1. Precision Diagnostics
AI algorithms analyze medical imaging and genetic data to diagnose diseases more accurately and at earlier stages, tailored to the specific genetic makeup and risk factors of individual patients.
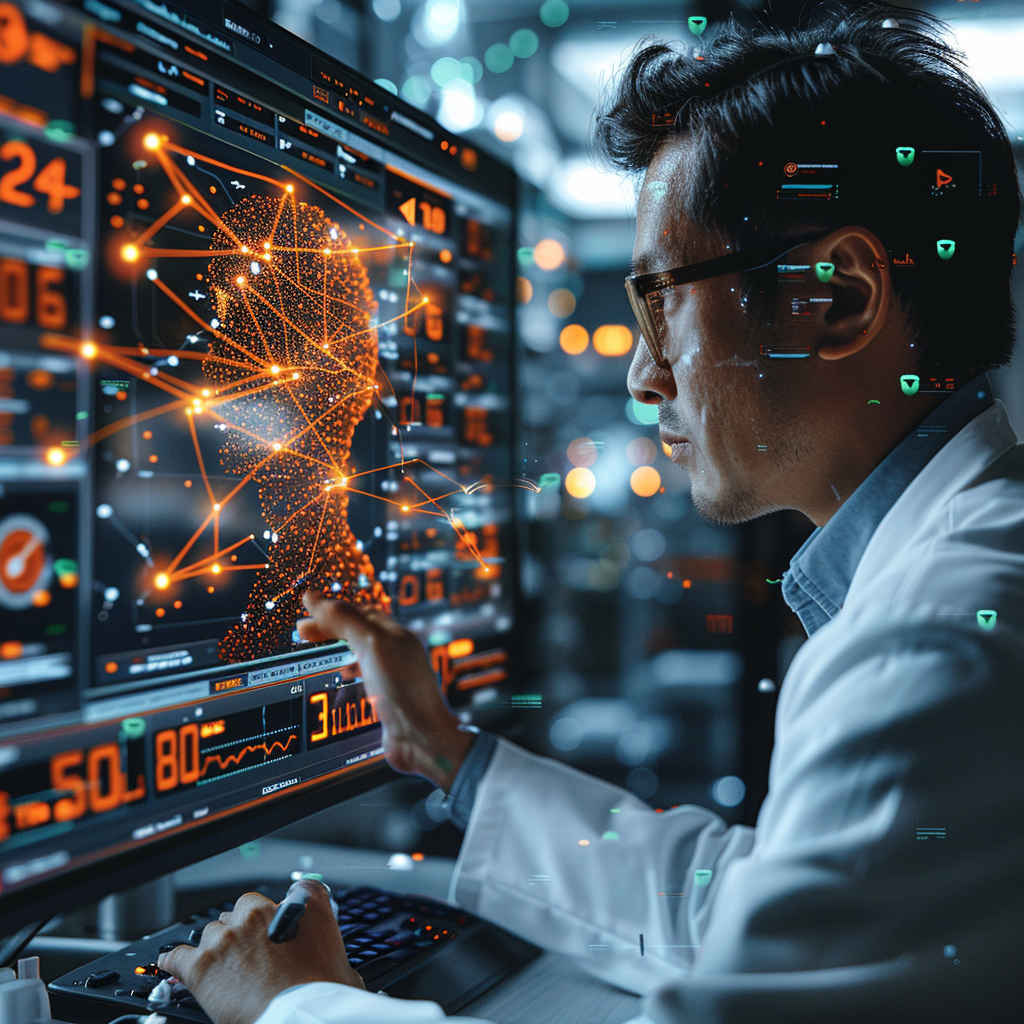
AI algorithms are transforming diagnostics by analyzing complex medical imaging and genetic data to identify diseases with a high degree of accuracy. These algorithms can detect subtle patterns in data that might be missed by human eyes, enabling earlier and more precise diagnoses. For example, AI can identify specific cancer types by analyzing tumor patterns in imaging data, leading to more tailored treatment strategies that consider the individual characteristics of each patient’s disease.
2. Treatment Customization
AI helps in designing personalized treatment plans based on a patient's unique health profile, including their medical history, lifestyle, and genetic information, ensuring more effective treatment outcomes.
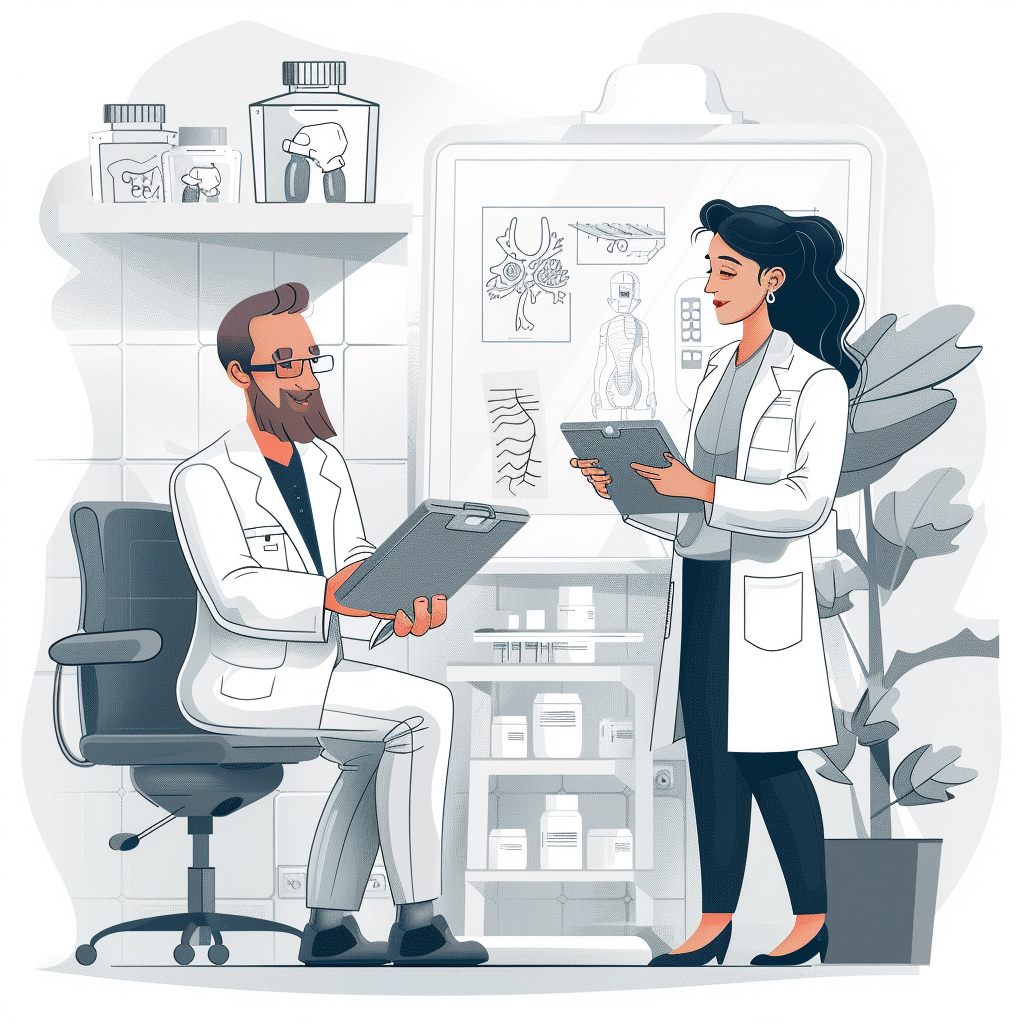
AI plays a critical role in customizing treatment plans by considering a wide range of factors unique to each patient, including genetic profiles, past medical history, and even lifestyle choices. By analyzing these factors, AI can help clinicians develop optimized treatment protocols that are more likely to be effective for the individual, thereby improving the chances of successful outcomes and reducing the risk of side effects.
3. Drug Development
AI accelerates the process of drug discovery and development by predicting how different drugs might interact with various genetic markers, leading to more effective and customized medication options.
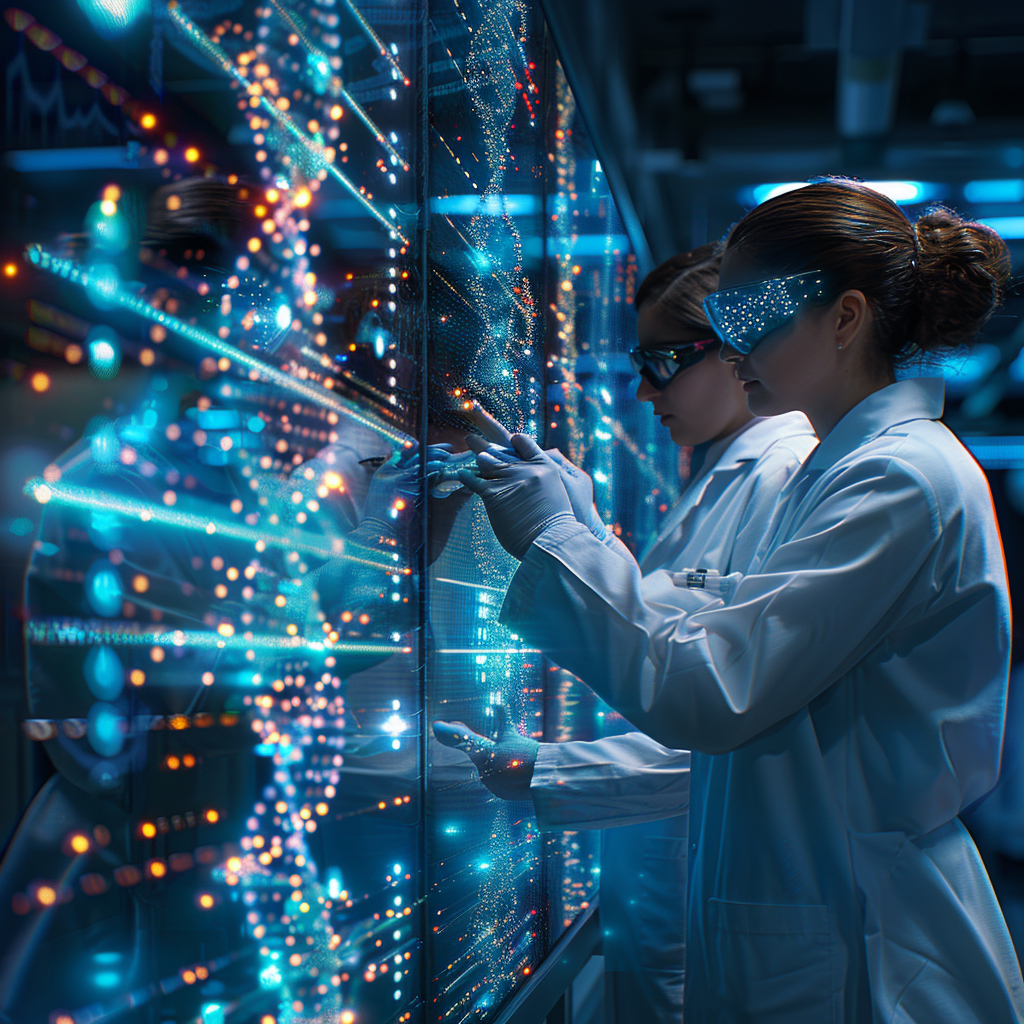
In drug development, AI accelerates the discovery of new medications by simulating how drugs interact with various biological pathways. This capability allows researchers to predict the efficacy and safety of drugs for specific genetic profiles, significantly speeding up the drug development process and paving the way for customized medication solutions tailored to individual genetic makeups.
4. Predictive Analytics
AI uses patient data to predict health risks and disease progression, allowing for preventive measures and early interventions that are specifically tailored to the individual’s health needs.
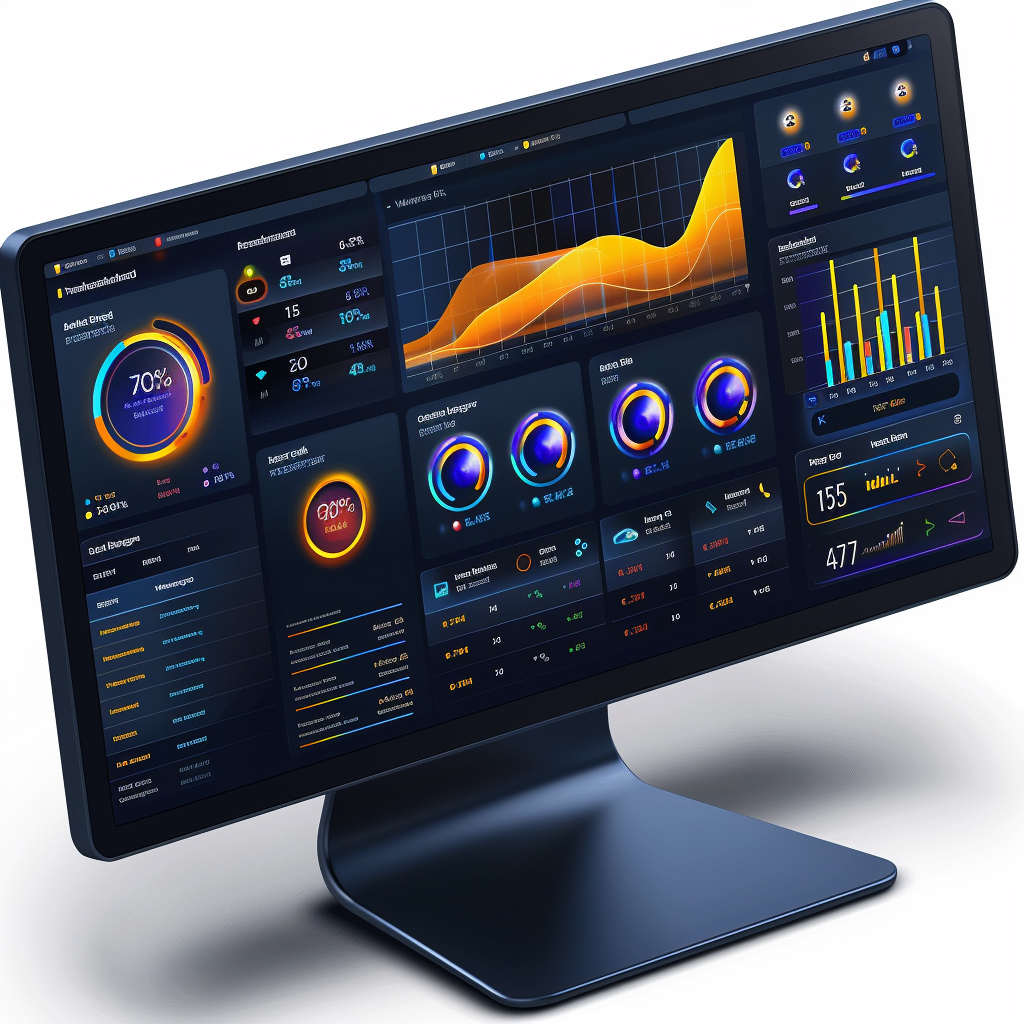
AI utilizes vast amounts of health data to forecast future health events, enabling preventive healthcare that is customized to individual risk profiles. This includes predicting the likelihood of diseases based on genetic markers, lifestyle factors, and existing health conditions. Predictive analytics can guide patients towards preventive measures, lifestyle changes, and early treatments tailored specifically to their predicted health risks.
5. Wearable Health Monitoring
AI integrates with wearable technology to continuously monitor health indicators such as heart rate, blood pressure, and glucose levels, providing real-time insights and alerts tailored to the patient’s specific conditions.
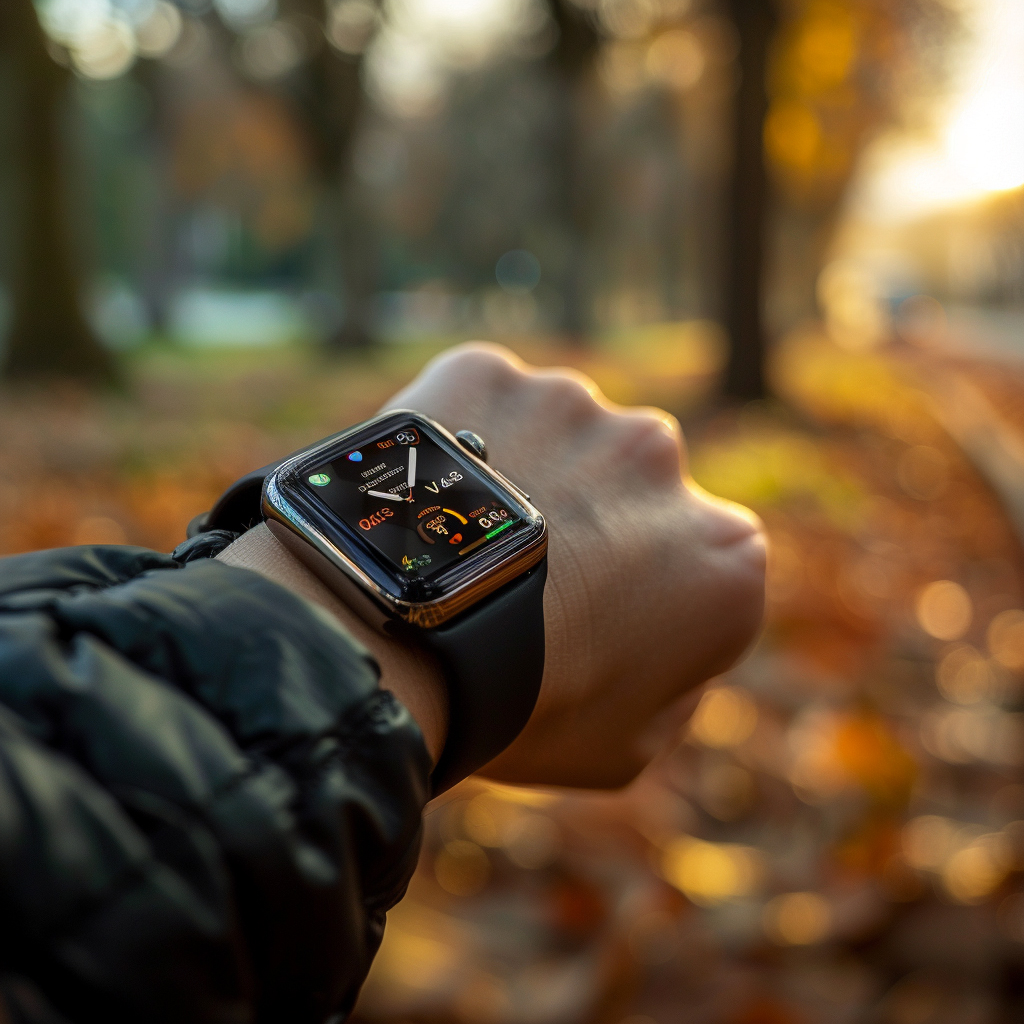
Wearable technology equipped with AI analyzes real-time data such as heart rate variability, activity levels, and sleep patterns. This ongoing monitoring allows for immediate adjustments in treatment plans and lifestyle recommendations based on the patient's current health status, providing a highly personalized healthcare approach that adapts to daily changes in the patient's body.
6. Gene Therapy
AI analyses genetic data to identify the best candidates for gene therapy and helps in designing gene editing tools like CRISPR, making these therapies safer and more effective.
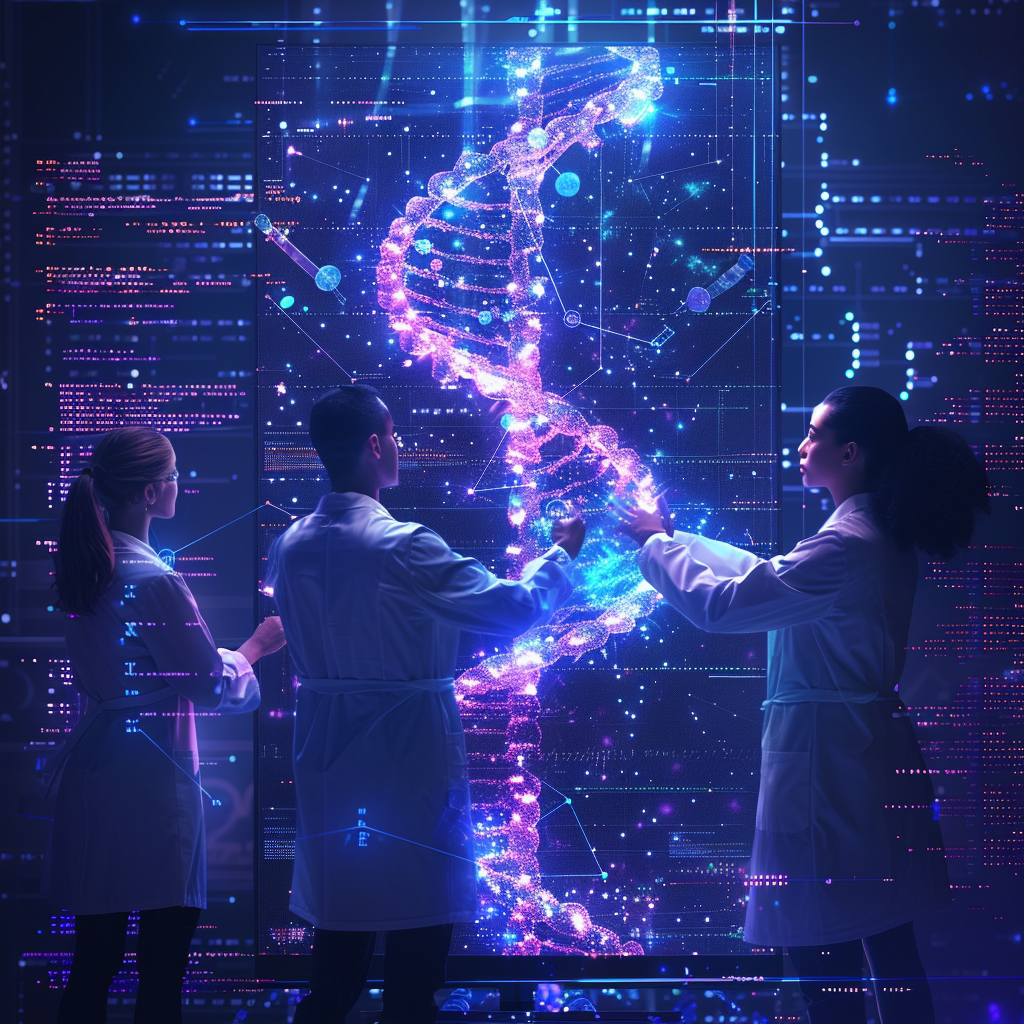
AI contributes significantly to the field of gene therapy by analyzing genetic data to identify mutations that can be targeted by therapies such as CRISPR. AI helps optimize the design of these therapies to ensure precision and efficacy in editing genes, potentially curing genetic disorders at their source. This level of customization ensures that gene therapies are both safe and tailored to the genetic profiles of individual patients.
7. Automation of Routine Tasks
AI automates routine tasks such as data entry and patient scheduling, allowing healthcare providers to focus more on patient care and less on administrative duties.
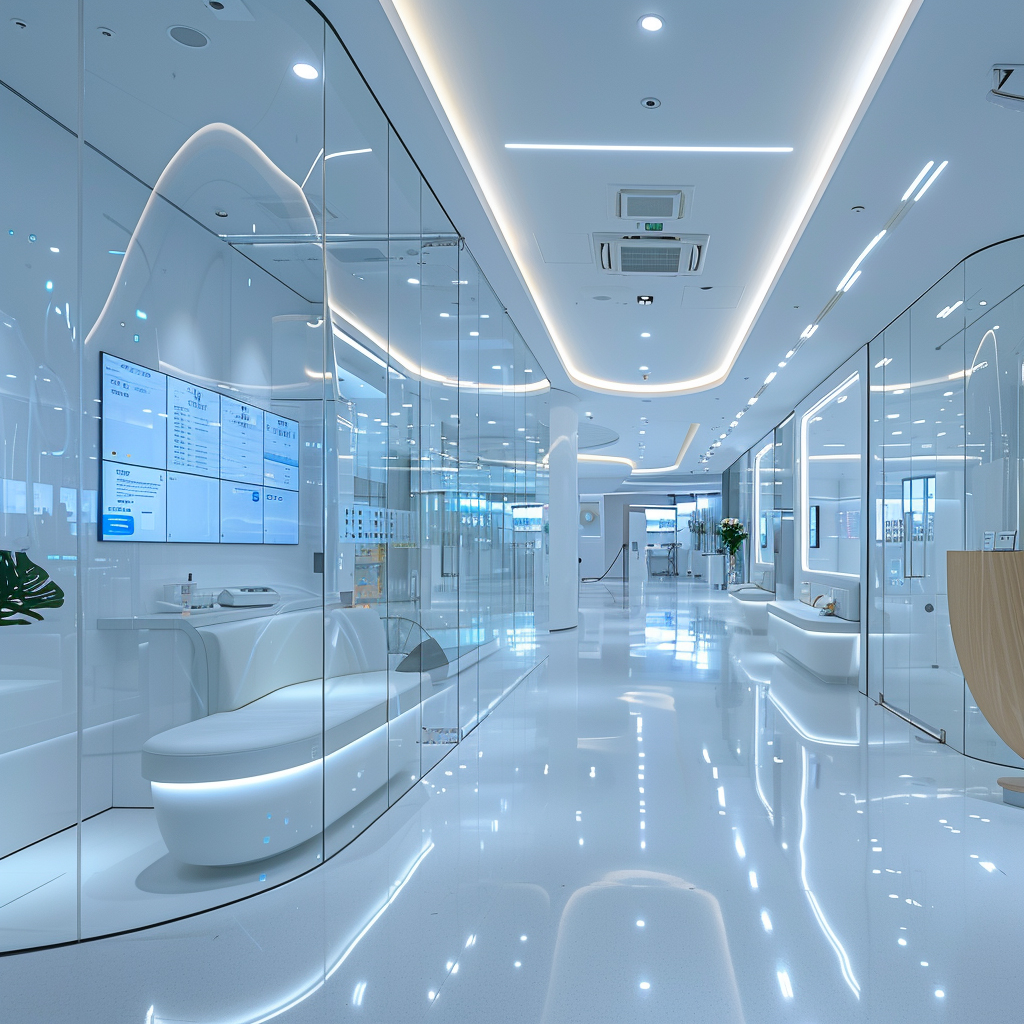
By automating routine administrative tasks in healthcare settings, such as data entry, appointment scheduling, and patient tracking, AI frees up medical professionals to spend more time on direct patient care. This not only increases operational efficiency but also enhances the quality of care by allowing providers to focus more on patient interaction and treatment.
8. Virtual Health Assistants
AI-powered virtual assistants provide personalized healthcare guidance and reminders for medication, exercise, and nutrition based on the user’s health data and preferences.
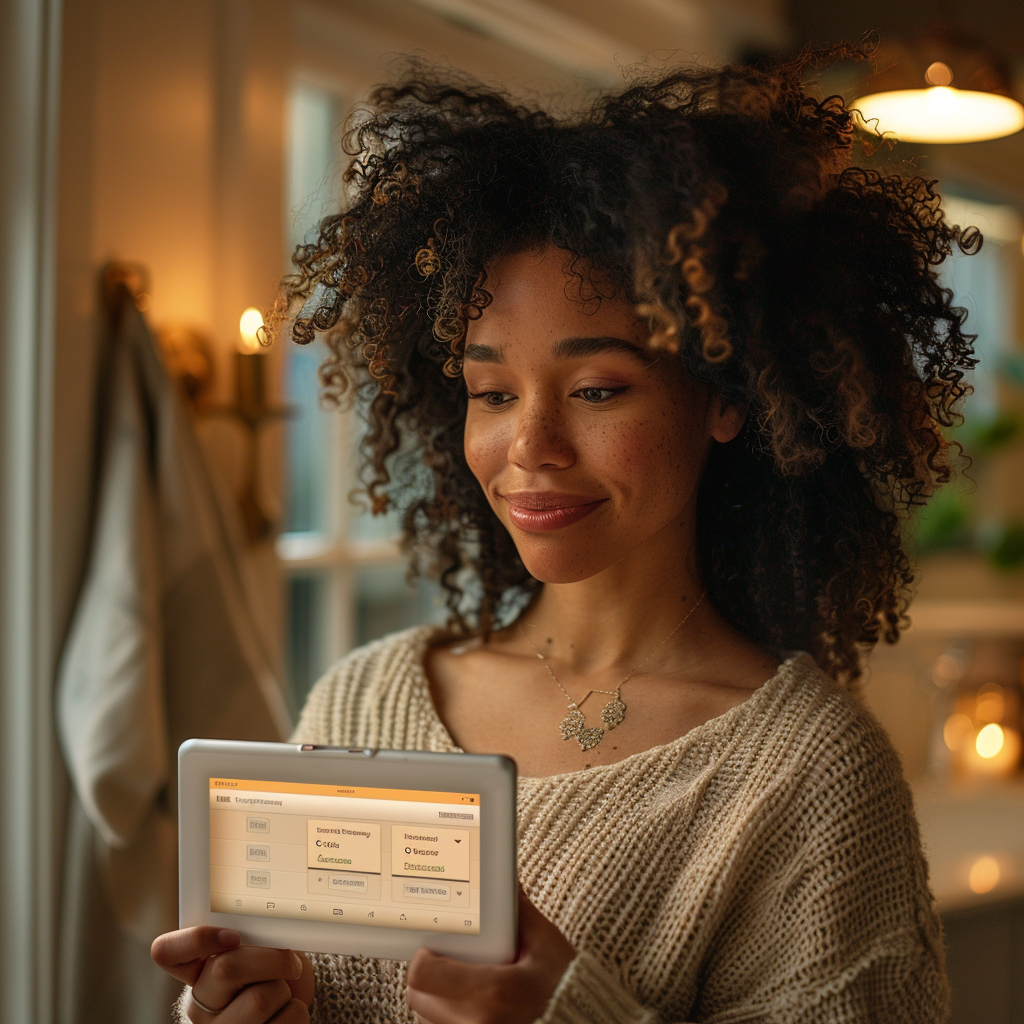
AI-powered virtual health assistants provide personalized health management support, offering reminders for medications, appointments, and health-related activities. These assistants use individual health data to tailor their interactions, ensuring that recommendations are based on the specific health needs and goals of each user.
9. Healthcare Bots for Patient Engagement
AI-driven chatbots engage with patients, providing personalized advice and answering questions based on individual health records, improving patient engagement and compliance with treatment plans.
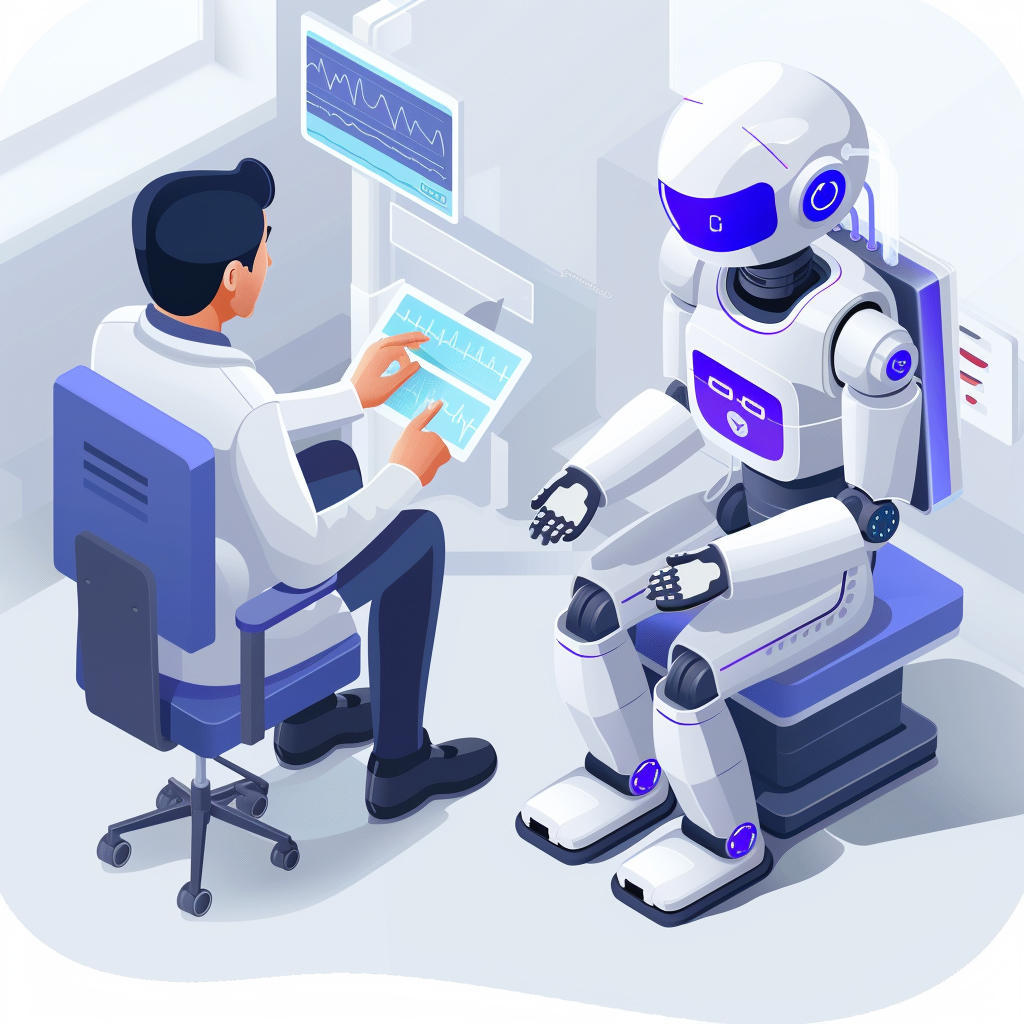
AI-driven healthcare bots engage patients by providing customized health advice, answering questions, and facilitating communication between visits. These bots analyze individual health records to provide consistent, personalized support, improving patient adherence to treatment plans and enhancing overall engagement with their health care.
10. Advanced Prosthetics
AI is used to design and operate advanced prosthetic devices that are customized to the specific anatomical and functional needs of patients, enhancing their mobility and quality of life.
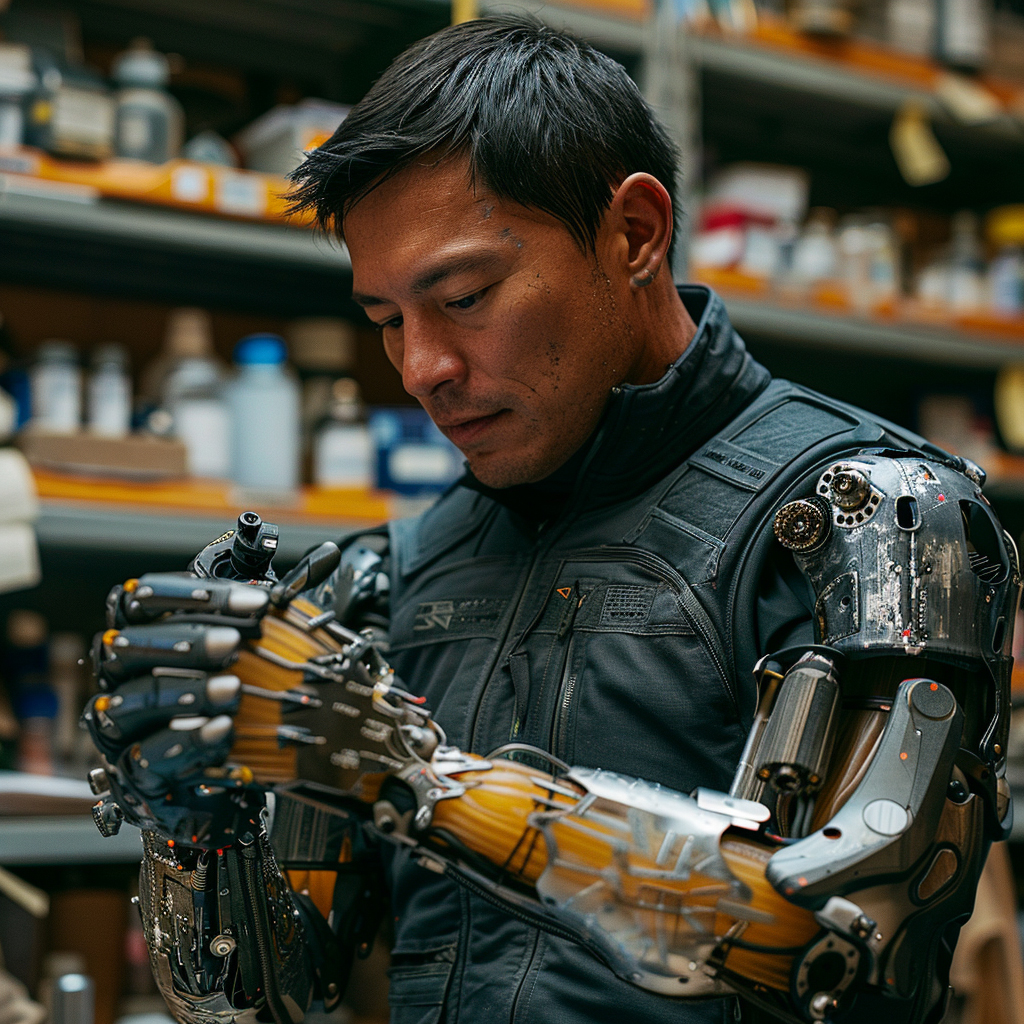
AI is used in the design and functioning of advanced prosthetic devices, ensuring that these devices meet the unique anatomical and functional needs of patients. Through AI, prosthetics can be precisely calibrated for optimal performance according to the movement patterns and lifestyle of the user, significantly improving the usability and comfort of prosthetics.