1. Enhanced Decision Making
AI enables RPA bots to make complex decisions based on the analysis of large data sets, moving beyond simple rule-based automation to handle more nuanced tasks.
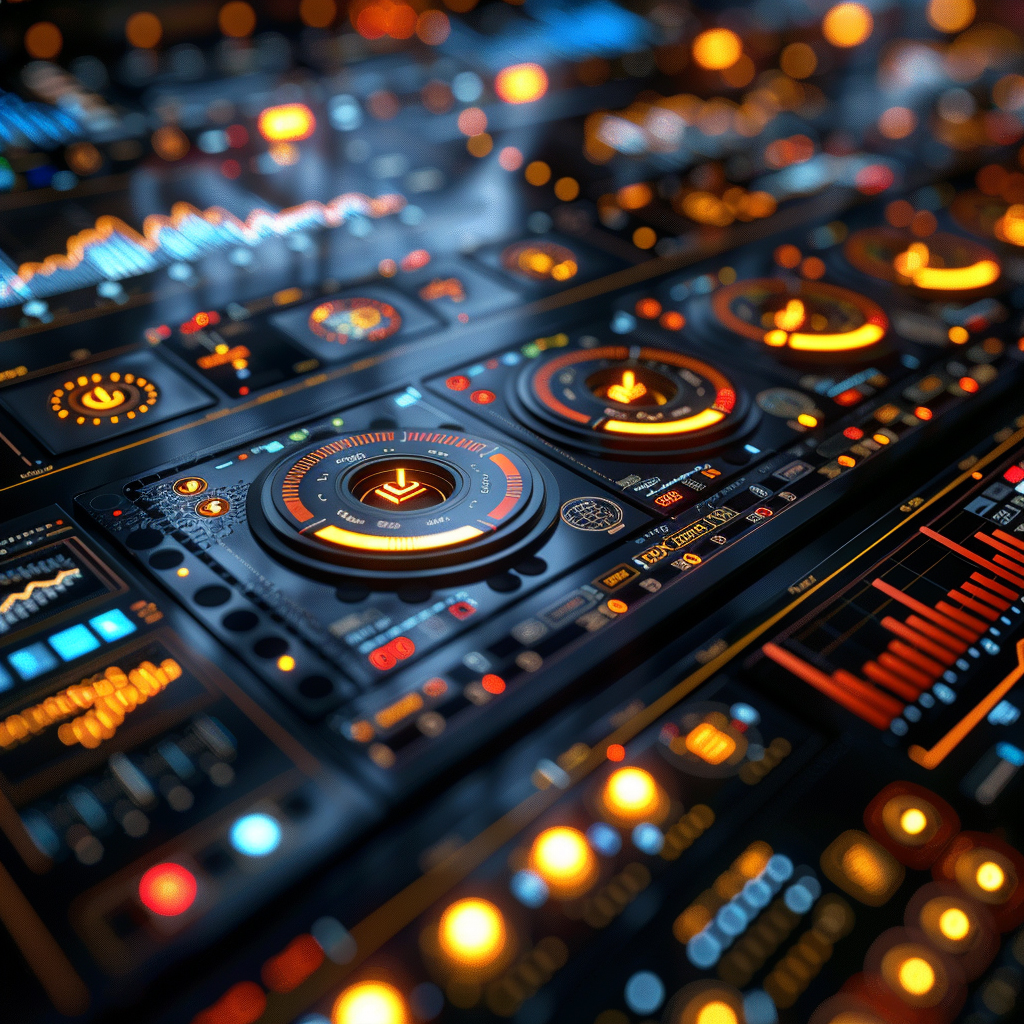
AI significantly boosts the decision-making capabilities of RPA systems by enabling them to analyze complex data sets and make informed decisions. This integration allows RPA bots to handle tasks that involve judgment and adapt to various scenarios based on historical data and learned outcomes, moving beyond simple rule-based operations to more strategic activities.
2. Improved Data Processing
AI integrates with RPA to process unstructured data such as images, emails, and natural language, enabling bots to handle a broader range of tasks that require understanding and interpretation.
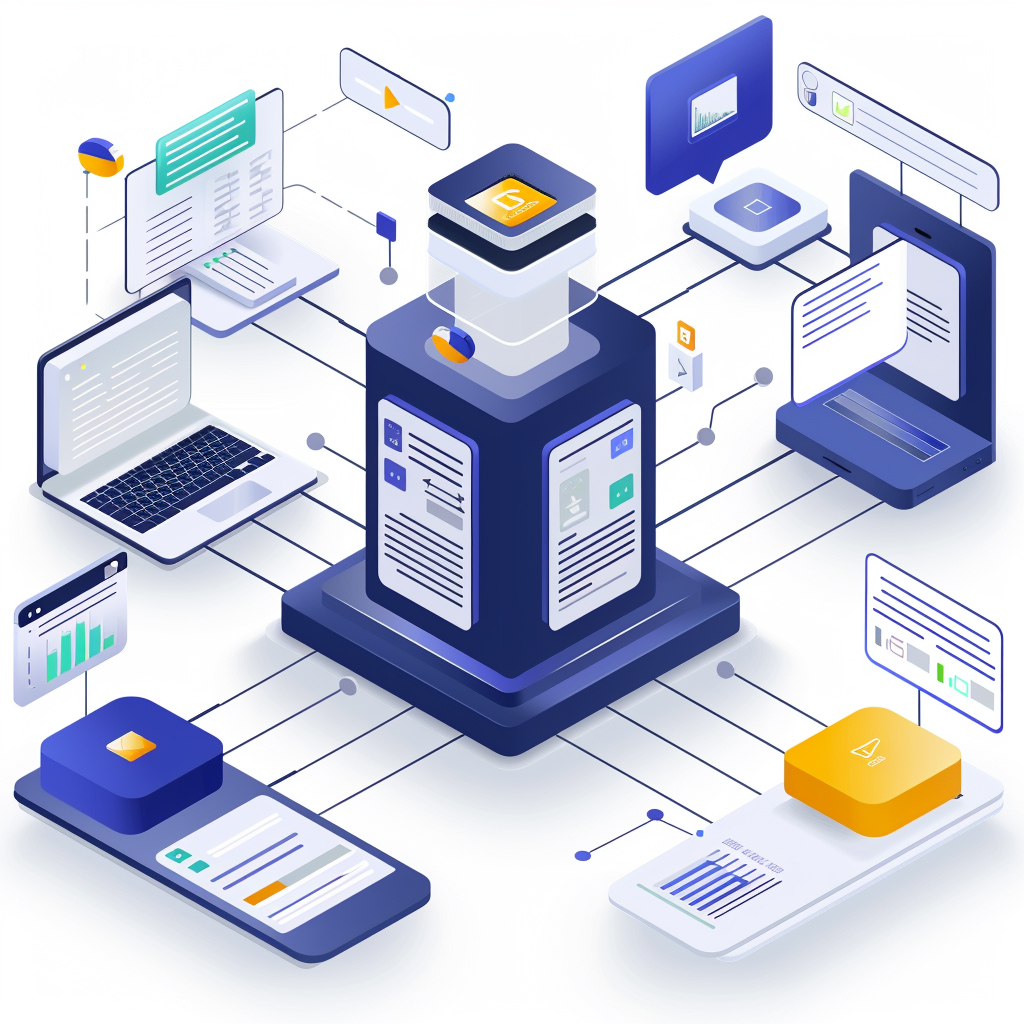
Integrating AI with RPA enables the processing of unstructured data such as text from emails, documents, social media, images, and spoken language. This capability expands the scope of RPA applications to include tasks like extracting relevant information from free-form documents or understanding customer sentiments from communications, which traditionally required human intervention.
3. Adaptive Learning
AI allows RPA systems to learn from their operations and adapt to new or changing environments without human intervention, increasing their effectiveness and reducing maintenance.
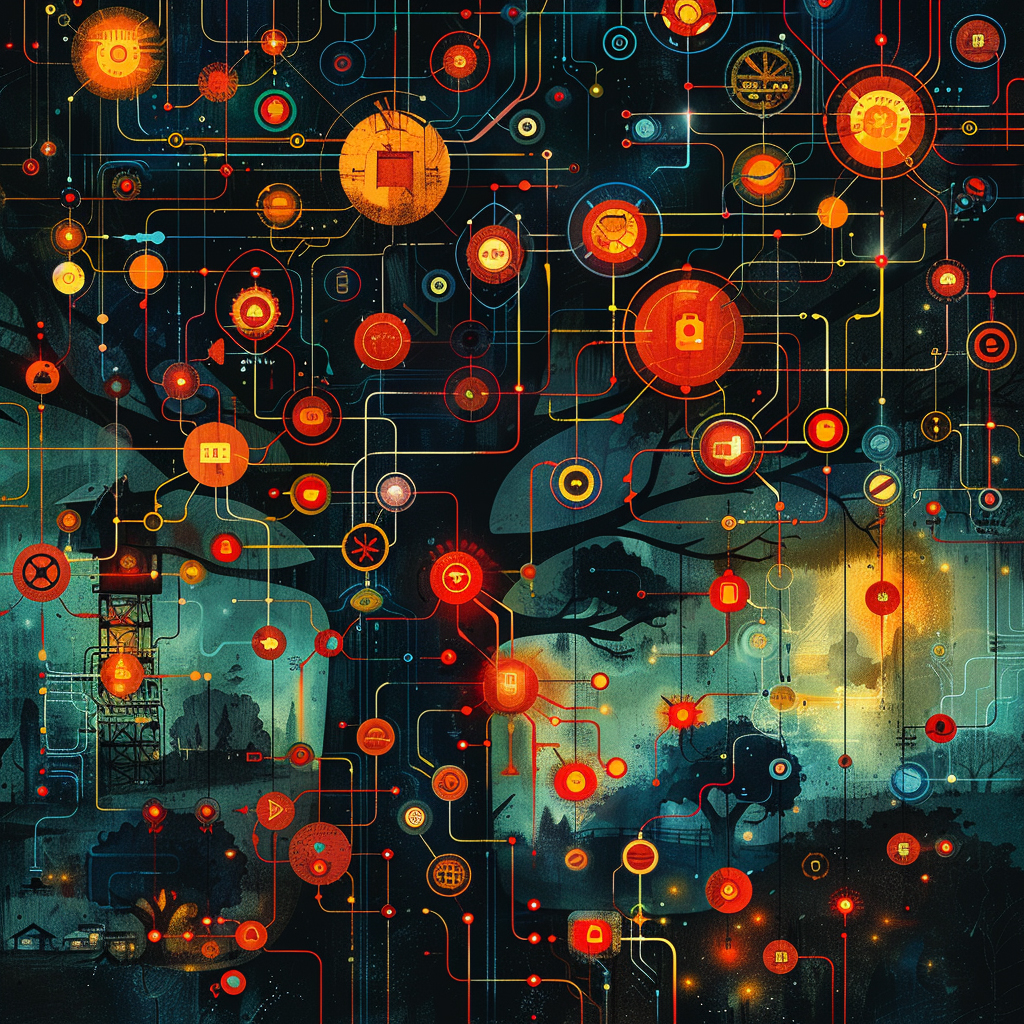
AI equips RPA systems with machine learning algorithms that allow them to learn from the outcomes of their operations and continually adapt to new or changing environments. This learning capability means that RPA bots can improve their efficiency and accuracy over time, reducing the need for human oversight and frequent updates.
4. Predictive Analytics
AI enhances RPA with predictive capabilities, allowing bots to forecast outcomes based on historical data and optimize processes proactively.
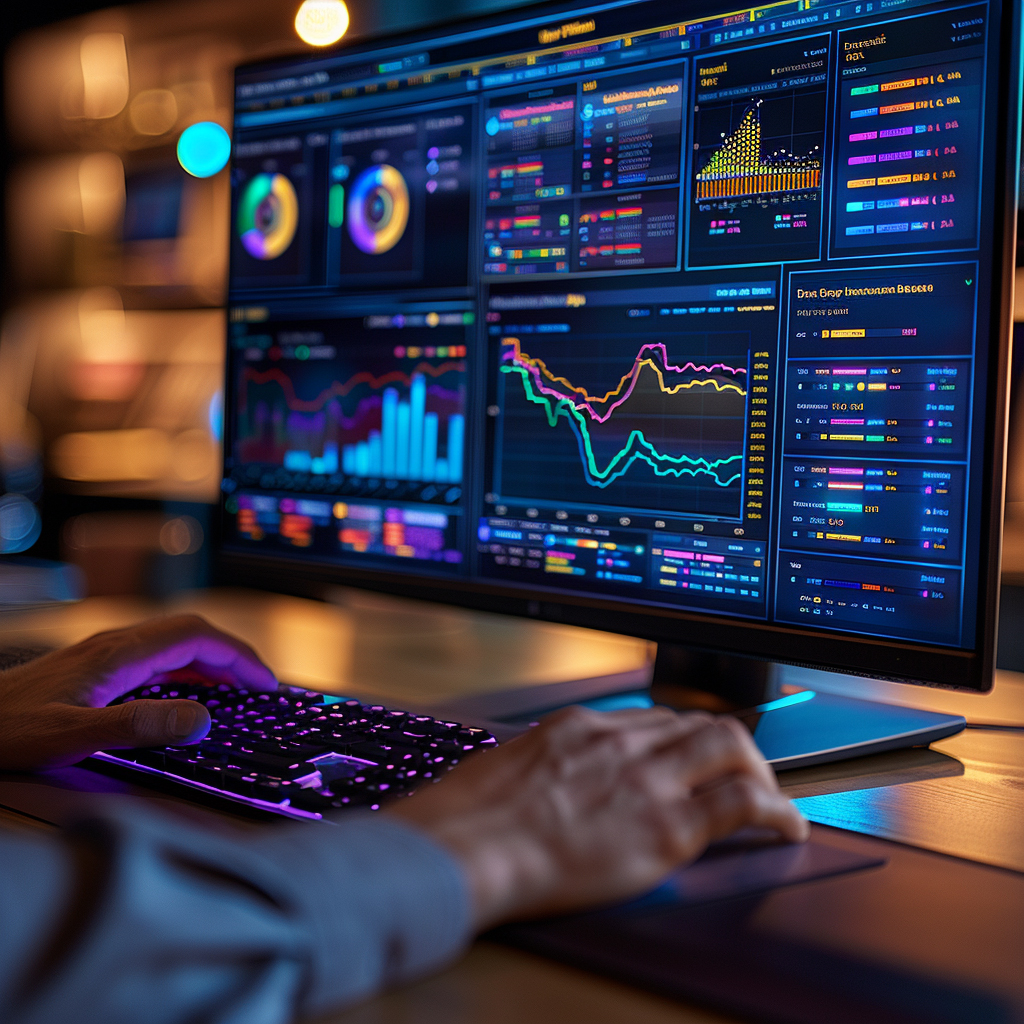
By incorporating AI, RPA bots gain predictive analytics capabilities, enabling them to anticipate future trends and outcomes based on historical data. This allows organizations to optimize processes proactively, such as adjusting supply chain operations before anticipated changes in demand, enhancing overall operational agility and efficiency.
5. Natural Language Processing (NLP)
AI-powered NLP enables RPA bots to understand and generate human-like text, allowing them to perform tasks that involve communicating with customers or employees.
-0.jpg)
AI-powered NLP transforms RPA bots into more sophisticated tools capable of understanding and generating human-like text. This enhancement allows RPA to automate complex tasks that involve interacting with customers or employees, such as customer support queries, scheduling meetings, or generating written reports.
6. Computer Vision
AI extends RPA capabilities to visual tasks, such as reading documents, scanning images, and interpreting videos, broadening the scope of automation.
-1.jpg)
With AI-driven computer vision, RPA can perform tasks that require visual understanding, such as reading content from images, processing scanned documents, and even interpreting videos for actionable insights. This application is particularly useful in areas like claims processing, quality control, and compliance monitoring.
7. Process Mining and Optimization
AI algorithms can analyze system logs to map and optimize business processes automatically, identifying inefficiencies that RPA can address.
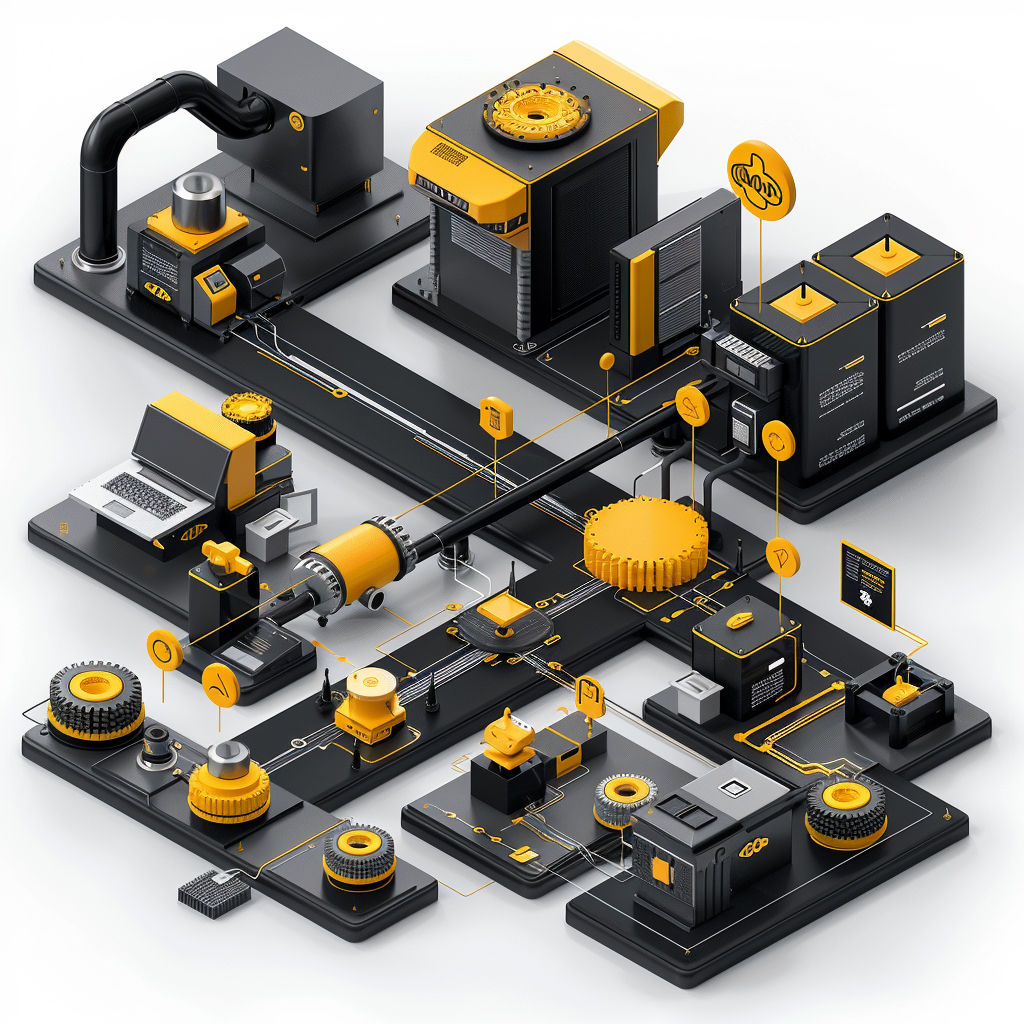
AI algorithms can automatically analyze system logs to map out and optimize business processes. This process mining helps identify inefficiencies and areas where RPA can be applied to improve speed, reduce costs, and eliminate human errors. Furthermore, continual analysis allows for ongoing refinement of processes, making operations smoother and more efficient.
8. Error Reduction
AI enhances the accuracy of RPA by learning from past mistakes and fine-tuning processes, significantly reducing the error rate in automated tasks.
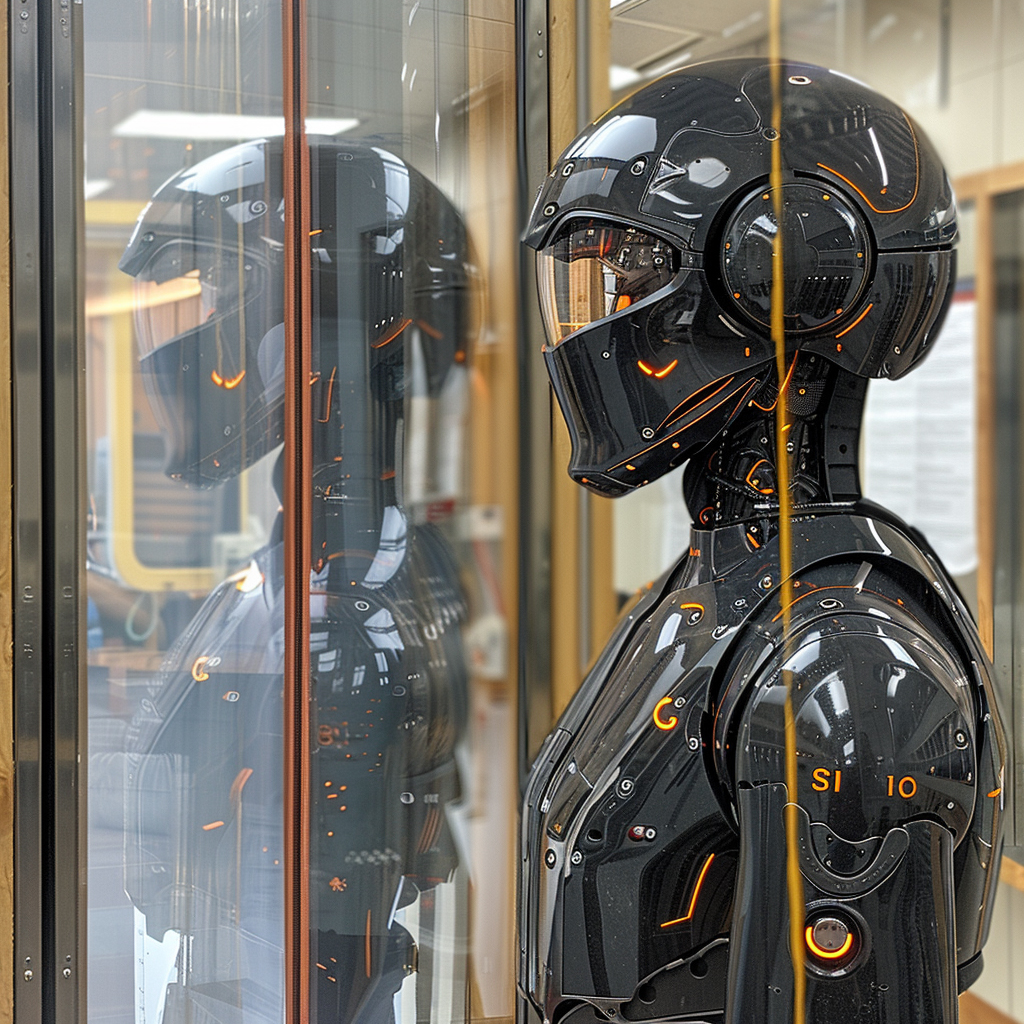
AI enhances RPA by learning from previous errors and fine-tuning the automation processes. This learning leads to a significant reduction in the error rate, increasing reliability, and trust in automated tasks. This is particularly critical in industries where precision is paramount, such as finance and healthcare.
9. Enhanced Customer Interaction
AI integrates with RPA to automate customer service tasks, using chatbots that can handle complex queries and provide personalized responses.
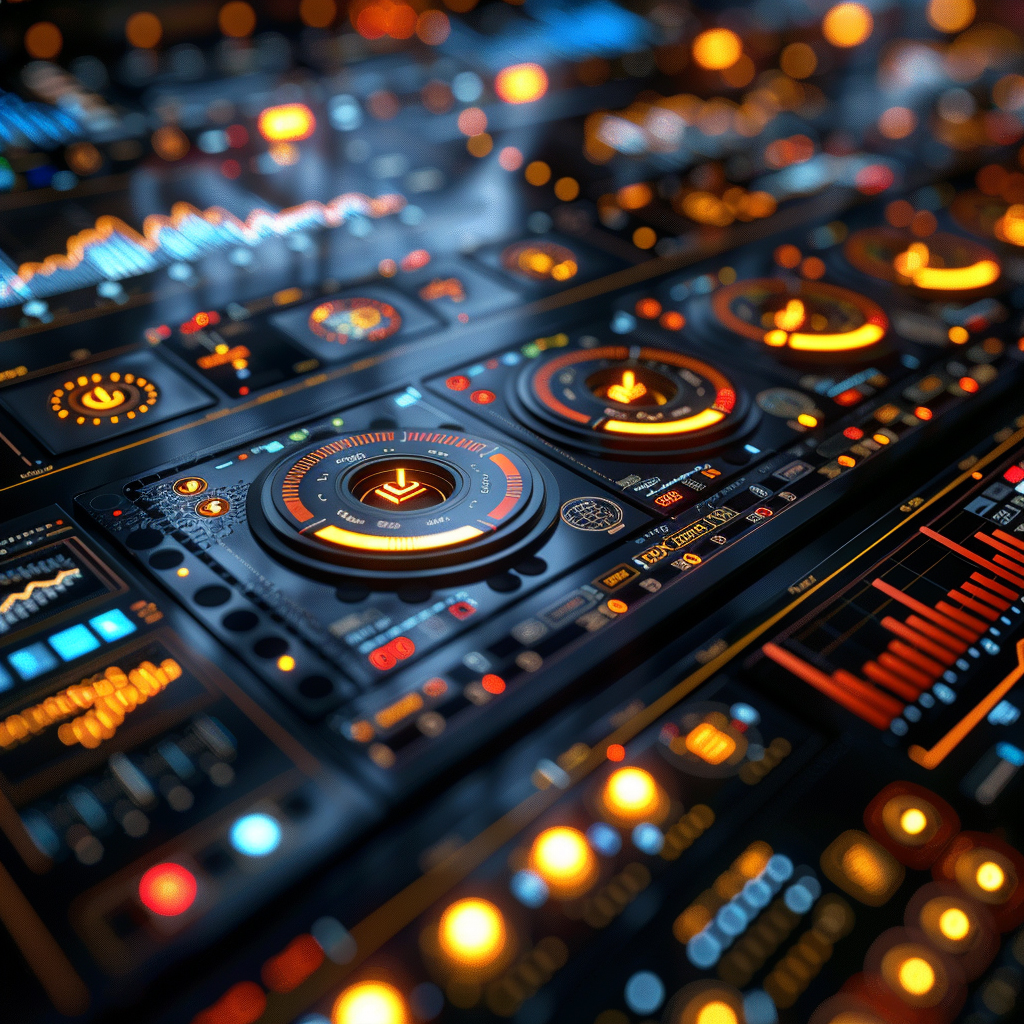
AI integration enables RPA bots to automate complex customer service tasks more effectively. AI-powered chatbots can handle a wider range of inquiries with the ability to provide more accurate and contextually relevant responses, improving customer satisfaction and freeing up human agents to handle more nuanced customer needs.
10. Real-Time Decision Making
AI enables RPA bots to make decisions in real-time, reacting to live data and events, which is crucial for applications such as dynamic pricing or inventory management.
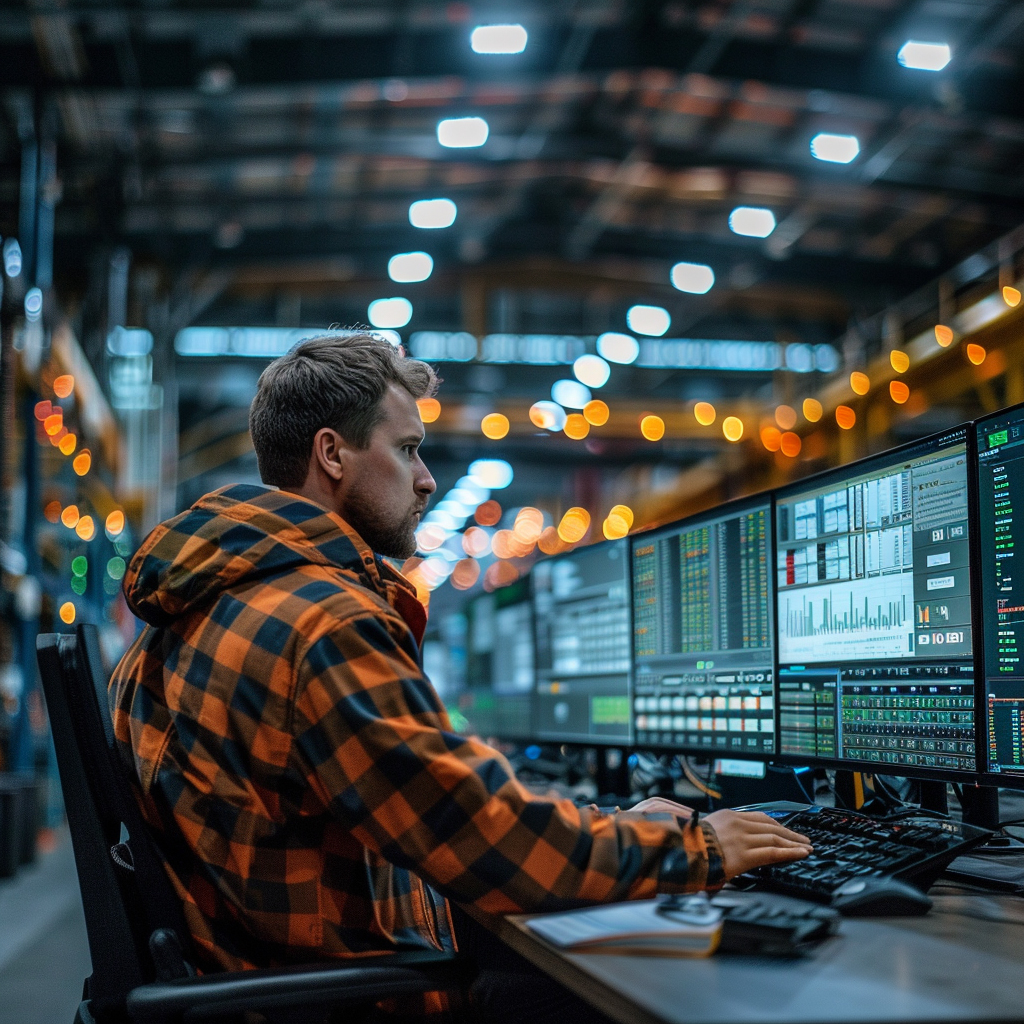
AI empowers RPA bots to make informed decisions in real-time, reacting instantly to live data and events. This capability is crucial in environments where conditions change rapidly, such as in dynamic pricing models in e-commerce or real-time inventory adjustments in logistics, allowing companies to stay responsive and competitive.