1. Advanced Data Processing
AI algorithms can process and analyze massive datasets far more efficiently than traditional methods, identifying complex patterns and correlations that are not easily detectable by human analysts.
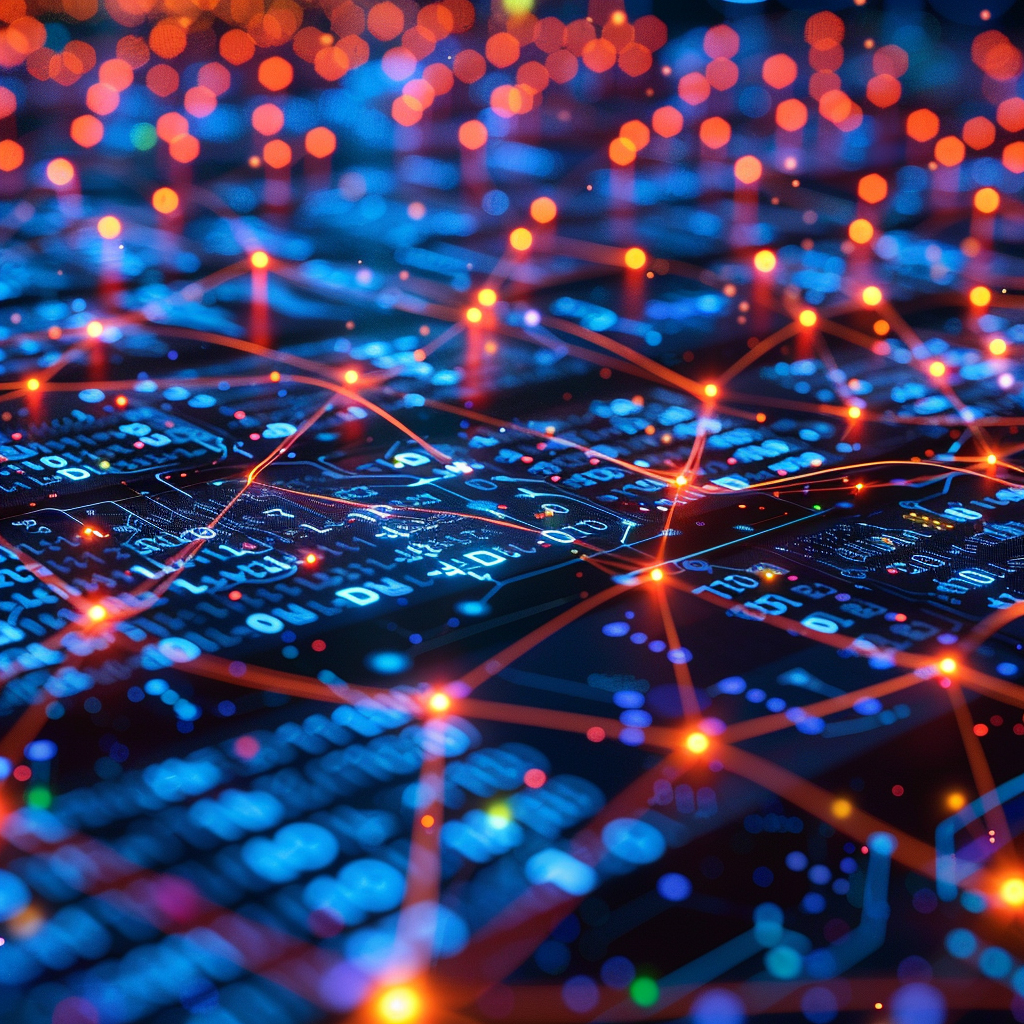
AI algorithms are designed to efficiently process vast amounts of data, handling complexities and volumes that are unmanageable for traditional analytics. These algorithms can detect intricate patterns and hidden correlations within the data, enabling more accurate predictions and insights. By automating data processing and analysis, AI significantly reduces the time and resources needed, allowing businesses to focus on strategy and implementation.
2. Real-Time Predictions
AI enables real-time data analysis, allowing businesses to make informed decisions quickly. This is particularly important in industries like finance or emergency services, where conditions can change rapidly.
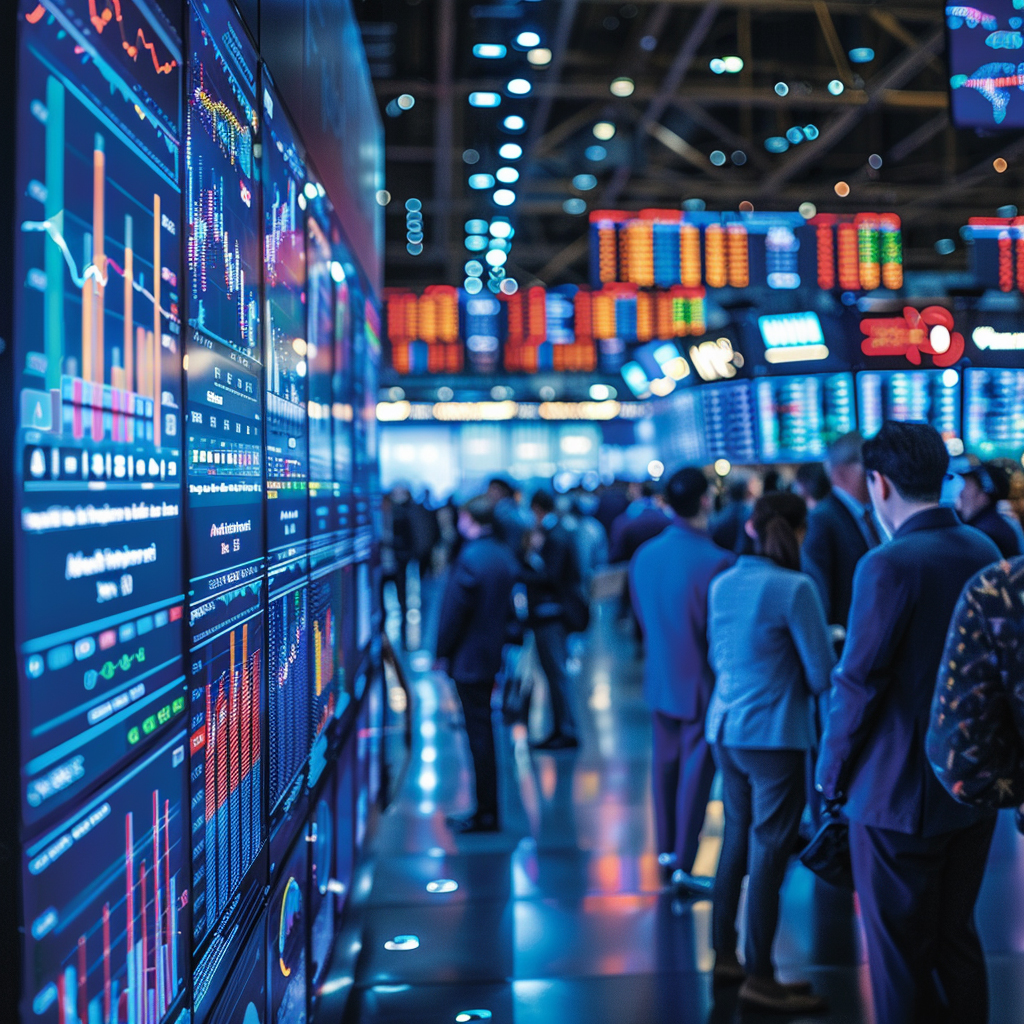
AI enhances predictive analytics by enabling real-time data processing and prediction capabilities. This is crucial in fast-paced environments like financial trading or emergency response, where being able to react instantly to changing conditions can make a significant difference. Real-time analytics powered by AI helps organizations act immediately on insights, providing a competitive edge by responding more swiftly to market or environmental changes.
3. Increased Accuracy
AI models, particularly deep learning, have significantly improved the accuracy of predictions by learning from large amounts of historical data and continuously adjusting as new data comes in.
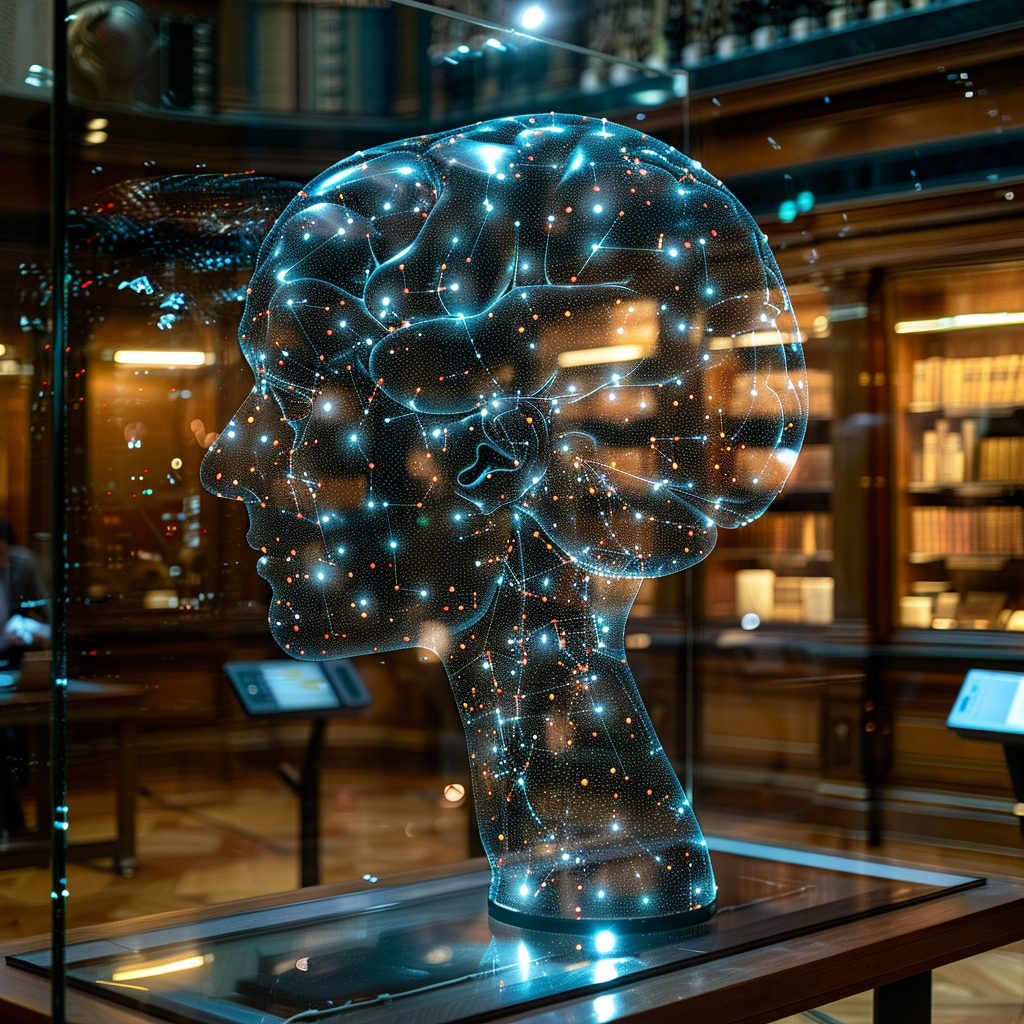
Deep learning and other advanced AI techniques have improved the accuracy of predictive models by learning from extensive historical datasets and continuously updating as new data becomes available. These models can understand complex relationships between variables that humans might overlook, leading to more reliable predictions and fewer false positives or negatives.
4. Automated Model Adjustment
AI systems can automatically update and refine their predictive models based on new data, ensuring that the predictions remain relevant and accurate over time without manual intervention.
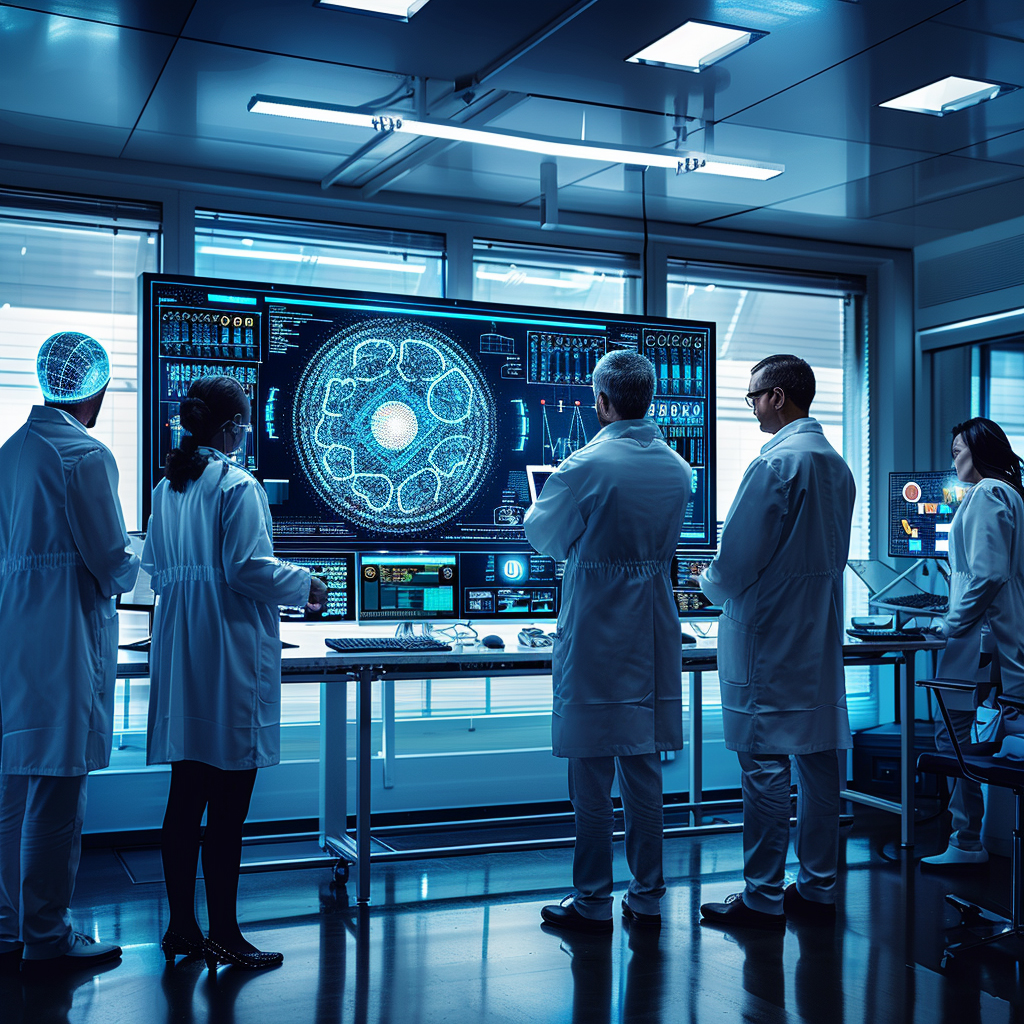
AI systems are equipped with the capability to automatically update and fine-tune their predictive models based on incoming data. This means that as conditions change or new trends emerge, the AI can adapt without requiring manual recalibration. This dynamic adjustment helps maintain the relevance and accuracy of predictive analytics over time, even in rapidly evolving industries.
5. Anomaly Detection
AI is highly effective at identifying outliers or anomalies in data that could indicate important trends or errors, such as fraudulent activity in banking transactions or potential failures in manufacturing processes.
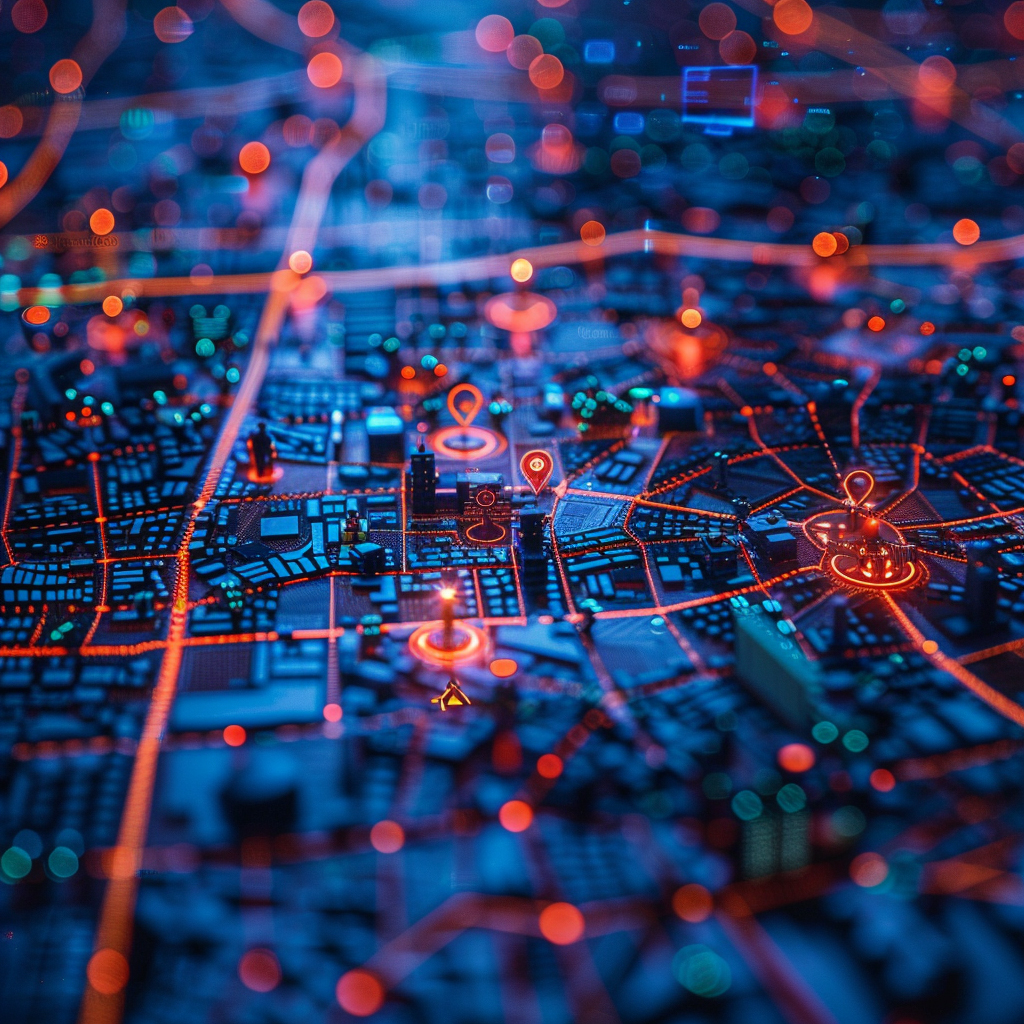
AI excels at identifying anomalies or outliers in data that might indicate critical insights, such as potential fraud in financial transactions or impending equipment failures in industrial settings. By detecting these anomalies early, AI enables organizations to take preemptive actions to mitigate risks, often saving substantial costs and enhancing security.
6. Enhanced Customer Insights
AI-driven predictive analytics can delve deeply into customer behavior patterns to forecast future buying behaviors, helping businesses tailor their marketing strategies and product offerings.
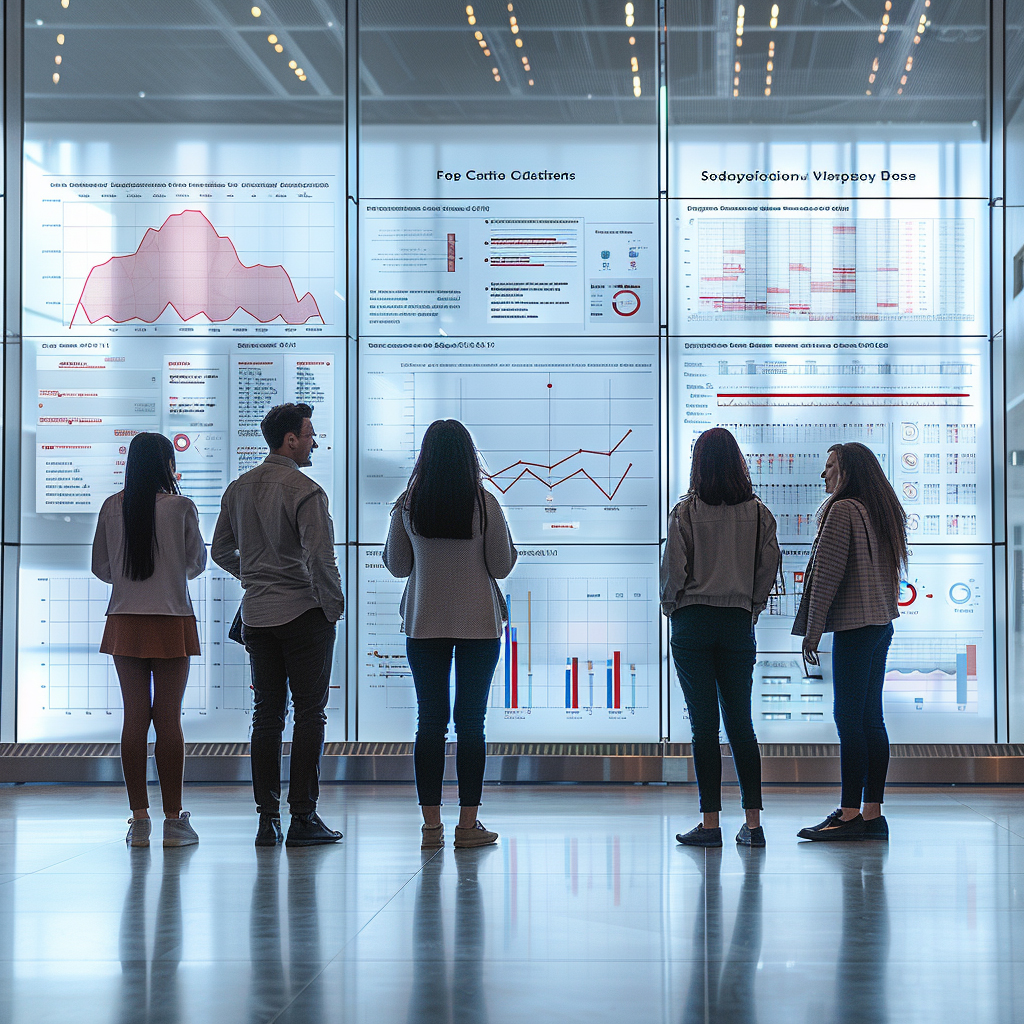
By analyzing customer behavior patterns and preferences, AI-driven predictive analytics can accurately forecast future buying behaviors and trends. This allows companies to optimize their marketing strategies, personalize customer interactions, and align product offerings with consumer expectations, ultimately enhancing customer satisfaction and loyalty.
7. Risk Assessment
In sectors like insurance and lending, AI helps companies assess risks more accurately by considering a wider range of variables and their possible interactions, leading to more precise risk profiles
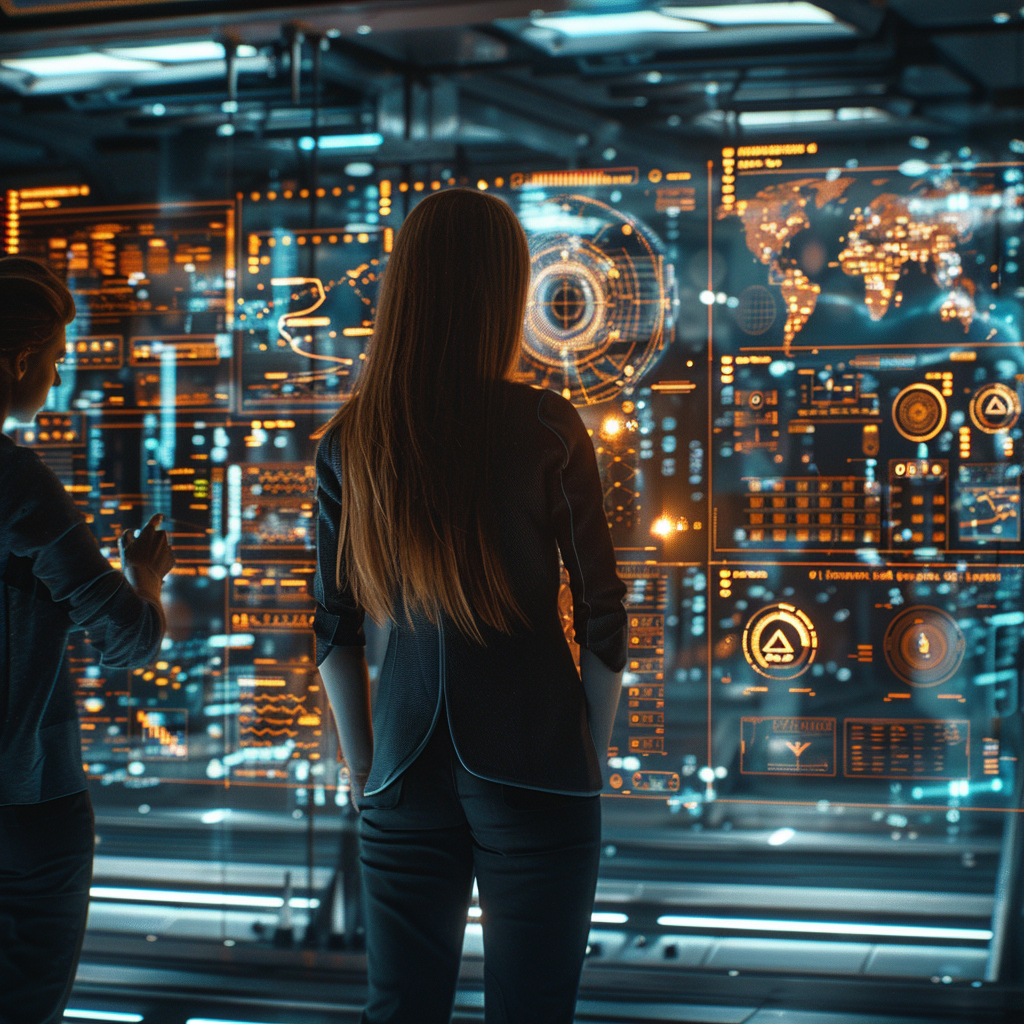
AI-powered analytics transform risk assessment in industries like insurance and finance by integrating and analyzing a broader array of variables. AI models can simulate various scenarios and their impacts, creating detailed risk profiles that help companies make better-informed decisions about pricing, policy offerings, and risk mitigation strategies.
8. Predictive Maintenance
In manufacturing and other asset-intensive industries, AI predicts when equipment is likely to fail, enabling preemptive maintenance that can prevent costly downtimes and extend asset life.
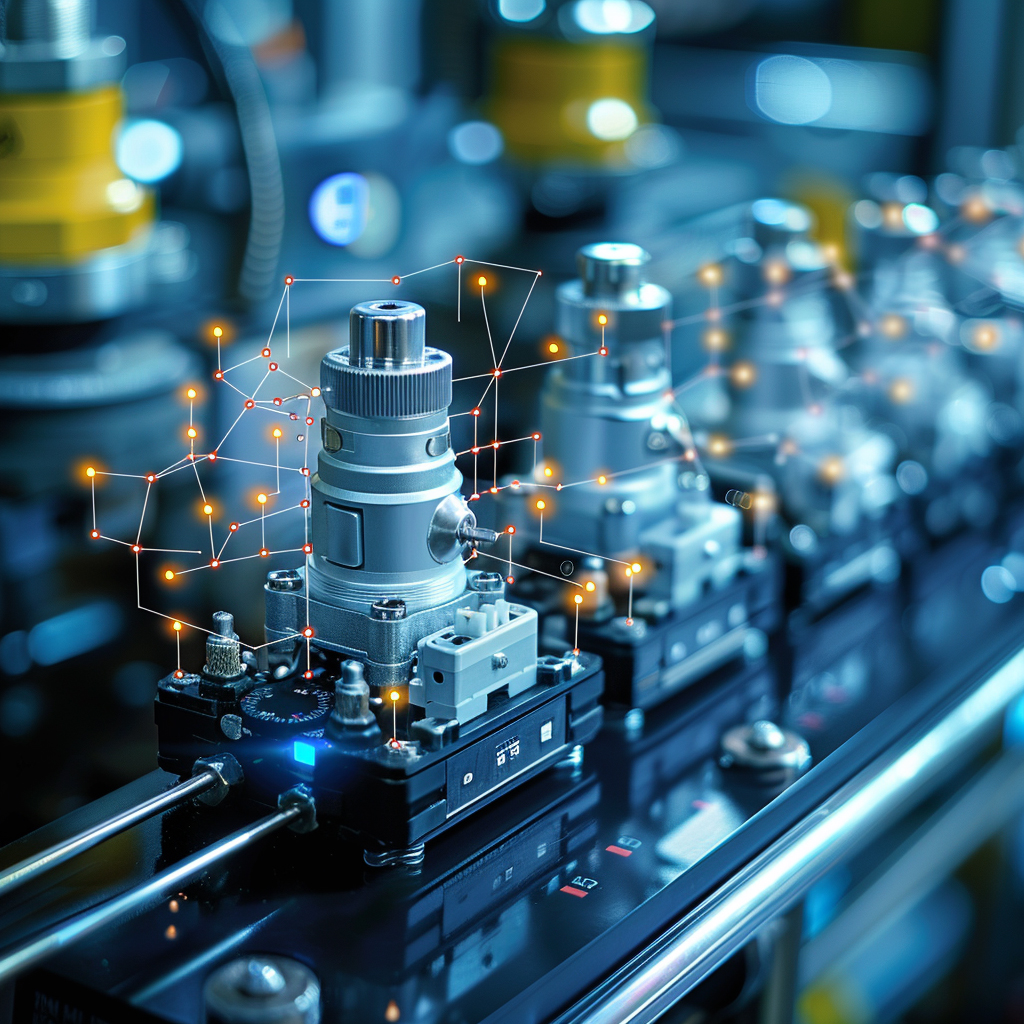
In manufacturing and other sectors reliant on heavy machinery, AI-driven predictive maintenance anticipates potential equipment failures before they occur. By analyzing data from sensors and maintenance logs, AI predicts when and where breakdowns might happen, scheduling maintenance that prevents interruptions and extends the lifespan of the equipment.
9. Optimization of Supply Chains
AI enhances predictive analytics in supply chain management, forecasting product demand and supply needs, optimizing inventory levels, and identifying potential disruptions.
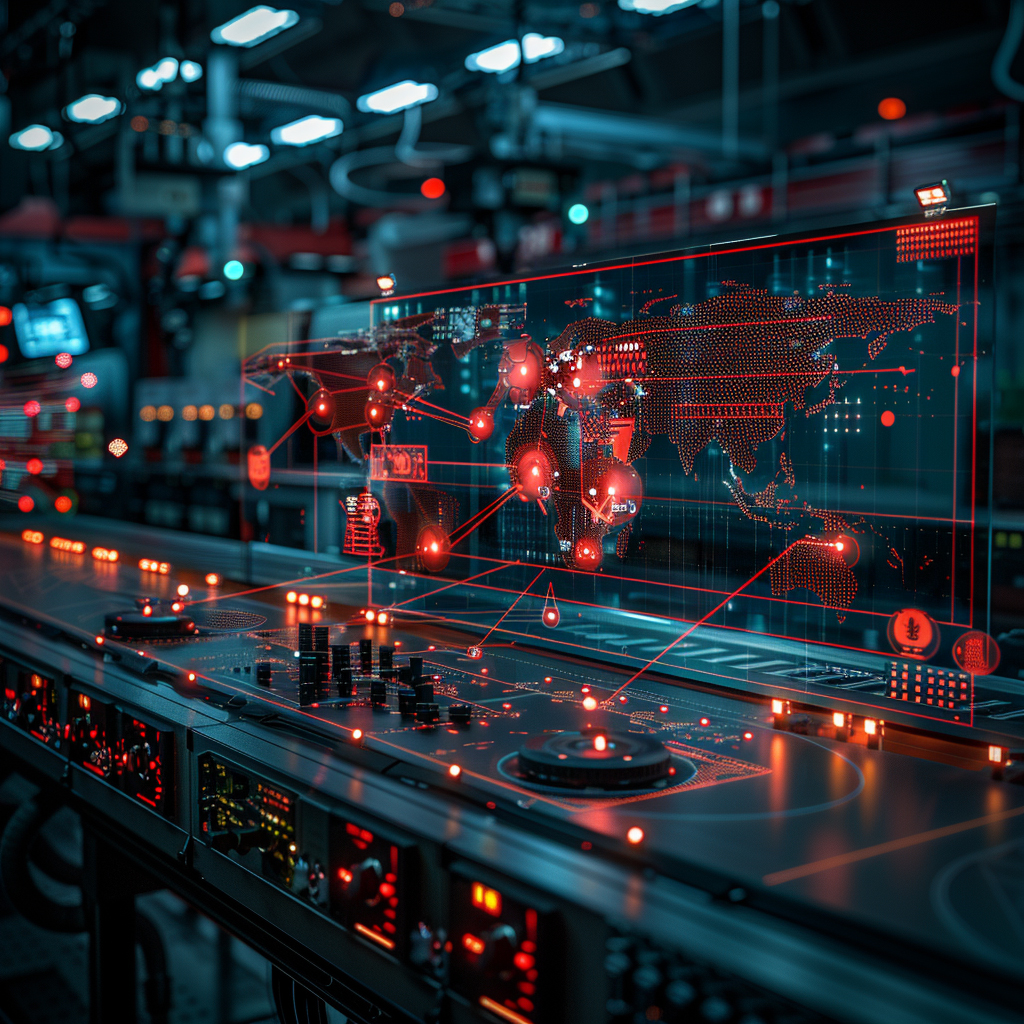
AI improves supply chain management by forecasting demands, supply conditions, and potential logistical disruptions with high accuracy. This predictive insight helps businesses manage inventory more efficiently, reduce costs, and ensure the smooth operation of supply chains even under challenging conditions.
10. Healthcare Predictions
In healthcare, AI-driven predictive analytics can anticipate outbreaks, patient admissions, and even potential complications, improving patient outcomes and operational efficiency.
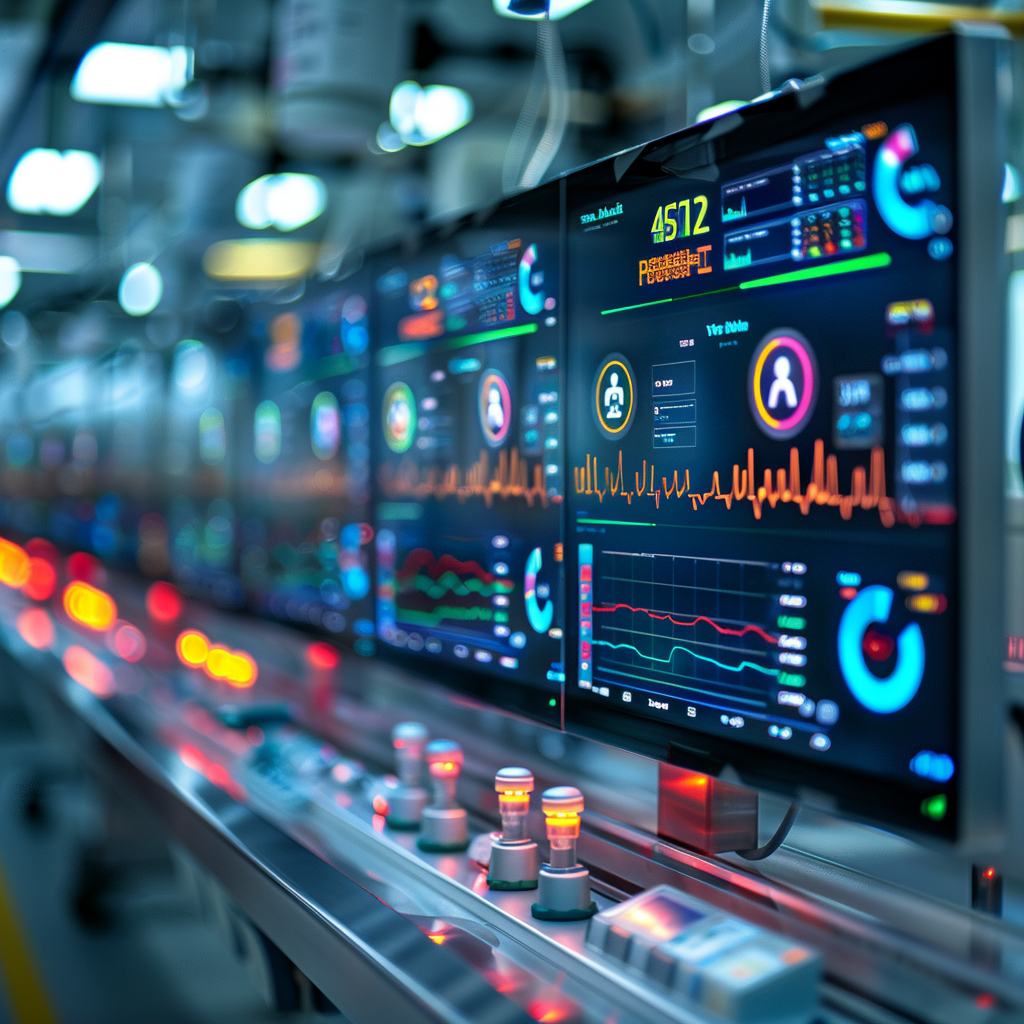
In the healthcare sector, AI-driven predictive analytics plays a crucial role in anticipating medical events such as disease outbreaks, patient admission rates, and potential complications. These predictions enable healthcare providers to allocate resources more effectively, improve patient care, and enhance overall operational efficiency in medical facilities.