1. Audience Targeting
AI analyzes vast amounts of data to identify and target specific demographic groups most likely to respond to certain advertisements, enhancing campaign relevance and effectiveness.
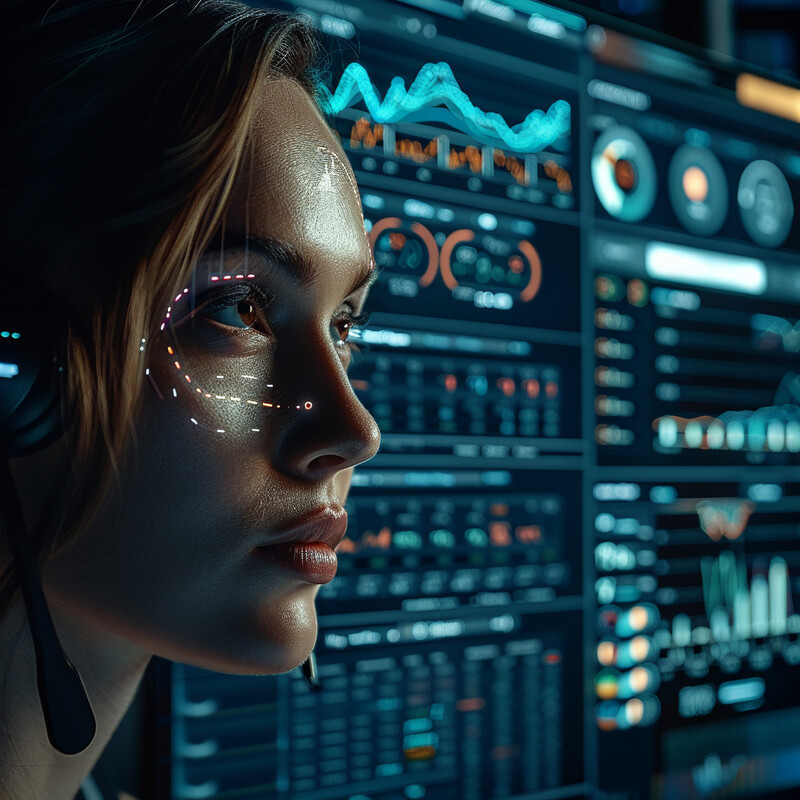
AI leverages complex algorithms to analyze user data such as demographics, interests, and behavior to precisely identify target audiences for specific ad campaigns. By segmenting audiences based on their likelihood to engage or convert, AI enables advertisers to tailor their strategies to reach the most receptive users, thereby increasing the effectiveness and efficiency of advertising efforts.
2. Real-time Bidding (RTB)
AI automates the process of real-time bidding for ad placements, enabling advertisers to purchase the best ad spots at the optimal price, maximizing return on investment.
-3.jpg)
AI plays a crucial role in automating real-time bidding in programmatic advertising. It quickly assesses the value of ad impressions as they become available and makes instantaneous bidding decisions based on the likelihood of user engagement. This automation allows advertisers to secure optimal ad placements at competitive prices, maximizing their return on investment.
3. Ad Personalization
AI tailors ads to individual users based on their browsing history, purchasing behavior, and personal preferences, making the ads more engaging and likely to lead to conversions.
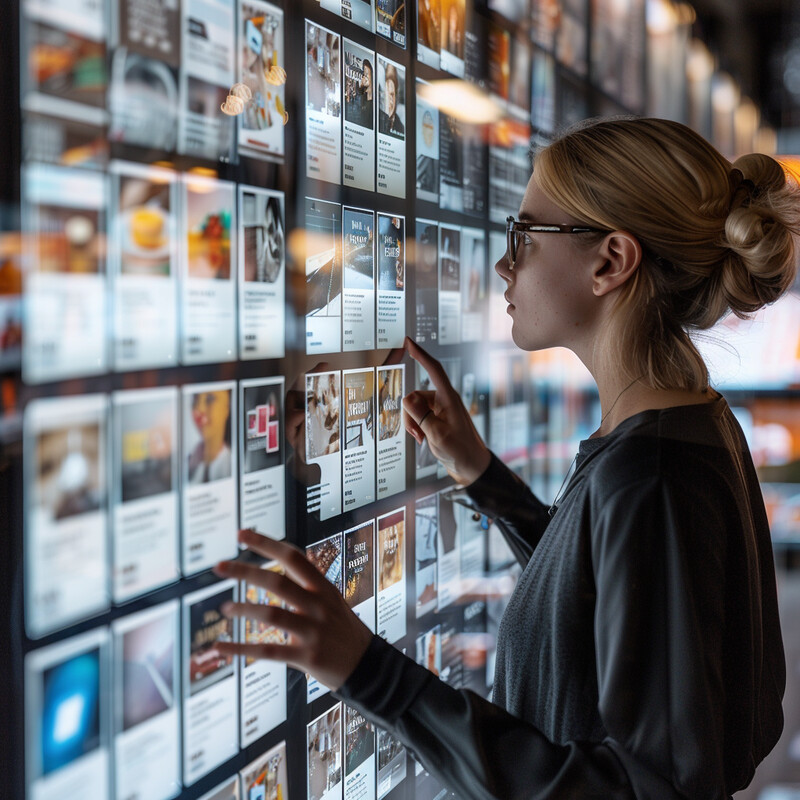
AI enhances ad personalization by analyzing individual user data, including browsing history, purchase behavior, and even the time spent on certain content. This data informs the creation of highly personalized ads tailored to each user's preferences and previous interactions, significantly increasing the chances of engagement and conversion.
4. Predictive Analytics for Ad Performance
AI uses historical data to predict the future performance of ad campaigns, allowing marketers to optimize strategies in advance based on predicted outcomes.
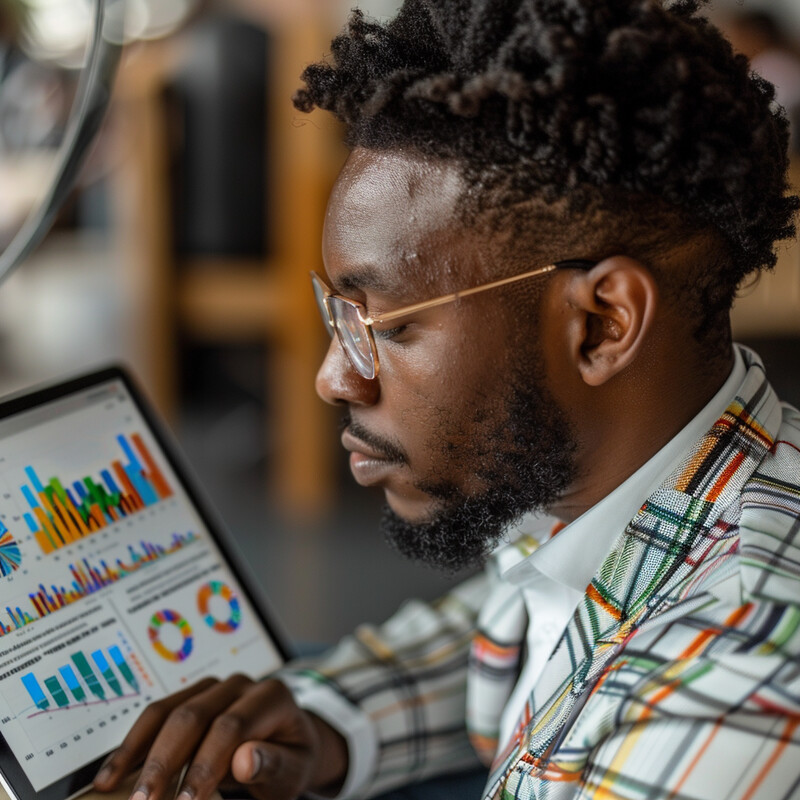
Utilizing predictive analytics, AI forecasts the performance of advertising campaigns by analyzing historical data and identifying trends and patterns. Marketers can use these insights to adjust their strategies proactively, aligning their campaigns with anticipated market movements and consumer responses, thus optimizing future ad performance.
5. Fraud Detection
AI monitors online ad traffic to identify and prevent fraudulent activities, such as fake clicks from bots, ensuring that advertisers' budgets are spent on genuine user engagements.
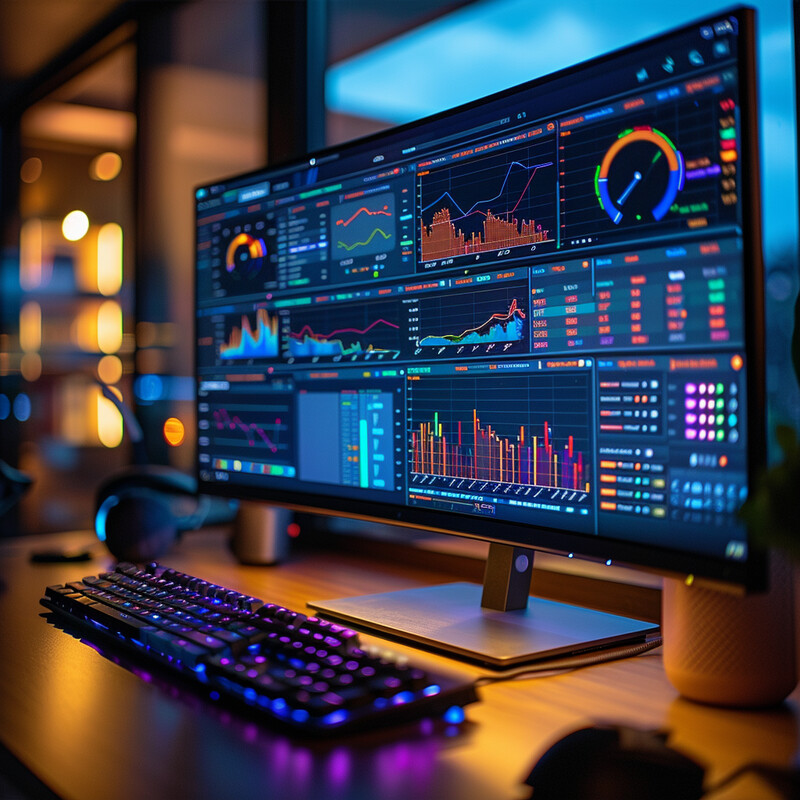
AI improves the integrity of online advertising by detecting fraudulent activities such as click fraud, where bots or unscrupulous entities mimic user interactions. By identifying irregular patterns and behaviors that deviate from human actions, AI helps ensure that advertising budgets are spent on genuine engagements, protecting advertisers from wasted expenditures.
6. Optimal Ad Placement
AI evaluates content relevance and user engagement metrics to determine the best placement for ads on websites and platforms, ensuring higher visibility and interaction rates.
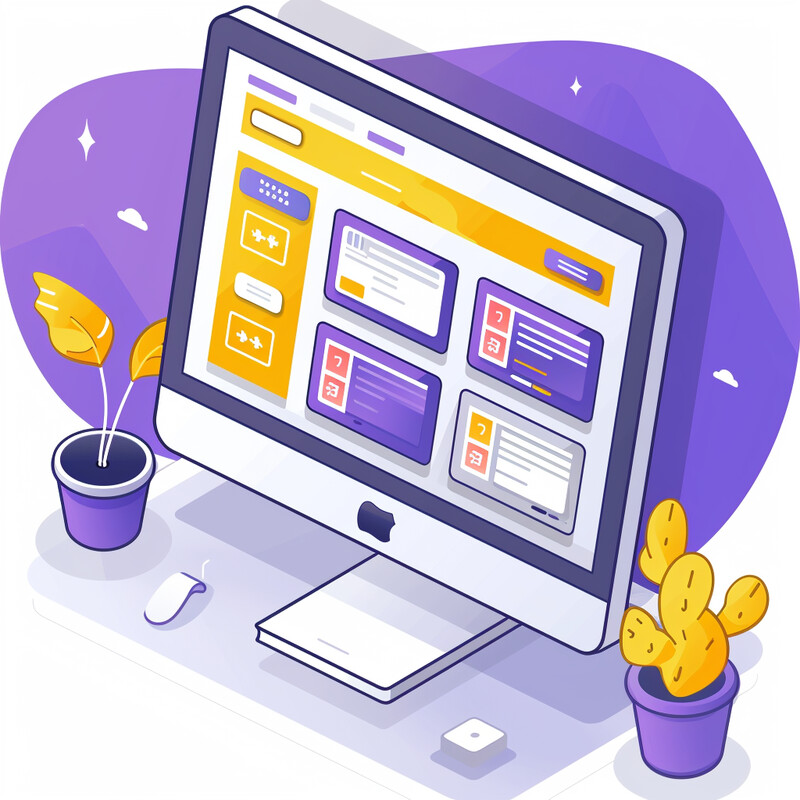
AI evaluates various factors like user engagement, content relevance, and past performance to determine the most effective placements for ads within websites or digital platforms. This strategic placement driven by AI maximizes ad visibility and interaction rates, enhancing the overall impact of advertising campaigns.
7. Dynamic Creative Optimization (DCO)
AI adjusts creative elements of ads in real-time based on user interaction and feedback, optimizing visual elements, messaging, and calls-to-action to increase engagement.
-3.jpg)
Dynamic Creative Optimization uses AI to modify the creative elements of advertisements in real-time based on user interactions and feedback. By adjusting aspects such as imagery, copy, and calls-to-action, AI ensures that ads remain appealing to the viewer, thus improving engagement rates and campaign performance.
8. Cross-channel Marketing Optimization
AI analyzes how users interact with ads across different platforms and devices, enabling marketers to create a unified and consistent marketing strategy that optimizes conversions across all channels.
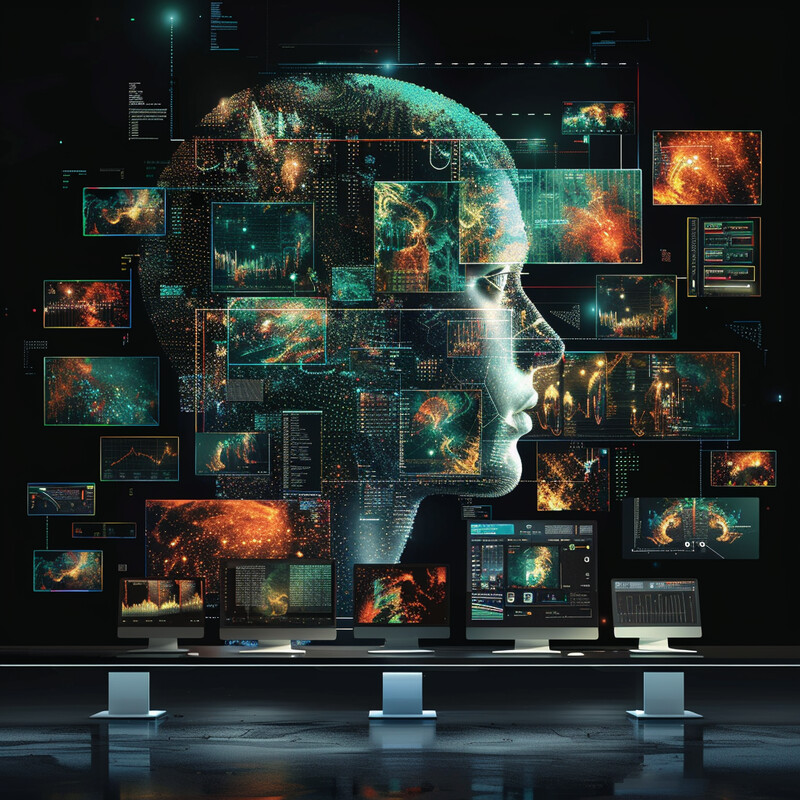
AI analyzes interactions across different marketing channels and devices to provide a cohesive view of user behavior and preferences. This comprehensive insight enables marketers to create integrated strategies that deliver a consistent message and user experience across all platforms, optimizing engagement and conversion rates throughout the customer journey.
9. Customer Lifetime Value Prediction
AI predicts the potential lifetime value of customers acquired through online ads, helping marketers focus their efforts and budget on acquiring high-value customers.
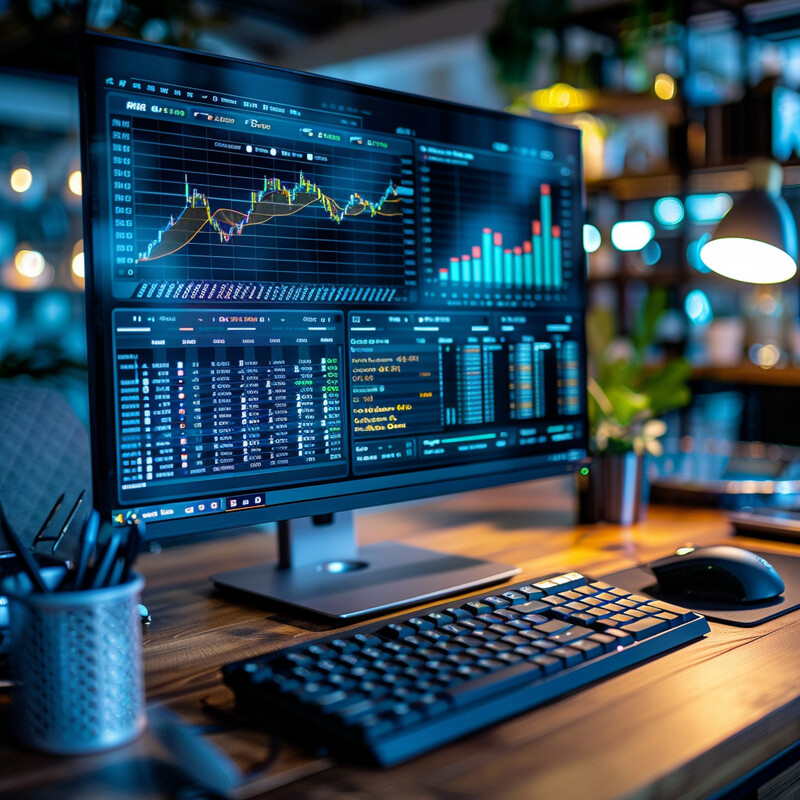
AI models estimate the potential lifetime value of customers acquired through online ads, enabling marketers to focus their resources on segments that are likely to offer the highest return over time. This targeted approach enhances the overall efficiency of marketing budgets by prioritizing high-value customer engagements.
10. Content Optimization
AI assists in creating and optimizing the content of ads based on what resonates best with the target audience, using natural language processing and image recognition to refine messaging and visuals.
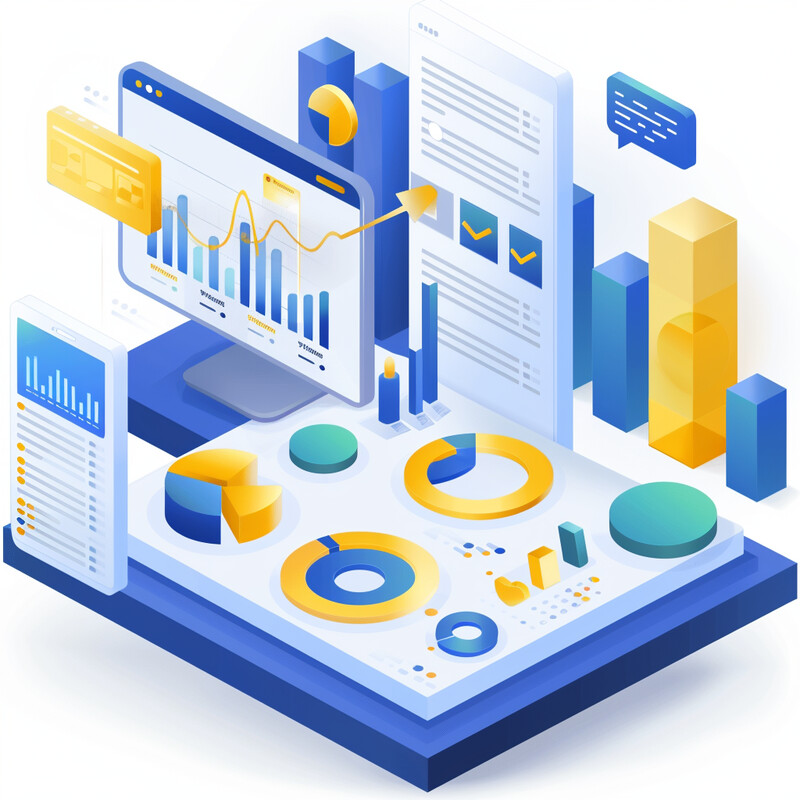
AI aids in optimizing the content of ads by using natural language processing to analyze text and image recognition to evaluate visuals. It identifies which elements perform best with specific audiences and adjusts the content accordingly to maximize resonance and effectiveness.