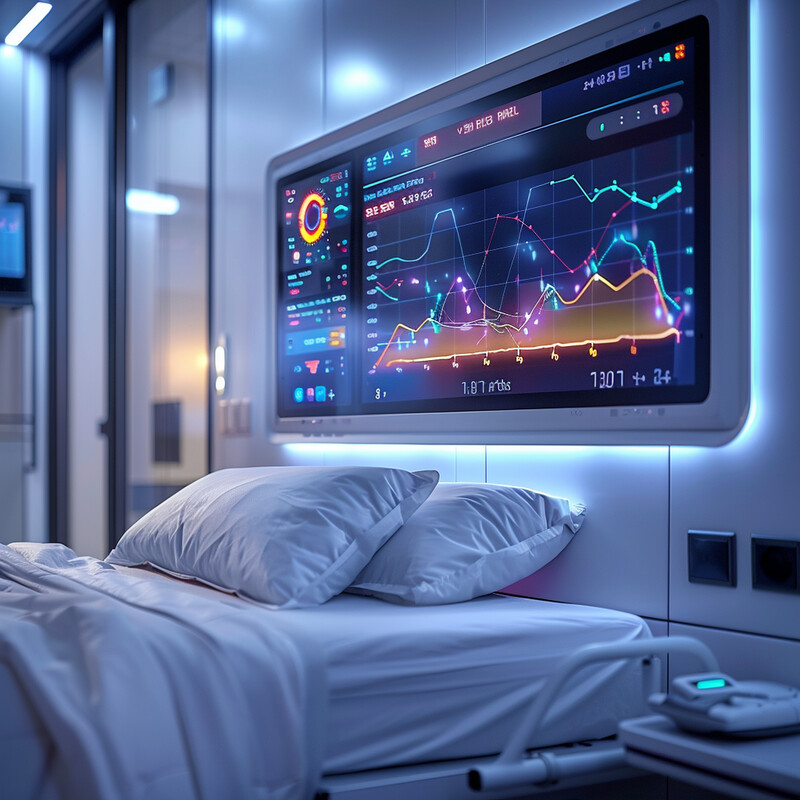
Large-scale analyses underscore how stable yet distinctive individual sleep patterns can be when viewed over long periods. A 2024 study examined over 6.4 million sleep nights recorded by Fitbit devices from about 6,785 adults (part of a national research program) to chart long-term sleep habits. It found the median sleep duration was approximately 6.7 hours per night, and this average remained remarkably consistent from 2017 through 2022 across the studied population. In other words, on a population level, people’s sleep duration didn’t dramatically change year-to-year during those five years. However, by using AI to slice the data, researchers noted significant differences in sleep patterns when broken down by demographics and lifestyle. For example, women on average slept slightly longer than men, and individuals who never smoked had different sleep profiles (in terms of quality and duration) than those who smoke or drink heavily. These insights were only possible by aggregating and analyzing multi-year data via machine learning models. Similarly, for an individual user, a sleep-tracking app’s AI might highlight, say, “Over the past 3 months, your average nightly sleep increased by 30 minutes once you started your new exercise routine,” validating the benefit of that lifestyle change. This long-term perspective is invaluable – it enables both scientists and everyday users to see beyond night-to-night variability and understand real trends and drivers in sleep health.
8. Integration with Health Management
AI is integrating sleep metrics with other health data to provide a truly holistic view of an individual’s well-being. Instead of viewing sleep in isolation, modern health platforms use AI to combine sleep information with exercise, nutrition, stress levels, and medical data. This integrated approach recognizes that sleep influences – and is influenced by – many other aspects of health. For example, an AI health app might notice that on days when a user’s stress (measured by heart rate variability) is high, their sleep quality that night is poor, prompting suggestions for relaxation techniques. It may also tie consistent short sleep duration to weight gain or higher blood pressure readings over time, alerting the user and their healthcare providers to a potential risk. Some electronic health record systems are incorporating sleep data so that doctors can see a patient’s sleep alongside blood glucose trends or medication schedules. By analyzing these interconnected data streams, AI can identify patterns such as “poor sleep and low physical activity together predict higher risk of mood issues” or that improving sleep could help improve blood sugar control in diabetics. Ultimately, integrating sleep data into the larger health puzzle allows AI to make personalized lifestyle recommendations – like adjusting workout intensity or meal timing to improve sleep – and to flag health conditions where sleep might be a contributing factor.
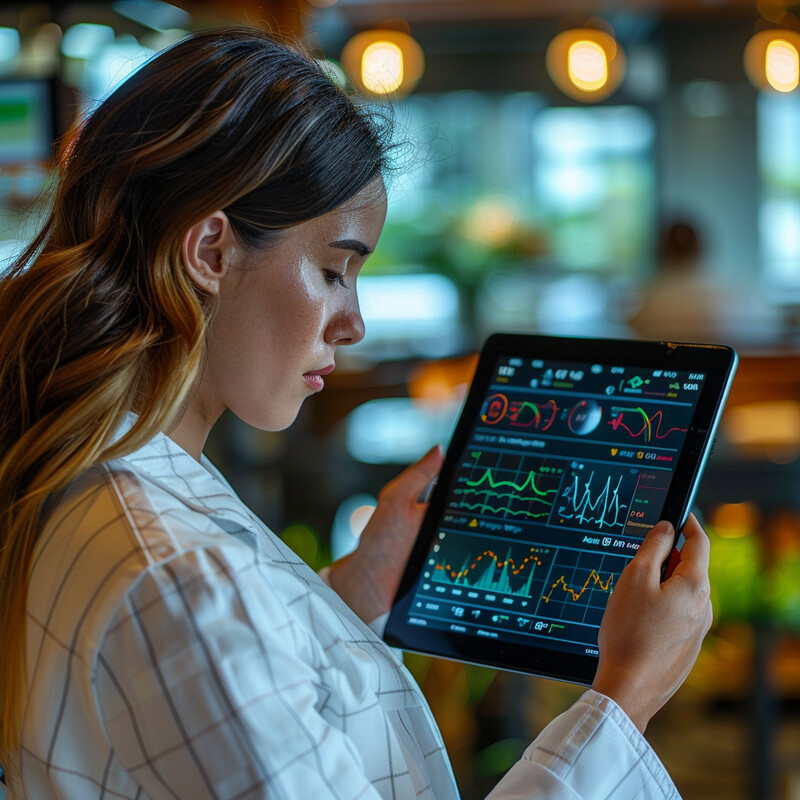
Major health organizations are emphasizing the integration of sleep with overall health, underscoring how critical this link is. In 2022, the American Heart Association updated its cardiovascular health checklist (formerly known as “Life’s Simple 7”) to add healthy sleep as the eighth essential component for heart and brain health. This initiative, called Life’s Essential 8, puts getting 7–9 hours of sleep per night on the same level of importance as diet, exercise, cholesterol, blood pressure, blood sugar, weight, and avoiding smoking. The inclusion was driven by extensive research showing that insufficient or poor-quality sleep elevates the risk of hypertension, obesity, type 2 diabetes, and other heart-related problems. For instance, chronic short sleep has been linked to higher incidence of coronary artery disease and stroke. By formally recognizing sleep in the core health metric, the AHA encourages clinicians and patients to discuss and manage sleep just as they would other vital signs of health. This kind of integration is increasingly powered by AI in practice: many wellness apps now compile a “health score” that incorporates sleep duration and quality as key inputs alongside physical activity and diet. Some predictive models in use can take a person’s combined data – say, a stretch of poor sleep plus elevated resting heart rate and blood pressure – and forecast an increased risk of illness, prompting preventive action. In short, both the medical community and AI-driven tools are treating sleep as an integral part of the whole health picture, not an isolated silo.
9. Interactive Sleep Education
AI is powering interactive educational platforms that teach people about sleep in a personalized and engaging way. Traditional sleep education (pamphlets, generic advice articles) can be one-size-fits-all, but AI allows the content to adapt to the user. For example, chatbots and virtual assistants can answer questions about sleep hygiene or explain scientific concepts (like what REM sleep does) in simple terms, available 24/7. These tools often use a person’s own data to illustrate lessons – for instance, showing a user their sleep stages graph and explaining how REM sleep helps memory, based on the user’s actual REM amounts. Gamified apps might quiz users on sleep facts and use AI to focus on topics the user seems less aware of. Some programs even simulate an “interactive sleep doctor,” where users can describe their sleep issues in a chat and the AI provides tailored education and tips in response. Because the AI can process natural language, users can essentially have a conversation (“Why do I feel groggy after 8 hours of sleep?”) and get research-backed answers instantly. This dynamic, dialogue-based learning helps dispel myths (like “I can catch up on weekends” or “older adults need far less sleep”) and reinforce healthy behaviors. Overall, AI-driven interactive education empowers users with knowledge about sleep’s importance and how to improve it, in a way that is accessible and customized to their needs.
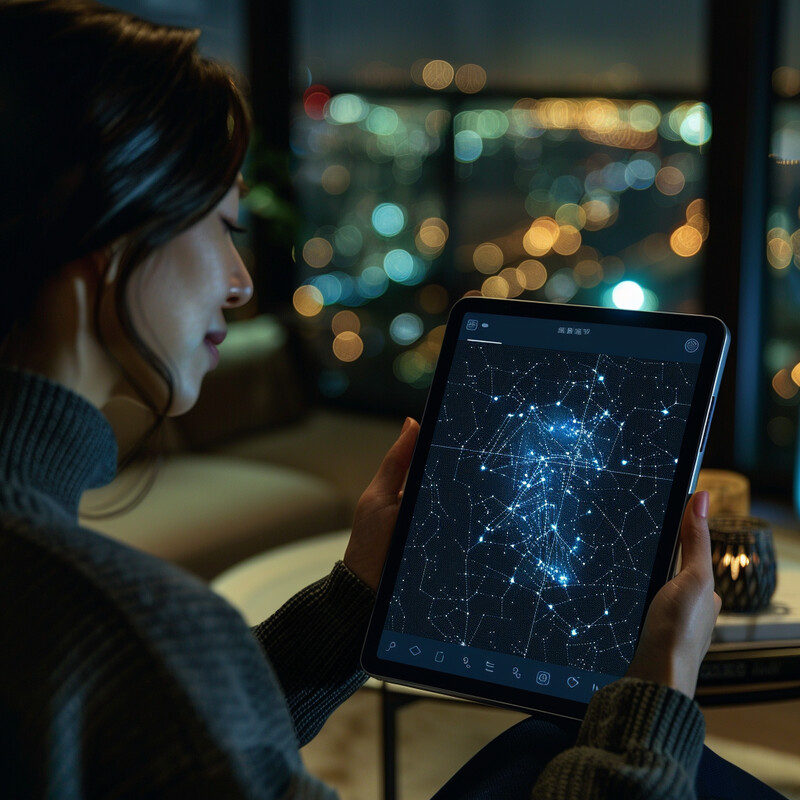
Advanced conversational AIs have demonstrated an impressive ability to educate users about sleep accurately. In a 2024 study, researchers tested ChatGPT-4 (a state-of-the-art generative AI) on its knowledge of common sleep myths and sleep health information. The findings showed that ChatGPT could “accurately address sleep-related queries and debunk sleep-related myths, with a performance comparable to sleep experts.”. In the study, sleep physicians provided a list of 20 widespread myths (for example, “you can train yourself to need only 4 hours of sleep”) and the AI was tasked with evaluating and correcting them. ChatGPT-4 correctly labeled 85% of the myths as false and provided explanations that were largely in line with expert answers. Its responses were noted to be in an accessible style, translating technical jargon into user-friendly language. This suggests that AI chatbots can serve as effective virtual sleep educators – they not only convey accurate information (as validated by sleep specialists) but do so in a way laypeople can easily understand. Moreover, these AI tools can personalize the interaction: if a user’s question is about why they wake at 3 AM, the AI can tailor the explanation to that scenario and suggest specific strategies. Such interactive AI systems are already being incorporated into sleep apps and websites, guiding users through lessons on topics like sleep stages, insomnia prevention, and relaxation techniques. As long as they are kept up-to-date with verified medical knowledge, AI-based educators could significantly broaden public access to quality sleep education.
10. Research and Development
AI is accelerating research and development in the field of sleep science by handling “big data” and uncovering insights that would be very hard to find otherwise. Modern sleep studies often involve enormous datasets – years of nightly recordings, or genetic and biometric data from tens of thousands of people – and AI algorithms can analyze these efficiently to spot subtle patterns. For example, machine learning can comb through thousands of full-night EEG recordings to detect a previously unknown biomarker (a specific brain-wave pattern) that predicts a certain sleep disorder. AI is also being used to model and simulate sleep processes, helping scientists test hypotheses (like how a certain drug affects sleep architecture) in silico before doing clinical trials. In terms of developing new treatments, AI can identify which patients are likely to respond to a given therapy by recognizing patterns in their sleep data. It can also suggest novel therapeutic targets; for instance, if AI finds that people with a particular gene variant have disrupted sleep cycles, that gene pathway might become a target for new medication. Additionally, AI-driven analysis of sleep and health datasets is revealing how sleep interacts with other conditions (e.g. how sleep patterns might predict onset of Alzheimer’s disease, or recovery after heart surgery), guiding researchers toward integrated care approaches. Overall, AI acts as a catalyst in sleep R&D – processing data at superhuman scales and speed, generating new hypotheses, and even powering the next generation of smart sleep technologies (like brain stimulation devices to enhance deep sleep). The end result is a faster pipeline from discovery to real-world sleep solutions.
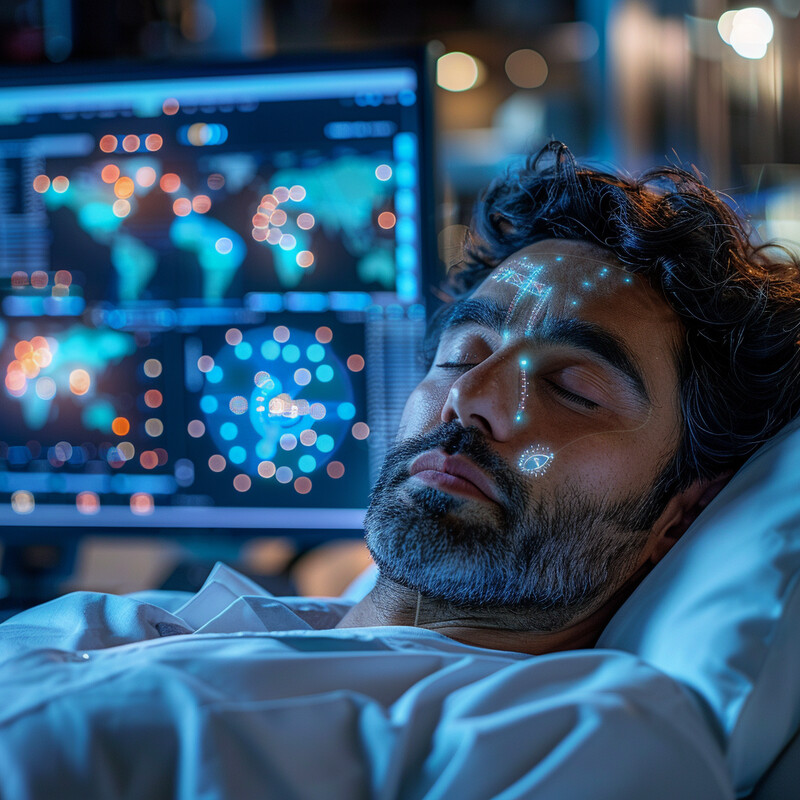
Thanks to AI-based data mining, scientists are making discoveries about sleep’s impact on health that were not evident before. One example comes from the 2024 analysis of the All of Us research dataset, where AI examined relationships between detailed sleep metrics and future health outcomes in thousands of individuals. The researchers found a notable link between diminished REM/deep sleep and cardiovascular issues – specifically, an inverse association between the amount of deep/REM sleep and the likelihood of developing atrial fibrillation (a heart rhythm disorder). In simpler terms, people who consistently got less REM and deep sleep were more prone to later experience atrial fibrillation, suggesting that those sleep stages may have a protective effect on heart rhythm. Additionally, the AI uncovered that irregular sleep patterns (high night-to-night variability in sleep duration) were associated with higher risks of conditions like obesity, hypertension, and depression. These kinds of findings broaden our understanding of how sleep quality can be a predictor or contributor to various chronic diseases. They also open up new research questions – for instance, could improving someone’s REM sleep reduce their atrial fibrillation risk? Such hypotheses can now be tested in clinical trials. Beyond epidemiology, AI is also accelerating R&D in drug discovery for sleep disorders: algorithms are screening thousands of compounds to predict which might enhance specific sleep phases or normalize circadian rhythms, dramatically narrowing down candidates for laboratory testing. In sum, AI is not only confirming known links (like “sleep is important for health”) but quantifying and detailing those links in actionable ways, thereby driving the development of targeted interventions and innovative therapies in sleep medicine.