1. Demand Forecasting
AI analyzes historical sales data, market trends, and consumer behavior to predict future product demand, helping retailers optimize their stock levels and reduce overstock or stockouts.
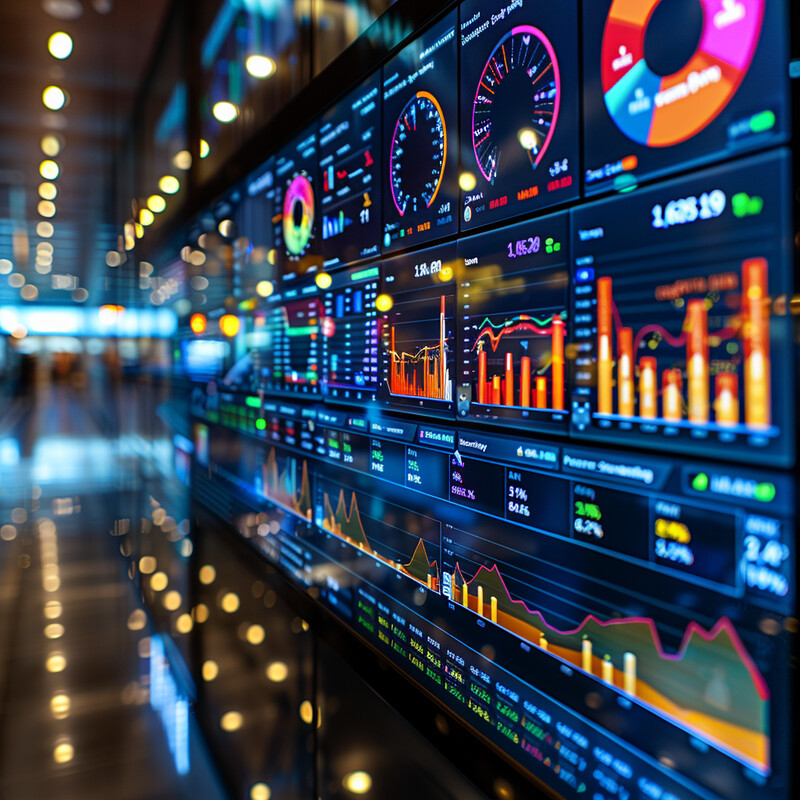
AI leverages complex algorithms to analyze historical sales data, consumer behavior trends, market conditions, and even external factors like weather or economic indicators to accurately predict future product demand. This sophisticated analysis helps retailers maintain optimal inventory levels, ensuring they have enough stock to meet demand without overstocking, which can lead to tied-up capital and increased storage costs.
2. Automated Replenishment
AI algorithms monitor stock levels in real-time and automatically trigger replenishment orders when inventory falls below predetermined thresholds, ensuring shelves are never empty.
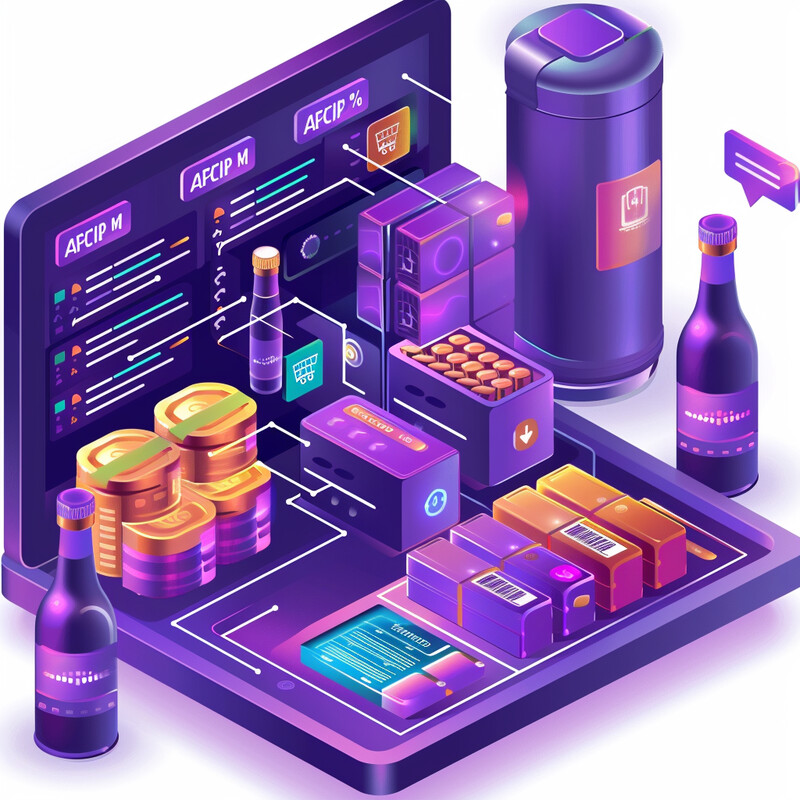
AI algorithms continuously monitor inventory levels and automatically place orders for replenishment when stocks drop below predefined thresholds. This automation ensures that inventory is always sufficient to meet consumer demand, reduces the burden on staff, and helps avoid potential sales losses due to out-of-stock situations.
3. Price Optimization
AI utilizes dynamic pricing strategies based on demand, competition, and inventory levels to adjust prices in real-time, maximizing revenue and reducing excess stock.
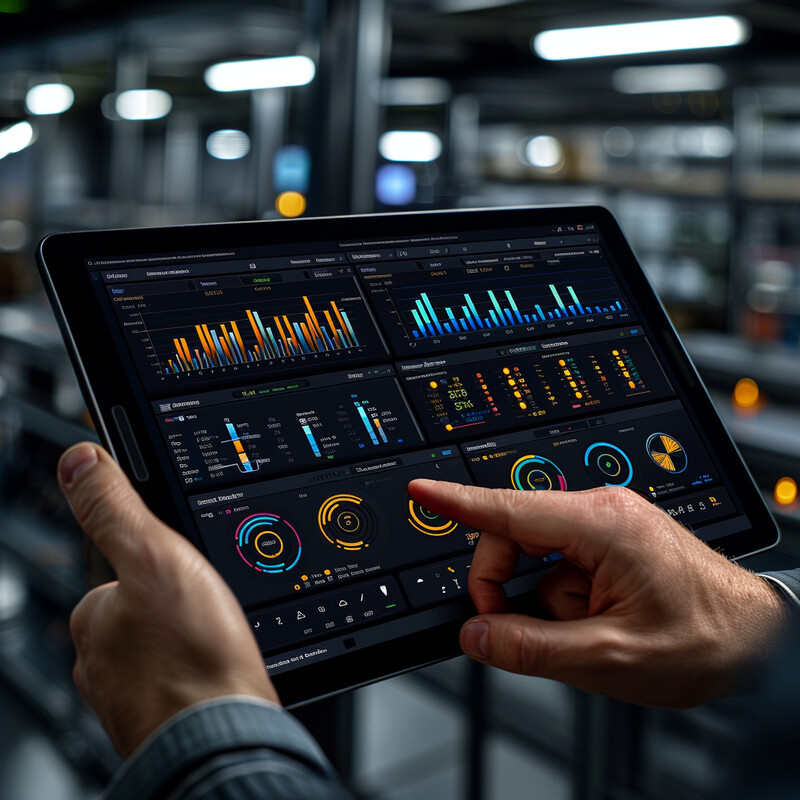
Utilizing dynamic pricing models, AI analyzes current demand, inventory levels, competitor pricing, and market trends to adjust prices in real-time. This strategy helps maximize profits by raising prices when demand is high and lowering them to accelerate sales when inventory is excessive, ensuring efficient stock turnover.
4. Supplier Performance Analysis
AI evaluates supplier reliability and performance by analyzing delivery times, product quality, and compliance rates, aiding retailers in choosing and managing suppliers more effectively.
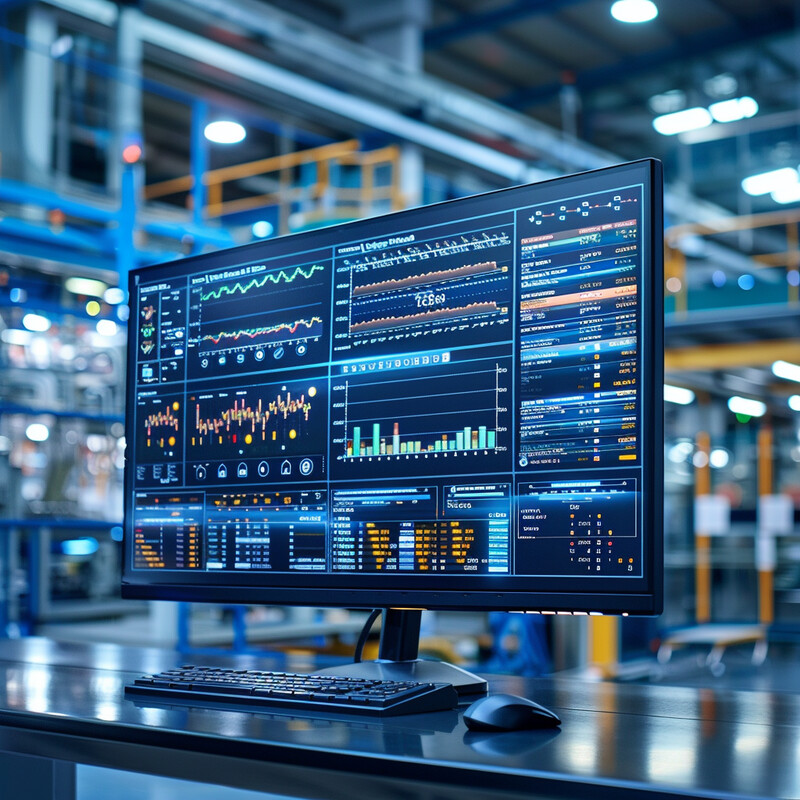
AI evaluates suppliers based on various performance metrics such as delivery times, quality of goods, and adherence to contract terms. This data-driven analysis assists retailers in selecting reliable suppliers and managing supply chain relationships more effectively, thereby reducing potential disruptions and maintaining quality standards.
5. Product Placement Optimization
AI analyzes in-store traffic patterns and purchasing data to determine the optimal placement of products within a store, enhancing visibility and sales potential.
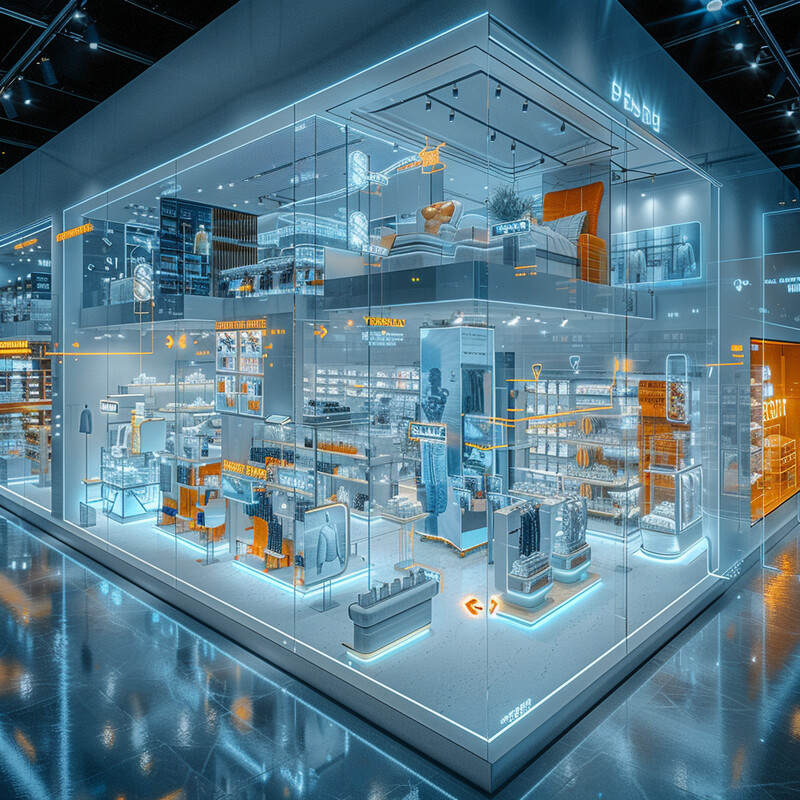
By analyzing data on in-store traffic and sales, AI determines the most effective placement of products within a store. This optimization enhances product visibility and accessibility, potentially increasing impulse purchases and overall sales by positioning items where consumers are most likely to notice them.
6. Loss Prevention
AI monitors and analyzes transactional data and video feeds to identify patterns that may indicate theft or fraud, significantly reducing shrinkage.
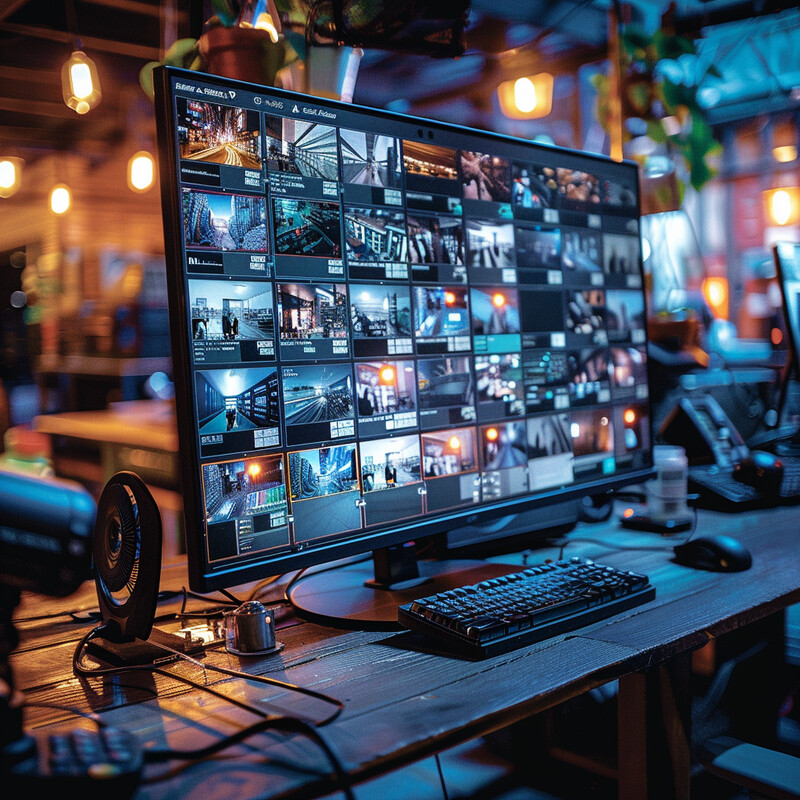
AI systems enhance loss prevention by integrating and analyzing data from POS systems and in-store video surveillance. These systems can detect unusual patterns that may indicate shoplifting or employee theft, enabling timely interventions and reducing losses due to fraud.
7. Waste Reduction in Perishables
AI predicts the shelf life of perishable goods and optimizes their prices or promotions to ensure they are sold before expiring, minimizing waste.
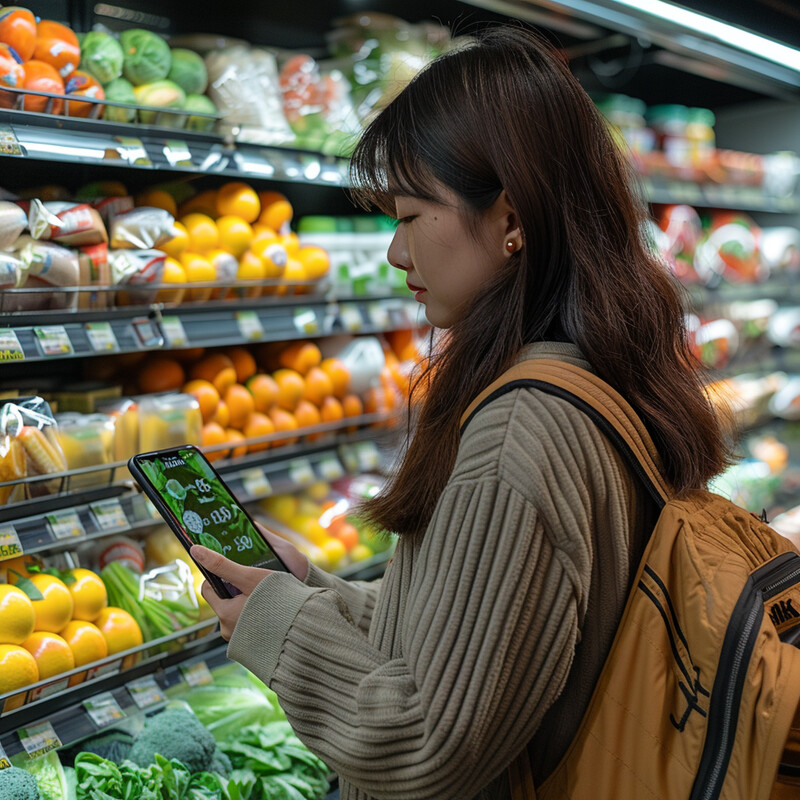
AI predicts the shelf life of perishable goods and optimizes pricing strategies to sell products before they expire. For instance, AI can suggest promotional discounts for items approaching their sell-by date, reducing waste and salvaging revenue that would otherwise be lost.
8. Customer Insights and Personalization
AI tracks customer preferences and purchasing habits, allowing retailers to tailor stock to meet local consumer demands and personalize marketing efforts.
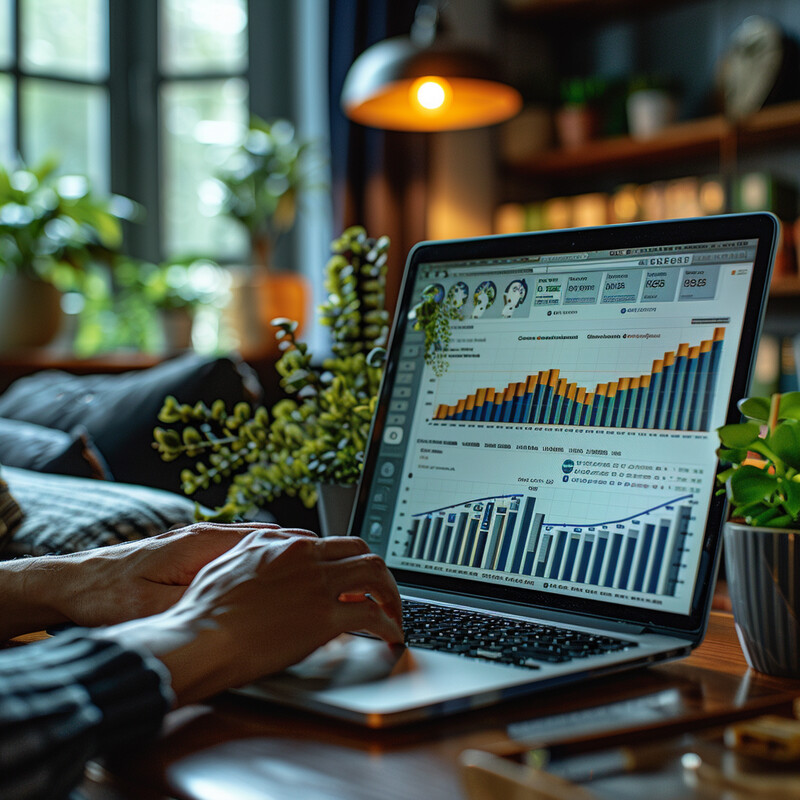
AI tools analyze customer data collected through loyalty programs and shopping habits to gain insights into consumer preferences. Retailers can use this information to stock products that better align with local consumer tastes and to create personalized marketing campaigns that resonate more effectively with their customer base.
9. Returns Management
AI helps manage and optimize the returns process by predicting return rates, analyzing return reasons, and determining the best course of action for returned items.
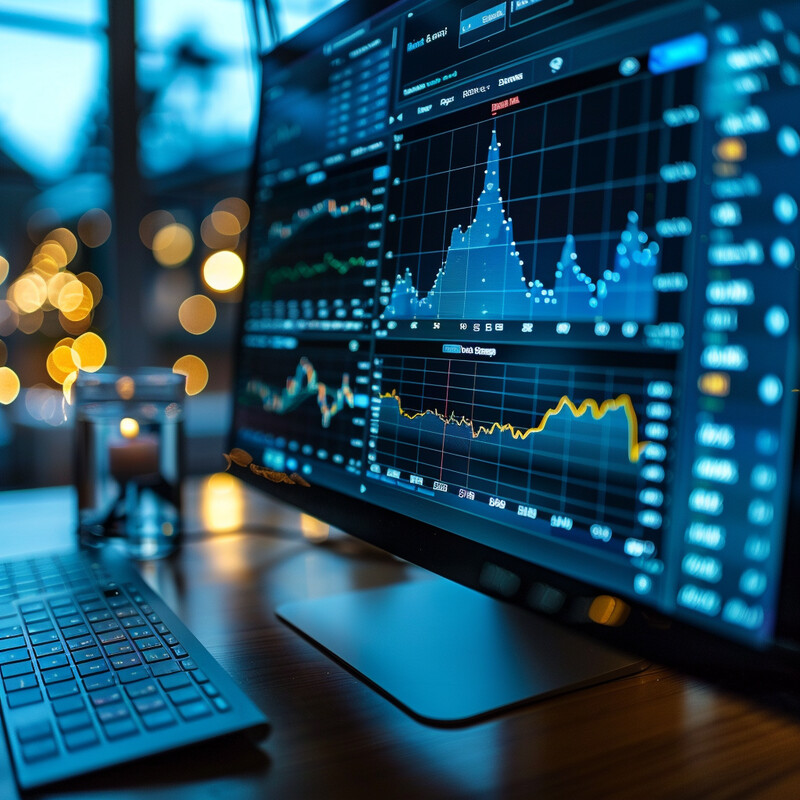
AI assists in managing product returns by analyzing return patterns and reasons. It predicts which items are likely to be returned and why, allowing retailers to address underlying issues. AI can also recommend whether returned items should be restocked, discounted, or written off, optimizing the handling of returns.
10. Integrated Omnichannel Experience
AI synchronizes inventory across all sales channels, including online and brick-and-mortar stores, providing a seamless shopping experience and efficient stock management.
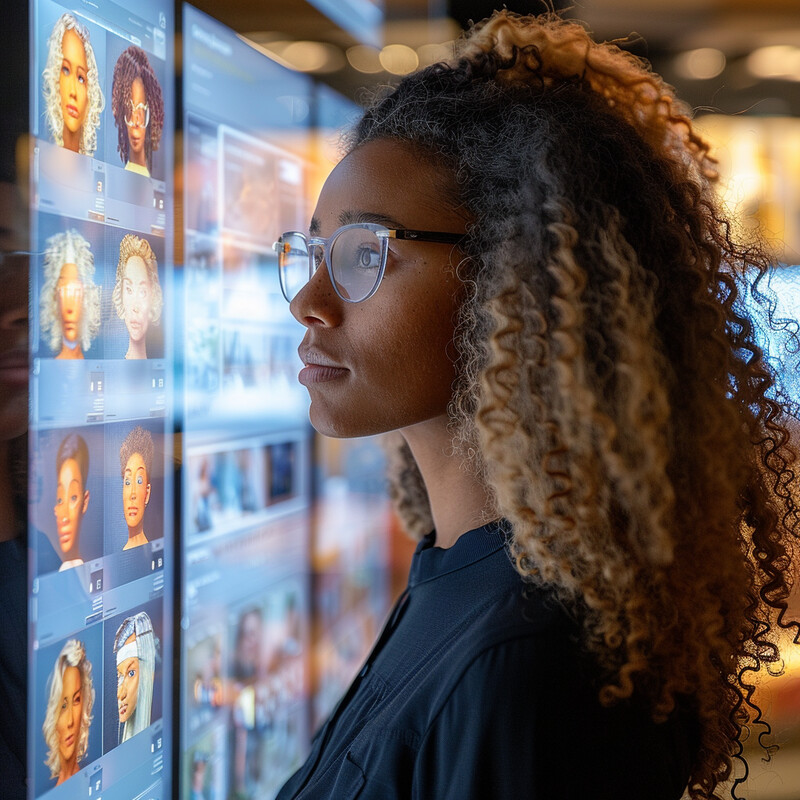
AI synchronizes inventory management across multiple sales channels, including online platforms and physical stores. This integration ensures consistent product availability, enabling a seamless customer experience whether shopping online or offline and improving overall inventory efficiency.