1. Enhanced Natural Language Understanding (NLU)
AI models better understand the nuances of language, including slang, idioms, and cultural variations, improving the accuracy of sentiment interpretation.
-1.jpg)
AI-powered Natural Language Understanding (NLU) systems delve deeper into language complexities, interpreting nuances such as idioms, colloquialisms, and even regional dialects with greater accuracy. These systems can distinguish subtle differences in sentiment that traditional keyword-based analyses might miss, ensuring a more accurate representation of people's true feelings and opinions expressed in text.
2. Contextual Analysis
AI can consider the broader context of conversations or texts, recognizing how the sentiment might shift based on surrounding content or the overall topic.
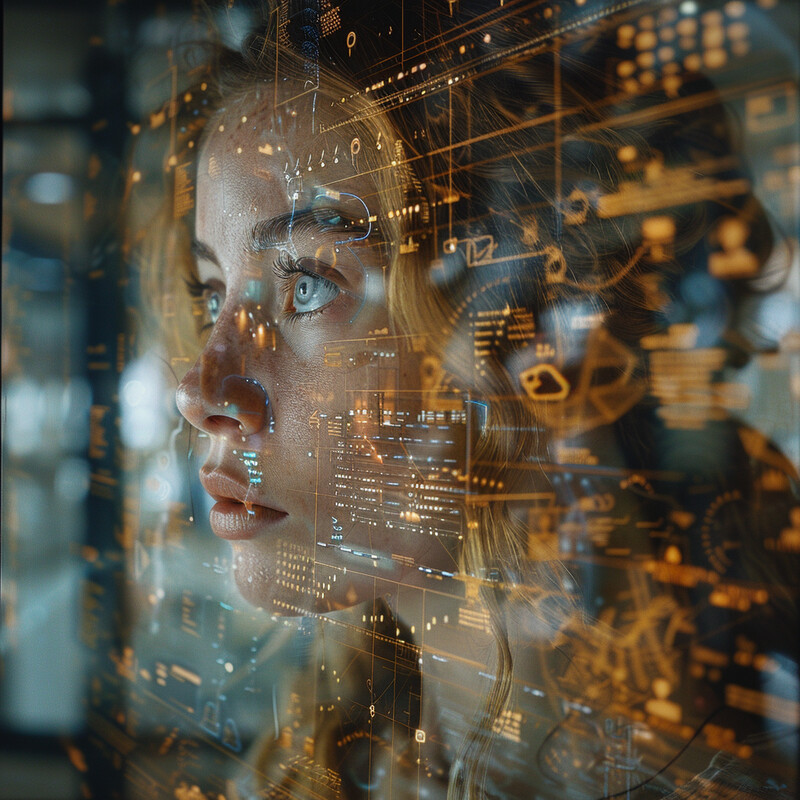
AI improves sentiment analysis by taking into account the broader context in which words or phrases are used. It understands that the sentiment expressed in a sentence can depend heavily on the surrounding text and the overarching subject matter. This capability allows AI to accurately capture the intended emotions and opinions, even in complex scenarios where sentiments are not explicitly stated but implied by context.
3. Real-time Sentiment Tracking
AI enables the real-time analysis of sentiment from social media feeds, customer reviews, or live conversations, allowing businesses to respond promptly to customer sentiments.
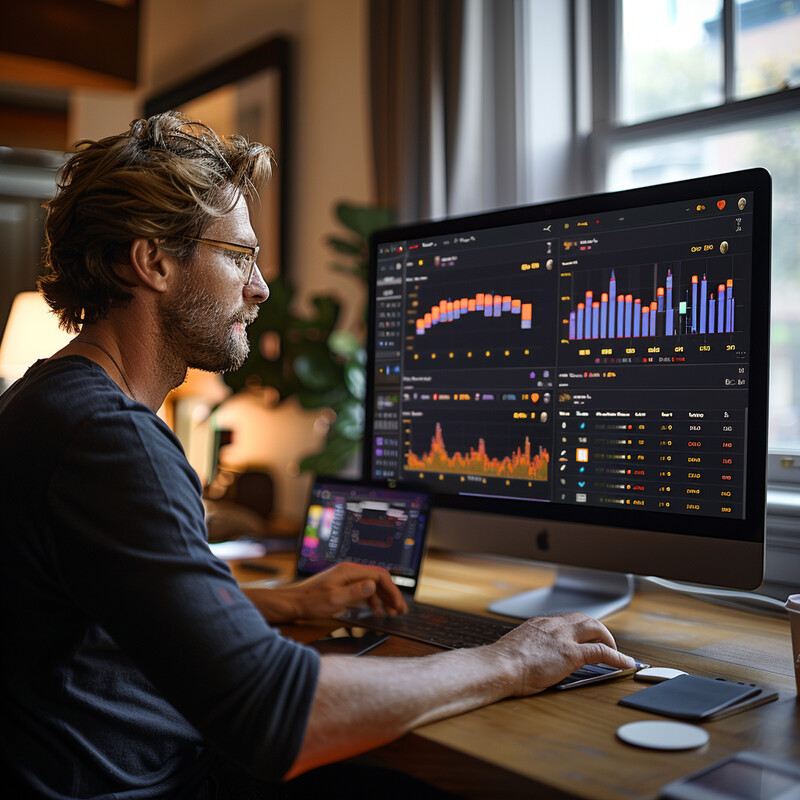
AI enables the monitoring and analysis of sentiment in real-time, which is particularly valuable for social media platforms, live customer feedback, or during events. This immediate analysis allows businesses and organizations to quickly gauge public reaction and adjust their strategies or responses on the fly to better align with their audience's mood and feedback.
4. Multilingual Sentiment Analysis
Advanced AI algorithms can analyze sentiment in multiple languages, breaking down linguistic barriers and providing more global insights.
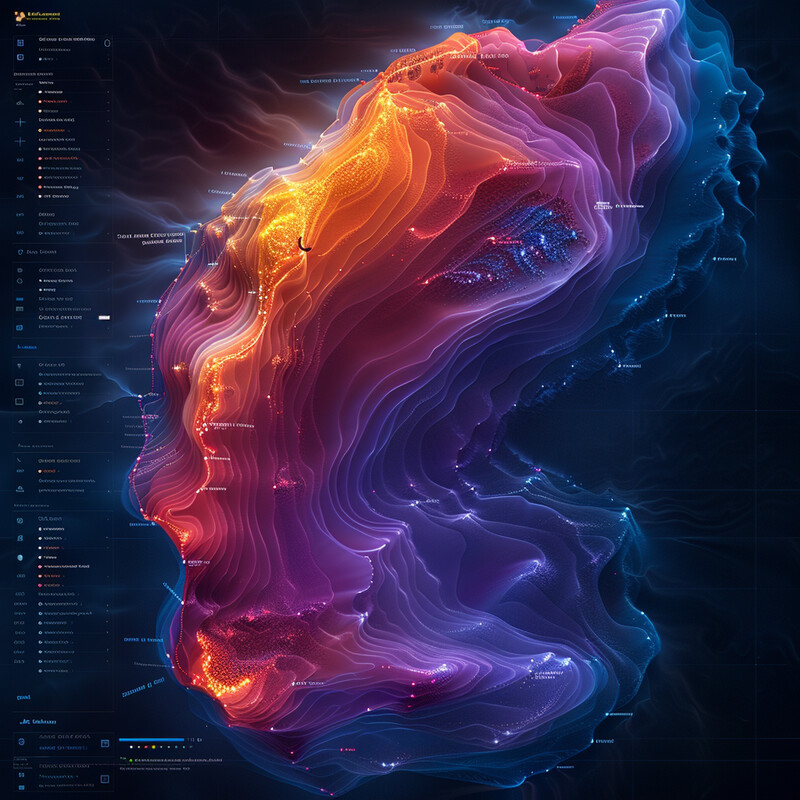
Advanced AI algorithms are capable of understanding and analyzing sentiments across multiple languages without significant loss in accuracy. This is crucial for global businesses and applications where user-generated content comes from diverse linguistic backgrounds, allowing for consistent sentiment analysis across different markets and cultural contexts.
5. Emotion Detection
Beyond simple positive or negative classifications, AI can identify complex emotional states such as happiness, frustration, or sarcasm, providing deeper insights into consumer behavior.
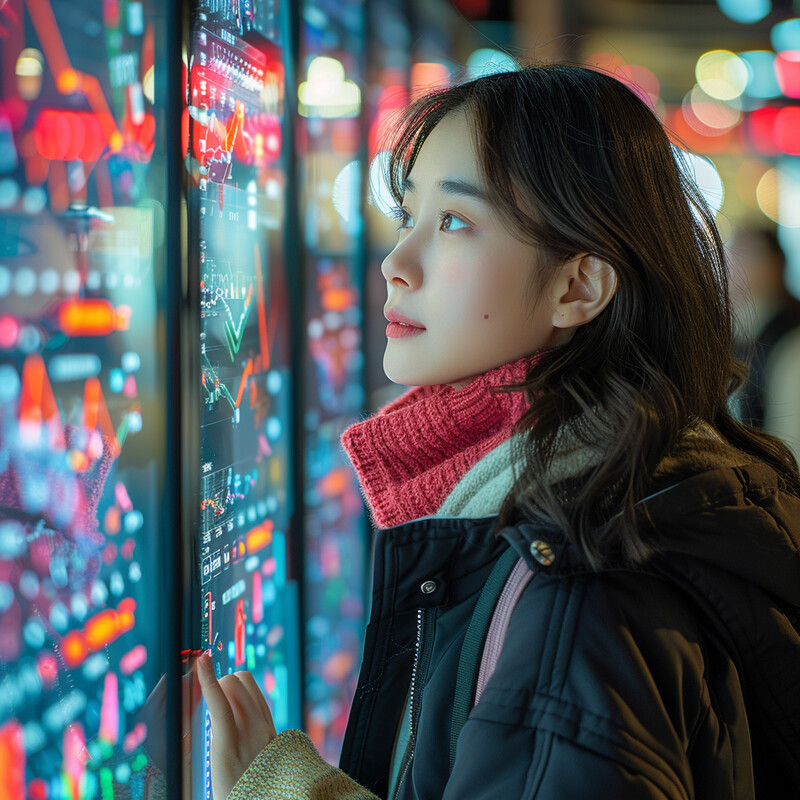
AI systems can identify a range of complex emotional states from text, such as joy, anger, sadness, or sarcasm, providing a nuanced understanding of sentiment beyond basic positive or negative categories. This detailed emotional analysis helps companies understand consumer sentiments more deeply, enabling more tailored and empathetic responses.
6. Integration with Other Data Sources
AI integrates sentiment analysis with other data types, such as purchase history or demographic information, to offer a more comprehensive view of consumer attitudes and behaviors.
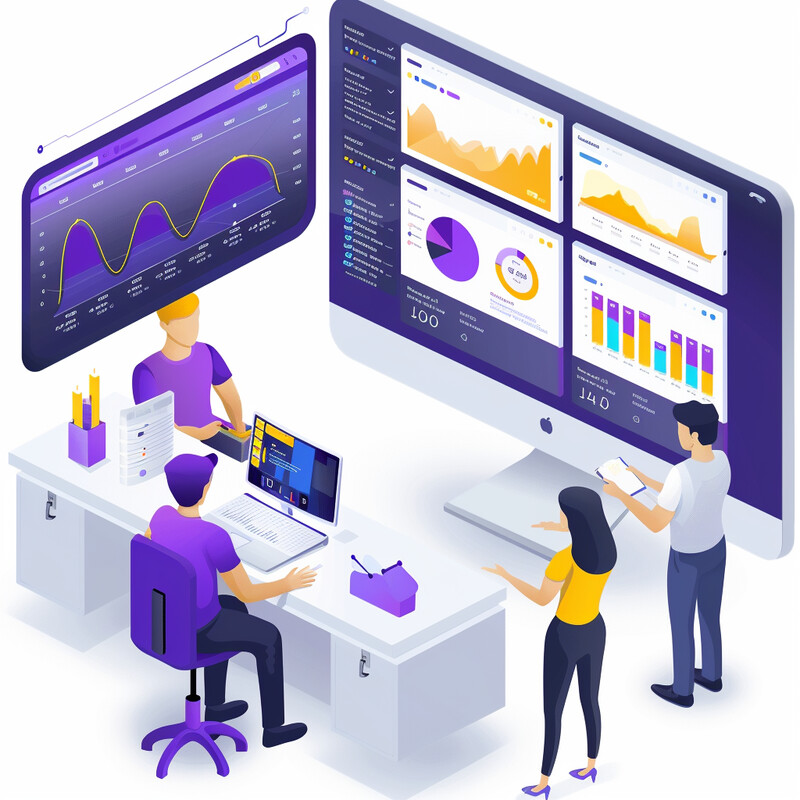
AI enhances sentiment analysis by integrating it with other relevant data sources, such as behavioral data, purchase history, or demographic information. This holistic approach provides a richer, more detailed picture of consumer attitudes and behaviors, aiding businesses in crafting more targeted and effective marketing and engagement strategies.
7. Scalability
AI systems can process and analyze vast amounts of data much more quickly than humans, making sentiment analysis scalable across large datasets and broad digital platforms.
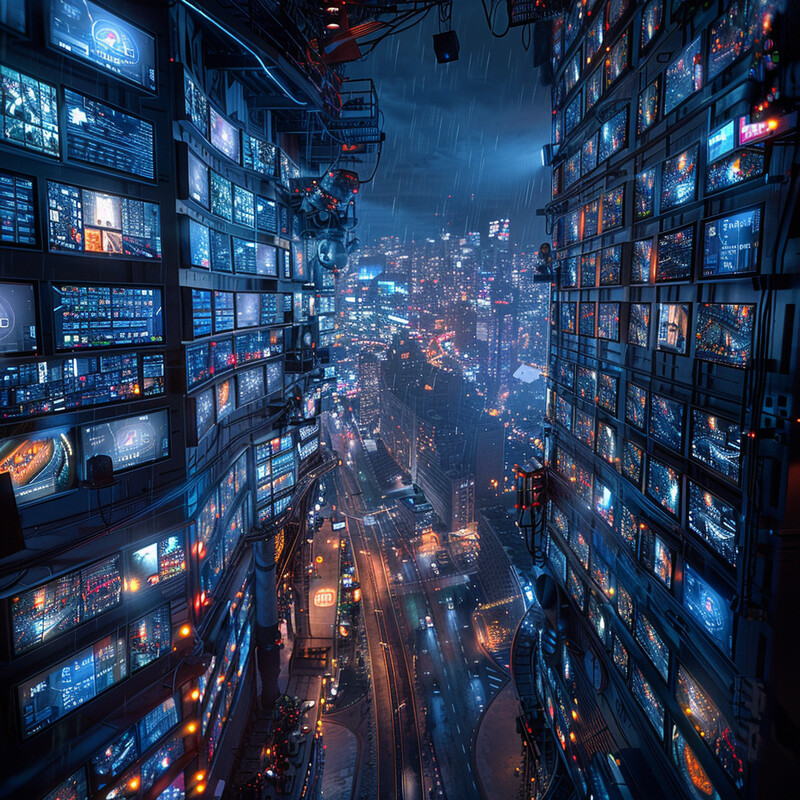
AI can efficiently process and analyze vast quantities of data from various sources, such as social media posts, customer reviews, or forum discussions, quickly and accurately. This scalability ensures that sentiment analysis can be applied broadly across extensive datasets, providing valuable insights that are statistically significant and actionable.
8. Precision and Customization
I models can be trained to recognize industry-specific language and sentiments, enhancing precision and relevance in fields like healthcare, finance, or customer service.
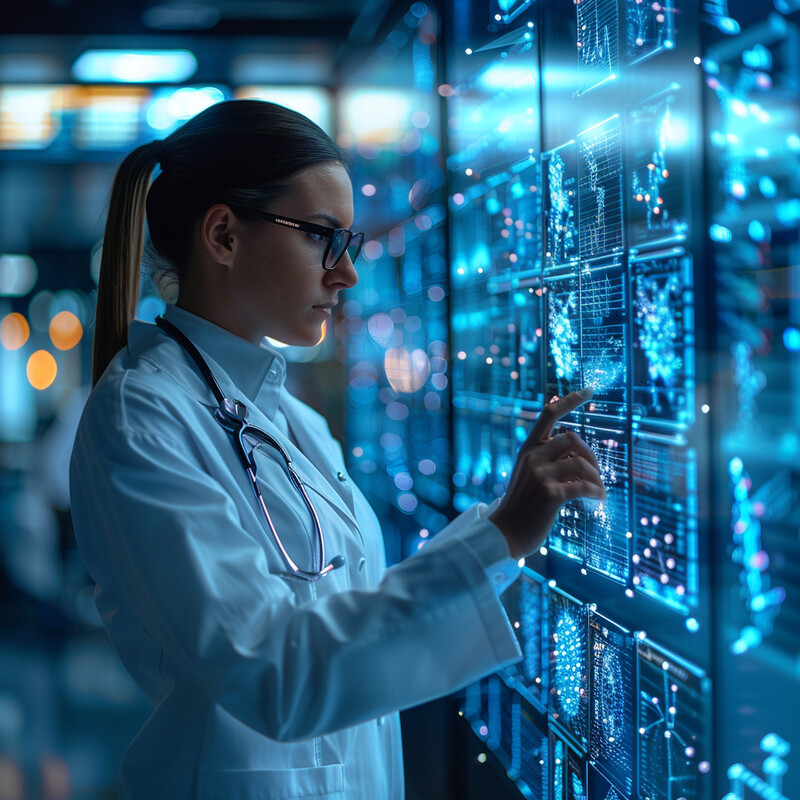
AI models can be customized and trained to recognize specific jargon and sentiments related to particular industries or fields. For instance, the sentiment analysis for medical patient feedback would be tailored differently than for a financial advice forum, enhancing both the relevance and accuracy of the analysis in different contexts.
9. Predictive Analysis
By analyzing trends in sentiment data, AI can predict future changes in public opinion or consumer preferences, helping companies to strategize effectively.
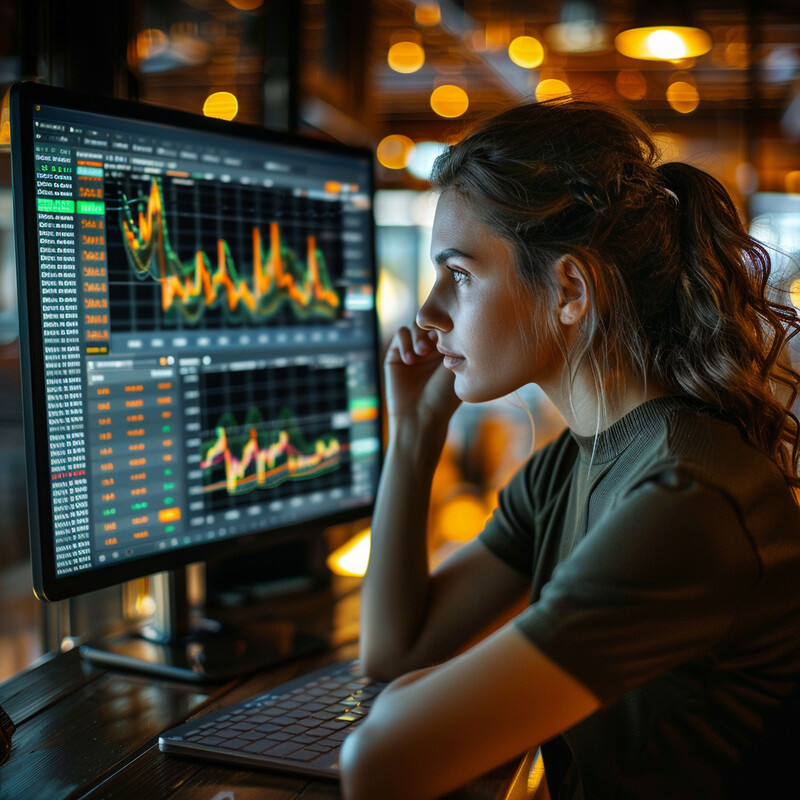
AI leverages historical sentiment data to forecast future trends in public opinion or consumer preferences. This predictive capability allows businesses to anticipate market shifts, adapt their products or services early, and stay ahead of consumer trends by understanding how sentiments evolve over time.
10. Visual Sentiment Analysis
AI extends sentiment analysis to visual content, interpreting emotions and reactions from images and videos, such as social media or advertising campaigns.
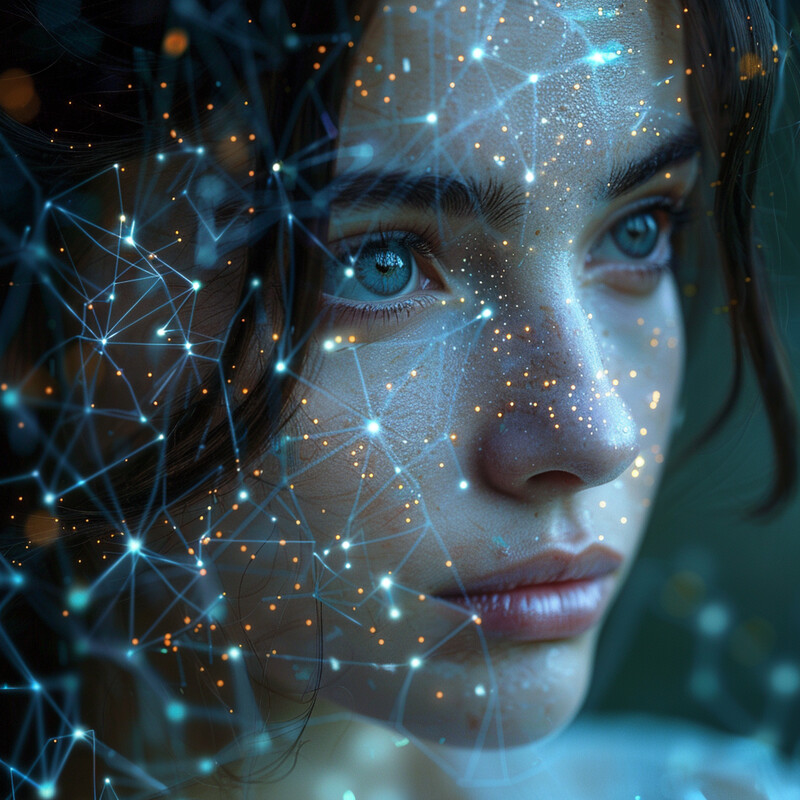
Expanding beyond text, AI applies sentiment analysis techniques to visual content, analyzing images and videos to interpret the emotions conveyed by facial expressions, body language, and other visual cues. This capability is particularly useful in marketing and advertising, where visual content plays a crucial role in influencing consumer perceptions and behaviors.