1. Improved Matchmaking Accuracy
AI analyzes user preferences, behaviors, and interaction patterns to provide highly accurate matchmaking, predicting compatibility between users more effectively.
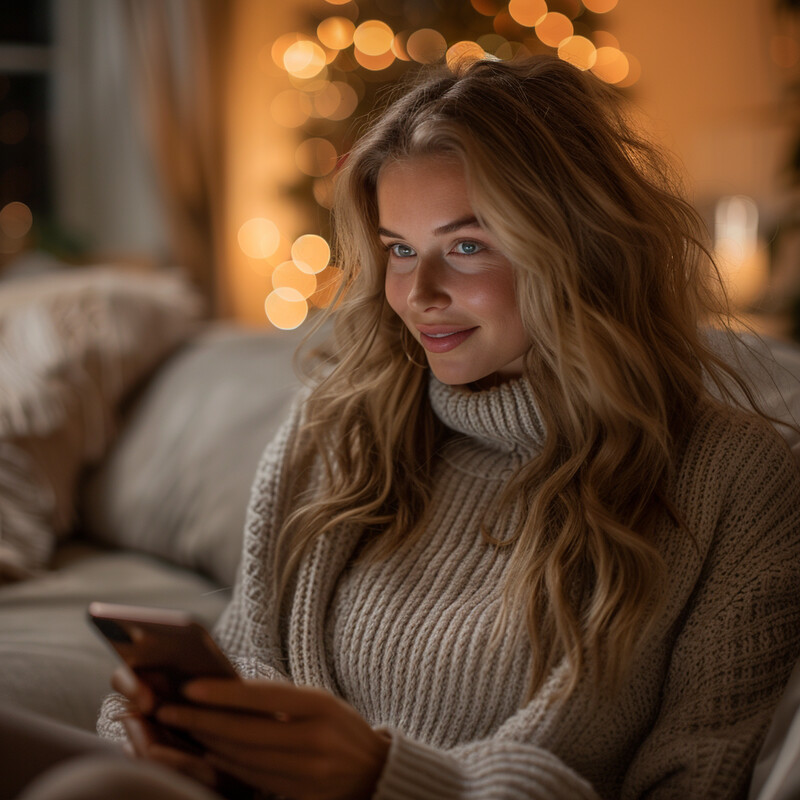
AI algorithms in online dating platforms analyze vast amounts of user data—including profile information, preferences, and in-app behavior—to provide highly accurate matchmaking. By understanding subtle preferences and patterns, AI can predict compatibility between users with remarkable precision, offering match suggestions that are more likely to result in successful and satisfying connections.
2. Behavioral Analytics
AI delves into behavioral data to understand user preferences more deeply, using this information to refine matchmaking algorithms and suggest potential matches that align more closely with individual user behaviors and preferences.
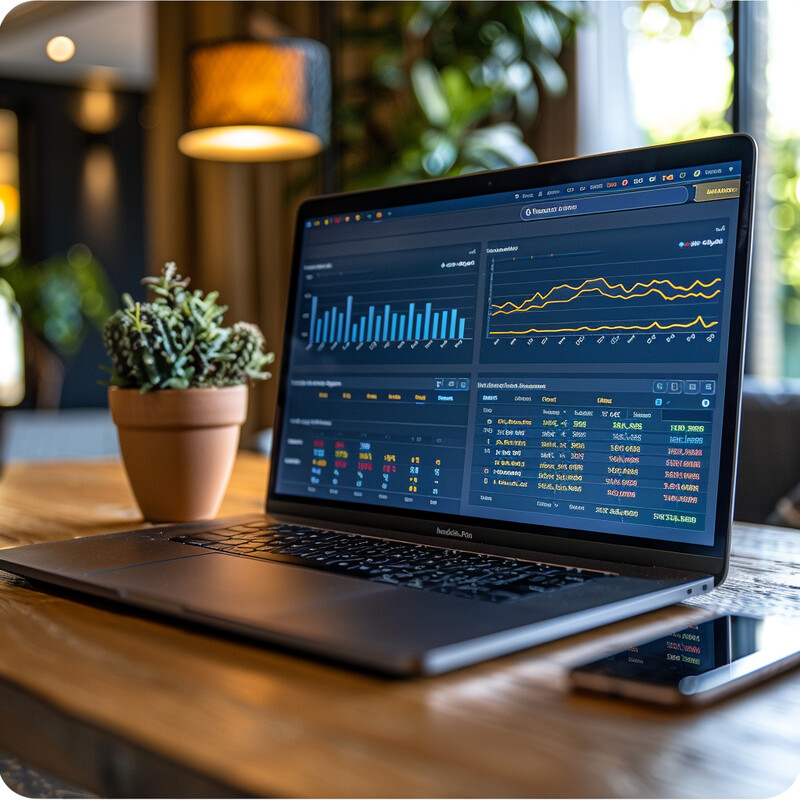
AI delves deep into users' behavioral data, such as their swiping patterns, interaction rates, and time spent on profiles. By examining these behaviors, AI refines its matchmaking algorithms to align more closely with each user's implicit preferences, enhancing the quality of match suggestions beyond explicit preferences listed in profiles.
3. Fraud Detection and Security
AI improves the safety and integrity of online dating platforms by identifying and flagging potentially fraudulent or malicious accounts, ensuring a safer dating environment.
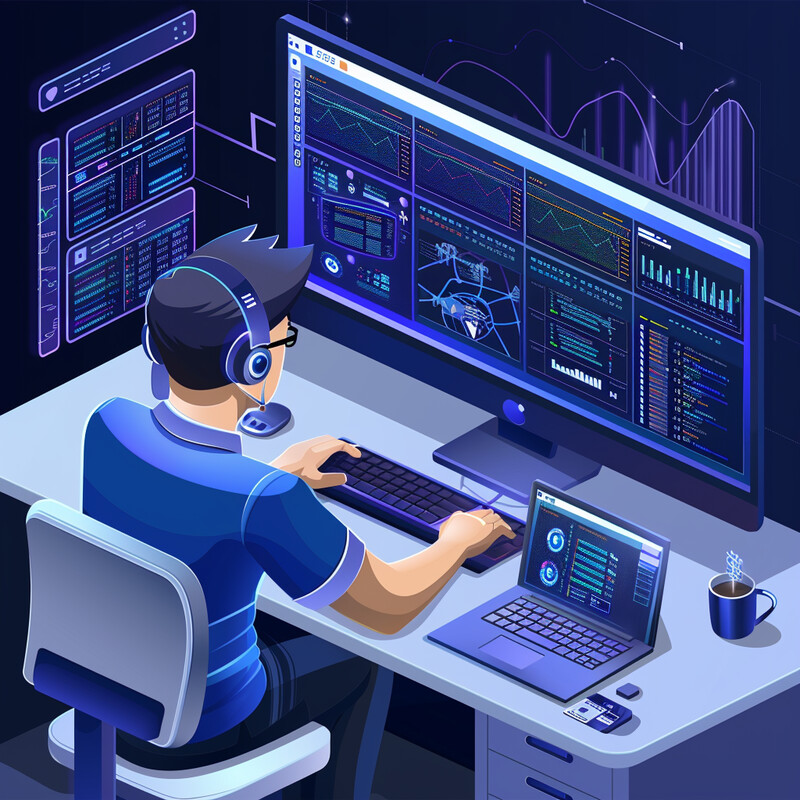
AI enhances the security of online dating platforms by detecting anomalies and patterns indicative of fraudulent behavior. This includes spotting fake profiles, phishing attempts, and bots. AI systems continuously monitor user activities to ensure interactions are legitimate and safe, thus maintaining trust and security within the platform.
4. Personalized User Experiences
AI personalizes the user experience by dynamically adjusting the interface and recommendations based on individual user actions and feedback, enhancing user engagement and satisfaction.
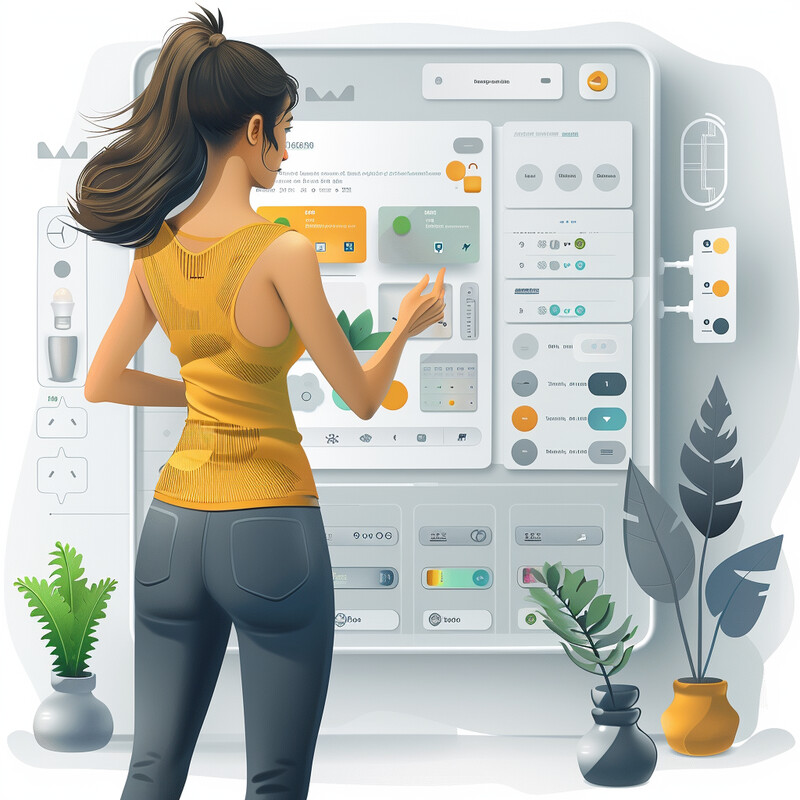
AI personalizes the online dating experience by adapting the user interface and functionalities based on individual user actions and preferences. This can include customizing the layout, suggesting features, and highlighting profiles based on interactive trends, ensuring a more engaging and user-centric experience.
5. Predictive Analytics for User Compatibility
AI uses predictive analytics to forecast the potential success of connections by analyzing communication patterns, mutual interests, and psychological traits, helping to foster more meaningful connections.
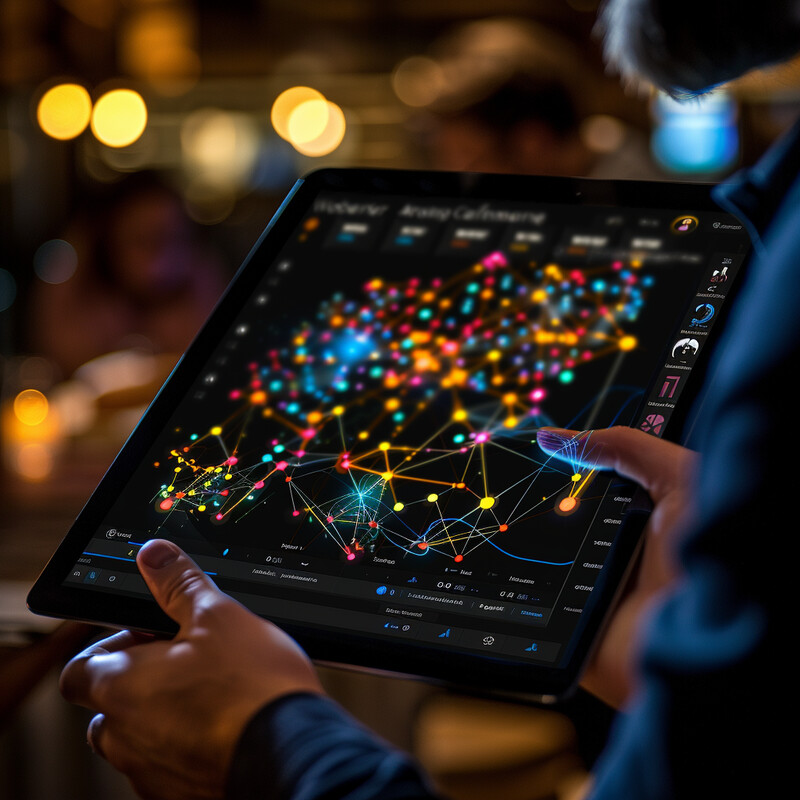
Using predictive analytics, AI assesses the potential compatibility of users based on their interactions, mutual interests, and psychological profiles. This approach allows AI to forecast the potential success of relationships, helping users find partners with whom they are more likely to have lasting and fulfilling relationships.
6. Natural Language Processing for Communication Analysis
AI utilizes natural language processing to analyze chat conversations, suggesting users who are more likely to have meaningful conversations based on communication style and content compatibility.
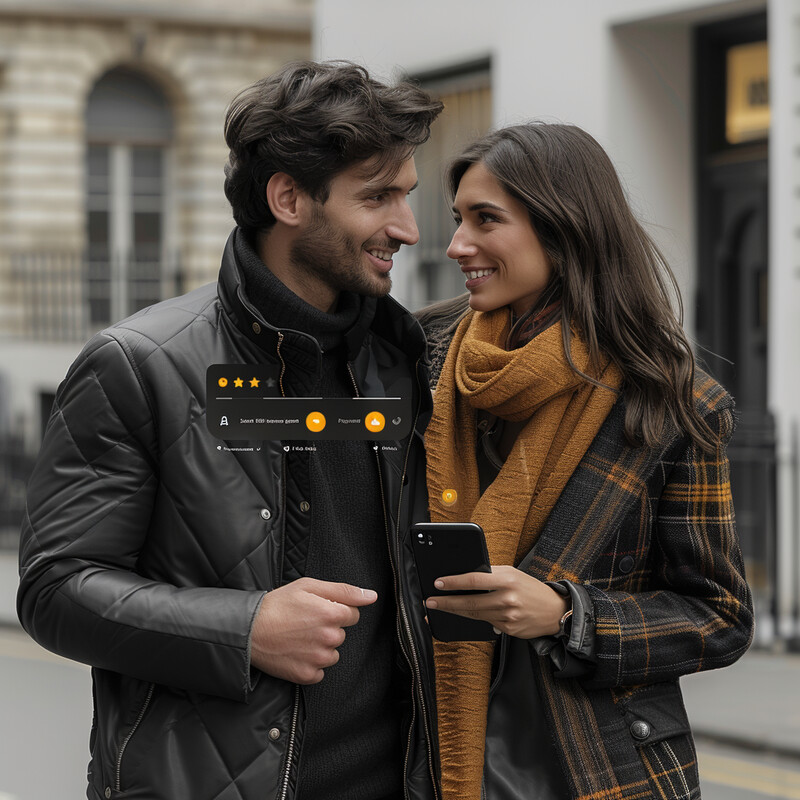
AI employs natural language processing to analyze messaging content between users, identifying compatibility based on communication styles, interests, and conversation depth. This technology helps suggest matches likely to enjoy meaningful conversations, potentially leading to more significant connections.
7. Emotional Analysis
Through sentiment analysis and emotional recognition, AI evaluates emotional tones in user communications to better understand individual emotional states and preferences, tailoring match suggestions accordingly.
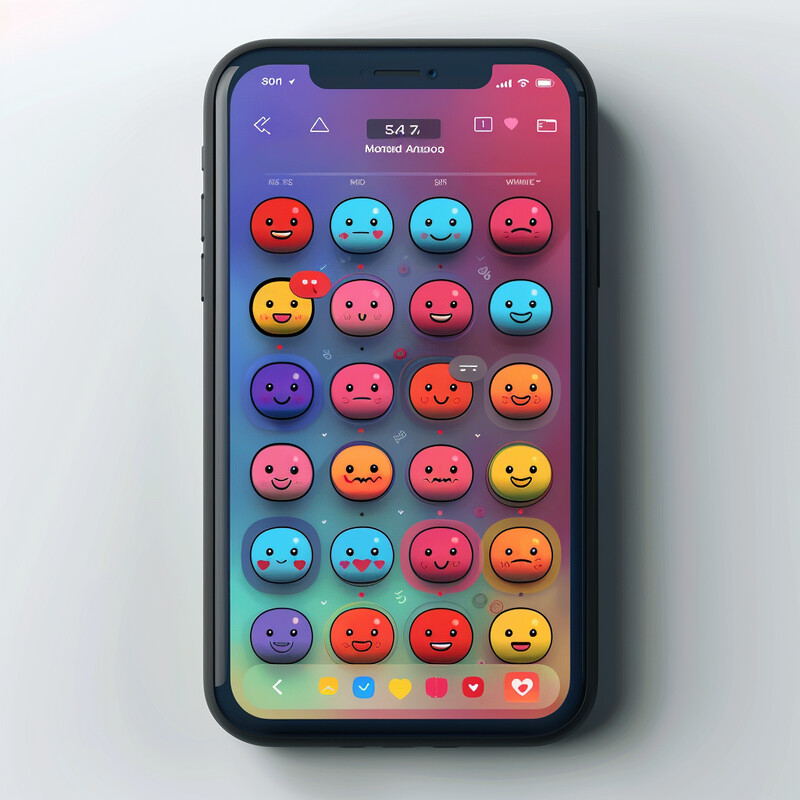
AI analyzes emotional undertones in user communications using sentiment analysis and emotional recognition technologies. By understanding how users express emotions in text or through in-app reactions, AI can better tailor match suggestions that resonate with each user’s emotional preferences and states.
8. Geographic and Real-time Data Usage
AI integrates geographic information and real-time data to suggest matches based on proximity and real-time activities, enhancing the chances of users meeting in person.
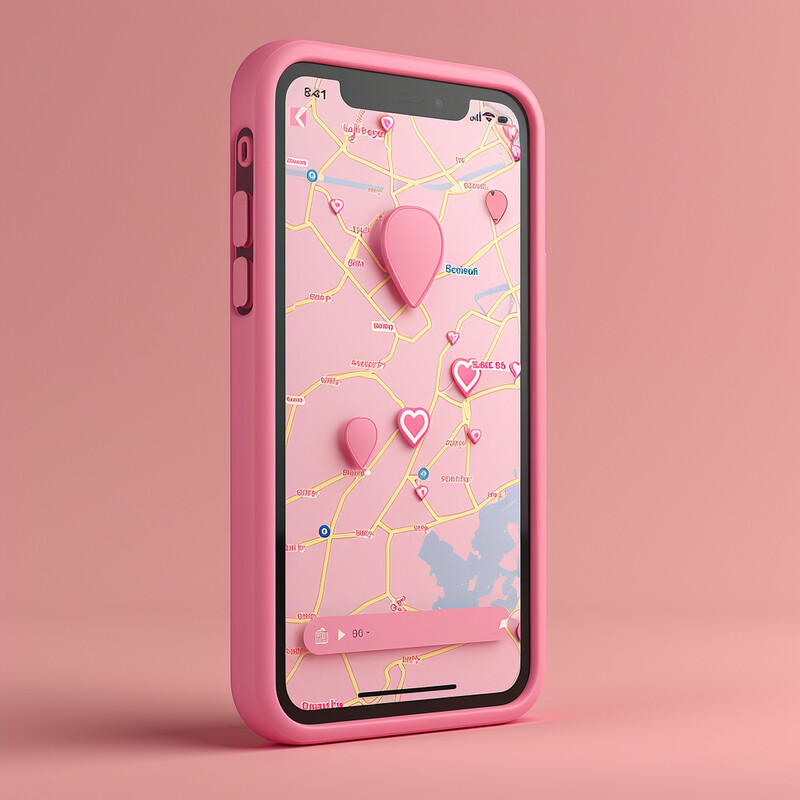
Incorporating geographic information and real-time data, AI enhances the relevance of match suggestions by recommending users who are not only compatible but also physically proximate. This feature is particularly useful for users interested in spontaneous meetups or who value a practical aspect in their relationship dynamics.
9. Feedback Loop Integration
AI incorporates feedback from past interactions and dating outcomes to continuously improve and refine the matching algorithms, ensuring that they adapt to user preferences and experiences over time.
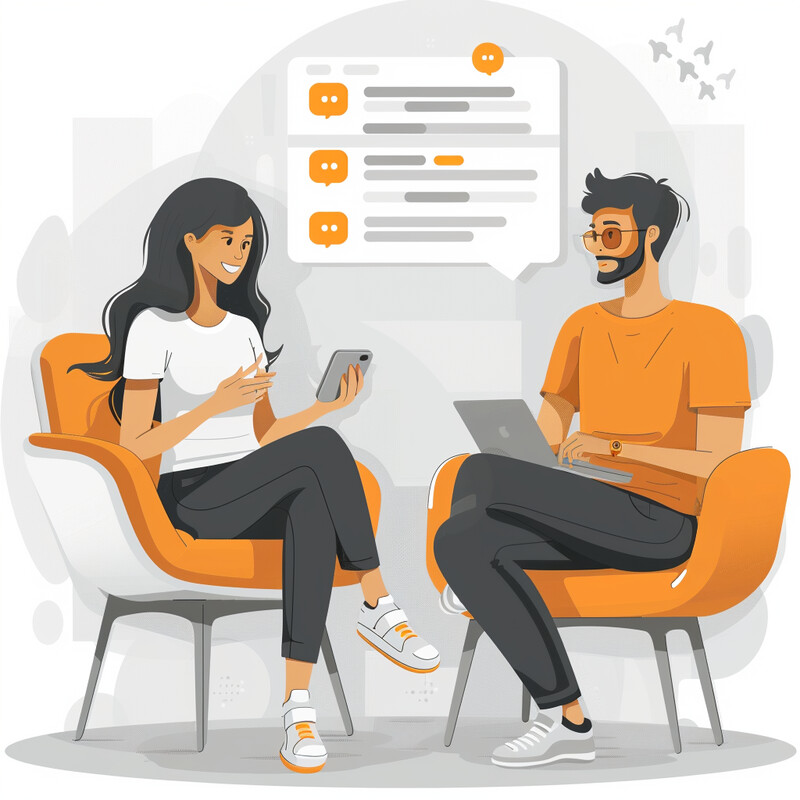
AI integrates feedback loops by collecting data from users' past dating experiences and interactions. This feedback helps continuously refine and adjust the algorithms, ensuring they evolve according to real-world outcomes and user satisfaction, thereby improving the effectiveness of the matchmaking process over time.
10. Automated Moderation and Content Control
AI monitors user-generated content, ensuring compliance with platform guidelines and fostering a respectful and positive community environment, which is crucial for user retention and satisfaction.
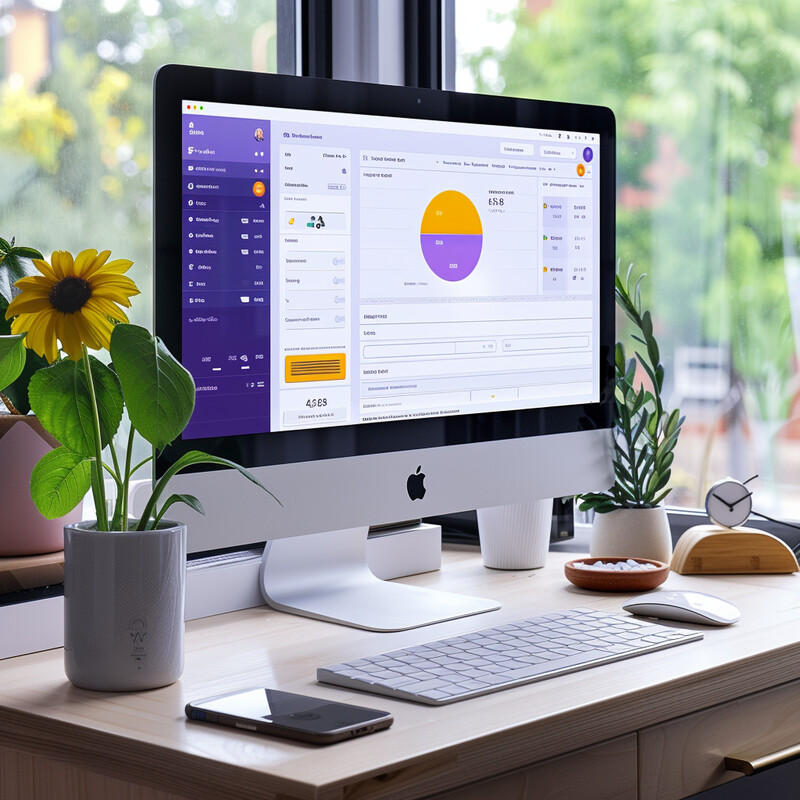
AI monitors and controls user-generated content on dating platforms, ensuring it adheres to community guidelines and respects user boundaries. This automated moderation helps maintain a positive, respectful community environment, crucial for user retention and overall satisfaction with the platform.