1. Electronic Health Records (EHR) Integration
AI streamlines the integration of diverse health data sources into EHRs, ensuring comprehensive and accessible patient records.
-integration-1.jpg)
AI significantly enhances the integration of diverse healthcare data sources into Electronic Health Records (EHRs). By automating the aggregation and organization of data from different systems and formats, AI ensures that EHRs are comprehensive, up-to-date, and readily accessible. This streamlined integration aids healthcare providers in accessing complete patient histories, improving diagnostic accuracy and treatment effectiveness.
2. Predictive Analytics
AI utilizes historical patient data to predict future health events or conditions, enabling proactive care and personalized treatment plans.
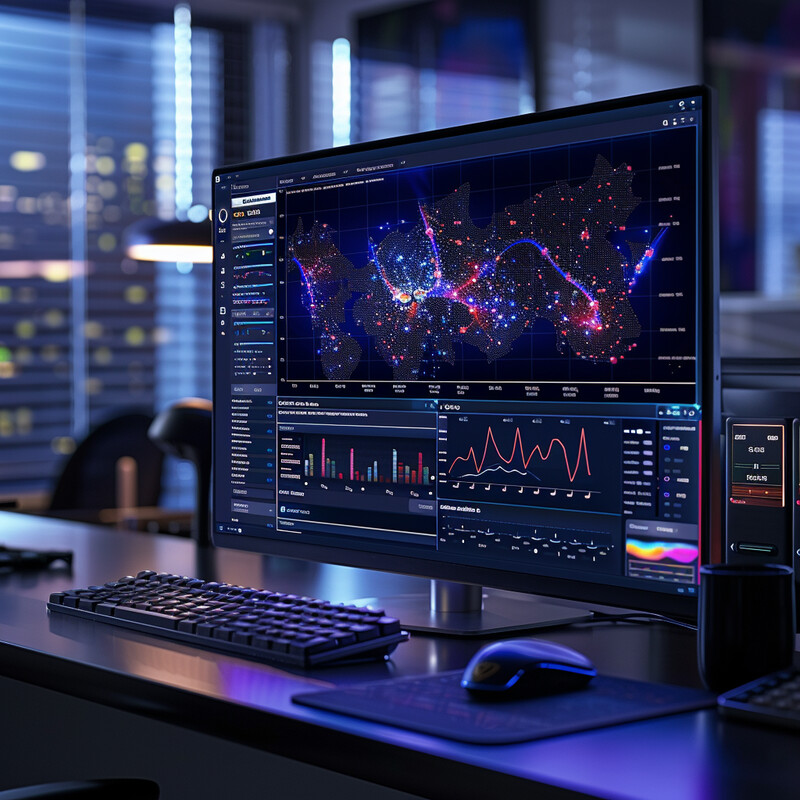
AI leverages historical patient data to forecast future health events or conditions, enabling healthcare providers to anticipate patient needs and tailor preventive or therapeutic interventions. Predictive analytics can identify patients at risk of chronic diseases, hospital readmission, or other adverse health outcomes, allowing for interventions that can prevent deterioration and improve patient outcomes.
3. Data Security and Privacy
AI improves the security and privacy of patient data through advanced algorithms that detect and counter potential cyber threats and breaches.
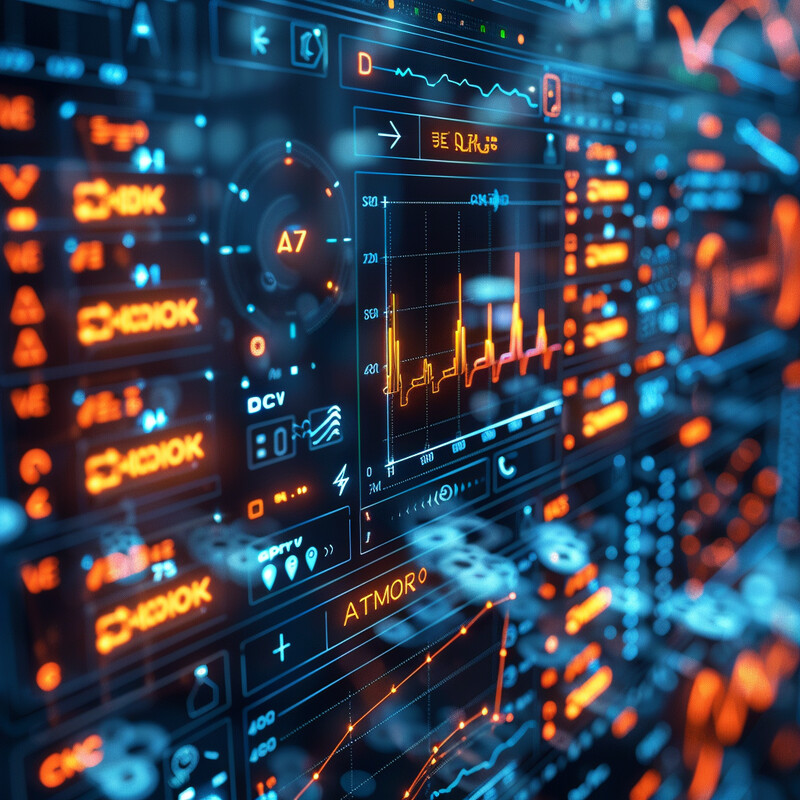
AI enhances the security and privacy of patient data through sophisticated algorithms that monitor and analyze data access patterns, detect anomalies, and prevent unauthorized access and data breaches. These systems are crucial in maintaining the integrity of sensitive health information and complying with regulatory requirements such as HIPAA.
4. Natural Language Processing (NLP)
AI uses NLP to understand and organize unstructured data from clinical notes, converting it into structured, actionable information in patient records.
-3.jpg)
AI employs natural language processing (NLP) to extract and structure valuable information from unstructured clinical notes and narrative text entries in medical records. NLP enables the conversion of this text into standardized data formats that can be easily searched and analyzed, improving data usability and supporting better clinical decision-making.
5. Real-time Data Analysis
AI processes real-time data from medical devices and health monitors, providing healthcare professionals with timely insights for immediate decision-making.
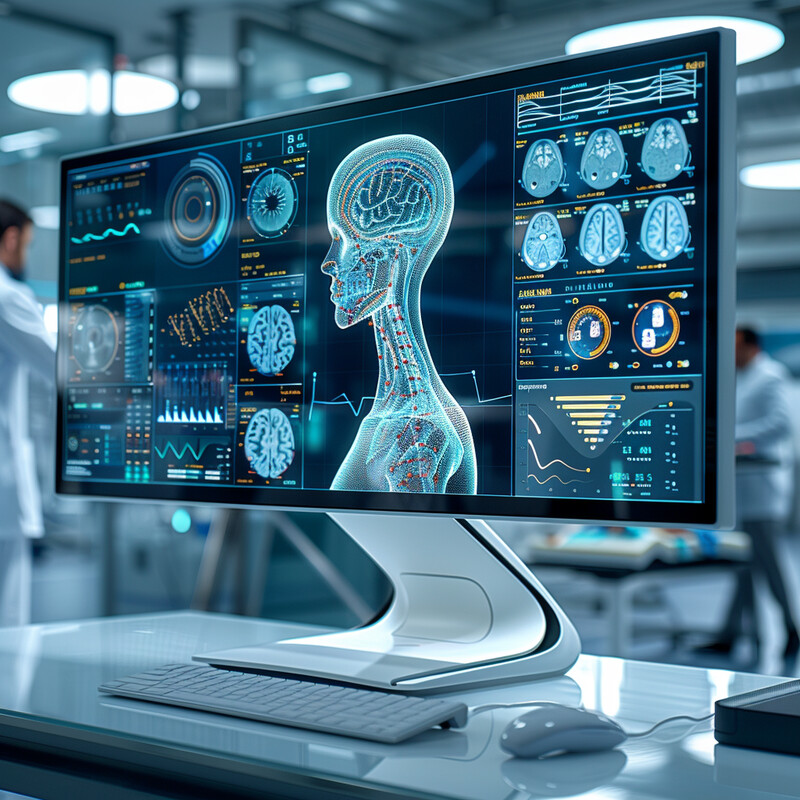
AI processes and analyzes real-time data streaming from medical devices and health monitors, providing instant insights into patient conditions. This capability allows healthcare professionals to make informed decisions quickly, potentially saving lives in critical care situations by highlighting urgent abnormalities that require immediate attention.
6. Patient Data Access and Sharing
AI facilitates secure and efficient sharing of patient data among healthcare providers, ensuring coordinated care and eliminating redundant tests and procedures.
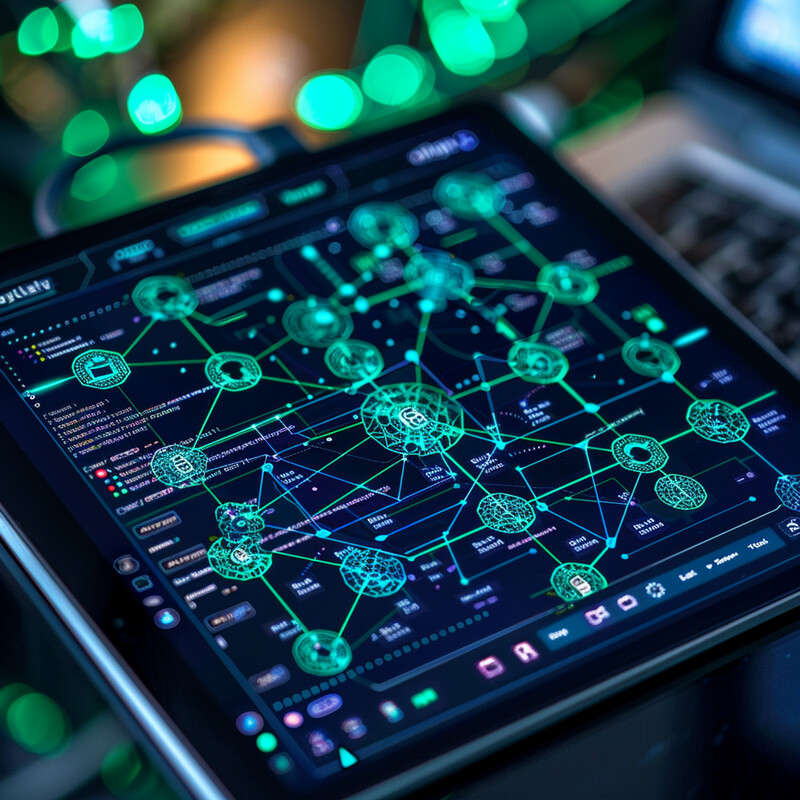
AI facilitates the efficient and secure sharing of patient data across different healthcare providers. By ensuring that all relevant parties have access to the same comprehensive data sets, AI supports coordinated care, reduces the likelihood of duplicate tests and conflicting treatments, and ultimately enhances the overall healthcare experience for patients.
7. Image Analysis
AI enhances the analysis of medical images (such as MRIs, CT scans, and X-rays) with greater accuracy and speed, leading to quicker diagnoses and treatments.
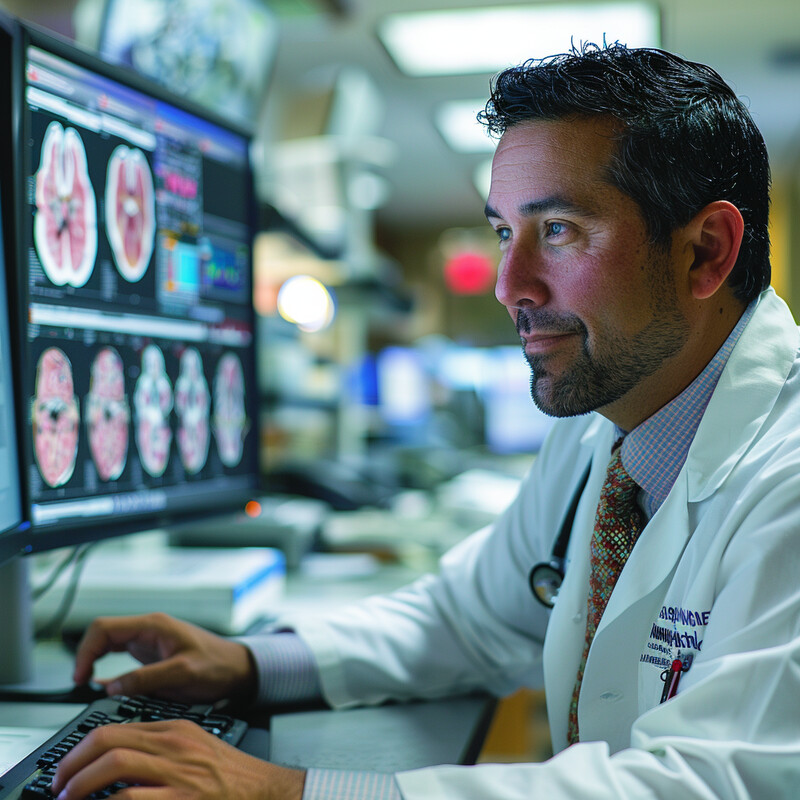
AI technologies significantly improve the analysis of medical imaging such as MRIs, CT scans, and X-rays. By providing automated, high-precision assessments of images, AI assists radiologists and other specialists in diagnosing conditions more quickly and accurately, often detecting subtleties that may be overlooked in manual reviews.
8. Automated Alerts and Reminders
AI systems generate alerts and reminders for healthcare providers about important patient events, such as medication doses or follow-up appointments, enhancing patient compliance and care continuity.
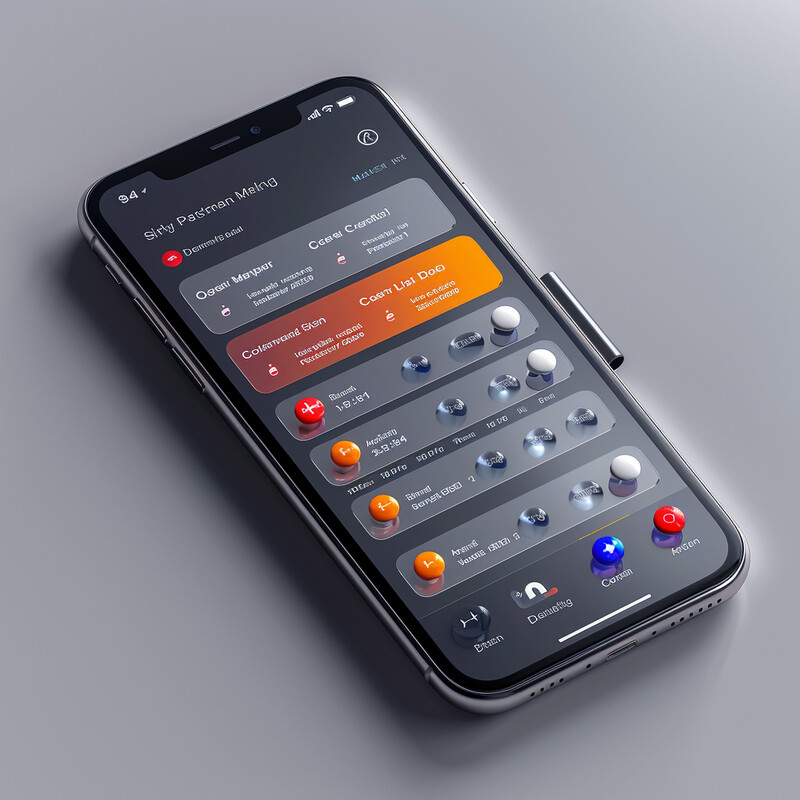
AI systems generate automated alerts and reminders for healthcare providers regarding critical patient care activities, such as medication administration times, upcoming appointments, or preventive care needs. These reminders help ensure that nothing is overlooked in patient care routines, improving adherence to treatment protocols and patient safety.
9. Chronic Disease Management
AI analyzes data trends over time to assist in managing chronic diseases, providing insights that help tailor treatment approaches to individual patient needs.
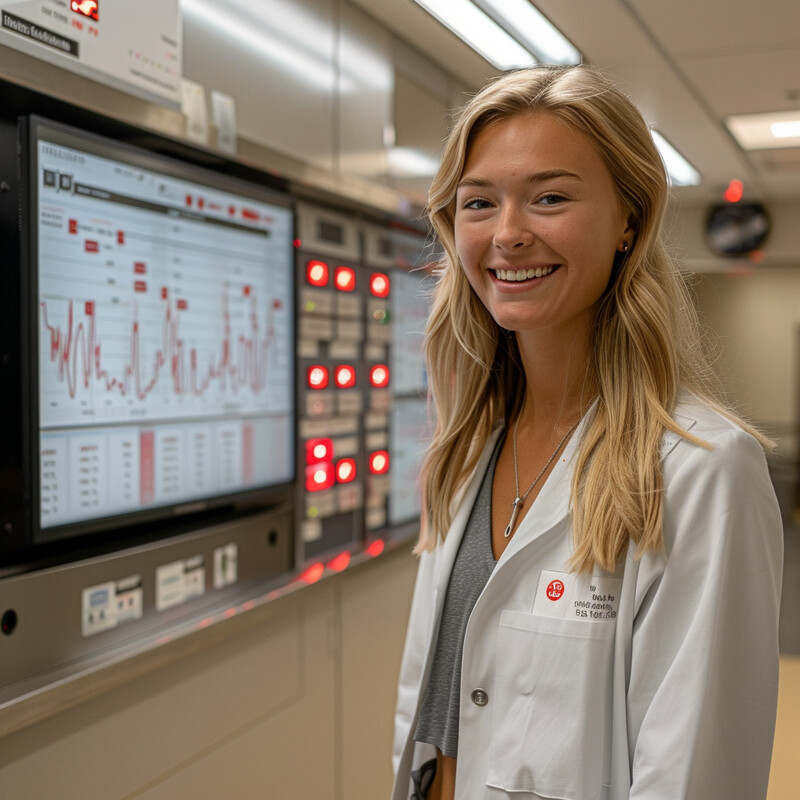
AI analyzes longitudinal patient data to aid in the management of chronic diseases. By tracking how patients respond to different treatments over time, AI can help clinicians adjust medications and other interventions in a manner that is specifically tailored to each patient’s evolving needs, enhancing the efficacy of chronic disease management.
10. Clinical Trial Matching
AI matches patients with appropriate clinical trials based on their detailed health profiles, facilitating the advancement of medical research and providing patients with access to cutting-edge treatments.
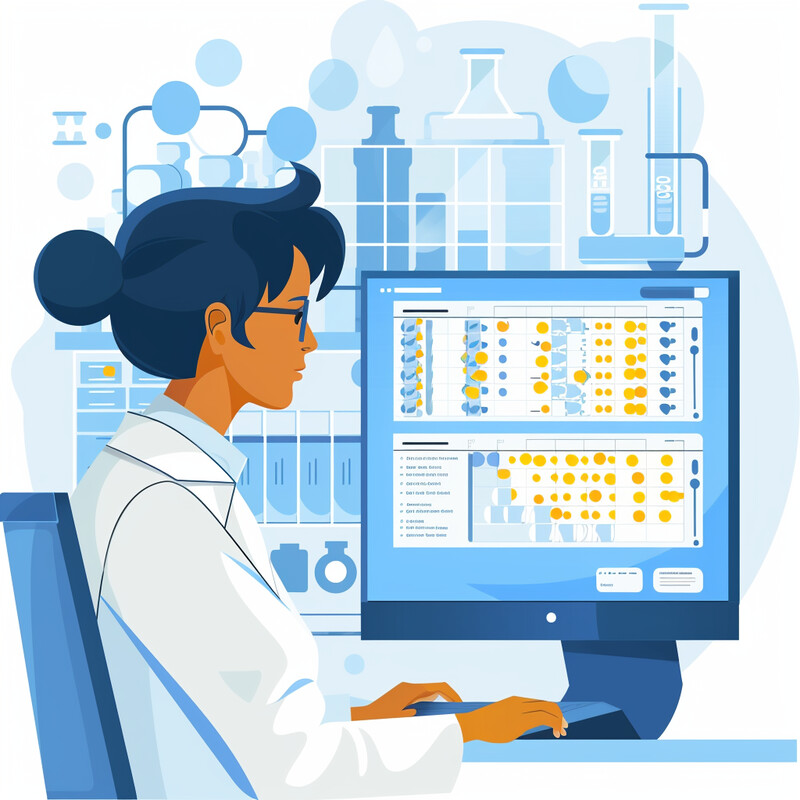
AI automates the process of matching patients with clinical trials by analyzing detailed health profiles against inclusion and exclusion criteria for various studies. This not only speeds up the recruitment process for research but also provides patients with opportunities to access new and potentially life-saving treatments that are specifically suited to their health conditions.