1. Improved Accuracy on Unstructured Texts
Artificial intelligence has greatly increased OCR accuracy, especially for documents with irregular layouts or mixed content. Modern deep learning OCR can handle invoices, receipts, and handwritten notes that previously confounded rule-based systems. By training on diverse fonts and writing styles, AI models minimize errors when extracting text from complex, unstructured documents. These systems continuously refine their character recognition through large datasets, approaching human-level accuracy in many cases. In essence, AI enables OCR to reliably read text in situations (e.g. messy forms or overlapping text) that older OCR struggled with, drastically improving data extraction from unstructured sources.
AI algorithms have increased OCR accuracy even on unstructured text layouts, such as invoices, receipts, and handwritten notes, by better recognizing various fonts and handwriting styles.
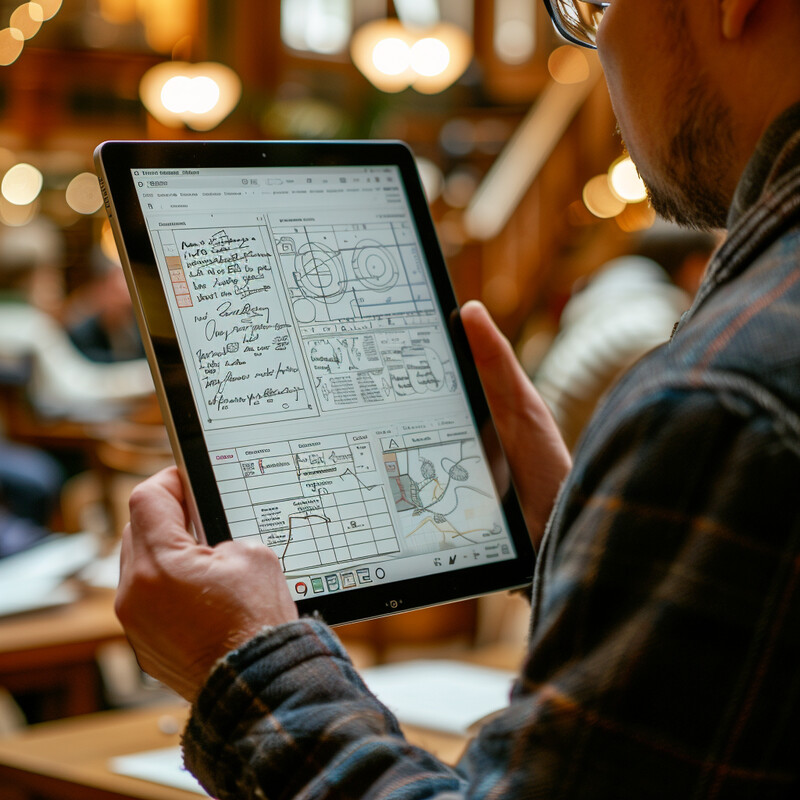
Recent benchmarks demonstrate the leap in accuracy achieved with AI-based OCR on unstructured content. For example, in a 2025 evaluation, Google’s Vision OCR (leveraging deep learning) correctly extracted about 98% of text from a mixed set of printed and handwritten documents – a level of performance far beyond traditional OCR on such complex inputs. Across many OCR tools tested, all exceeded 99% accuracy on clean typed text, but the AI-powered models distinguished themselves by maintaining high accuracy even on jumbled layouts and cursive handwriting. This underscores how AI algorithms, trained on diverse and difficult formats, significantly boost OCR accuracy for unstructured or noisy texts.
AI has significantly advanced OCR capabilities in handling unstructured text formats. By leveraging deep learning techniques, OCR can now accurately recognize and extract text from complex documents such as invoices, receipts, and handwritten notes. These AI models are trained on diverse datasets, enabling them to decipher a wide range of fonts and handwriting styles, thus minimizing errors and improving data extraction accuracy.
2. Language Recognition
AI-driven OCR systems now support a far wider range of languages and scripts than earlier generations. Machine learning allows OCR to automatically detect multiple languages in a document and accurately convert each to text. This multilingual capability means a single OCR engine can handle English, Chinese, Arabic, Cyrillic alphabets, and even lesser-known languages without separate setups. AI models learn the character patterns and context of different languages, improving recognition accuracy for each. As a result, businesses and users can apply one OCR solution globally, benefiting from expanded language support and even translation features built on the OCR output.
AI-powered OCR systems can now recognize and accurately translate text from multiple languages, expanding their usability globally.
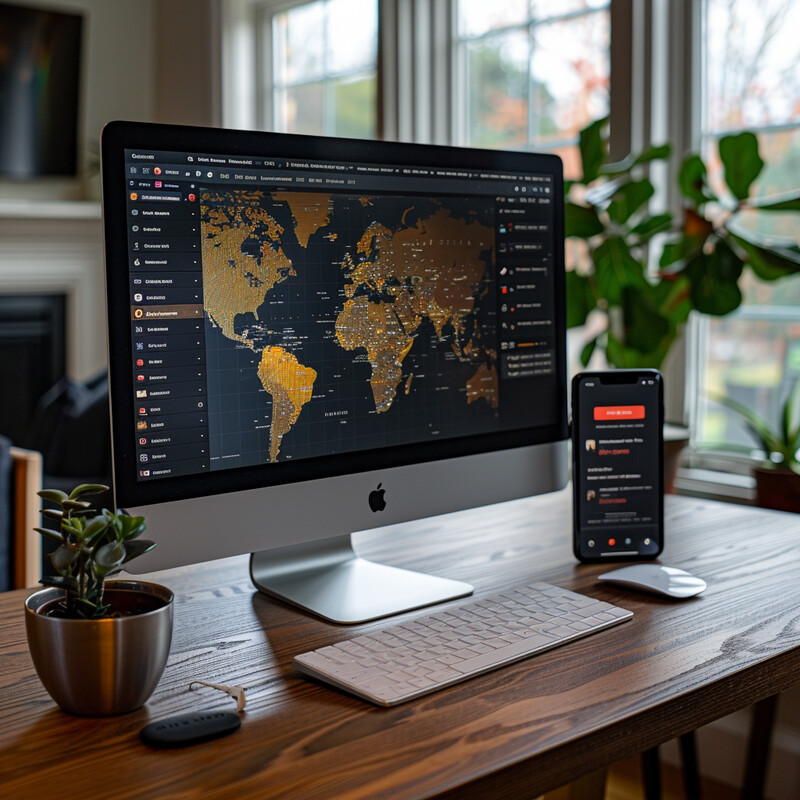
Thanks to AI training on multilingual data, today’s top OCR software can recognize hundreds of languages. For instance, ABBYY’s AI-enhanced FineReader OCR can read text in 198 languages, including non-Latin scripts like Burmese and Tibetan, as well as historical or cursive scripts. This broad language recognition is achieved by deep neural networks that have been fine-tuned with diverse language datasets, including rare dialects and ancient manuscripts. Such AI OCR tools also handle right-to-left scripts (e.g. Arabic) and vertical text (as in East Asian languages) seamlessly. The ability to accurately digitize documents in nearly 200 languages highlights how AI has made OCR a truly global, multilingual technology.
Modern AI-powered OCR systems can recognize and process multiple languages, greatly enhancing their applicability in global contexts. This feature is particularly useful for businesses and organizations that deal with international documents, as it allows for automatic language detection and accurate text conversion, facilitating smoother communication and workflow across different linguistic environments.
3. Contextual Understanding
Artificial intelligence enables OCR to go beyond raw character reading and actually understand context. By integrating Natural Language Processing (NLP) techniques and even large language models, AI-powered OCR can interpret the meaning of the text it transcribes. This means the OCR can correct errors using surrounding words (context clues) and better handle ambiguous characters or abbreviations. In complex documents like contracts or medical records, context-aware OCR can discern that a certain term is a medication name or a legal clause based on context, reducing misreads. Overall, AI gives OCR a form of comprehension – the system doesn’t just see letters in isolation, but also grasps how they form words and sentences in context, leading to more accurate and useful text outputs.
AI integrates with Natural Language Processing (NLP) to understand the context of the words it scans, improving the accuracy of text interpretation, especially in complex documents like legal contracts or medical records.
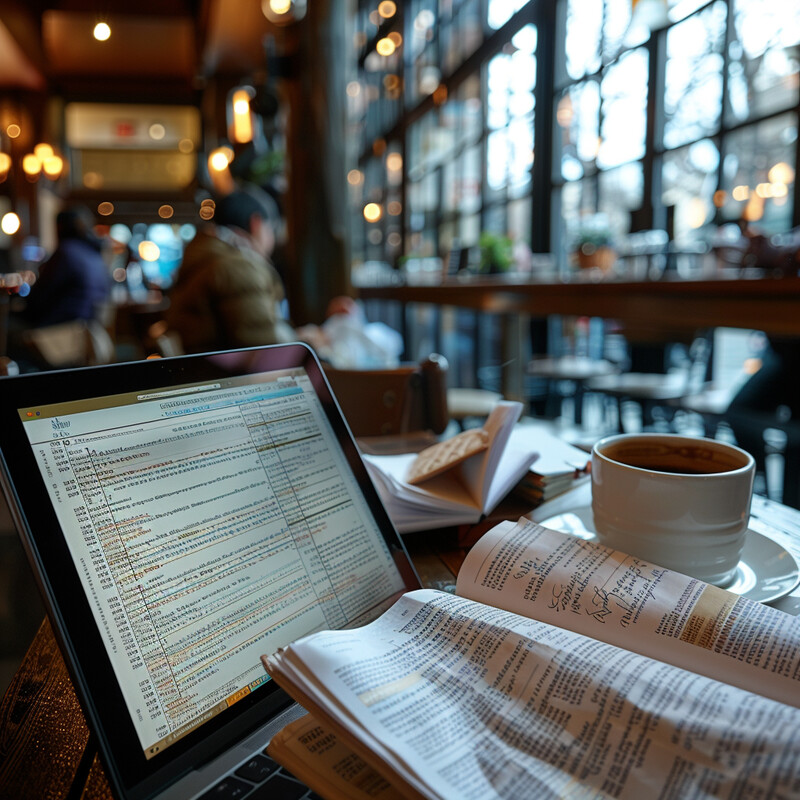
Research shows that coupling OCR with AI language models markedly improves interpretation of scanned text. Mahadevkar et al. (2024) note that incorporating an NLP component allows an OCR system to use context for error correction – for example, distinguishing “O” from “0” or deciphering a partially obscured word by its surrounding text. In practice, an OCR enhanced by a large language model can understand the context of what it’s reading and fix many mistakes that a traditional engine would make. This was demonstrated in legal documents where AI-OCR identified terms correctly by considering neighboring words, and in a case where an OCR confused “1O” for “10,” an AI model recognized from context that “10” was intended). Such context-driven corrections show how AI gives OCR a deeper understanding of text, greatly refining accuracy in real-world documents.
Integrating OCR with Natural Language Processing (NLP) enables the system to understand the context surrounding the scanned text. This is especially beneficial in complex and specialized documents like legal contracts or medical records, where understanding the context can significantly affect the interpretation of the information. AI-driven contextual understanding helps ensure that the text is not only extracted but also correctly interpreted and used.
4. Real-time Processing
AI has enabled OCR to operate in real time, processing images and video streams almost instantly. Advanced neural networks run efficiently on modern hardware (GPUs, mobile chips), so text can be extracted from camera input on the fly. This allows use cases like live translation apps (point your phone at a sign and see the translation immediately) or instant data capture from video feeds (e.g. reading license plates on moving cars). The speedups come from both algorithmic improvements – deep learning models that quickly detect and recognize text – and optimizations like processing multiple characters/words in parallel. As a result, AI-powered OCR can handle high volumes (pages per second) and deliver immediate results, drastically improving productivity and user experience for scanning and translating tasks.
AI has enabled OCR technology to process documents in real-time, greatly reducing the time from scanning to text conversion, which is particularly useful in dynamic environments like airports or train stations.
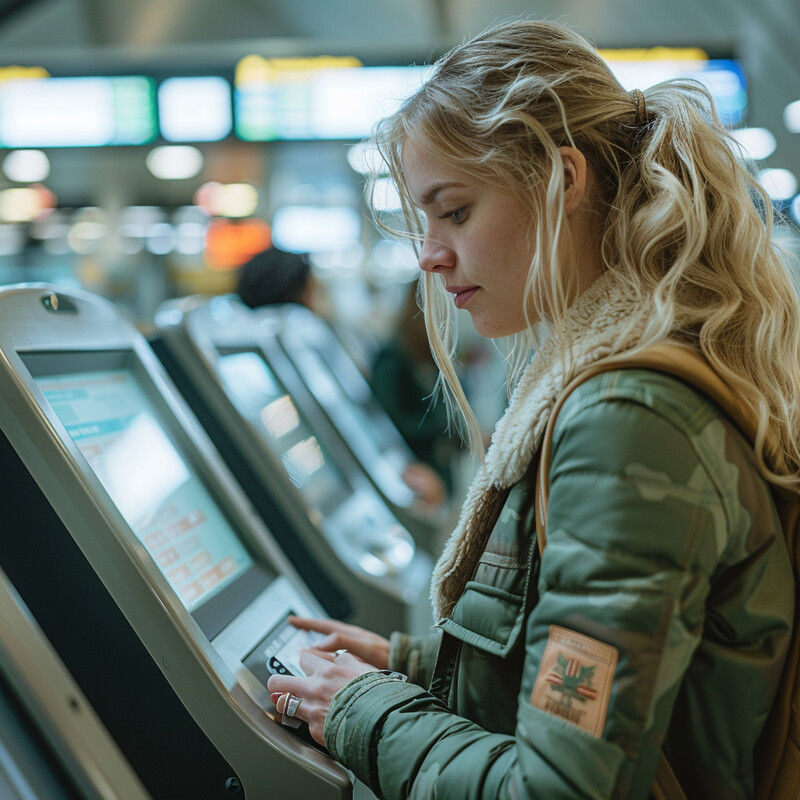
The efficiency of AI-driven OCR is evident in its throughput: modern systems can handle dozens of pages per minute, enabling near-instantaneous text capture. In 2024, industry observers noted that some mobile OCR apps (enhanced with AI) now process over 60 pages per minute, whereas older methods might take several seconds per page. This high speed means a user can scan a multi-page document with a smartphone and have all text digitized in seconds. Likewise, AI OCR integrated with video can extract text from each frame fast enough to act in real time – for example, reading and displaying translations of restaurant menus as a user’s camera hovers over them. These real-time capabilities, powered by optimized deep learning models, show how AI has made OCR fast enough for live use cases that were impractical before.
AI has enabled OCR technology to perform real-time document scanning and text recognition. This capability is crucial in environments requiring immediate data processing, such as during boarding pass checks at airports or identity verification at registration desks. Real-time OCR helps streamline operations and reduce wait times, enhancing overall efficiency.
5. Integration with Other Systems
Modern OCR solutions powered by AI are designed for easy integration into larger workflows and software ecosystems. Instead of being standalone tools, AI OCR often comes with APIs and modules that allow it to plug into business processes, document management systems, and robotic process automation (RPA) bots. For instance, an AI OCR engine can feed extracted data directly into a database or trigger an automated workflow once text is recognized. This integration is enhanced by AI’s flexibility – the OCR can adapt to various document sources and formats without heavy reconfiguration, making it a versatile component. The end result is that companies can seamlessly incorporate OCR into their digital pipelines (like automatically reading incoming forms and updating records), greatly streamlining operations.
AI-enhanced OCR systems easily integrate with other digital systems, such as document management systems or customer relationship management (CRM) tools, allowing for seamless data extraction and storage.
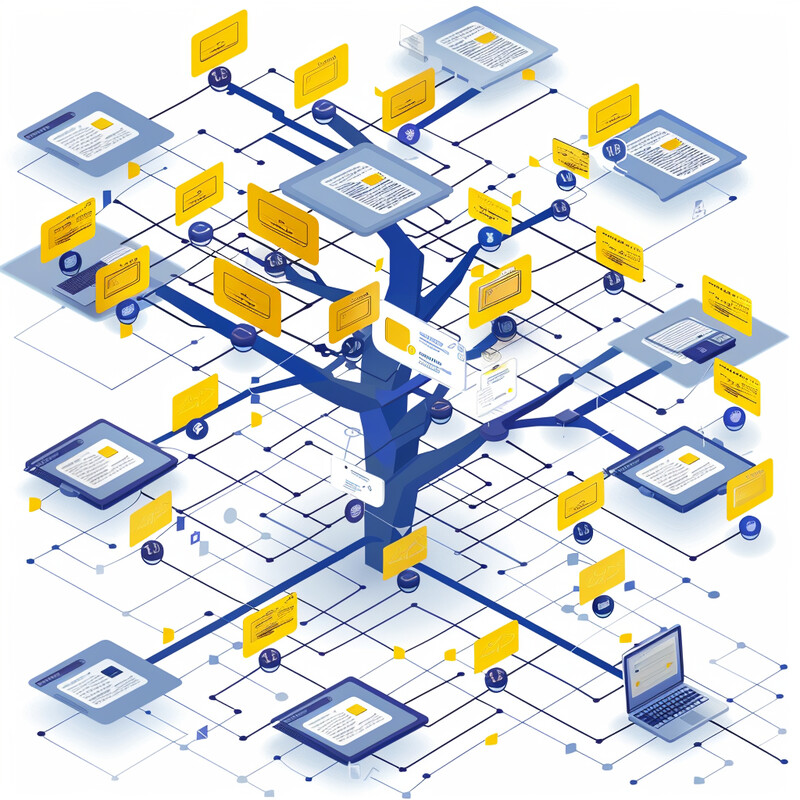
AI-powered OCR is now a key part of intelligent automation platforms. A recent study noted that enterprise RPA tools (such as UiPath) natively integrate leading OCR engines from Google and Microsoft, enabling end-to-end automation of document-centric tasks. For example, an RPA workflow for invoice processing can use AI OCR to read each invoice, an NLP module to interpret the fields, and then automatically enter the data into an accounting system – without any human steps. The AI ensures the OCR can handle the variety of real invoices received (different layouts, languages, etc.), making the integration robust. This tight coupling of AI OCR with other systems has been transformative: common processes like accounts payable, KYC checks, and insurance claims are now often fully automated, with the OCR acting as the “eyes” that feed text data into complex digital workflows.
AI-enhanced OCR systems can seamlessly integrate with various digital platforms such as enterprise resource planning (ERP) systems, document management systems, or customer relationship management (CRM) tools. This integration facilitates the automatic extraction and direct storage of data, eliminating manual data entry and associated errors, thus improving operational efficiency.
6. Enhanced Security Features
AI is also improving the security of OCR systems, enabling them to detect fraud and protect sensitive information. Traditional OCR would blindly read whatever is on the page, but AI-driven OCR can analyze documents for signs of tampering or forgery while reading them. For instance, machine learning models can flag if a font style changes suspiciously in the text (possibly indicating someone altered a number on a form) or if there are inconsistent spacing/artifacts that suggest digital editing. Additionally, AI OCR can be combined with security protocols to automatically redact personal data or watermark outputs to prevent misuse. These enhanced security features mean OCR isn’t just about reading text – it’s ensuring the integrity and privacy of that text, which is crucial for documents like IDs, financial statements, and legal papers.
AI improves the security aspects of OCR by providing more robust tools for detecting and redacting sensitive information from documents before they are processed or shared.
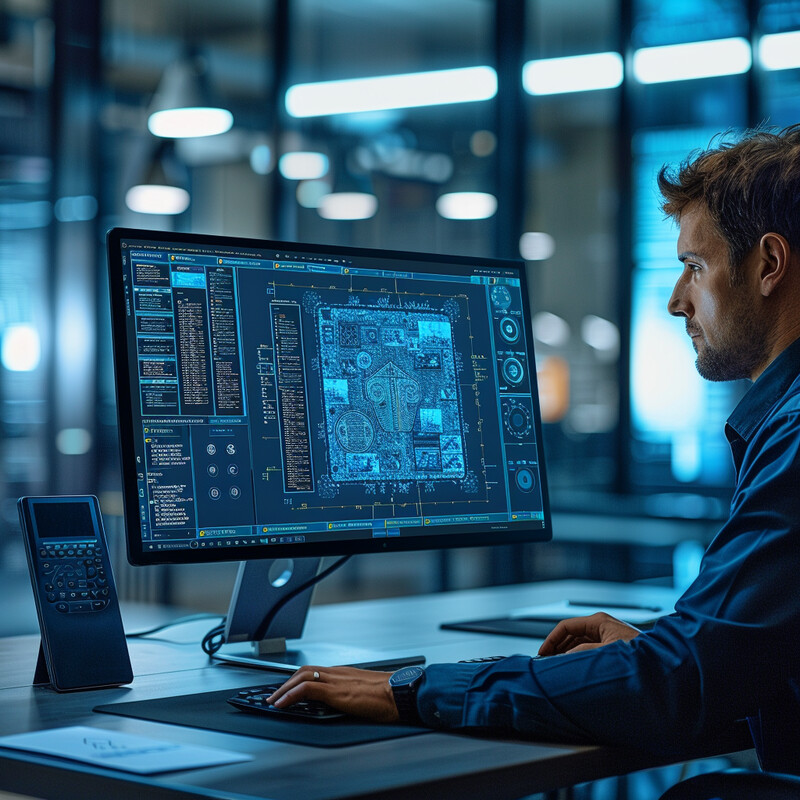
AI-based OCR systems now include fraud detection algorithms to bolster document security. A 2025 fintech study highlighted that anomaly detection can be built into OCR to catch subtle irregularities – for example, flagging mismatched fonts, abnormal spacing, or altered logos on a scanned document as potential evidence of forgery. In practice, if someone tries to edit a scanned invoice (e.g. changing an amount), an AI OCR service might notice that the numeric font is slightly different or that pixel-level noise patterns differ in that area, and it would raise an alert. This kind of intelligent scrutiny was not possible with older OCR but is now standard in AI-driven document processing for banks and government agencies. In short, AI-OCR not only reads text but also verifies authenticity and flags anomalies, providing a security layer during data extraction.
AI technologies enhance the security features of OCR by providing advanced tools to detect and redact sensitive information from documents automatically. This is crucial for complying with data protection regulations and safeguarding personal information, making OCR technology safer for processing confidential documents.
7. Image Quality Improvement
AI is used to enhance the quality of images before or during the OCR process, which dramatically improves recognition results. Often, documents are scanned in poor conditions – low resolution, shadows, noise, or faded text. AI techniques (like neural networks for super-resolution and denoising) can clean and correct these images so that the text becomes clearer for OCR. For example, an AI model can sharpen a blurry scan, remove background noise, correct skew or perspective, and even reconstruct missing parts of letters. By preprocessing images in this intelligent way, OCR systems can achieve much higher accuracy on what would otherwise be unreadable documents. This is especially valuable for historical archives, photographs of text, or any non-ideal inputs where simply running OCR raw would yield errors.
AI algorithms preprocess images to enhance clarity and contrast before text extraction, which is crucial for dealing with low-quality scans or photos taken in poor lighting conditions.
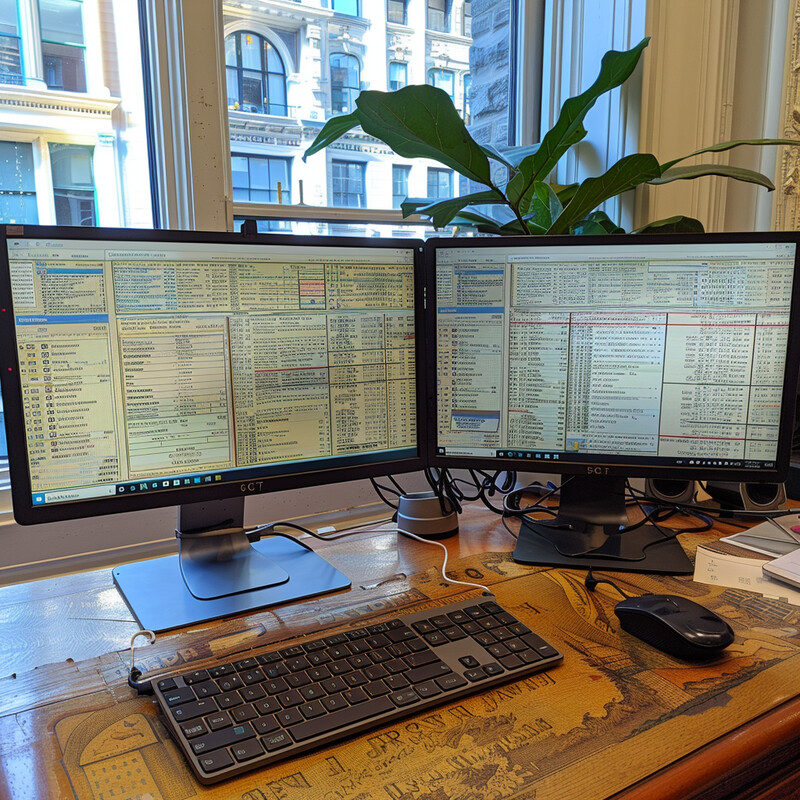
Studies confirm that applying AI-based image enhancement boosts OCR accuracy significantly. This was demonstrate by using a deep learning super-resolution model (BSRGAN) on low-resolution document images prior to OCR. The result was a substantial reduction in OCR error rates across multiple test datasets – in other words, many more words were correctly recognized after the images were AI-enhanced. The enhanced pipeline could even make faded ID cards and noisy receipts legible to the OCR, where the original scans had poor readability. In practical terms, this means an OCR system with AI image preprocessing can, for instance, extract text from a 100 DPI scan with the accuracy you’d expect from a 300 DPI scan. By cleaning up distortions, removing shadows, and sharpening text edges, AI ensures the OCR is “seeing” the best possible image, thus improving overall recognition outcomes.
Before extracting text, AI algorithms preprocess images to improve their quality, enhancing clarity and adjusting contrast. This preprocessing is vital for dealing with documents that are scanned under suboptimal conditions, such as low light or high-speed environments, ensuring that the text extraction remains accurate despite poor original image quality.
8. Adaptive Learning
One of the hallmark advantages of AI-driven OCR is adaptive learning – the system improves over time as it processes more data. Unlike static OCR software, an AI-based OCR can be retrained or fine-tuned with new examples, allowing it to get better with use. For instance, if the OCR consistently misreads a certain stylized font, developers can feed it more training samples of that font and the model will adjust its parameters to read it correctly in the future. Some advanced OCR services even learn on the fly: if a user corrects an output (says the model misread a word and the user fixes it), the AI can incorporate that feedback to avoid the mistake later. This continual learning means the accuracy and capabilities of the OCR are not fixed – they evolve, adapting to new document formats, novel handwriting styles, or any changes in the input domain. Over time, the OCR essentially becomes more knowledgeable and reliable through experience.
AI allows OCR systems to learn from corrections and adapt over time, continuously improving their accuracy and reducing the rate of errors in text recognition.
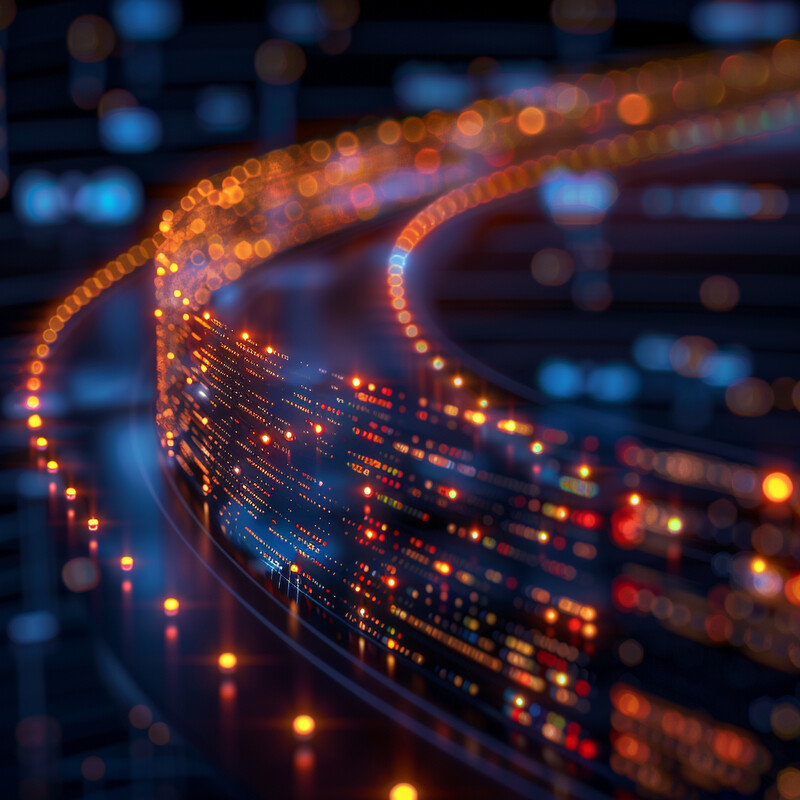
Modern AI-OCR systems are explicitly designed to continuously learn and improve. As one industry guide explains, advanced OCR models employ machine learning so that “they continuously learn and adapt based on feedback and new data”. In practical terms, a company might see their OCR accuracy climb from, say, 95% to 98% after a few months of active use, because the AI model retrained on the errors it initially made. Many vendors highlight this self-improving nature: the more documents you run through an AI-powered OCR, the smarter it gets at recognizing your specific documents. This adaptive learning also extends to learning new languages or formats on the go – for example, an AI OCR might not fully support a certain form at first, but after being trained on a dozen samples, it will start extracting the fields correctly. Such adaptability, driven by continuous machine learning, ensures AI-based OCR stays effective even as document types evolve.
AI enables OCR systems to learn from their outputs and adapt based on feedback. When corrections are made to OCR results, the system learns from these modifications, continuously refining its algorithms to reduce future errors. This adaptive learning capability ensures that OCR accuracy improves over time, adapting to specific user needs and document types.
9. Automation of Complex Workflows
AI-enhanced OCR is a catalyst for automating complex document workflows that involve multiple steps. In the past, even if OCR could digitize text, humans still had to interpret it or move the data to the next step. Now, AI OCR works in tandem with other AI components (like document classification, data validation, and business rule engines) to fully automate end-to-end processes. For example, in loan processing, an AI OCR can read all incoming application documents, an NLP model extracts key fields and checks for compliance, and an RPA bot feeds the data into a decision system – all automatically. This means entire workflows like invoice processing, mortgage approval, customs document clearance, or medical coding can be handled with minimal human intervention. AI makes OCR outputs structured and reliable enough that they can trigger other automated actions, effectively removing bottlenecks and manual data entry from complex workflows.
AI-powered OCR automates complex workflows by recognizing and categorizing different types of documents and extracting relevant information according to pre-defined rules.
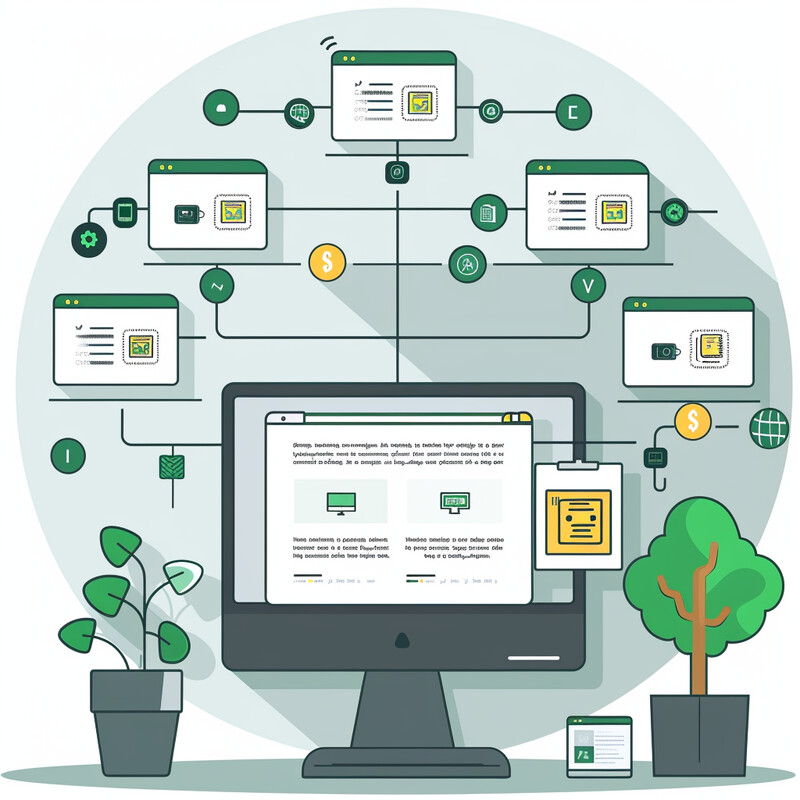
The impact of AI-OCR on workflow automation is reflected in industry trends and investment. Analysts project the Intelligent Document Processing (IDP) market – which centers on AI OCR integrated with workflow tools – to grow from about $2.4 billion in 2023 to $10.5 billion by 2028, a 35% annual growth rate. This rapid expansion is driven by organizations deploying AI to automate labor-intensive document workflows. Concretely, companies report that by using AI OCR in areas like insurance claims or customer onboarding, they have cut processing times from days to hours and reduced error rates drastically. For instance, a case study in 2024 showed a bank using AI OCR and automation to handle loan applications 70% faster, as documents that once waited in queues were processed straight-through by AI. These results underscore that AI-powered OCR isn’t just about reading text – it’s a pivotal technology enabling fully automated, complex business workflows at scale.
AI-driven OCR automates complex document-processing workflows by recognizing and categorizing different types of documents and extracting pertinent information according to predefined rules. This automation reduces the manual sorting and processing of documents, allowing organizations to handle larger volumes of data more efficiently and accurately.
10. Accessibility Features
AI improvements in OCR are making technology more accessible, especially for people with visual impairments. By leveraging AI OCR, assistive tools can convert printed text to speech or braille quickly and accurately, empowering blind or low-vision individuals to access written content in real time. AI helps by recognizing text even in challenging conditions (different fonts, low lighting) and by identifying context (so it can describe an entire scene or document structure, not just read characters). Features like currency recognition, scene description, or reading handwritten notes aloud are now feasible thanks to advanced OCR combined with object recognition – all driven by AI. In essence, AI-OCR is a backbone of modern accessibility apps, allowing them to interpret the visual world for those who cannot see, thereby greatly enhancing independence and quality of life.
AI-enhanced OCR technologies help create more accessible digital content by converting text from images and videos into readable or audible formats for people with visual impairments.
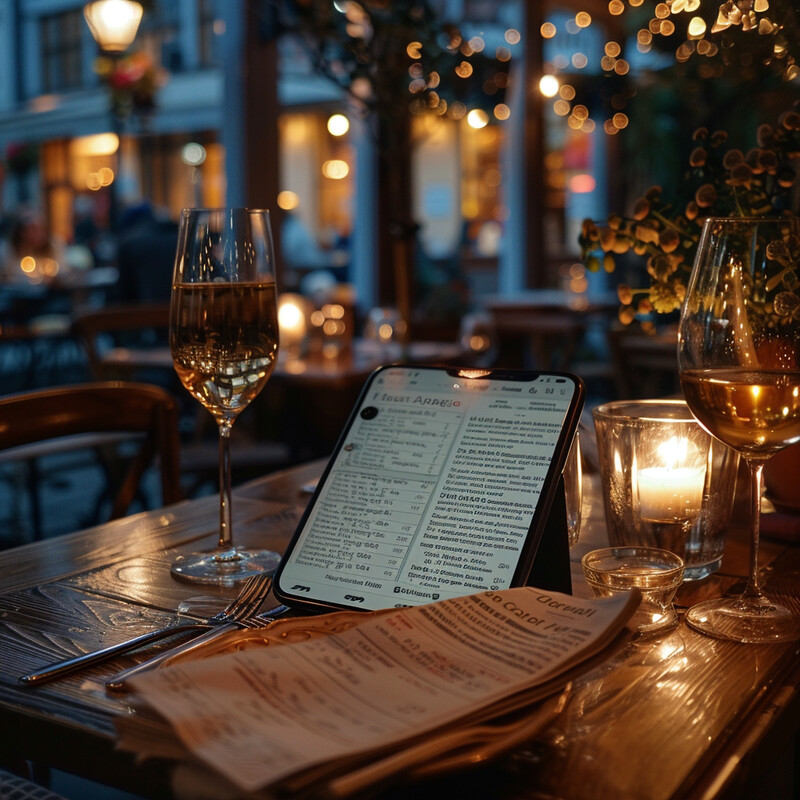
Assistive devices and apps using AI OCR have achieved remarkable accuracy, enabling users to perform everyday reading tasks independently. Researchers evaluated major AI-powered vision apps for the visually impaired and found they could reach 100% accuracy in reading printed text in tests (for example, apps like Microsoft Seeing AI and Google Lookout flawlessly read standard print documents). The same study noted around 90% accuracy for handwriting recognition in the best apps – a huge improvement over earlier tools that struggled with anything beyond clear print. In practical usage, this means a blind user can point their smartphone at a letter or restaurant menu and have the content read aloud almost perfectly. These advances illustrate how AI-driven OCR is breaking accessibility barriers: tasks like reading signs, labels, mail, and forms – once a significant challenge – are now routinely handled by personal AI assistants, granting visually impaired individuals greater autonomy in daily activities.
AI enhances the accessibility of OCR technologies by converting text found in images and videos into formats accessible to people with visual impairments, such as audio or large print. This application not only expands the usability of OCR but also promotes inclusivity, allowing individuals with disabilities better access to information in digital formats.