1. Error Correction
AI algorithms are being used to predict and correct quantum errors in quantum bits (qubits), which are inherently unstable and prone to errors due to quantum decoherence and other quantum noise.
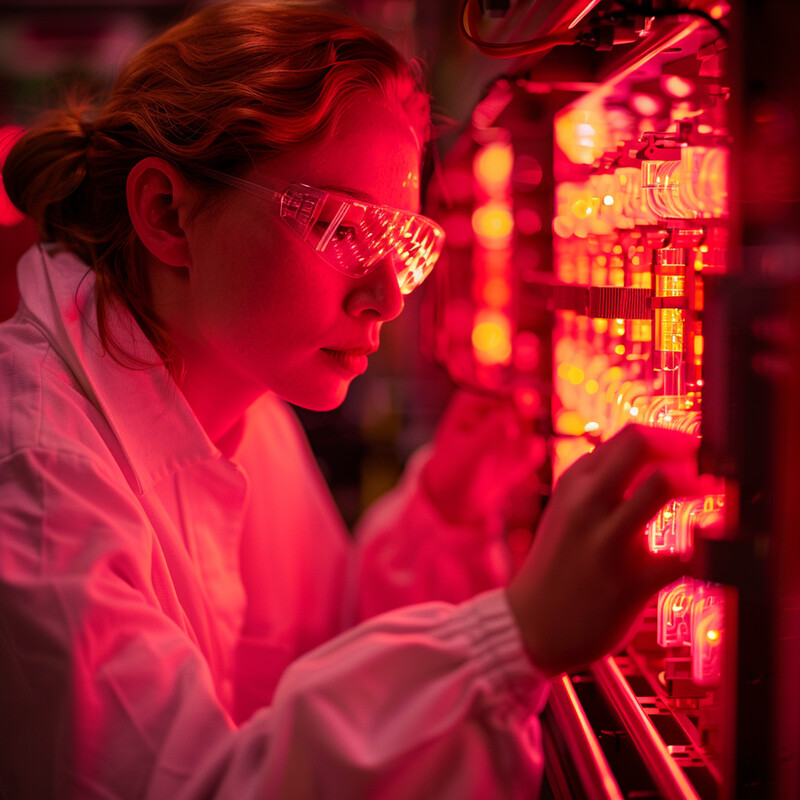
AI algorithms are crucial in identifying and correcting errors in quantum bits (qubits), which are significantly more susceptible to errors than classical bits due to quantum decoherence and interference. By predicting error patterns and their corrections, AI improves the reliability of quantum computations, maintaining the integrity of complex quantum operations which are essential for practical quantum computing applications.
2. Algorithm Optimization
AI helps optimize quantum algorithms, making them more efficient and effective by automating the design process to adapt to different quantum architectures and reduce computational complexity.
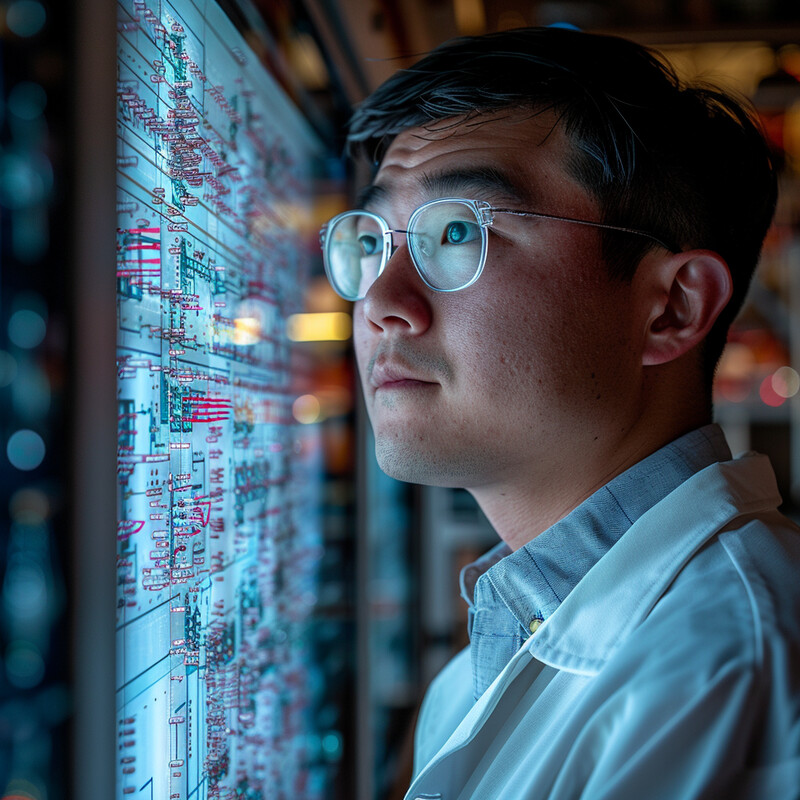
AI optimizes quantum algorithms by automating the design process, which involves selecting the best quantum gates and sequences to perform specific computations efficiently. This optimization is tailored to different quantum architectures, reducing the computational resources required and speeding up quantum calculations, thereby enhancing the performance and scalability of quantum systems.
3. Hardware Design
AI aids in the design and simulation of quantum computing hardware, predicting optimal configurations and materials that can enhance qubit coherence and scalability.
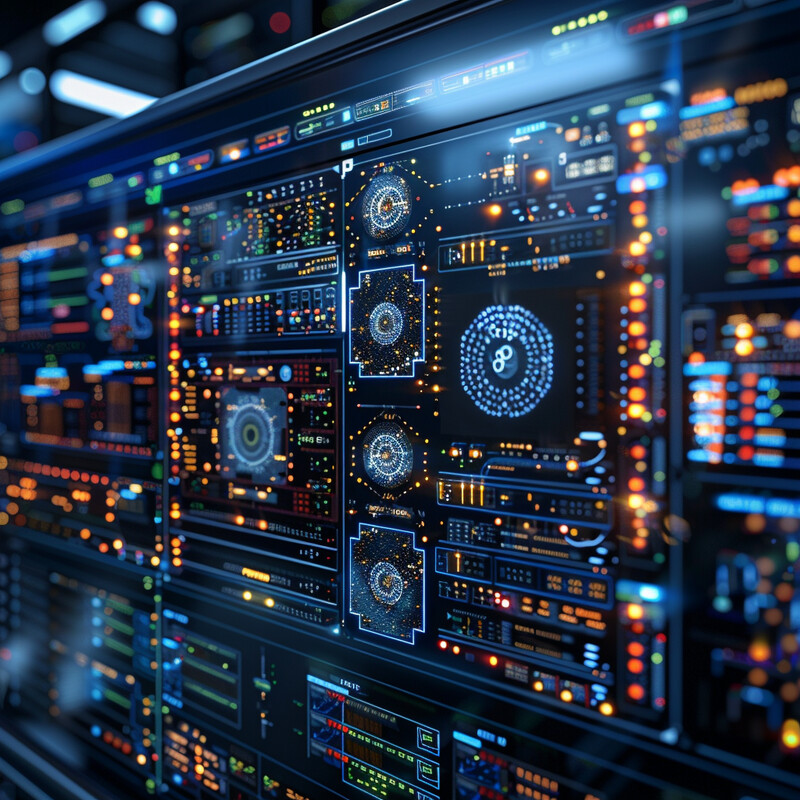
In quantum computing, the stability and coherence of qubits are paramount. AI aids in the hardware design process by simulating different architectural configurations and materials to determine those that enhance qubit performance and scalability. This involves predicting how changes in design affect qubit coherence times and the overall stability of the quantum system.
4. Gate Optimization
AI is used to optimize the quantum gates that control qubit behavior, improving the precision and reliability of quantum operations necessary for complex computations.
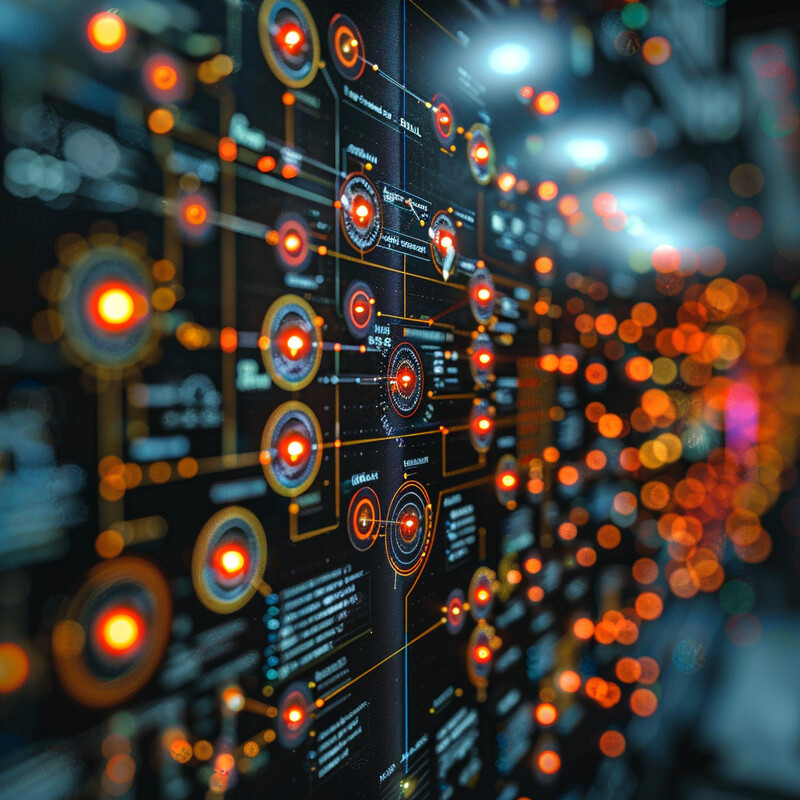
AI is used to fine-tune the operations of quantum gates, which control the behavior of qubits during quantum computations. By optimizing these gates, AI ensures that they execute quantum operations with high precision, crucial for achieving accurate results in quantum algorithms and minimizing computational errors.
5. Quantum State Characterization
AI techniques are applied to analyze and characterize quantum states, helping in the understanding and manipulation of qubits for better control in quantum computations.
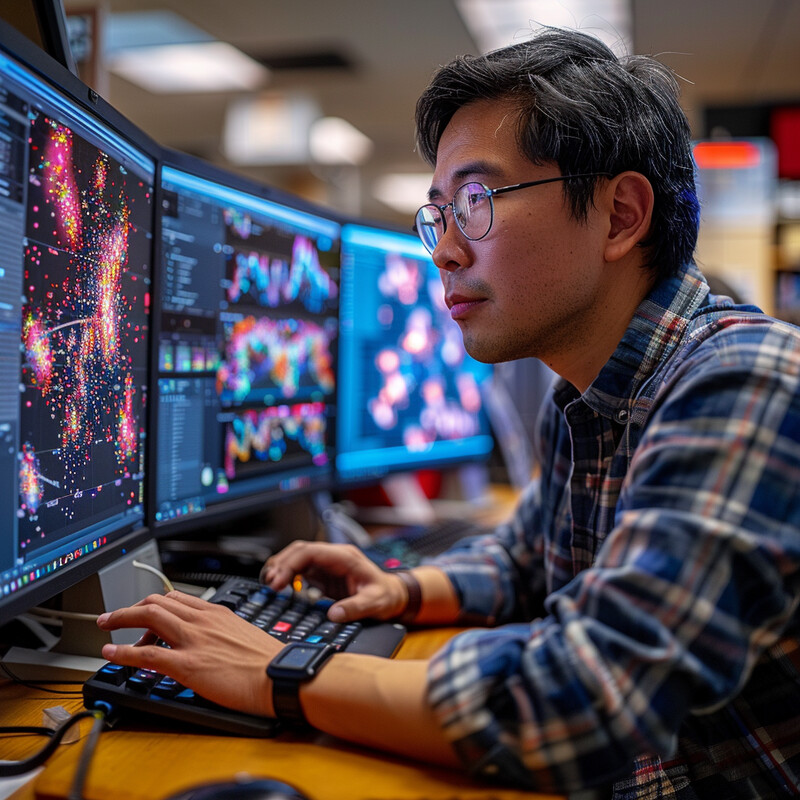
Characterizing quantum states accurately is fundamental for manipulating qubits effectively. AI applies advanced data analysis techniques to understand quantum states better, assisting researchers in controlling and utilizing these states for specific quantum computations, thereby improving the overall functionality of quantum computers.
6. Resource Management
AI manages resources in quantum computers, allocating qubits and quantum gates efficiently across different tasks to maximize the system's overall performance.
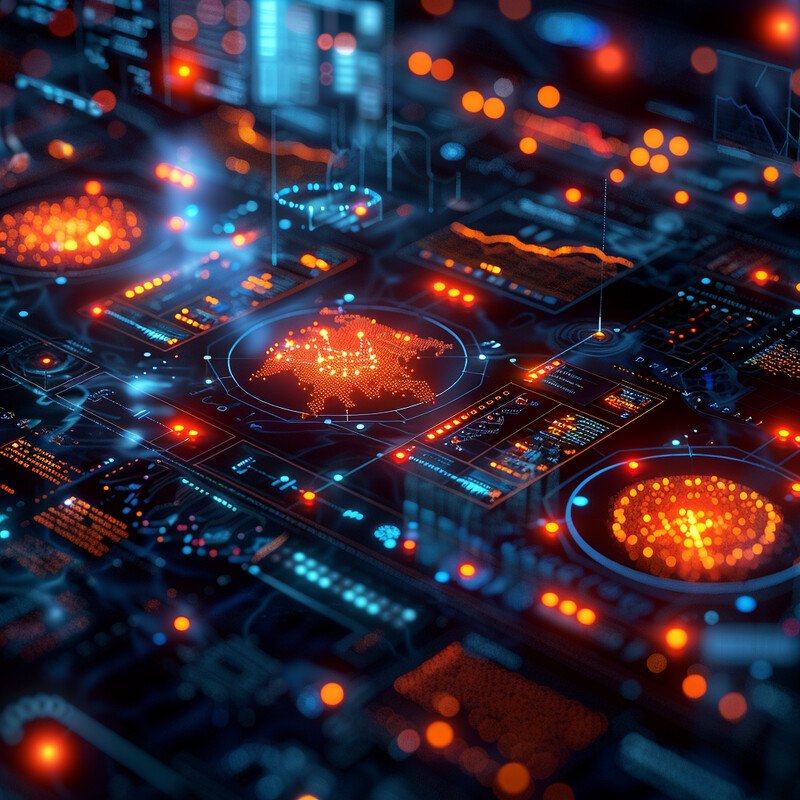
Efficient resource management is critical in quantum computing, where the number of qubits and quantum gates is still limited. AI manages these resources by allocating them optimally across various computational tasks, maximizing the utilization and efficiency of quantum computing systems.
7. System Calibration
AI continuously calibrates quantum computing systems to adjust for drifts in qubit properties and ensure that quantum gates function correctly, maintaining system accuracy over time.
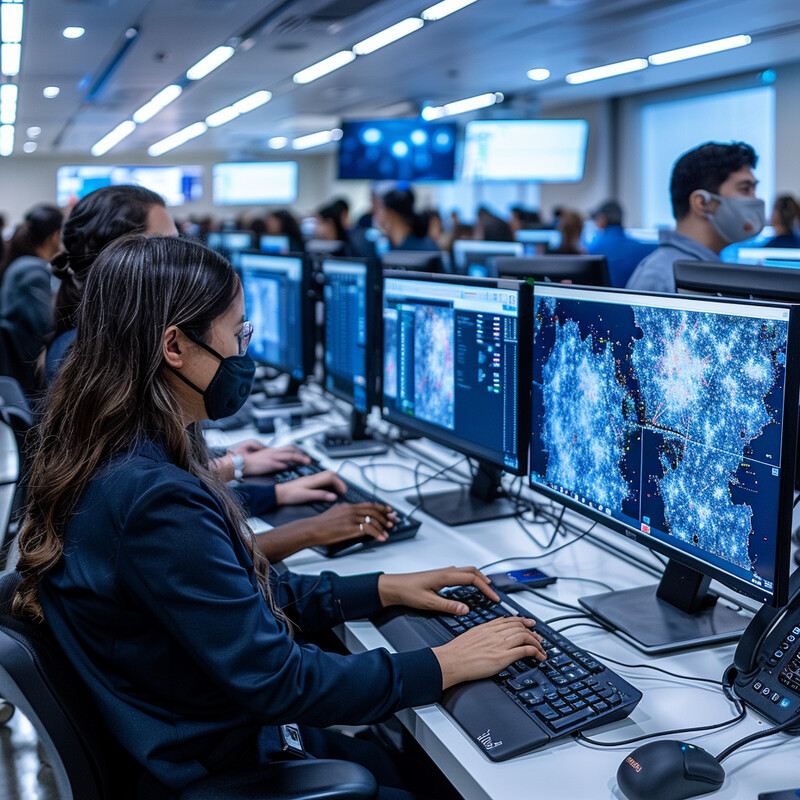
AI systems continuously monitor and calibrate quantum computing machinery to compensate for any drifts or changes in qubit properties over time. This ongoing calibration is essential to ensure that quantum operations are performed accurately, maintaining the system’s reliability and performance.
8. Temperature Control
AI monitors and adjusts the ultra-cold environments necessary for quantum computing, ensuring that temperature fluctuations do not interfere with qubit stability.
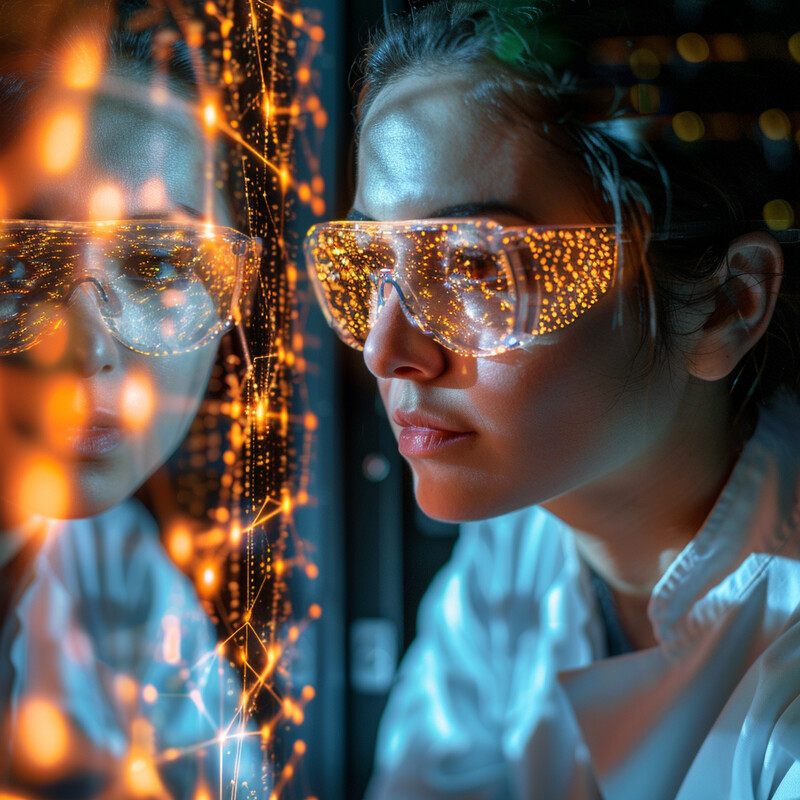
Maintaining ultra-cold temperatures is essential for the operation of many quantum computers, particularly those based on superconducting qubits. AI helps in monitoring and dynamically adjusting the cooling systems to prevent any temperature fluctuations that might affect the stability and coherence of qubits.
9. Quantum Simulation
I accelerates quantum simulations by predicting outcomes and refining models, allowing researchers to test and develop quantum algorithms more quickly and with better accuracy.
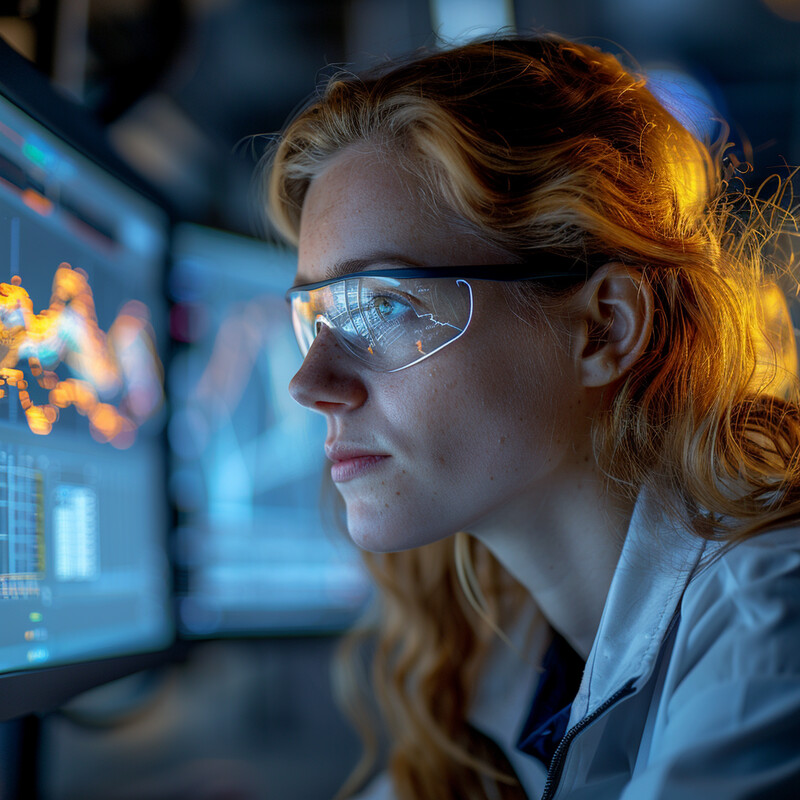
AI enhances quantum simulations by making them faster and more accurate. These simulations are crucial for testing and developing quantum algorithms before they are run on actual quantum machines. AI predicts outcomes and refines simulation models, reducing the time and computational power required to develop effective quantum algorithms.
10. Integration with Classical Systems
AI facilitates the integration of quantum and classical computing systems, enabling hybrid systems to operate efficiently and expanding the practical applications of quantum computing in real-world scenarios.
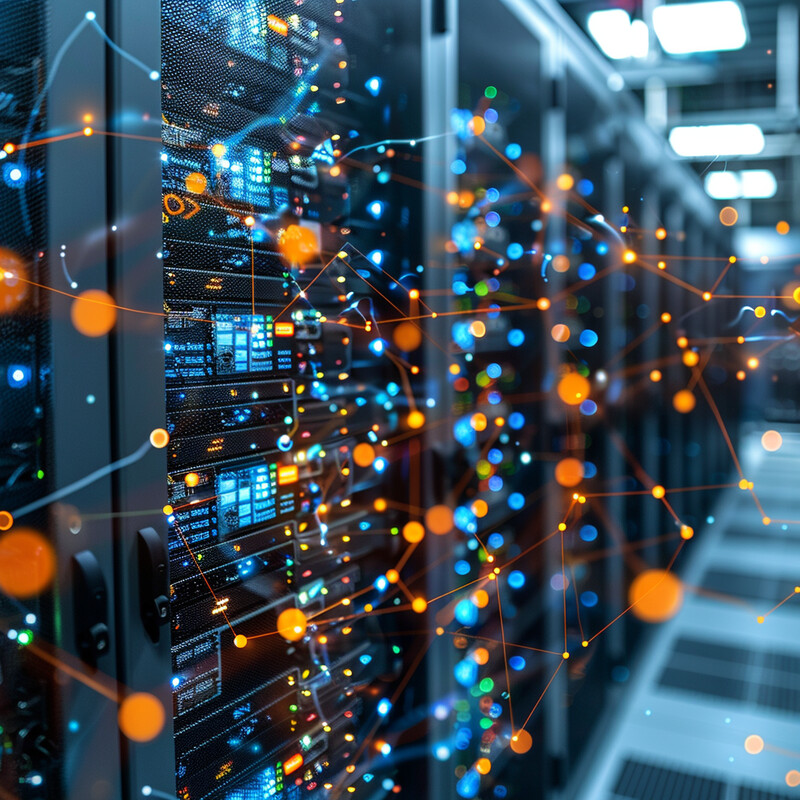
Integrating quantum and classical computing systems effectively is vital for the practical application of quantum technologies. AI facilitates this integration by managing the interaction between quantum and classical systems, ensuring that they work together seamlessly to solve complex computational problems more efficiently than could be achieved by either system alone.