1. Pattern Recognition
Artificial intelligence (AI) excels at identifying subtle patterns in seismic data that may precede or signify earthquakes. By training on vast amounts of historical seismic records, AI models can distinguish true earthquake signals from background noise far more effectively than traditional methods. This means many small tremors or precursory vibrations, which might be overlooked by human analysts or simple algorithms, are detected and cataloged. AI pattern recognition thus enhances our understanding of the precursors and frequency of earthquakes, contributing to improved (though still imperfect) prediction capabilities. Overall, AI’s ability to continually learn from new seismic data allows it to refine these pattern detections over time, potentially providing earlier indications of seismic activity than previously possible.
AI algorithms analyze seismic data to identify patterns and anomalies that may indicate potential earthquakes, enhancing the prediction accuracy.
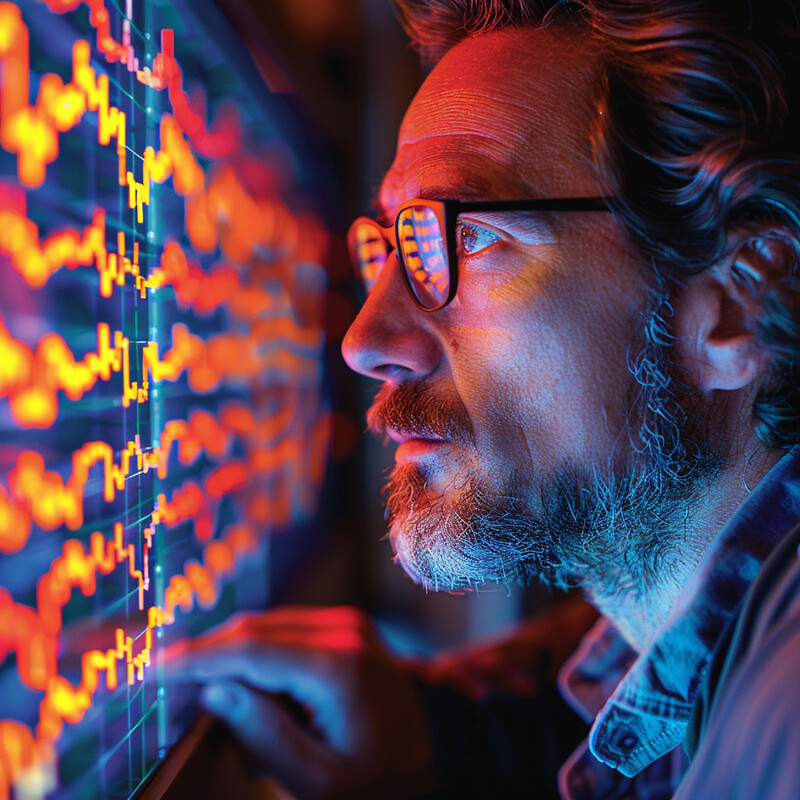
A 2024 scientific review noted that applying deep learning to seismic signal processing can increase the number of detected earthquakes by roughly an order of magnitude compared to conventional techniques. In practice, machine-learning algorithms have expanded earthquake catalogs by up to ten times, uncovering many micro-earthquakes that were missed before. This dramatic improvement in event detection gives seismologists a far more detailed picture of seismic activity patterns, which is a critical step toward better forecasting models.
AI utilizes advanced pattern recognition algorithms to analyze seismic data, identifying subtle signs that may precede an earthquake. By examining historical earthquake data and current seismic activity, AI can detect patterns that are indicative of future seismic events, improving prediction accuracy and giving valuable lead time for emergency preparedness.
2. Real-Time Data Processing
AI enables real-time processing of the continuous flood of seismic data coming from networks of sensors around the world. Traditional data analysis might take minutes or hours, but modern AI systems can interpret incoming vibration signals almost instantaneously. By rapidly filtering noise and identifying significant motion, AI helps seismologists recognize developing earthquakes as they happen. This immediacy is crucial in a crisis: quicker analysis means earlier warnings and more timely decisions (such as initiating shutdown procedures or evacuations). In essence, AI’s high-speed data crunching turns massive, complex seismic datasets into actionable information within seconds, greatly improving our responsiveness to seismic events.
AI can process vast amounts of seismic data in real-time, allowing for immediate analysis and quicker response times in case of seismic events.
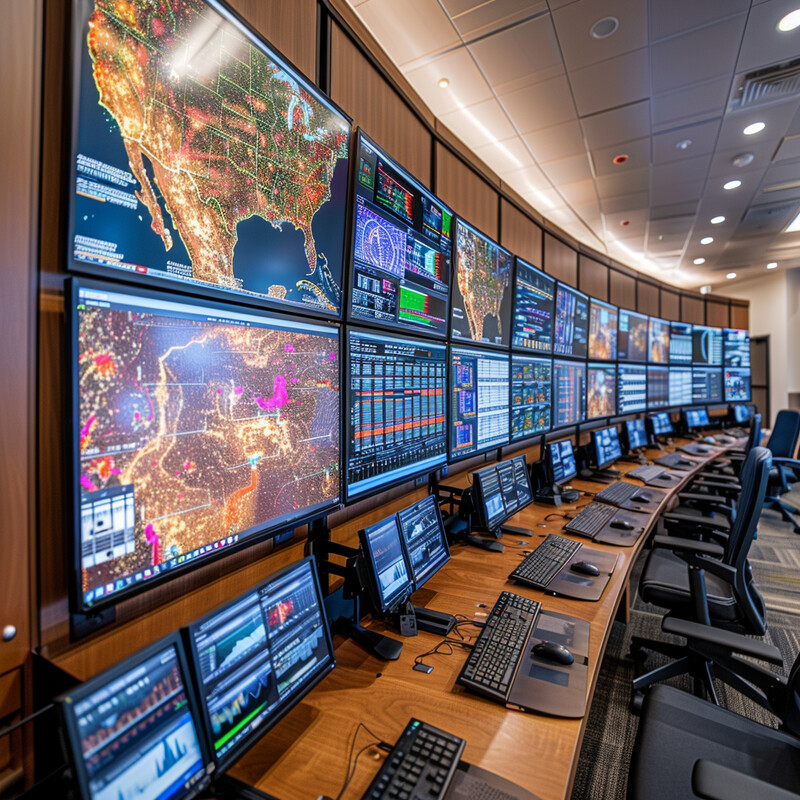
AI-based systems now operate at a scale and speed previously unattainable in seismology. For example, researchers demonstrated an earthquake detection framework in 2025 that analyzes data from over 8,000 distributed seismic sensors in real time. This AI-driven network, deployed in South Korea as part of the “CrowdQuake” project, can concurrently process thousands of signals and rapidly confirm an earthquake’s occurrence. Such capability shows how AI is handling “big data” in seismology, with rapid analysis across thousands of data streams allowing emergency systems to react almost immediately when an earthquake begins.
AI excels in processing large volumes of seismic data in real-time, allowing for instantaneous analysis that is crucial during seismic events. This capability enables seismologists and disaster response teams to make informed decisions quickly, potentially reducing the impact of earthquakes by facilitating timely evacuations and responses.
3. Early Warning Systems
AI is enhancing earthquake Early Warning Systems (EEWs) by making them faster and more accurate. In an earthquake, every second of advance notice counts, and AI algorithms can analyze the first ripples of a seismic event to predict the severity of shaking that will follow. By quickly distinguishing an actual quake from false signals (like industrial noise) and estimating its magnitude, AI helps trigger warnings to the public and shut down critical infrastructure moments after an earthquake starts. These improvements mean that people in affected areas could receive alerts several seconds sooner than with older systems. AI also helps reduce false alarms by learning the difference between benign vibrations and dangerous earthquakes, thereby increasing public trust in early warnings. Overall, integrating AI into EEWs leads to more reliable alerts and a bit more time for people to take cover before strong shaking arrives.
AI integrates with seismic sensors to provide early warnings to populations in earthquake-prone areas, potentially saving lives by giving people more time to seek safety.
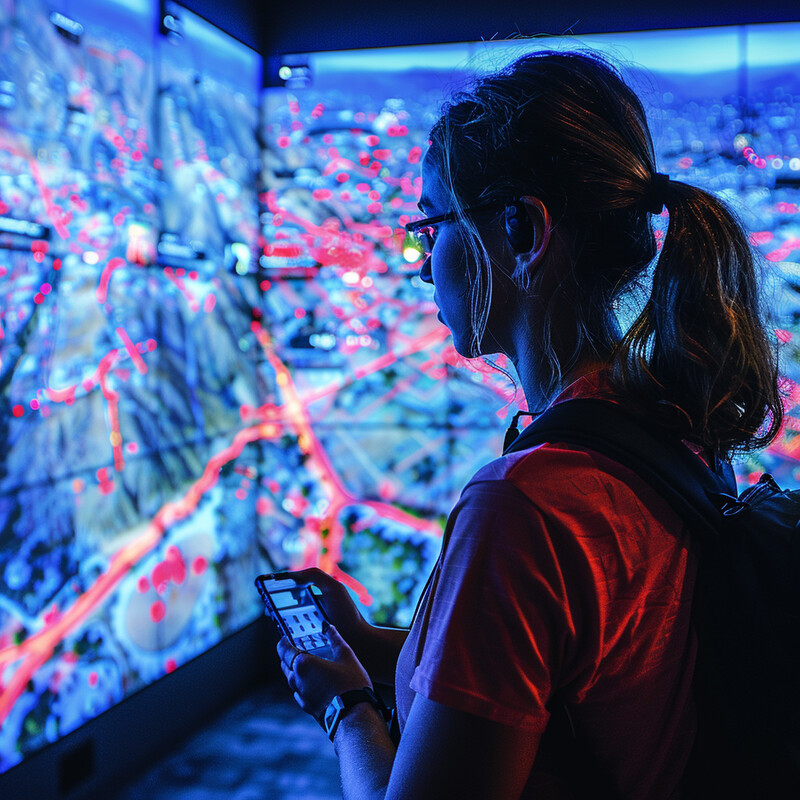
Recent developments show how AI can push the boundaries of early warning speed and reliability. In 2023, seismologists introduced an AI-driven warning system that uses the first 3 seconds of data from a single seismic station to detect an earthquake and estimate its magnitude. In tests, this Ensemble Earthquake Early Warning System (E3WS) achieved 99.9% accuracy in distinguishing quakes from non-quake noise, with virtually no false alarms. Remarkably, it updates its magnitude estimate every second as new data comes in, outperforming traditional multi-station methods and potentially giving several extra seconds of warning for people to act. Such AI-powered advancements demonstrate significantly improved performance over conventional early warning approaches.
Integrating AI with seismic sensors enhances early warning systems for earthquakes. AI analyzes the seismic data to quickly determine the potential severity of an earthquake and can automatically trigger alerts to warn residents and authorities in affected areas. This rapid response can save lives by providing people more time to evacuate or take cover.
4. Risk Assessment Mapping
AI is revolutionizing how we map earthquake risk by analyzing complex datasets that include geology, seismic history, and infrastructure vulnerability. Traditional seismic hazard maps are updated infrequently and at coarse resolution; AI models, in contrast, can continuously learn from new data and produce more detailed, dynamic risk maps. These models consider not just where earthquakes are likely, but also how soil conditions and building types in each locale might amplify damage. The result is a nuanced picture of risk: for instance, AI-generated maps can highlight specific neighborhoods within a city that would suffer more in a quake due to weaker ground or denser structures. Such information is invaluable for urban planners and emergency managers, as it guides them to reinforce critical buildings and prioritize high-risk zones in disaster planning. By integrating diverse data sources, AI-driven risk maps help communities allocate resources more effectively to mitigate earthquake impacts.
AI models generate detailed risk assessments for different regions by analyzing geological data, historical seismic activity, and urban infrastructure, helping in disaster preparedness and mitigation planning.
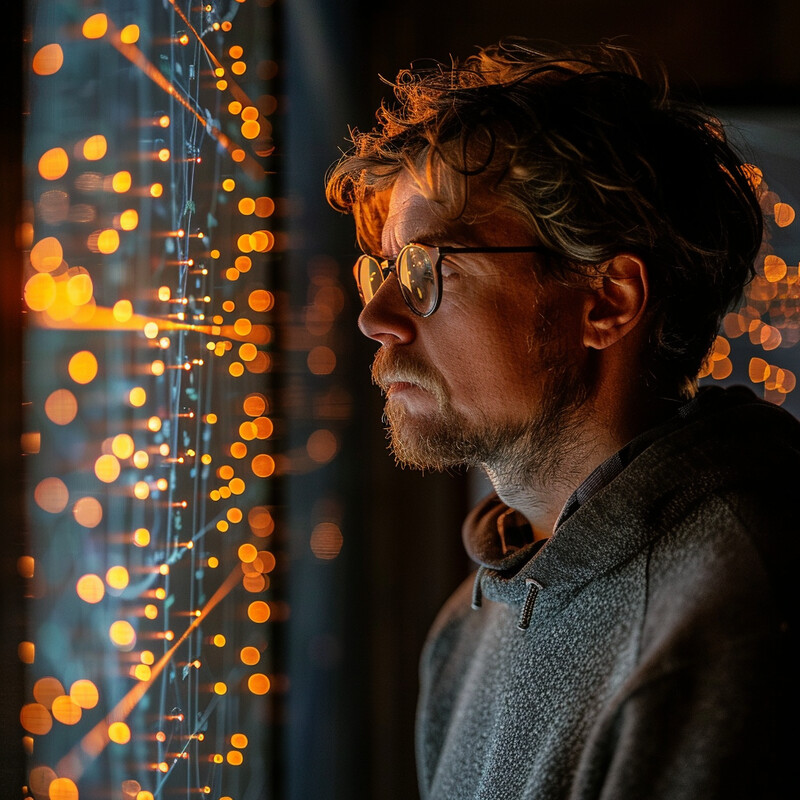
A recent study illustrates the power of AI in multi-factor risk analysis. In 2023, researchers used machine learning to combine seismic hazard data with socio-demographic and infrastructure information, producing high-resolution risk assessments for communities. Their AI model categorized about 34.1% of the examined cities in one region as facing significant multi-hazard risk (including earthquakes), and further identified that 15.9% of those high-risk cities also had high social vulnerability. This level of granular mapping—showing not just the physical risk but where populations would be most vulnerable—demonstrates how AI-based tools are informing more targeted earthquake preparedness and mitigation strategies. Such detailed risk maps allow for smarter allocation of resources, such as retrofitting buildings in the most at-risk areas first.
AI models help in creating detailed seismic risk assessments for different regions. By analyzing geological data, historical seismic activity, and the current state of urban infrastructure, AI provides insights into potential earthquake impacts, guiding urban planning and disaster readiness efforts to mitigate risks effectively.
5. Aftershock Prediction
Predicting aftershocks is an area where AI is making important strides. After a big earthquake, dozens or even hundreds of aftershocks can occur, and estimating their timing, locations, and magnitudes is crucial for ongoing emergency response. AI models are being trained on past earthquake sequences to recognize patterns in how aftershocks unfold. These models can incorporate many variables—such as the mainshock’s characteristics, stress changes in the crust, and even geological features—that traditional statistical models use only in a limited way. Early machine-learning efforts have shown promise in forecasting the likelihood of aftershocks more accurately, especially in identifying which areas are at higher risk. While no system can pinpoint every aftershock, AI can provide probability maps and magnitude estimates that improve upon decades-old methods like the Gutenberg-Richter and Omori law approaches. In summary, AI is helping to refine aftershock forecasts, giving responders better guidance on where strong aftershocks might strike and for how long the hazard will continue after a major quake.
After a major earthquake, AI predicts the likelihood, magnitude, and location of aftershocks, aiding emergency response teams in focusing their efforts where they are needed most.
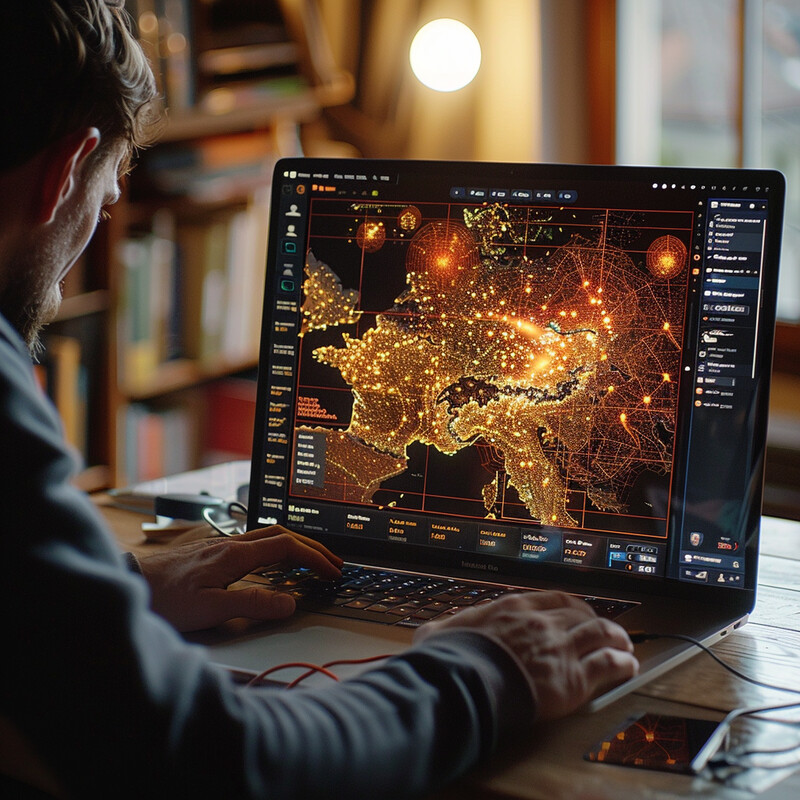
Established aftershock forecast models (in use since the 1980s) are relatively simple, but AI is starting to enhance these with more data-driven insight. An Annual Reviews report in 2024 observes that machine learning methods, fed by new high-resolution earthquake catalogs, are improving aftershock probability estimates. In practice, one deep-learning study was able to forecast the spatial distribution of aftershocks more accurately than the traditional stress-triggering models, by learning complex nonlinear relationships from thousands of past quakes (DeVries et al., 2018). According to Hardebeck et al. (2024), the integration of such AI techniques is expected to eventually boost the precision of aftershock forecasts, although as of mid-2024, the best results still combine AI with the reliability of proven statistical laws. This indicates a trend where AI augments—not outright replaces—classical aftershock prediction, leading to steadily better forecasts.
Following major earthquakes, AI is used to predict aftershocks, which can often be nearly as damaging as the initial quake. AI assesses the likelihood, magnitude, and potential locations of aftershocks, enabling emergency services to prioritize and plan their responses more effectively to protect lives and property.
6. Training Simulations
AI-powered simulations are transforming how emergency teams prepare for earthquakes. Instead of solely relying on static drills or past scenarios, responders can now train in virtual environments that are guided by AI to mimic real earthquake events with high realism. These simulations can generate countless different earthquake scenarios—varying magnitudes, locations, times of day, building collapses, etc.—to challenge and teach responders. Because the scenarios are AI-driven, they can adapt in real time to the trainees’ decisions, creating a dynamic training where the situation evolves like a real disaster would. This improves readiness by exposing teams to a wide range of possibilities and stress conditions in a safe setting. Importantly, AI simulations also allow for detailed performance tracking: the system can evaluate how quickly and effectively participants react, then provide feedback or adjust difficulty accordingly. By making training more immersive, varied, and interactive, AI helps emergency personnel and even the general public practice the best responses to an earthquake before one strikes.
AI-driven simulations train emergency response teams by creating realistic earthquake scenarios, helping improve response strategies and preparedness.
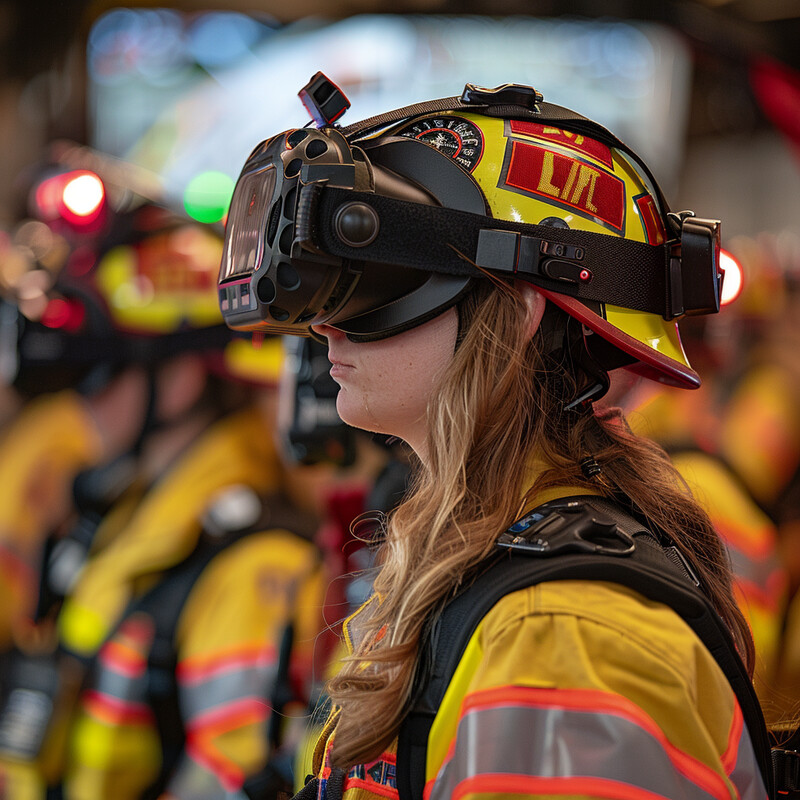
Research confirms that such high-tech training tools can significantly improve preparedness. A 2024 review of disaster training methods found that immersive virtual reality exercises—often enhanced by AI scenario generation—were more effective in developing emergency response plans than traditional tabletop drills. Trainees who used VR/AI simulations showed better retention of safety procedures and more confidence in what actions to take during a quake. These AI-driven simulations provide a life-like experience (e.g., realistic building shaking and damage, virtual victims, and time-pressured decision points) that is difficult to achieve otherwise. As a result, many emergency management institutions are now encouraging the adoption of AI-based virtual training to complement or even replace some physical drills, given the clear benefits in engagement and learning outcomes.
AI-driven training simulations provide emergency responders with realistic earthquake scenarios. These simulations help improve preparedness by allowing teams to practice their responses, making adjustments based on AI-generated scenarios that model various earthquake magnitudes and impacts.
7. Integration with Building Management Systems
AI is being integrated into building management and design to evaluate and improve structural resilience against earthquakes. Modern “smart” buildings may have an array of sensors (accelerometers, strain gauges, etc.) whose data can be continuously analyzed by AI to assess the building’s structural health. If an earthquake strikes, AI algorithms can instantly predict how the building will respond—identifying which parts might be overstressed or if there’s a risk of collapse—allowing for rapid building-specific alerts or even automatic safety actions (like unlocking doors or shutting off gas lines). In engineering design, AI tools can simulate thousands of earthquake scenarios on a computer model of a structure, identifying weaknesses and suggesting optimal reinforcement strategies. This helps architects and engineers create buildings that are not only code-compliant but truly optimized for seismic safety. In existing buildings, AI-driven analysis can guide retrofitting efforts by pinpointing the most vulnerable elements. By blending into building management systems, AI essentially acts as a 24/7 structural analyst, enhancing both the real-time emergency response for buildings and the long-term design of more resilient infrastructure.
AI algorithms analyze the structural integrity of buildings and predict how they would withstand earthquakes, providing valuable insights for constructing more resilient infrastructures.
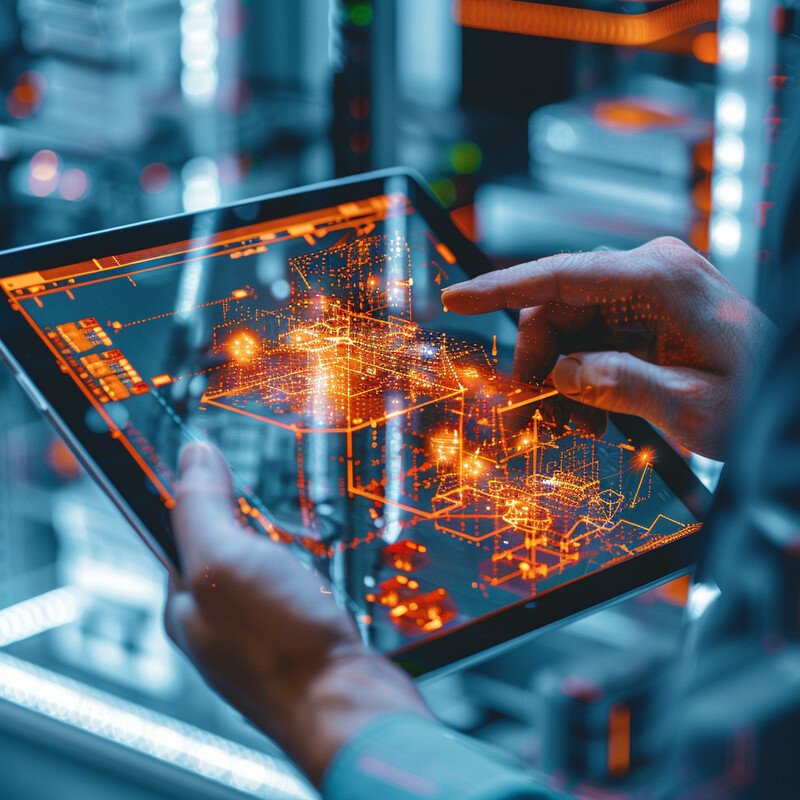
We are seeing concrete evidence of AI’s impact on structural resilience. A recent study demonstrated that an AI-controlled system can dramatically reduce a building’s motion during an earthquake. In simulations, an advanced neural-network-based controller cut building vibrations by up to 63% compared to an uncontrolled structure, whereas traditional control systems (using old methods like fuzzy logic) only achieved about a 12–13% reduction. This result, published in 2025, highlights how AI algorithms can far outperform conventional engineering approaches in keeping structures stable. By actively adjusting a building’s dampers or braces in real time, the AI system limited shaking much more effectively, which would translate to less damage in a real quake. Such findings underscore the potential of AI-integrated building systems to protect structures and save lives by intelligently managing structural responses during seismic events.
AI can assess the structural integrity of buildings and predict how they might respond to seismic events. This technology is vital for engineers and architects, who can use AI insights to design and retrofit buildings to withstand earthquakes, enhancing public safety and infrastructure resilience.
8. Public Education and Awareness
AI is playing an increasing role in educating the public about earthquake preparedness. Traditional public education (like brochures or generic websites) can be passive and one-size-fits-all, whereas AI allows for interactive and personalized learning experiences. For instance, AI-powered apps or chatbots can answer individuals’ questions about earthquakes in real time, guide them through making an emergency kit, or even quiz them on what to do during shaking. These tools can adapt the information based on a user’s location and circumstances—providing relevant tips if someone lives in an older house versus a new apartment, for example. Additionally, AI can drive simulation-based learning for the public: imagine a smartphone app that uses augmented reality to show what would fall over in your own living room during a severe quake, teaching you how to “quake-proof” your space. By making earthquake education more engaging (through gamification, Q&A bots, scenario simulations, etc.), AI helps people retain crucial safety knowledge. Ultimately, a more informed public means fewer injuries and quicker recovery when earthquakes happen, as citizens will know how to react and can even take preventative actions like retrofitting their homes with guidance from AI-driven tools.
AI-powered applications and tools educate the public about earthquake preparedness, simulating different scenarios and providing safety tips and procedures.
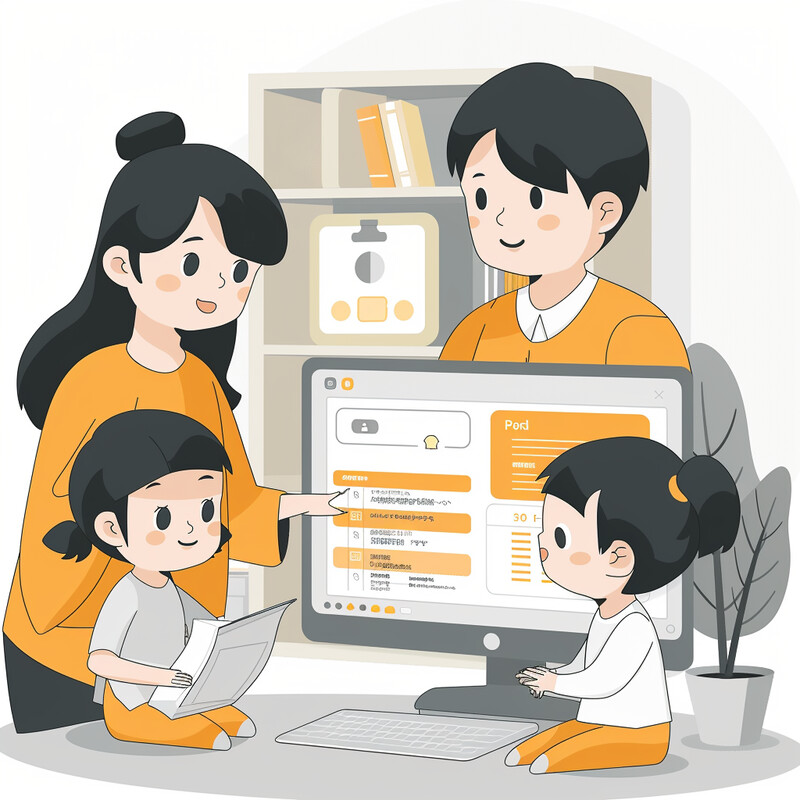
One emerging application is the use of AI to deliver customized preparedness advice. Researchers have noted that AI-driven platforms could analyze an individual’s dwelling and locale to provide specific safety recommendations. For example, an AI system might determine that a person’s home is in a high liquefaction zone and suggest securing heavy furniture and installing flexible gas line connectors as priority measures. Such a system can also present interactive earthquake scenarios: it might show a homeowner a simulation of how a nearby fault rupture would shake their neighborhood, underscoring the importance of the recommended preparations. While still in pilot stages, these personalized education approaches are being tested in various regions. Early results indicate that people who engage with AI-based earthquake education tools are more likely to take preparedness actions (Mukherjee & Chandra, 2024). This points to AI’s potential in closing the gap between awareness and action by tailoring advice to make earthquake readiness more relatable and urgent for each user.
AI-powered applications engage the public in learning about earthquake preparedness. These tools can simulate earthquake scenarios, offer personalized safety tips, and educate individuals on the best practices to follow during and after an earthquake, increasing community readiness and resilience.
9. Subsurface Exploration
AI is greatly improving the mapping of subsurface geological structures, which is vital for understanding where and how earthquakes happen. In seismic surveys (whether for research or oil exploration), huge volumes of data about underground rock layers and fault structures are collected. AI techniques can process this data faster and pick out features with finer detail than traditional methods. One advantage is the ability of AI to detect subtle signals in seismic waves that indicate small faults or fractures in the Earth’s crust. By identifying these hidden fault lines or weak zones, scientists get a more complete map of the tectonic setting, which in turn helps in assessing earthquake hazard. Moreover, AI algorithms can integrate different types of data—seismic, geologic, even gravity and magnetic surveys—to produce 3D models of the subsurface. These models reveal the arrangement of rock formations and fault networks at depth, shedding light on how stress might build up and release in an earthquake. In short, AI-driven analysis is like having X-ray vision underground: it enhances our ability to “see” the complex puzzle of tectonic plates and faults beneath us, improving both resource exploration and seismic risk evaluation.
AI analyzes seismic waves to map the Earth's subsurface structures, enhancing our understanding of tectonic movements and contributing to more accurate seismic predictions.
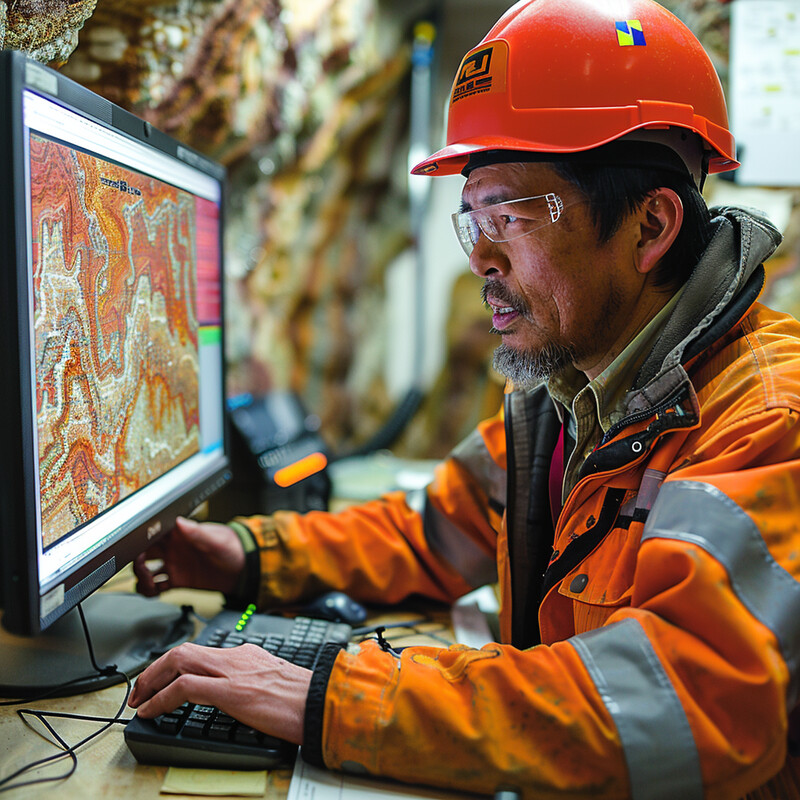
A cutting-edge example comes from Germany’s Fraunhofer Institute, where scientists in 2023 used machine learning to sharpen seismic imaging of the Earth’s crust. Their enhanced AI-based process can detect small fault zones and geologic discontinuities in seismic reflection data for the first time. These are tiny features—places where rock properties suddenly change—that older imaging techniques often blurred out. By making such faults visible in seismic images, the AI-driven method provides meter-scale precision in mapping underground structures (Fraunhofer ITWM, 2023). This breakthrough means that geologists can now identify previously unknown faults or fractures that could be sources of earthquakes or pathways for fluids. The ability to map these fine details deep below the surface represents a significant leap in subsurface exploration, directly contributing to more accurate assessments of earthquake-prone areas and better-informed decisions on things like where to build critical facilities or how to route tunnels and pipelines.
AI analyzes seismic waves to map subsurface geological structures, providing a deeper understanding of tectonic movements and fault lines. This detailed subsurface mapping contributes to more accurate predictions of seismic activity, offering a broader context for understanding earthquake mechanics.
10. Remote Sensing Data Analysis
AI extends our seismic monitoring capabilities by analyzing data from satellites and aerial sensors, which can detect ground changes over large areas. One important use of remote sensing is to track ground deformation via radar satellites (InSAR): slight uplifts or sinkage of the Earth’s surface can indicate strain buildup on faults. AI algorithms can comb through these massive satellite datasets to flag unusual deformation patterns that might precede an earthquake or indicate ongoing seismic creep. Similarly, after a quake, AI can rapidly process satellite images to map damage or landslides, helping responders target the worst-hit zones even if those areas are hard to reach on the ground. In addition, AI is being explored to detect other potential precursors in remote sensing data, such as thermal infrared anomalies or ionospheric disturbances that some studies have associated with impending quakes. While the jury is still out on many precursor signals, AI’s pattern-finding prowess is valuable in this research—sifting multi-spectral satellite records for any consistent changes before earthquakes. By integrating these diverse remote observations with ground-based seismic data, AI contributes to a more comprehensive and early detection system for seismic activity, effectively adding a “big picture” view from above to our toolkit.
AI processes data from satellites and aerial surveys to detect land deformations and other precursors to seismic activity, providing additional data points for comprehensive seismic monitoring.
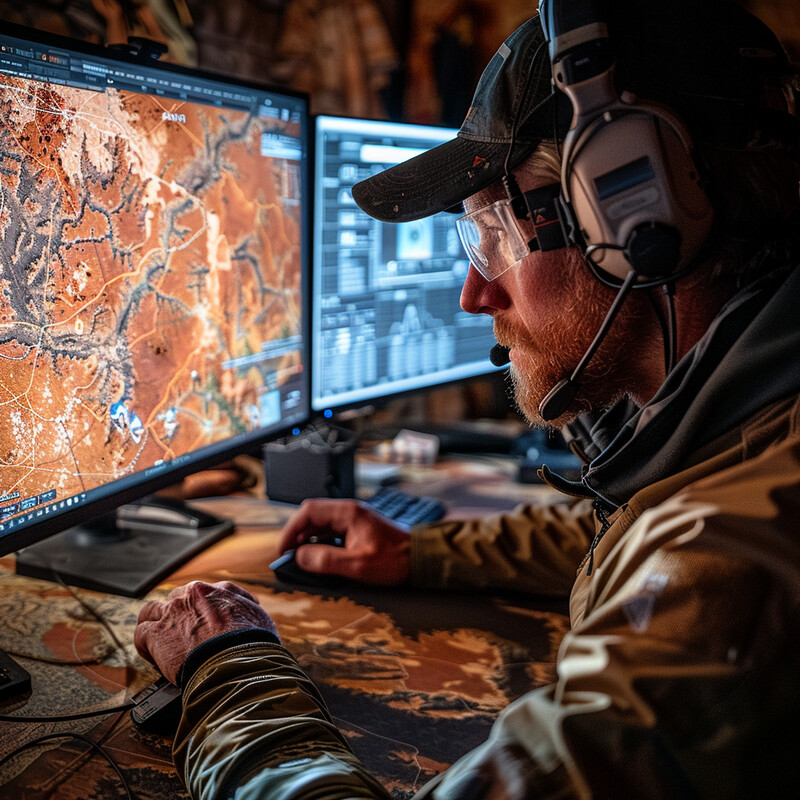
AI’s utility in remote sensing was demonstrated following the devastating February 2023 earthquakes in Turkey and Syria. In that event, deep-learning models were employed to analyze high-resolution satellite imagery and detected building collapses and ground ruptures across the earthquake zone within days, far faster than manual mapping could achieve (Zhu et al., 2023). On the front end of earthquake prediction, scientists are also leveraging AI to hunt for precursors. A 2025 study used machine learning on multi-satellite data to identify unusual atmospheric and land surface signals in the weeks before a Mw 7.5 earthquake in Japan. The system reported certain anomalies (e.g., slight atmospheric chemical changes and ground deformation patterns) that appeared to correlate with the quake’s epicentral area (Shahzad et al., 2025). While such findings are preliminary and require further validation, they showcase how AI can process complex remote sensing inputs to potentially give early hints of a coming earthquake. Whether for post-disaster damage assessment or the elusive goal of pre-disaster warnings, AI is becoming indispensable in extracting actionable information from remote sensing data.
AI processes remote sensing data, such as satellite imagery and aerial surveys, to detect land deformations and other potential indicators of seismic activity. This analysis adds a layer of data that complements traditional seismic monitoring tools, enhancing the overall capability to monitor and predict seismic events.