1. Crop Monitoring and Management
AI is transforming crop monitoring by using drones, satellite imagery, and on-field sensors to continuously assess crop health in real time. Machine vision algorithms can analyze aerial images to detect early signs of plant stress, diseases, or pest infestations that would be hard for the human eye to catch across large fields. This early detection allows farmers to intervene promptly and target problem areas, which helps prevent minor issues from escalating and significantly damaging yields. AI-driven monitoring systems also track plant growth and nutrient levels, enabling more precise fertilization and irrigation—so each part of the field gets exactly what it needs. Overall, AI improves decision-making in crop management by converting massive data inputs (like weather, soil, and plant condition data) into actionable insights, thereby increasing efficiency and reducing wasted resources. These technologies reduce the need for labor-intensive field scouting and provide a comprehensive view of crop conditions that farmers can access instantly via software dashboards or mobile devices.
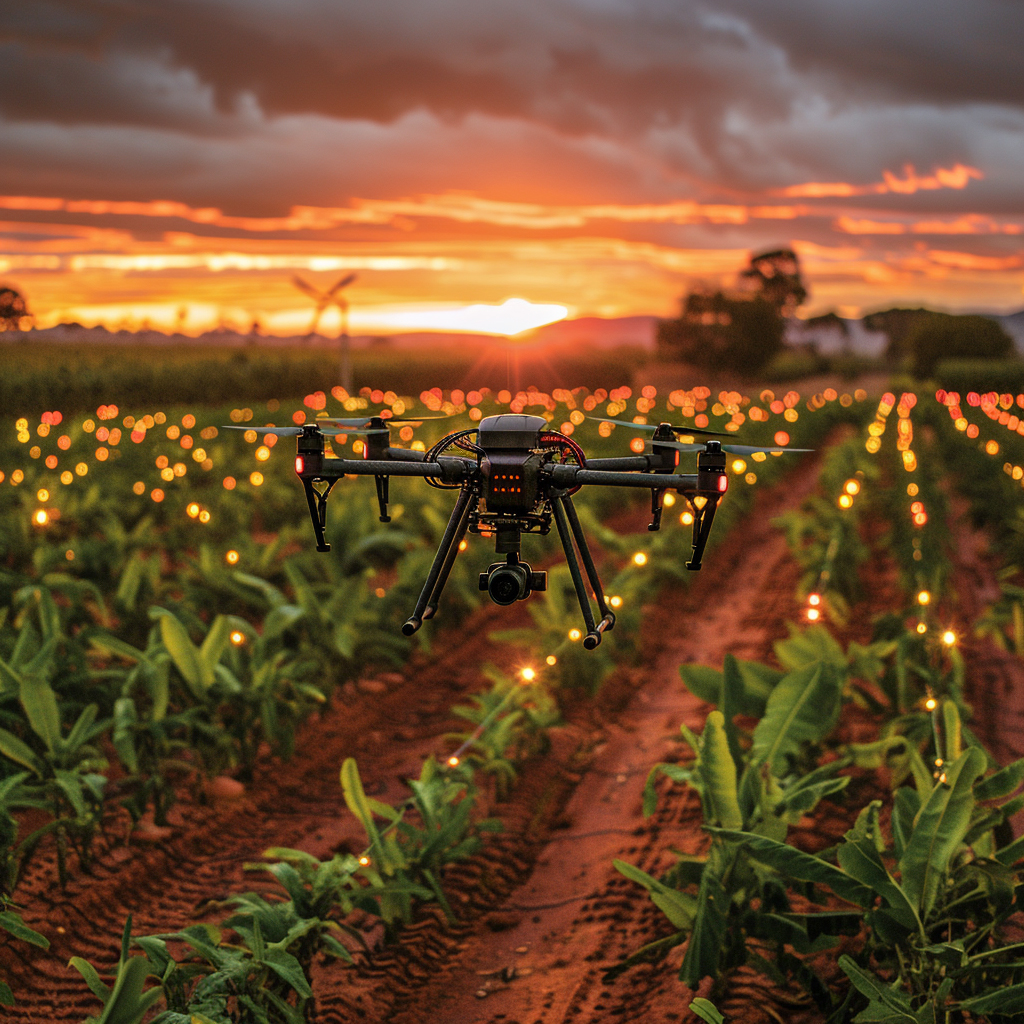
In 2024, 70% of farmers using AI-driven tools reported about a 20% boost in their operational efficiency and crop yields. This underscores how AI-based monitoring and management can directly translate into higher productivity on the farm.
2. Yield Prediction
AI is enabling more accurate crop yield prediction by analyzing an array of data sources that influence crop performance. Machine learning models ingest historical yield data, real-time weather patterns, soil conditions, and even genetic information of crop varieties to forecast how much harvest to expect. By recognizing complex patterns in these data, AI systems can predict yields earlier in the growing season with improving accuracy. Farmers use these predictions to make informed decisions—such as adjusting fertilizer application, planning storage and marketing of the crop, or mitigating risks if a lower yield is anticipated. Importantly, AI yield prediction models can adapt to changing climate conditions; for example, they learn from weather anomalies and crop responses to refine future forecasts. As a result, farmers are better equipped to allocate resources efficiently and avoid surprises at harvest time, ultimately stabilizing income and food supply through smarter planning.
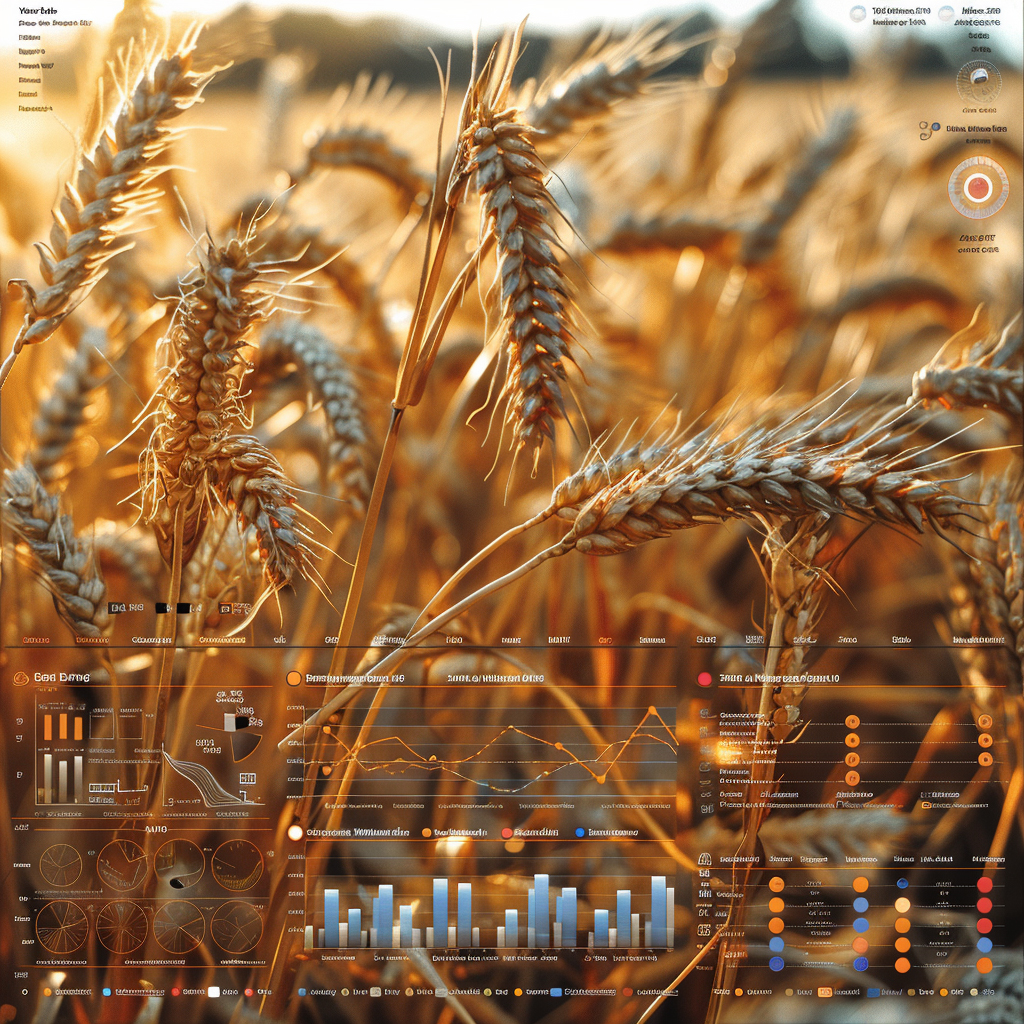
Advanced farming operations are rapidly adopting these technologies – by 2023, approximately 68% of large-scale U.S. crop farms were using yield monitors and maps to collect data for yield analysis and forecasting. This widespread use of yield-mapping technology reflects how integral data-driven yield prediction has become in modern agriculture.
3. Automated Weeding and Pesticide Application
AI-powered weeding and targeted pesticide application are making crop protection far more precise and environmentally friendly. Instead of spraying an entire field with herbicides or pesticides, smart robots and drones equipped with cameras and AI algorithms can identify weeds or pest hotspots at the plant level. These systems use computer vision to distinguish weeds from crops, and then they either mechanically remove the weeds or spray herbicide only on the unwanted plants. By treating just the affected areas (and nothing more), AI greatly reduces the volume of chemicals used. This precision not only cuts costs on herbicides and pesticides, but also minimizes chemical runoff into soil and water, thereby lessening environmental impact. Moreover, automated weeding robots can work around the clock and relieve farmers from hours of manual weeding labor. Over time, AI models also “learn” weed growth patterns and pesticide effectiveness, improving their ability to target the most resilient weeds. In essence, AI is helping farmers keep crops healthy with fewer chemicals and labor, contributing to more sustainable crop protection practices.
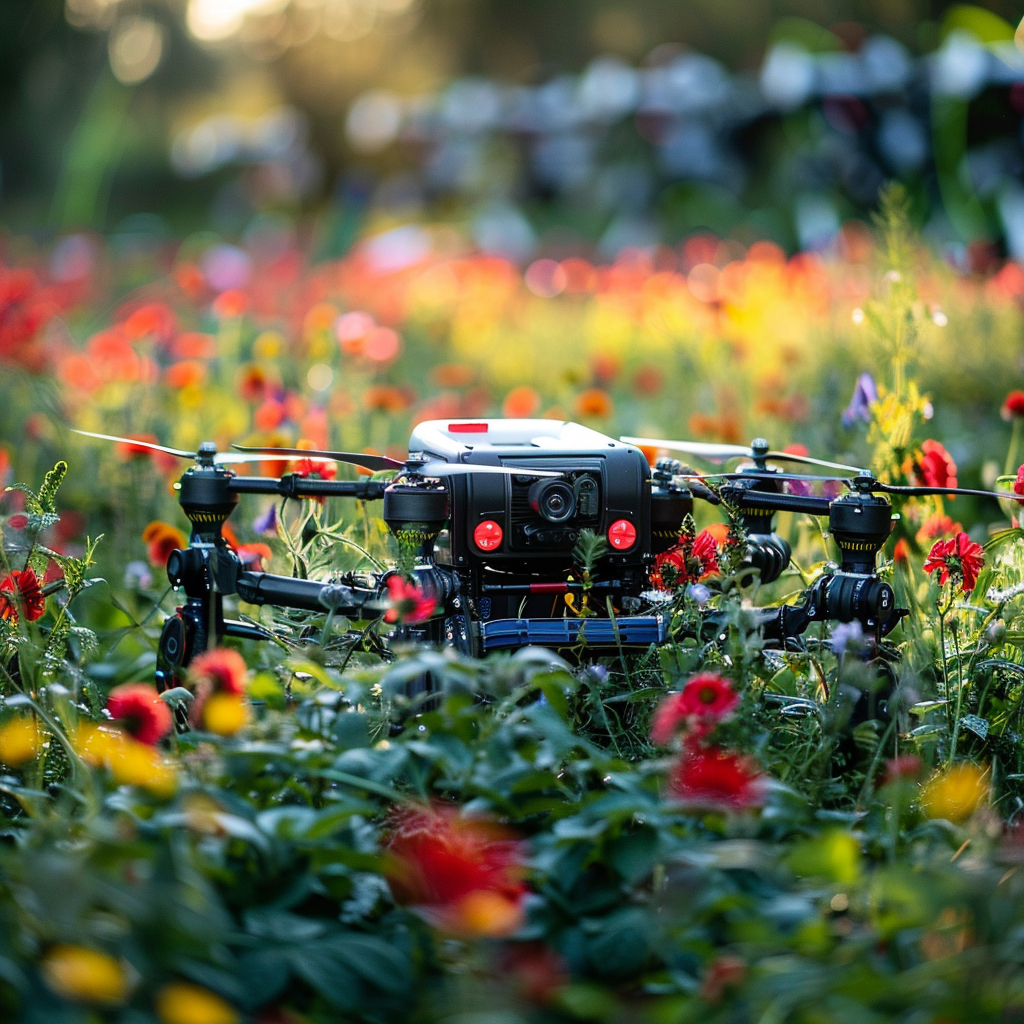
Field trials show that AI-guided “see-and-spray” technology can cut herbicide use by roughly 77% compared to conventional blanket spraying. This dramatic reduction – achieved by only spraying weeds and not bare ground – illustrates how precise AI-powered weeding translates into both cost savings and environmental benefits.
4. Soil Health Analysis
AI is improving how farmers assess and manage soil health, which is foundational for crop productivity. By integrating data from soil sensors (measuring moisture, pH, nutrient levels) with satellite and drone imagery of fields, AI systems can create detailed soil maps and recommendations. These AI tools identify variability in soil conditions within a farm—showing which zones are dryer, nutrient-deficient, or more acidic—and help farmers apply water, fertilizers, or lime more precisely. For example, machine learning models analyze soil nutrient data to suggest optimal fertilizer types and application rates for each part of a field, avoiding over-fertilization in rich spots and under-fertilization in poor spots. This not only maximizes crop uptake and yields but also reduces fertilizer runoff. AI-driven soil analysis can also detect gradual changes or emerging issues in soil quality (like organic matter decline or compaction) by comparing data over time, alerting farmers early so they can take corrective action (such as cover cropping or tillage adjustments). In sum, AI turns scattered soil data into actionable insights, enabling farmers to treat their soil’s needs on a micro scale and sustain long-term soil fertility.
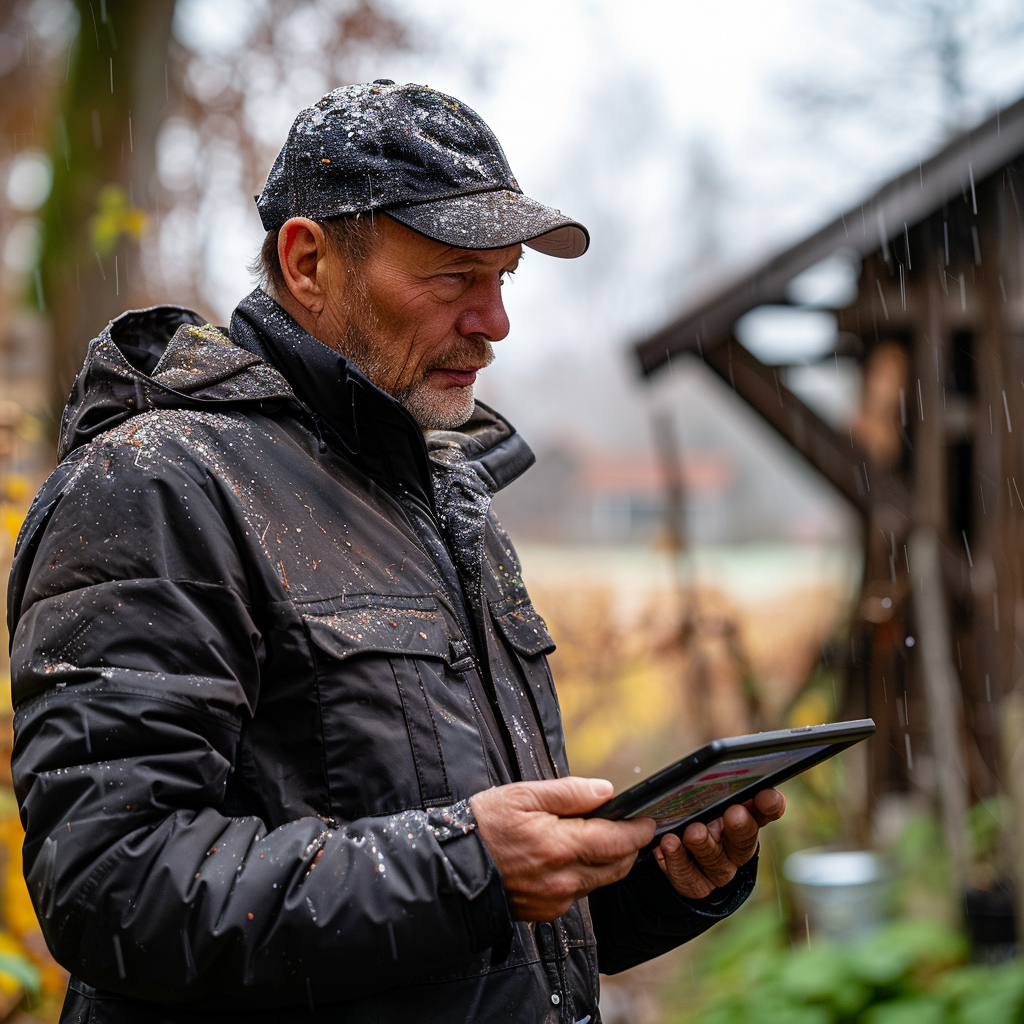
Research demonstrates that AI-based soil management can both cut input use and maintain yields. In one 2023 study, an AI-driven fertilizer optimization model led to a 15% reduction in fertilizer usage without any drop in crop yield, by tailoring nutrient supply to soil needs. This showcases how AI soil analysis can boost sustainability—saving fertilizer costs and preventing pollution—while still keeping crops productive.
5. Irrigation Management
AI is making irrigation far more efficient by intelligently determining when, where, and how much water crops need. Traditional irrigation often waters fields on a fixed schedule, but AI-driven irrigation systems instead analyze real-time inputs like soil moisture sensor readings, weather forecasts, and even plant stress indicators to decide optimal watering. Through machine learning, these systems learn the specific water needs of different fields and crop stages, and they can automatically adjust valves or pivots to deliver precise amounts of water only where needed. For instance, if one zone of a field received rain yesterday and another stayed dry, AI controllers might keep the pumps off for the wet zone but irrigate the dry zone a bit extra. This targeted approach prevents over-watering (which can leach nutrients and waste water) and under-watering (which can stress plants). Some AI irrigation platforms also use predictive analytics to anticipate drought conditions or heat waves, so they can increase watering in advance to help crops cope with stress. Overall, AI-managed irrigation leads to substantial water conservation and energy savings. It also improves crop yields and quality by ensuring plants consistently get just the right amount of water – not too much, not too little – throughout the growing season.
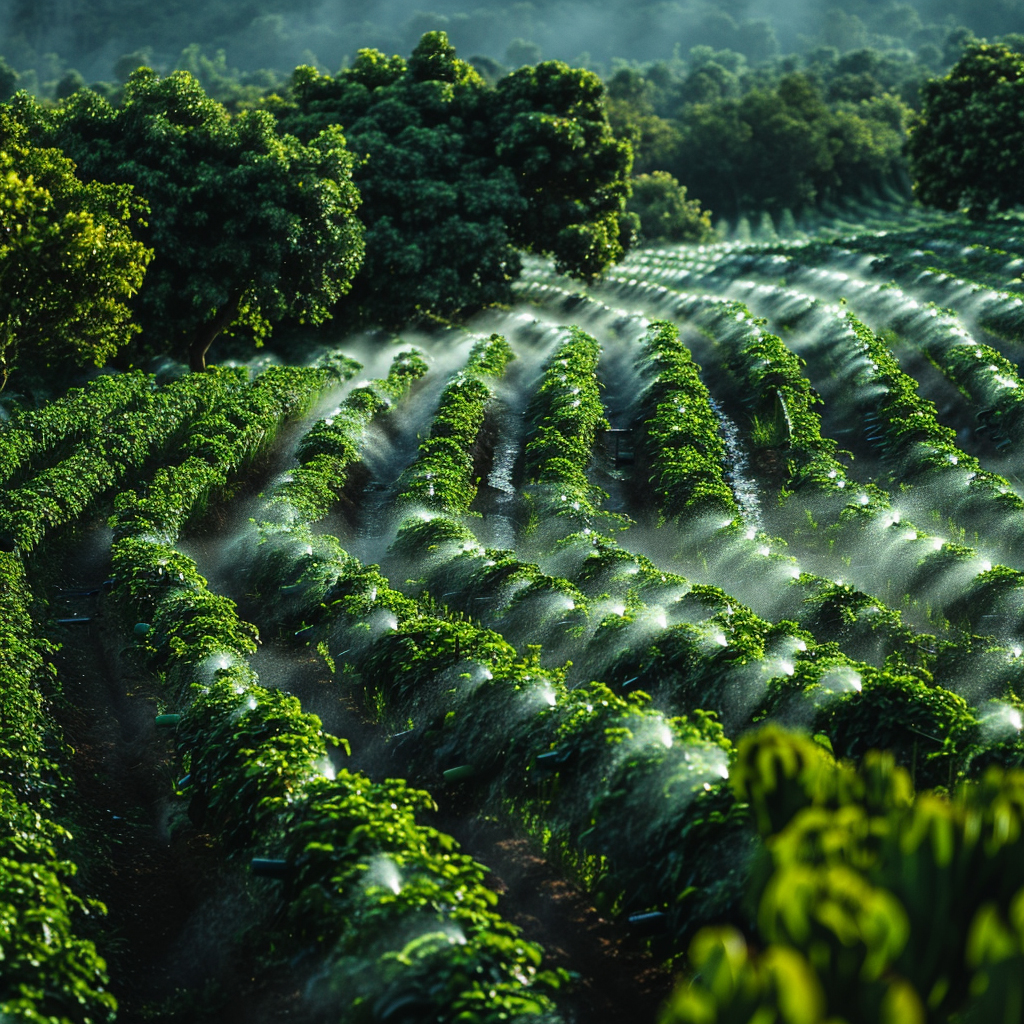
Real-world results show the impact of AI on water savings. For example, field tests at MIT’s GEAR Lab found that AI-based precision irrigation controllers reduced water consumption by over 40% compared to conventional methods, all while maintaining crop performance. Such systems use sensor data and adaptive algorithms to minimize waste, illustrating how AI can significantly conserve water in agriculture.
6. Plant Breeding
AI is accelerating plant breeding – the process of developing new crop varieties – by making it faster and more predictive. Traditionally, plant breeding involves years of trial-and-error: crossing plants, growing them out, and selecting the best performers over multiple generations. Now, AI algorithms can analyze vast genomic datasets and past breeding results to predict which gene combinations will produce desirable traits (like higher yield, drought tolerance, or pest resistance) without having to test every combination in the field. This approach, often called “genomic selection” or AI-assisted breeding, allows scientists to virtually screen thousands of potential crosses on a computer and identify the most promising ones to actually cultivate. Additionally, AI models can incorporate environmental data, predicting which plant gene traits will thrive under certain climate or soil conditions. This means breeders can tailor new crop varieties to specific local challenges (for instance, a corn line that will do well in a hotter, drier climate). AI also helps in analyzing phenotypic data (observable plant traits) more quickly – for example, image recognition software can measure plant growth or detect disease symptoms automatically, providing breeders with faster feedback on which genetic lines are performing well. By shortening the breeding cycle and improving accuracy in selecting parent plants, AI is helping release improved crop varieties to farmers much sooner, which is crucial for keeping pace with climate change and food security needs.
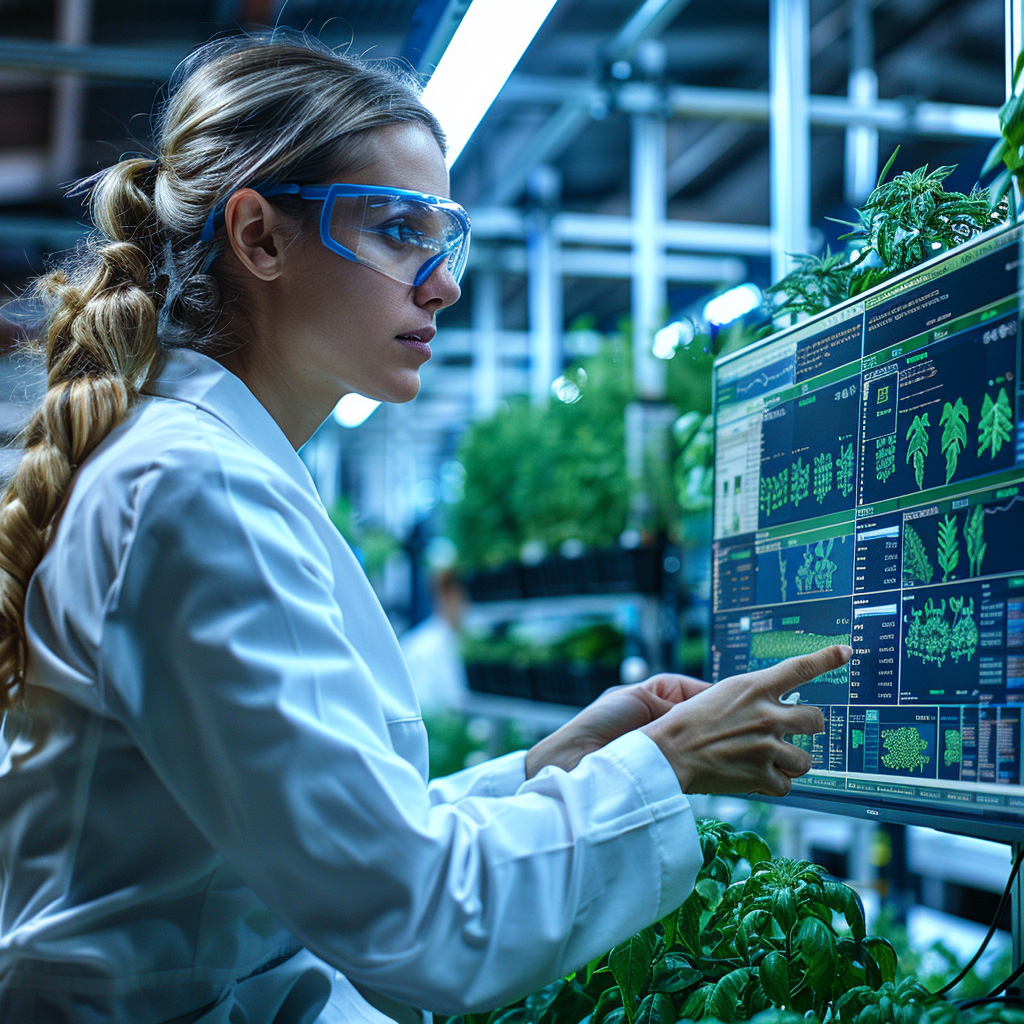
The breeding cycle for new crops is dramatically shortened with AI. A prominent example is a project by Alphabet’s spinout, Heritable Agriculture, which uses AI to predict optimal crop genetics – reducing the development of a new crop variety to roughly 1 year, whereas traditional breeding methods take around 7–10 years. This order-of-magnitude speedup illustrates how AI-driven breeding is revolutionizing crop development.
7. Supply Chain Optimization
AI is improving the agricultural supply chain by forecasting demand, streamlining logistics, and reducing waste from farm to market. One key role of AI is analyzing consumer demand patterns (using retail sales data, market trends, even social media cues) to help farmers and distributors align crop production with what the market needs in real time. This means growers can plan harvests and planting to avoid overproduction of perishable goods that might otherwise go unsold. AI also optimizes transportation and storage: for example, routing algorithms can determine the fastest or most efficient way to get fresh produce from farms to warehouses to stores, minimizing transit time and spoilage. In cold storage facilities, AI systems monitor and adjust conditions to keep food fresh longer. Some AI platforms monitor produce quality and inventory at each step, automatically redirecting shipments or adjusting prices to ensure that nearly-expiring goods are sold rather than wasted. By having a better handle on supply and demand, AI helps prevent the common scenario of food gluts in one region and shortages in another. Additionally, AI can coordinate the timing in supply chains – ensuring that packaging, trucks, and labor are available exactly when a crop is ready to harvest. The end result is a more efficient supply chain that cuts costs, reduces food waste, and delivers products to consumers at peak freshness.
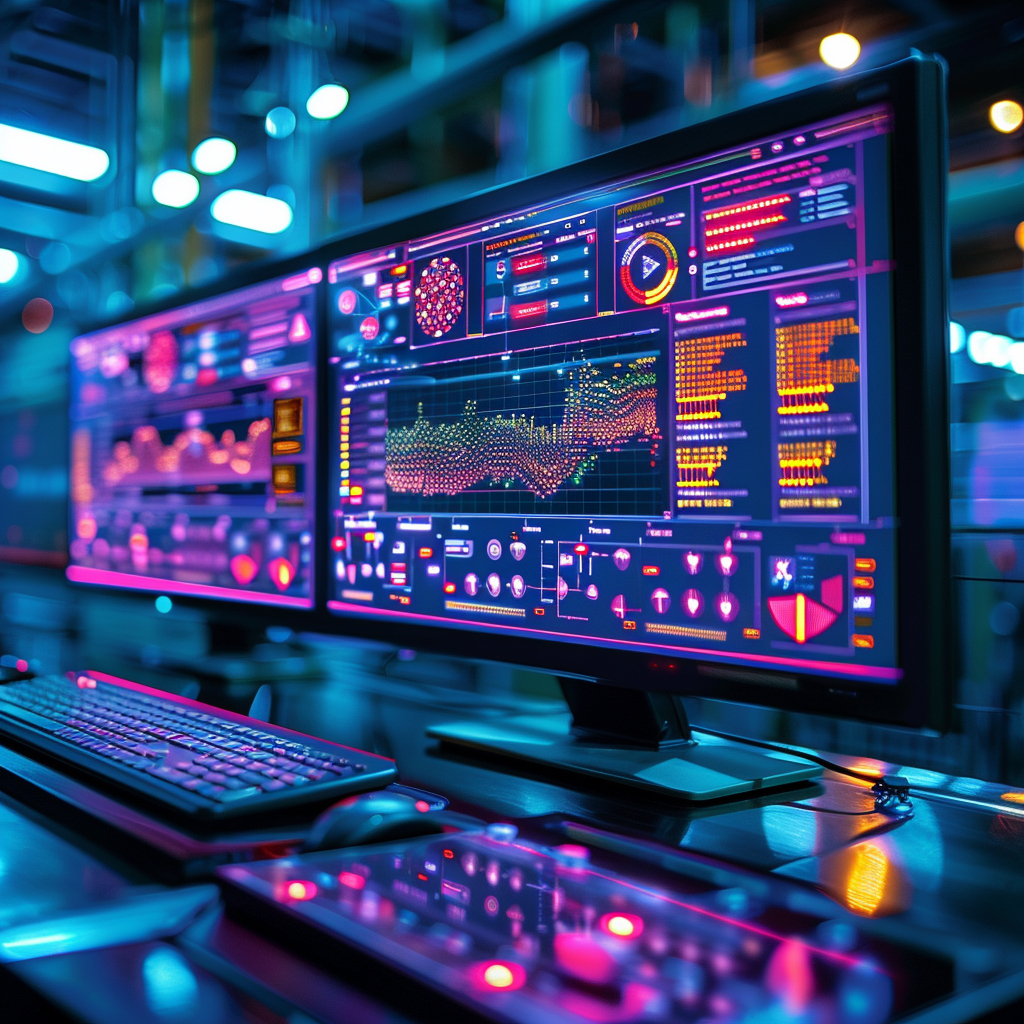
AI-driven inventory and ordering systems have shown striking success in cutting food waste in the supply chain. In pilot programs at grocery retailers, food waste decreased by about 15% per store on average after implementing AI for demand forecasting and automated ordering. These AI systems accurately predicted sales and optimized stock levels—leading not only to less spoilage, but also higher profits for the stores.
8. Livestock Monitoring
AI technologies are greatly enhancing livestock monitoring by continuously tracking the health, behavior, and welfare of farm animals. Wearable sensors (such as smart collars, ear tags, or leg bands) on cattle, pigs, and poultry can collect vital signs like body temperature, heart rate, rumination (chewing) activity, and movement patterns. These data are fed into AI systems that learn what “normal” looks like for each animal or herd. When an individual’s patterns deviate from the norm—say, a cow’s activity drops and temperature rises slightly—AI can flag this early as a potential illness, often days before outward symptoms are visible. Farmers receive alerts on their computers or phones pinpointing which animal might need attention, enabling timely veterinary care or isolation of the sick animal to prevent disease spread. AI monitoring also helps optimize feeding: smart cameras and sensors can observe how much each animal eats and drinks, and AI adjusts feed rations to ensure optimal nutrition or to prevent waste. In breeding, AI analyzes behavior and hormonal data to detect the best time for animal insemination, improving reproductive success rates. All this continuous monitoring means farmers no longer have to physically inspect every animal as frequently, yet health issues or stress conditions (like heat stress or overcrowding) are less likely to be missed. The improved health management not only enhances animal welfare but also boosts productivity (healthier animals gain weight, produce milk or eggs more consistently, etc.) and reduces costs from untreated illnesses or mortalities.
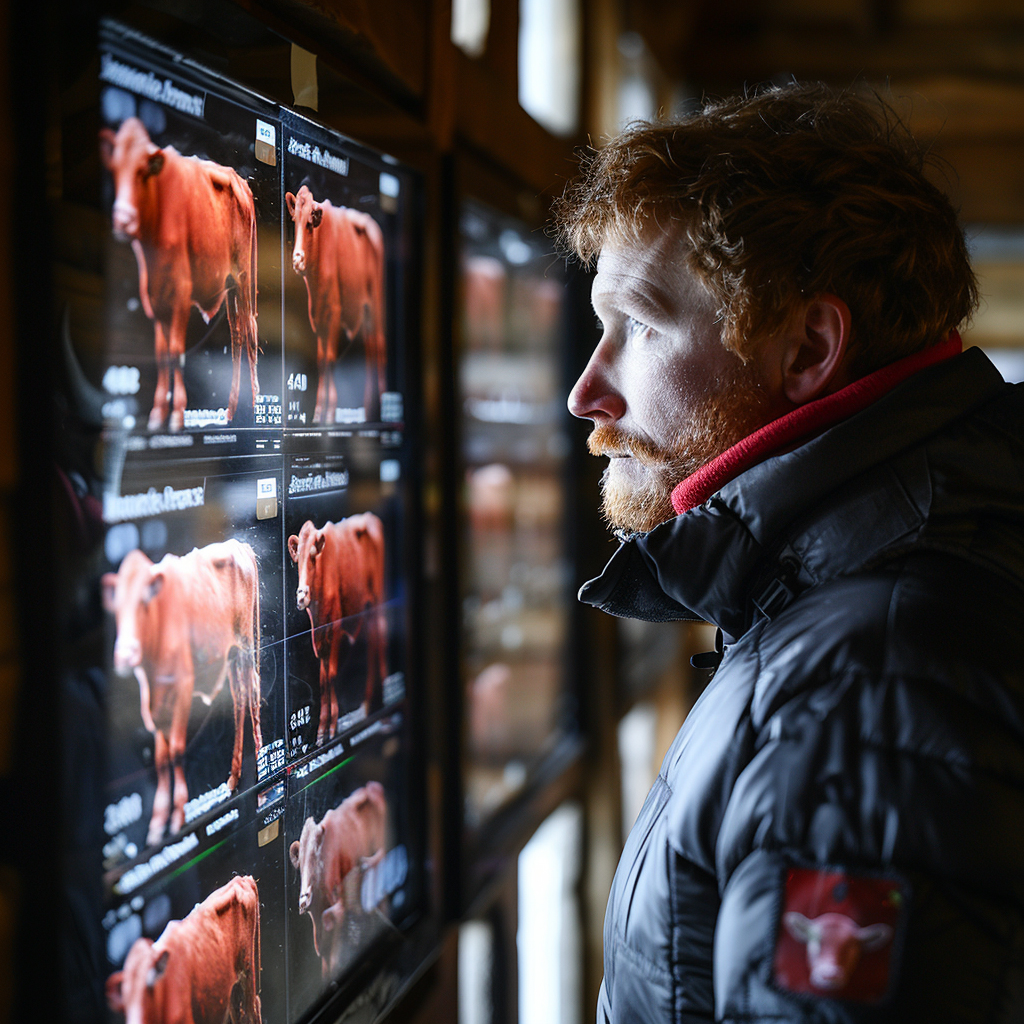
Implementing AI-based health trackers on livestock can lead to significantly earlier disease detection and lower mortality. For instance, smart ear tag systems have enabled identifying sick cattle 2–3 days earlier than traditional observation methods, which has resulted in a marked reduction in on-farm animal death rates. By catching illnesses in their initial stages, farmers can treat animals sooner and prevent small issues from becoming fatal outbreaks.
9. Precision Sowing
AI is refining precision sowing by ensuring seeds are planted in the right place, at the right depth, and at the optimal rate for maximum germination and yield. Modern precision planters outfitted with AI-guided control can adjust seeding on-the-fly across a field: for example, in fertile high-yield zones, the system might sow seeds at a higher density, while in poorer soil areas it uses a lower rate to avoid wasting seed where plants won’t thrive as much. Depth control is also crucial—AI uses feedback from soil sensors and furrow cameras to maintain each seed at the ideal depth in the soil moisture zone, even if the field terrain undulates or residue levels change. This leads to more uniform emergence of seedlings. Achieving uniform emergence means nearly all the plants sprout around the same time, so none are at a competitive disadvantage; those uniformly emerged crops tend to grow evenly and yield better. Additionally, AI-driven precision sowing equipment can correct issues like seed spacing; it identifies any skips or doubles (missing seeds or two seeds dropped together) and adjusts mechanisms to fix these in real time. By optimizing seeding rates and placement, AI helps maximize yield potential (each seed has the best chance to become a healthy plant) and minimizes wasted seed costs. It also allows farmers to experiment with variable strategies easily, since they can program sowing prescriptions into the AI system and let it execute them accurately. Ultimately, AI takes into account soil variability, hybrid characteristics, and environmental conditions to sow crops in a manner that sets the stage for a strong, uniform stand and efficient use of inputs.
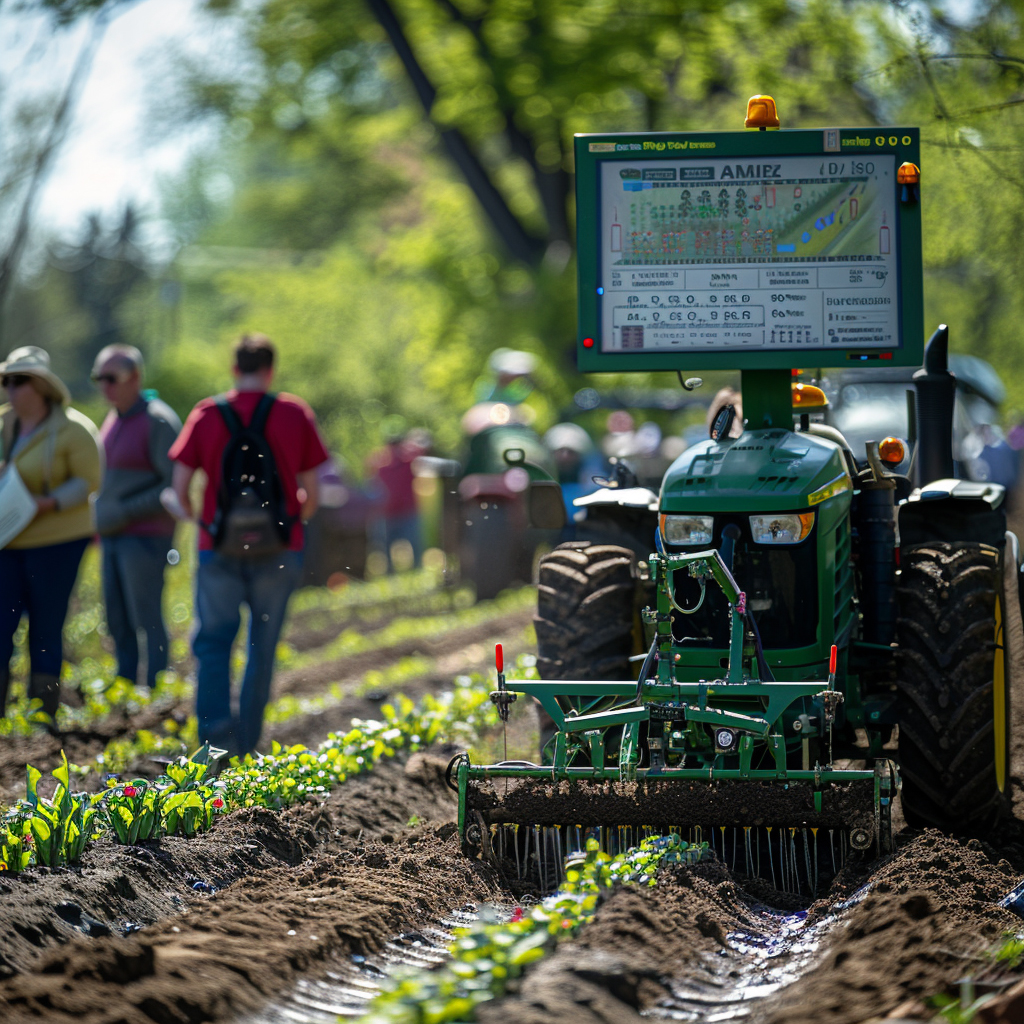
Consistent seedling emergence is critical for yield – research summaries indicate that achieving uniform emergence can influence corn yields by about 5–9%. AI-equipped planters that precisely control seed depth and spacing help hit this target, preventing the yield losses that occur when some plants emerge later than their neighbors.
10. Data Integration and Analysis
AI plays a pivotal role in integrating and analyzing the diverse data streams on a modern farm, turning “big data” into actionable farming decisions. Today’s farmers can collect data from many sources – soil sensors, weather stations, drone imagery, machinery logs, market prices, etc. – but making sense of all this information manually is overwhelming. AI comes to the rescue by aggregating these disparate data types into one platform and finding meaningful patterns. For example, an AI system might correlate soil moisture data with irrigation records and weather forecasts to advise when and where to water next, or combine pest scouting reports with climate data to predict an upcoming infestation risk. Crucially, AI doesn’t just look at one type of data in isolation; it learns from the complex interactions (perhaps finding that a certain field produces best when a specific fertilizer rate coincides with particular rainfall and planting date conditions, for instance). These insights help farmers make more informed, holistic decisions rather than siloed ones. Additionally, AI-driven farm management dashboards present the integrated data in user-friendly maps and charts – showing, say, real-time machine performance alongside crop growth stages and budget metrics – giving farmers a comprehensive operational picture at a glance. Over time, AI can also benchmark and learn what strategies work best for a particular farm by analyzing past years’ results. By breaking down data silos and continuously mining the farm’s data, AI ensures nothing valuable is overlooked and that lessons from one part of the operation inform the others. This integrated analysis leads to better coordination (e.g., planting, fertilizing, and marketing plans all aligned) and a more resilient, optimized farming system overall.
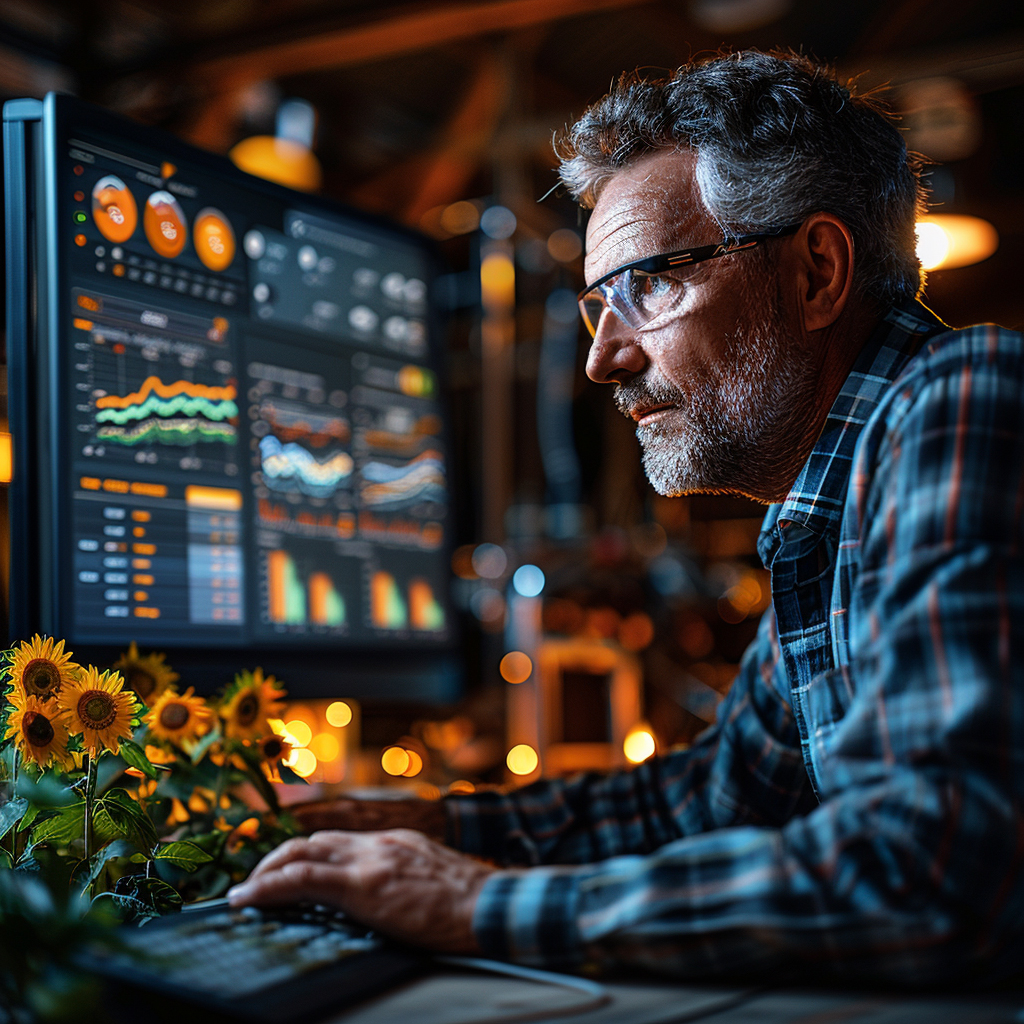
The sheer volume of farm data underscores the need for AI integration. It’s estimated that the average farmer generates about 500,000 data points per day, ranging from sensor readings to equipment data – and this daily data generation is projected to increase by 800% by 2036 with the spread of connected devices. AI is essential to process and synthesize this flood of information into useful guidance for farmers.