1. Content Personalization
AI analyzes listener preferences and behaviors to personalize content feeds, suggesting podcasts and radio shows tailored to individual tastes.
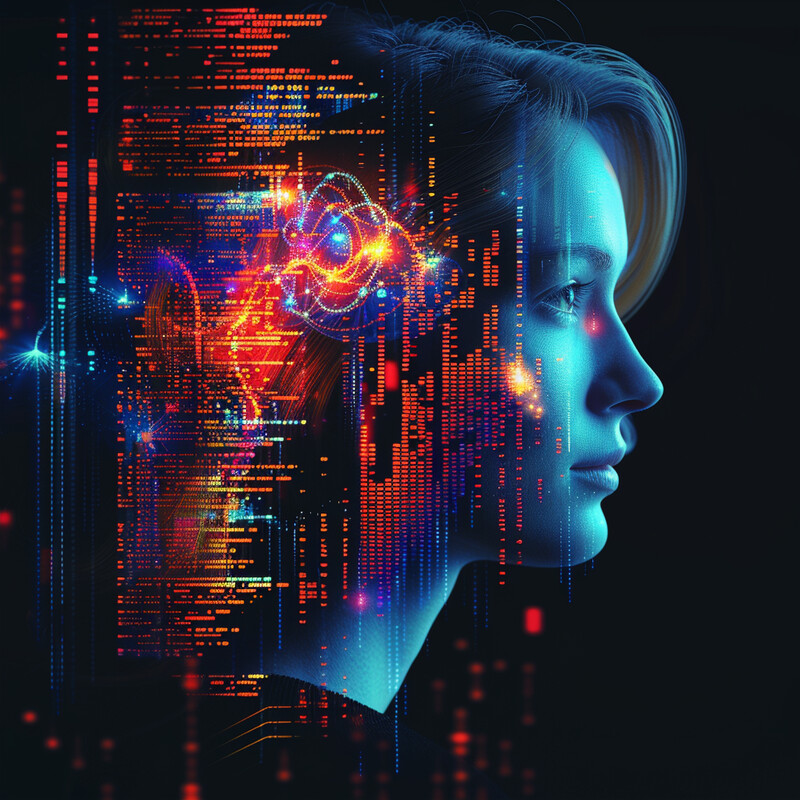
AI leverages listener data such as previous episodes played, interaction rates, and preferences to personalize content recommendations. By analyzing these behaviors, AI can curate podcast playlists and radio shows that align with individual tastes, significantly enhancing listener satisfaction and retention.
2. Speech-to-Text Transcription
AI automates the transcription of spoken content, making it easier to produce written records of broadcasts and podcasts for accessibility and indexing.
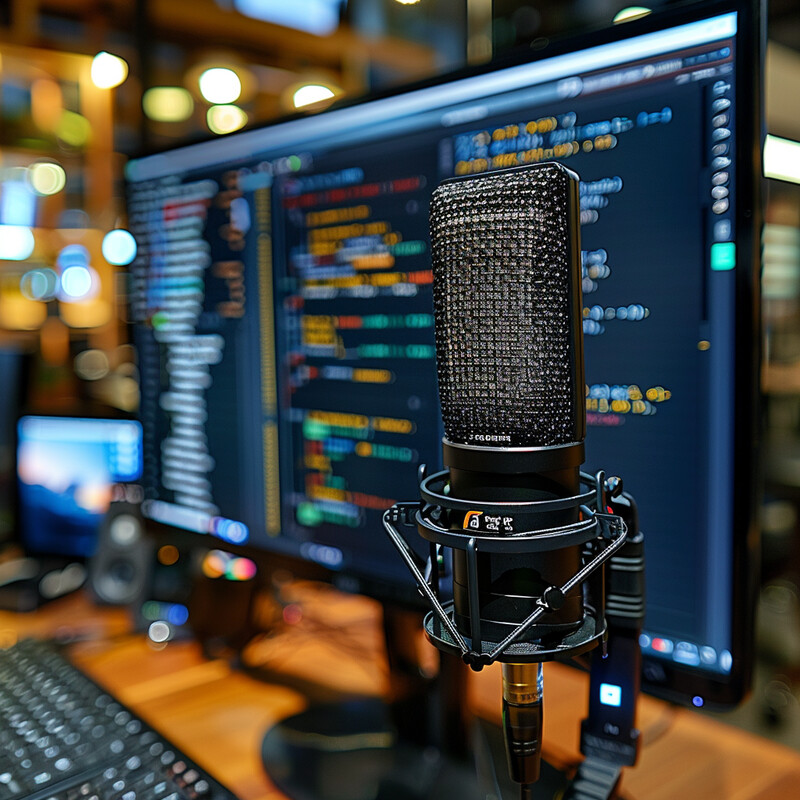
AI-powered speech-to-text technology automatically transcribes audio content from podcasts and radio broadcasts. This transcription is invaluable for creating searchable content, improving accessibility for the hearing impaired, and allowing producers to easily repurpose spoken content into blogs, articles, or social media posts.
3. Audio Quality Enhancement
AI improves audio quality by filtering out background noise and normalizing volume levels, ensuring clear and professional sound across various listening environments.
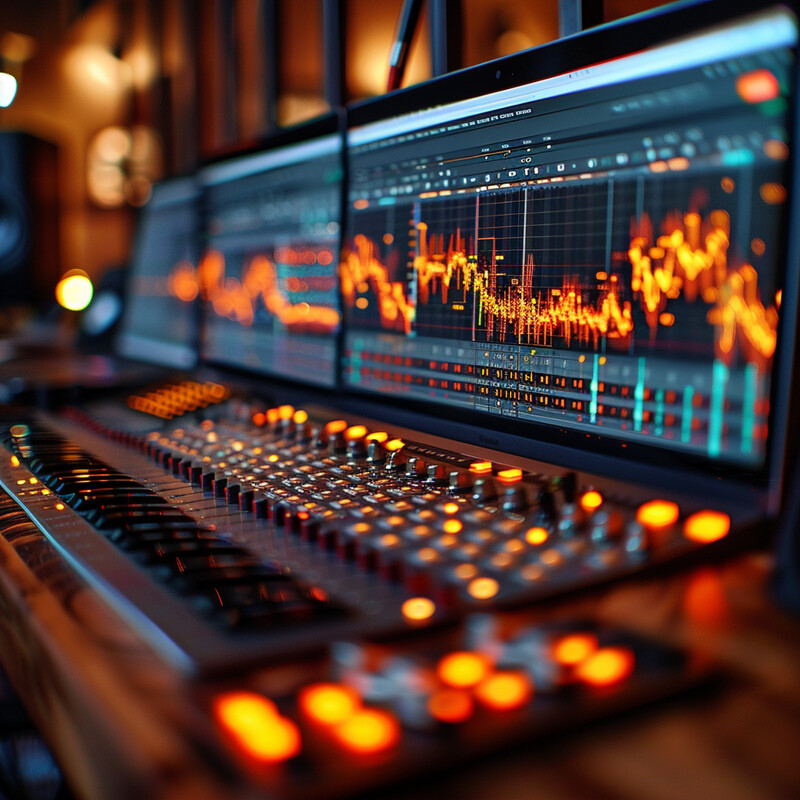
AI algorithms enhance audio quality by automatically reducing background noise, balancing sound levels, and improving clarity. This ensures that the audio is consistently clear, regardless of the recording environment, which is crucial for maintaining professional quality standards in podcast and radio production.
4. Automated Editing
AI streamlines the editing process by automatically cutting out silences, ums, and ahs, and even composing episodes from different audio clips based on predefined rules.
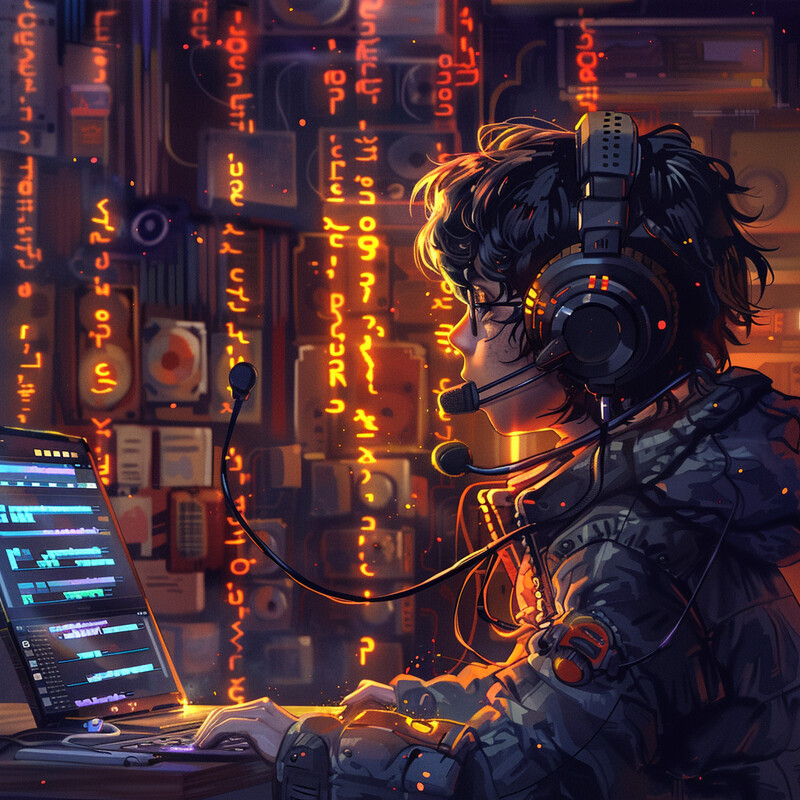
AI simplifies the editing process by automatically trimming silences and removing filler words like "uhm" and "ah." It can also seamlessly piece together different segments of audio to produce a cohesive episode based on set parameters, reducing the time and effort required in post-production.
5. Advertisement Insertion
AI optimizes ad placements within podcasts and radio shows by analyzing listener data to determine the most effective times for ad breaks that maximize listener retention and revenue.
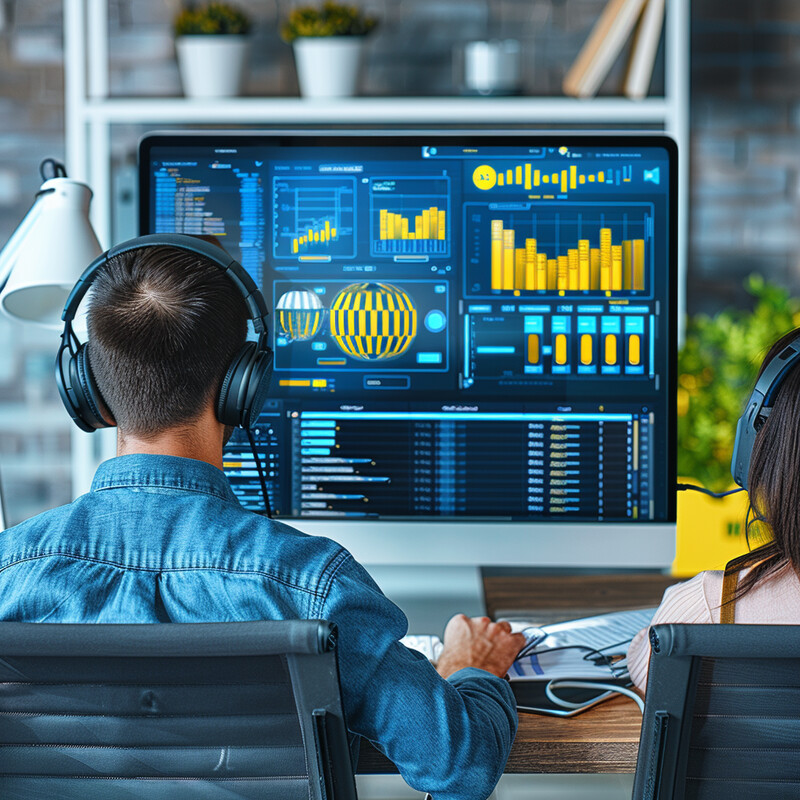
AI optimizes advertisement insertion by analyzing listener engagement patterns and preferences to strategically place ads where they are likely to have the most impact. This targeted placement helps maximize ad revenue without disrupting the listener experience, ensuring ads are as effective and unobtrusive as possible.
6. Voice Synthesis
AI generates synthetic voices that can read scripts or provide commentary, offering a cost-effective solution for content production and enabling new creative possibilities.
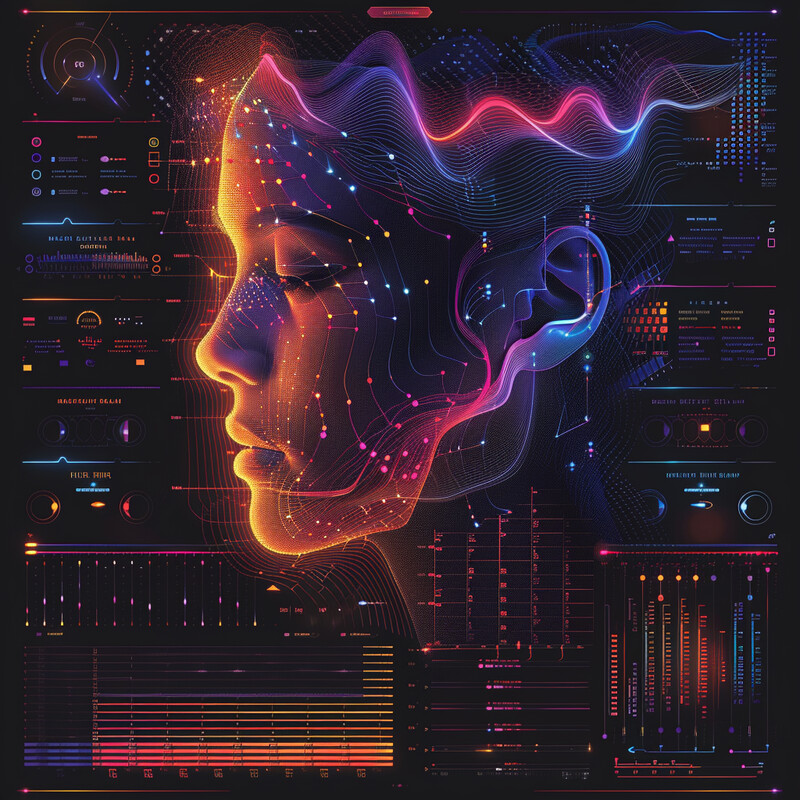
AI-generated synthetic voices can perform various speaking roles, from reading news to acting in audio dramas. This technology provides a cost-effective alternative to hiring voice actors for every piece of content and allows for creative experimentation with different vocal tones and styles.
7. Music Recommendation and Mixing
AI helps select and mix background music based on the tone and content of the show, enhancing the auditory experience without manual intervention.
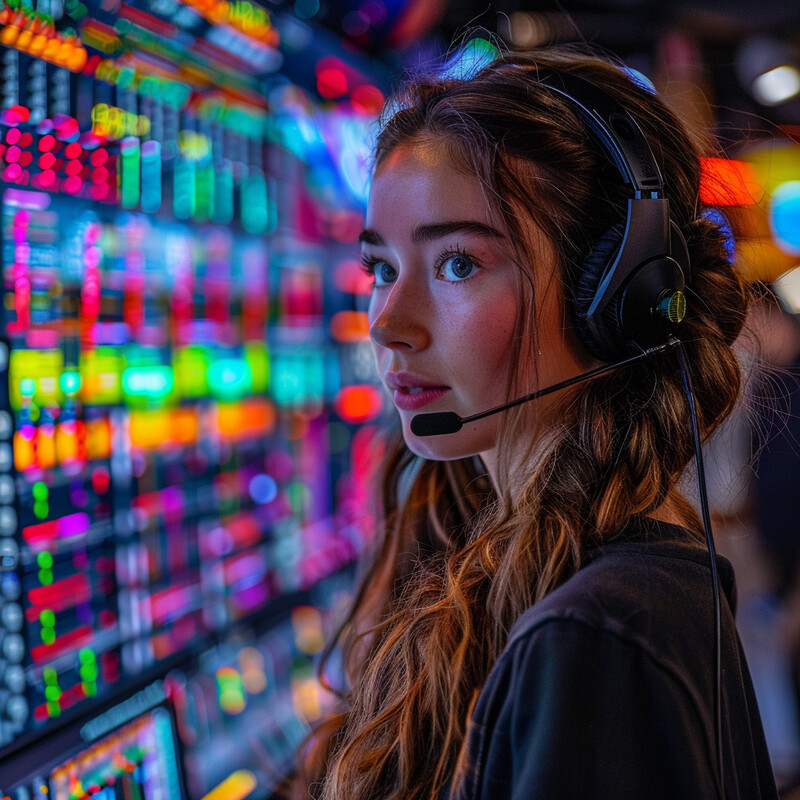
AI assists in selecting and mixing background music that complements the mood and theme of the audio content. By analyzing the tone and pace of spoken content, AI can recommend music tracks from a library that enhance the overall auditory experience of the episode.
8. Predictive Analytics for Trending Topics
AI predicts and tracks trending topics by analyzing social media and listener data, helping producers create content that is timely and relevant to current events.
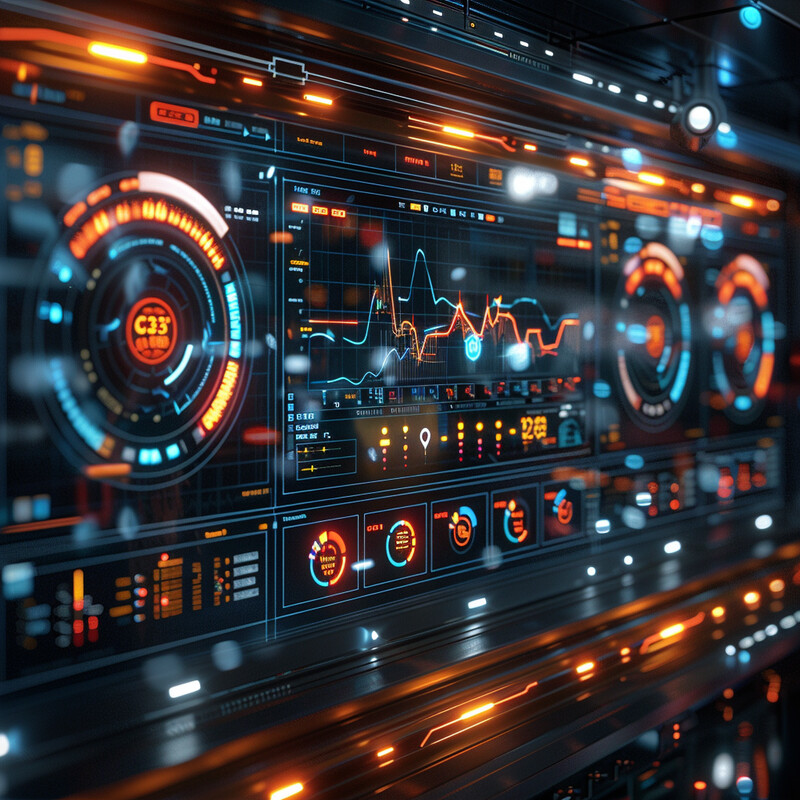
AI uses predictive analytics to identify and track trending topics across various platforms, including social media and web searches. This insight allows content creators to produce relevant and timely content that captures listener interest and capitalizes on current trends.
9. Listener Engagement Analysis
AI tools analyze listener engagement metrics, such as play rates, skip rates, and completion rates, to provide insights into what content resonates best with audiences.
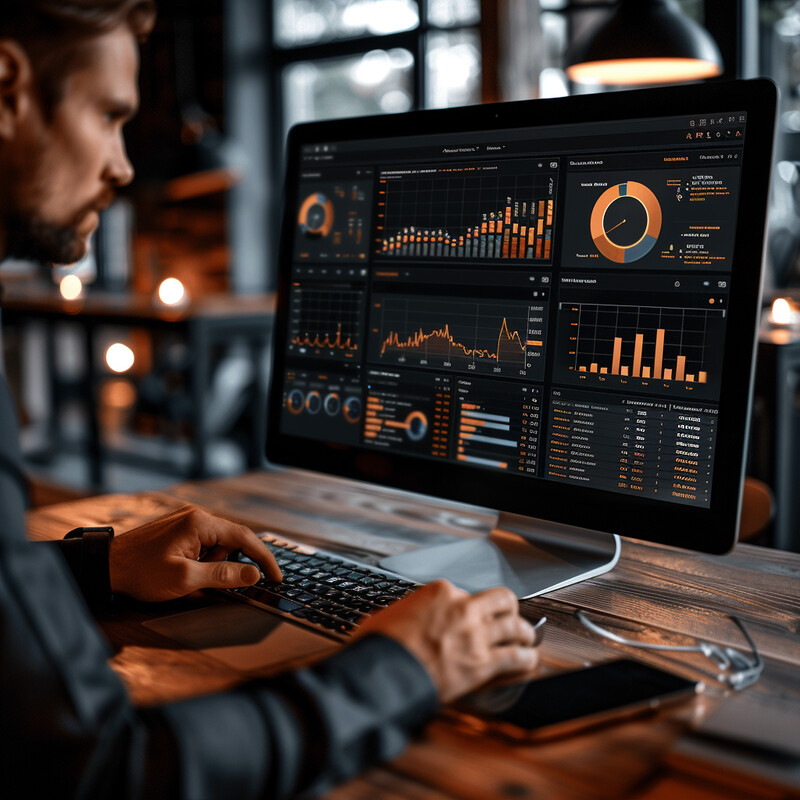
AI tools analyze detailed engagement metrics such as how long listeners stay tuned, what segments they skip, and where they stop listening. This data helps producers understand what content works and what doesn’t, guiding content strategy and improvements to keep audiences engaged.
10. Language Translation and Dubbing
AI provides real-time translation and dubbing capabilities, allowing podcasts and radio shows to reach a global audience by overcoming language barriers.
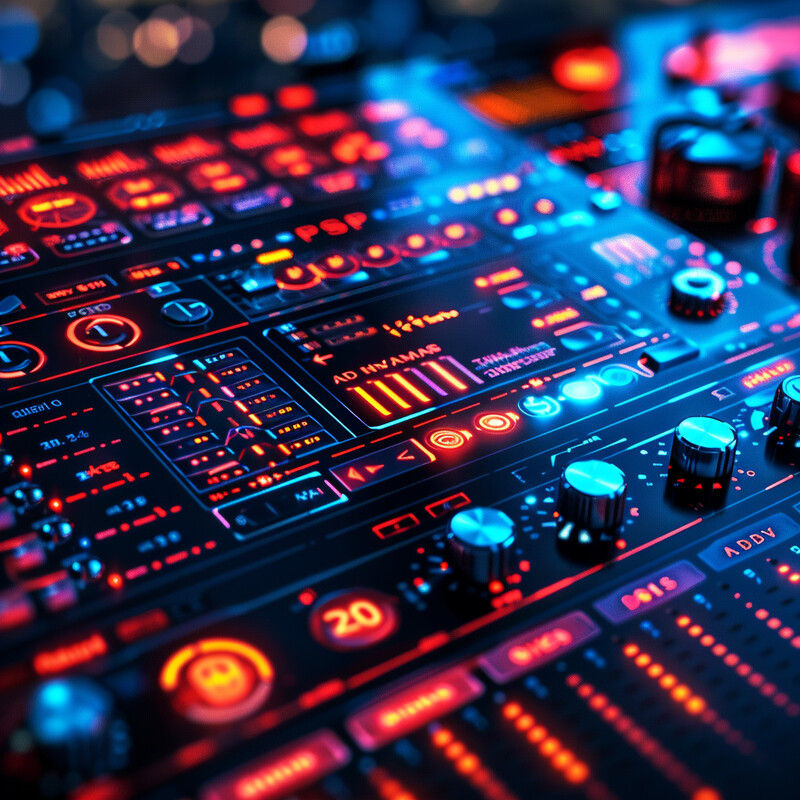
AI enables real-time translation and dubbing of audio content, making podcasts and radio shows accessible to a global audience. This technology not only breaks down language barriers but also expands the potential listener base, allowing content creators to reach international markets.