1. Autonomous Underwater Vehicles (AUVs)
AI enables AUVs to operate independently in complex underwater environments, dramatically extending the reach of ocean exploration. With AI-based navigation, an AUV can make real-time decisions to avoid obstacles and adapt to changing ocean conditions without human input. These vehicles collect data on oceanographic conditions, marine life, and seabed features continuously, even in hazardous or deep zones where human divers or tethered robots cannot easily go. By reducing the need for direct human control, AI-powered AUVs increase the efficiency of surveys and mapping missions, covering large areas over long durations. This not only frees up ship time and human resources but also improves safety, as AUVs can explore in dangerous or extreme environments (like under ice or near hydrothermal vents) without risking lives. Overall, AI has transformed AUVs into intelligent explorers that greatly enhance the scale and depth of ocean research.
-2.jpg)
In 2024, a commercial trial deployed the world’s first AI-driven autonomous underwater vehicle to inspect offshore wind farm structures without human control. The results were striking: the AI-guided AUV reduced inspection times by up to 50% compared to conventional methods. By cutting the inspection timeline in half, this approach also significantly lowered operational costs and minimized the exposure of human divers to risky conditions. Additionally, the AI system improved the quality of inspection data, enabling detailed 3D reconstructions of underwater assets during the same missions. This real-world example underscores how AI enhancements to AUVs can boost efficiency and safety in ocean operations.
2. Data Analysis and Interpretation
Modern oceanography generates massive datasets—from satellites, autonomous sensors, research vessels, and profiling floats—that are far too vast for manual analysis. AI algorithms excel at processing these large and complex data streams, detecting patterns and anomalies in ocean currents, temperature, salinity, and other variables much faster than humans. By finding meaningful signals in the noise, AI systems help scientists interpret phenomena like El Niño events, marine heatwaves, or shifting marine biodiversity trends in near real time. Machine learning models can also fuse diverse data sources (e.g. combining satellite imagery with in-situ sensor readings) to provide a more comprehensive picture of ocean conditions. This rapid, integrative analysis enables informed decision-making in areas such as fisheries management, climate change adaptation, and marine conservation, as researchers can respond to environmental changes with much shorter lag times.
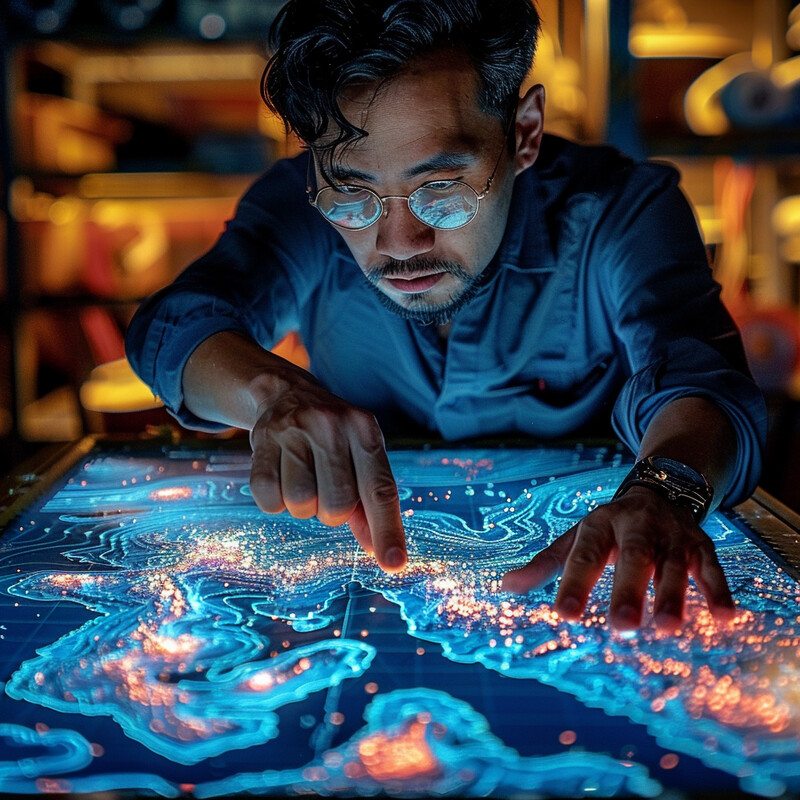
In modern oceanography, thousands of instruments and sensors collectively produce an unprecedented volume of data that only AI can manage efficiently. The Monterey Bay Aquarium Research Institute (MBARI), for example, reported downloading 55.6 petabytes of underwater soundscape data in 2023 alone. (For perspective, 55.6 petabytes equals over 55 million gigabytes of data.) Processing and analyzing such a massive dataset within a reasonable time frame is feasible only with advanced AI and machine learning algorithms. These AI tools automatically sift through the data to identify relevant signals—such as distinguishing biological sounds from background noise in the acoustic recordings—far faster than a human analyst could. As a result, researchers can glean insights (like detecting the presence of certain marine species by their sounds or spotting long-term trends in ocean noise) that would likely be missed or delayed without AI-driven data interpretation.
3. Habitat Mapping
AI greatly enhances the mapping of marine habitats by processing imagery and sensor inputs to create detailed maps of the seafloor and vital ecosystems. High-resolution satellite and drone images, along with sonar data from ships and AUVs, can be fed into AI models that classify different underwater features (coral reefs, seagrass beds, mangroves, etc.) much faster and more consistently than manual charting. This provides scientists and conservationists with precise, up-to-date spatial information on ecosystem distribution and health. AI-driven mapping also allows for frequent updates, so changes in habitats (like coral bleaching events or kelp forest decline) can be monitored over time. By automating habitat mapping, AI not only accelerates the pace of research but also helps identify previously unknown features (such as undiscovered reefs or deep-sea vent communities) that might have been overlooked, thereby improving our understanding and management of marine ecosystems.
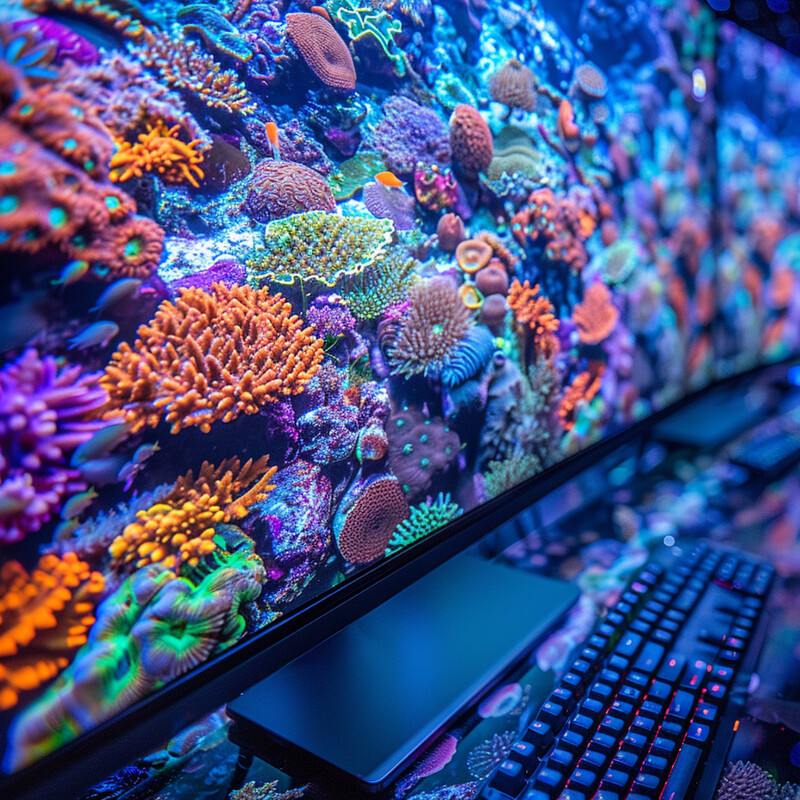
In 2023, the Allen Coral Atlas – which employs AI to analyze high-resolution satellite imagery – completed a mapping of approximately 423,589 square kilometers of the world’s shallow coral reefs. This area is comparable to the size of a large country (about the land area of Papua New Guinea), illustrating the vast scope of habitat information synthesized by the project. The AI system classified reef habitats from satellite data in unprecedented detail, significantly improving upon earlier global reef maps. In fact, the Atlas revealed more coral reef area than previously recorded by conventional mapping in the 1990s, filling knowledge gaps in reef distribution. These AI-generated maps serve as a baseline for monitoring changes in reef health; for example, scientists can detect where coral cover declines or recovers by comparing new satellite images against the Atlas maps. The ability to continuously update and refine habitat maps with AI is proving invaluable for guiding marine protected area planning and tracking the impacts of climate change on critical ecosystems.
4. Species Identification and Tracking
AI is transforming how researchers identify and monitor marine species, enabling analyses at scales and speeds previously unattainable. Machine learning models can be trained on underwater photographs, videos, or audio recordings to recognize different species automatically—whether it’s identifying fish and coral in imagery or detecting whale and dolphin calls in acoustic data. This allows for large-scale biodiversity surveys where AI rapidly processes thousands of hours of footage or sound, flagging the presence of species of interest far more efficiently than a human reviewer. AI-powered identification is also often more consistent and can sometimes discern subtle patterns (such as markings on a fish) that humans might miss. By automating species counts and tracking movements (through tagging data or pattern recognition), AI aids conservation efforts with timely data on population trends, migration routes, and the behaviors of endangered species. Overall, these tools expand our capacity to monitor ocean life, providing a richer and more continuous picture of marine biodiversity.
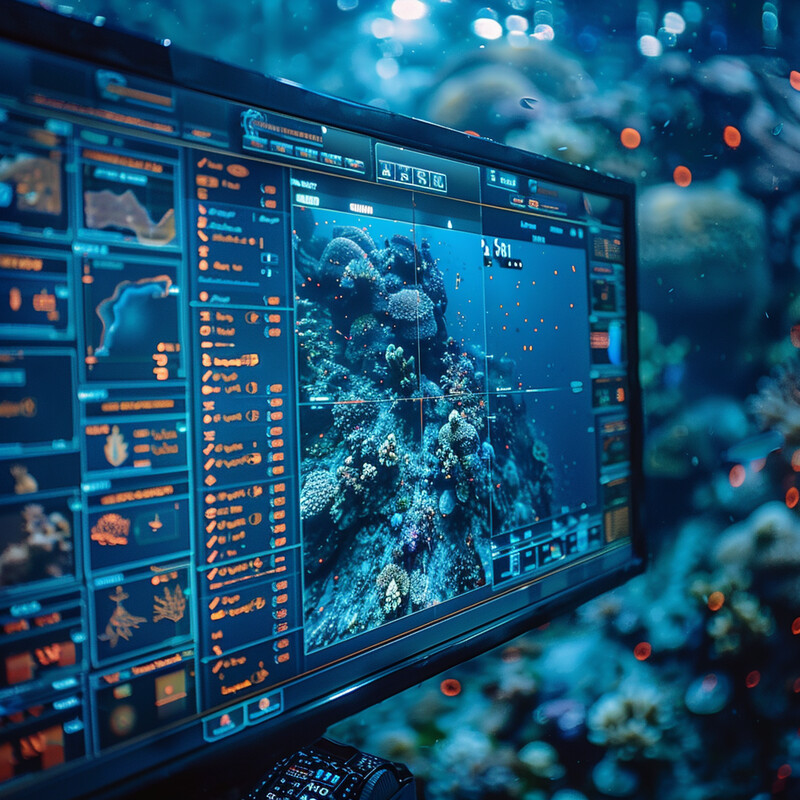
In 2025, ocean scientists demonstrated an AI system that identifies fish presence on coral reefs by analyzing sound recordings – matching human expert accuracy but accomplishing the task more than 25 times faster. The neural network was trained to recognize fish calls amid the complex ambient sounds of a reef and could analyze acoustic data in real time. This leap in speed means researchers can monitor reef ecosystems almost continuously and respond quickly to changes in fish populations. Importantly, the AI model maintained accuracy comparable to skilled biologists in deciphering these acoustical trends. Such AI-driven bioacoustics, combined with computer vision for analyzing underwater video footage, is revolutionizing wildlife surveys. Researchers are now able to conduct large-scale, automated tracking of marine species (from tiny plankton to large mammals) with high precision, significantly accelerating biodiversity assessments and improving conservation planning.
5. Pollution Monitoring
AI plays a critical role in detecting and monitoring pollution in the oceans quickly and accurately. Advanced image recognition algorithms can scan satellite images or aerial drone footage to spot signs of pollution—such as oil spills, algal blooms, or rafts of floating plastic—much faster than human observers. Similarly, AI-enhanced sensors can be deployed in the water to identify chemical pollutants or microplastics, distinguishing them from natural substances in real time. By automating these detection processes, AI provides early warnings of environmental hazards, enabling quicker response efforts to contain damage (for example, deploying cleanup crews to an oil spill before it spreads). In addition, AI systems continuously monitor pollution indicators over vast areas, compiling data that help scientists understand pollution sources, movement (like tracking plastic drift patterns), and the effectiveness of mitigation measures. This leads to more informed policies and targeted actions to protect marine ecosystems from pollution.
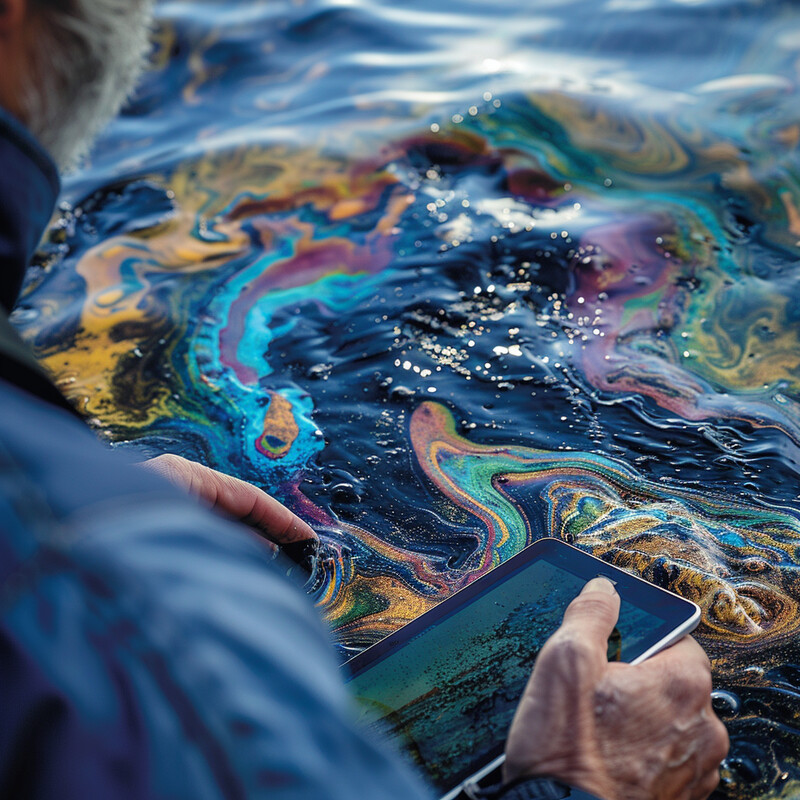
A recent study demonstrates the effectiveness of AI in identifying marine pollutants. In 2024, scientists developed a low-cost sensing system called SEAGULL that uses AI to determine the type of underwater plastic debris with over 85% accuracy. The system analyzes how different plastics reflect light underwater and classifies the debris by its material (its resin identification code) on the spot. This means a diver or robotic vehicle equipped with SEAGULL can immediately distinguish, for instance, polyethylene from polypropylene debris without needing to send a sample to a lab. In field tests, the AI correctly differentiated the main plastic categories despite varying water conditions, proving its robustness in real-world settings. Beyond plastics, similar AI-driven approaches are being used to automatically detect oil slicks in satellite imagery and to identify areas of high nutrient runoff (which can trigger harmful algal blooms). By providing rapid and accurate pollution detection, AI tools allow for quicker clean-up responses and better-informed strategies to prevent and mitigate ocean pollution.
6. Climate Change Research
AI has become an indispensable tool in modeling and predicting the effects of climate change on the oceans. Climate and ocean systems are extremely complex, producing huge datasets that AI can help analyze to find patterns or make forecasts that traditional methods struggle with. For example, machine learning models are used to predict ocean warming events, such as El Niño and marine heatwaves, with longer lead times or greater accuracy by learning from vast historical data. AI can also downscale global climate model outputs to local scales, providing more detailed projections of sea-level rise or coastal flooding risks for specific regions. Additionally, by assimilating diverse data sources (ocean floats, satellite altimetry, historical ship logs, etc.), AI improves our understanding of how climate change alters ocean currents, marine ecosystems, and biogeochemical cycles. These enhanced predictive insights are crucial for developing adaptation strategies—informing fisheries management, coral reef protection, and coastal infrastructure planning in the face of changing ocean conditions.
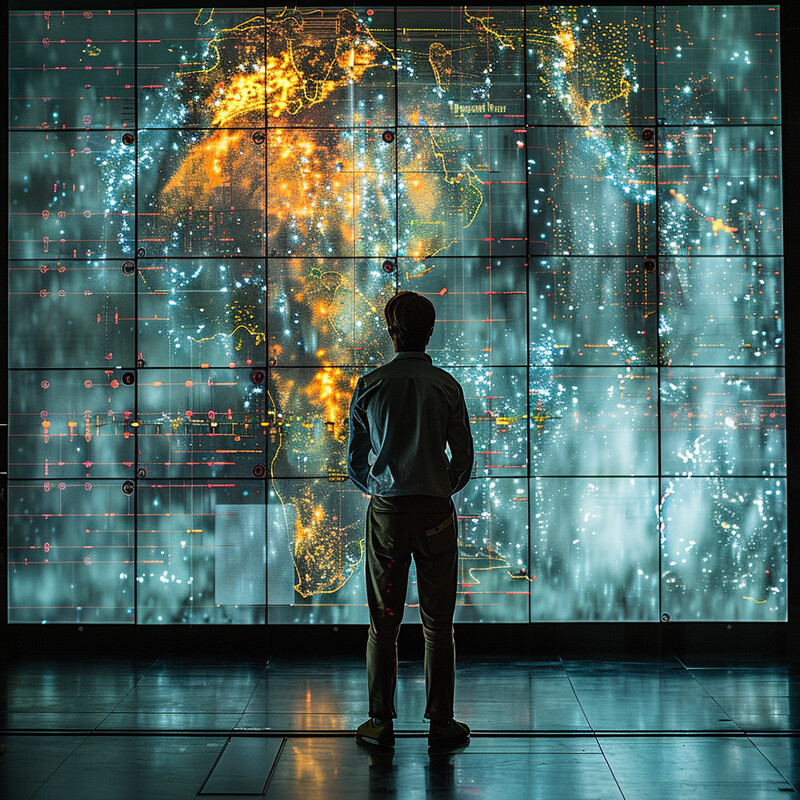
AI-driven models are extending the horizon of climate predictions related to the oceans. For example, a 2023 study applied artificial intelligence to forecast the El Niño–Southern Oscillation (ENSO) and achieved an effective prediction lead time of 22 months. Traditional physics-based models typically struggle to predict El Niño events more than about 12 months in advance, so this AI result nearly doubled the warning time for such climate oscillations. Improved long-range ENSO forecasts give coastal communities and industries (like agriculture and fisheries) much more time to prepare for the global weather shifts that strong El Niño or La Niña events can cause. Beyond ENSO, researchers are employing AI in climate models to better project regional sea-level changes and the frequency of extreme ocean warming events, areas where pattern recognition in vast data sets can yield insights beyond what manual analysis provides. By enhancing both the accuracy and the lead time of ocean climate forecasts, AI is helping scientists and policymakers anticipate and plan for climate change impacts on marine environments.
7. Deep-sea Mining Exploration
AI is aiding the nascent field of deep-sea mining by making the exploration for underwater mineral deposits more efficient and less environmentally intrusive. The deep ocean floor contains valuable mineral resources (like polymetallic nodules, cobalt crusts, and sulfide deposits), but finding the richest deposits among vast seafloor areas is challenging. AI helps by analyzing geological maps, sonar scans, and geochemical data to predict where high concentrations of target minerals are likely to occur, guiding exploration vessels to those spots rather than relying on trial-and-error. This targeted approach reduces the area that needs to be disturbed during exploratory surveys. Additionally, AI can optimize the operation of exploration vehicles on the seafloor, helping them navigate complex terrain (such as seamounts or trench valleys) and even recognizing signs of sensitive habitats to avoid. By improving discovery while mitigating impact, AI contributes to a more sustainable pathway as companies and researchers evaluate whether and how to extract deep-sea resources needed for technologies like batteries and renewable energy.
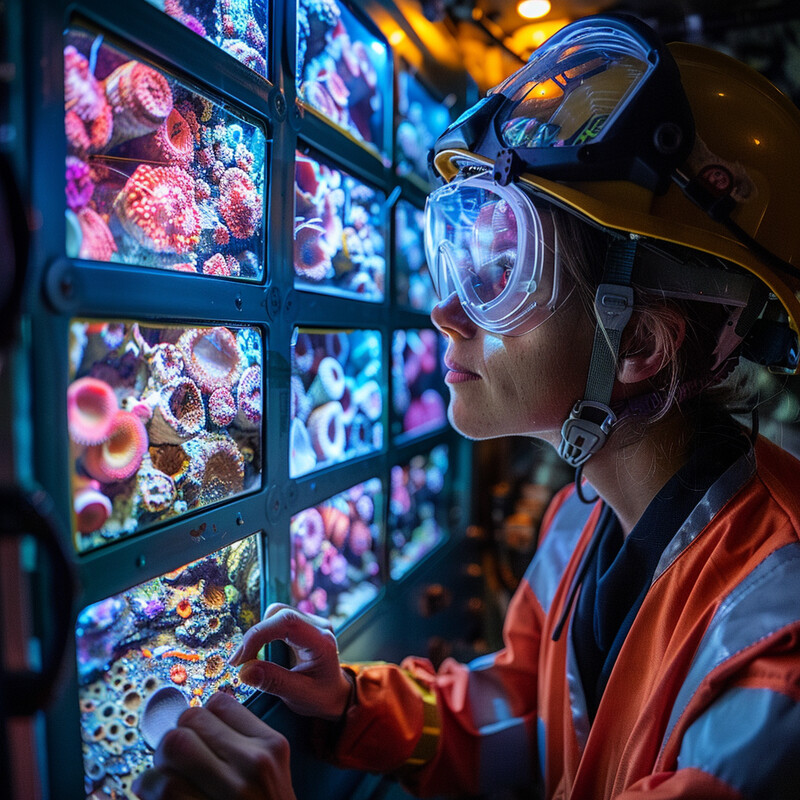
The potential mineral wealth on the deep-ocean floor is enormous, and AI helps pinpoint these riches. In the Pacific’s Clarion–Clipperton Zone (CCZ), for instance, an estimated 21.1 billion tons of polymetallic nodules lie on the seafloor. These potato-sized nodules are rich in metals such as nickel, cobalt, and copper, making the CCZ a prime area of interest for future deep-sea mining. AI-based models can digest geological survey data from the CCZ’s ~9 million square kilometers and predict where the densest nodule concentrations are, guiding submersibles and sampling equipment directly to those high-yield sites. Such focused exploration maximizes mineral finds while minimizing unnecessary disturbance to the seabed. This is critical for environmental reasons: the deep sea is home to unique ecosystems, and reducing the footprint of exploration (thanks to AI’s more precise targeting) helps protect fragile habitats from broad disruption. In short, by leveraging AI’s predictive power, explorers can more efficiently locate undersea mineral deposits that rival or exceed known on-land reserves, all while exercising greater care for the marine environment.
8. Predictive Maintenance for Exploration Equipment
Ocean exploration missions depend on complex equipment—research vessels, deep-sea submersibles, autonomous vehicles, sensors arrays—operating reliably in harsh marine conditions. AI-driven predictive maintenance ensures these critical systems remain operational by analyzing data from equipment (vibrations, temperatures, pressures, etc.) to predict when a component might fail. Instead of adhering strictly to fixed maintenance schedules or reacting after a breakdown, AI systems forecast maintenance needs so that parts can be serviced or replaced just before performance degrades. This approach minimizes unexpected equipment failures during expeditions, which is vital when a malfunction in a remote deep-sea environment could jeopardize a mission or researcher safety. Predictive maintenance also optimizes logistics: it reduces unnecessary routine servicing (saving time and cost) and ensures spare parts and technicians are available when truly needed. In essence, AI helps maintain ocean exploration gear in peak condition, improving the overall efficiency, safety, and cost-effectiveness of deep-sea operations.
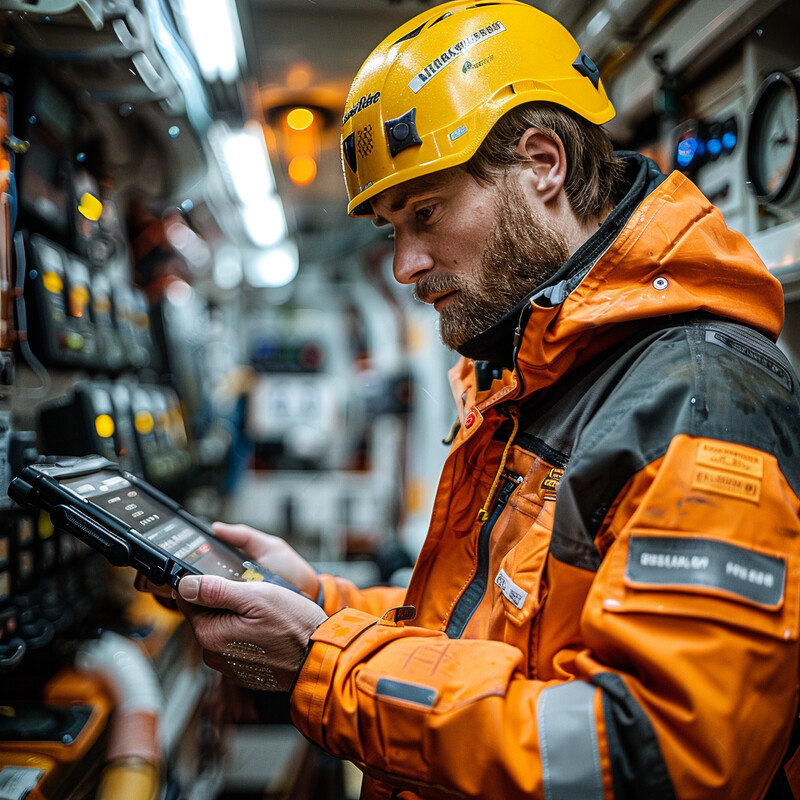
Industry data show that AI-driven predictive maintenance can significantly improve uptime and reduce costs. The U.S. Department of Energy reports that adopting predictive maintenance leads to a 25–30% reduction in maintenance costs and about a 70–75% decrease in equipment breakdowns on average. Organizations also experience roughly a 35–45% reduction in downtime because repairs can be scheduled proactively rather than reactively. In the context of ocean exploration, this means research ships, submersibles, and remote instruments are far less likely to fail unexpectedly in the middle of a critical mission. For instance, if AI analytics detect that a ship’s engine or an ROV’s thruster is trending toward a fault, maintenance can be performed at the next port call or dive interval before a catastrophic failure occurs. This not only avoids costly mission aborts and repairs but also improves safety by preventing dangerous malfunctions. Over time, the savings from fewer emergency fixes and longer equipment lifespans are substantial, which is why predictive maintenance programs have been shown to offer returns on investment on the order of ten-to-one for many organizations.
9. Communication Enhancement
Underwater communication is inherently difficult due to the physics of water, but AI is helping to improve both the reliability and capacity of data transmissions in the ocean. Because radio waves attenuate quickly in saltwater, undersea vehicles and instruments often rely on acoustic (sound) signals to communicate, which have very limited bandwidth and are susceptible to echoes and noise. AI algorithms can mitigate these issues by intelligently filtering out ambient noise and correcting signal distortions in real time, which makes acoustic communication channels clearer and more stable. Moreover, AI can dynamically optimize communication protocols—adjusting frequencies, modulation, or routing of data packets between underwater nodes—based on changing conditions (like water depth, temperature, or interference). This adaptive approach significantly enhances the amount of information that can be sent, for example allowing higher-resolution sensor data or video to be transmitted from the seafloor to a surface ship. By improving underwater connectivity, AI enables more coordinated operations among AUVs, ROVs, and ships and ensures that scientists receive critical data from the deep ocean with minimal delay or loss.
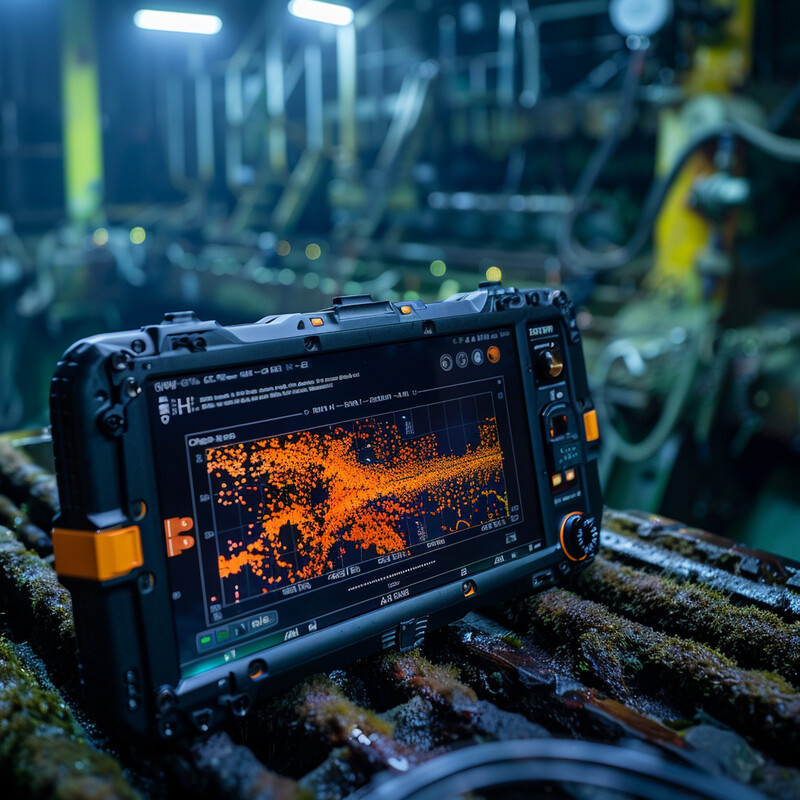
Conventional underwater acoustic communication systems are quite limited: they typically offer bandwidth on the order of only a few kilohertz, which restricts data transfer rates to mere kilobits per second over long distances. (By contrast, a standard Wi-Fi network has thousands of times more bandwidth.) Given this constraint, researchers are leveraging AI-based signal processing to make the most of the narrow underwater communication channel. Machine learning algorithms can dynamically adjust how data are encoded on acoustic waves in response to environmental conditions, effectively squeezing higher efficiency out of the channel and reducing errors. In experimental trials, such AI-driven approaches have managed to improve throughput and extend the range of underwater networks by selecting the optimal frequencies and encoding schemes on the fly. AI is also used in underwater network routing—deciding the best path for data to hop between instruments—resulting in more reliable multi-node communication even in challenging environments. While undersea data rates remain much lower than terrestrial ones, these AI enhancements are steadily improving the flow of information from the ocean depths, enabling timelier and richer data retrieval during exploration missions.
10. Enhanced Safety Protocols
AI contributes to safer ocean exploration by helping to predict and prevent dangerous situations for human crews and mission-critical equipment. Through advanced simulations, AI can model a wide range of “what-if” scenarios (from sudden equipment failures in a submersible to unexpected storms at sea) and identify potential hazards before missions even commence. This allows teams to refine their safety protocols and training; for example, submersible pilots can practice emergency ascent procedures in AI-generated virtual drills. During expeditions, AI systems continuously monitor sensor data for anomalies or warning signs—such as unusual pressure readings on a sub’s hull or deteriorating air quality in a habitat—and can alert operators to take corrective action or even automatically initiate safety measures. By augmenting human decision-making with rapid risk analysis and reminders, AI reduces the likelihood of oversight or error in high-pressure situations. Overall, embedding AI in safety planning and operations creates an additional layer of protection, ensuring that researchers and explorers are better prepared for the inherent risks of deep-sea ventures.
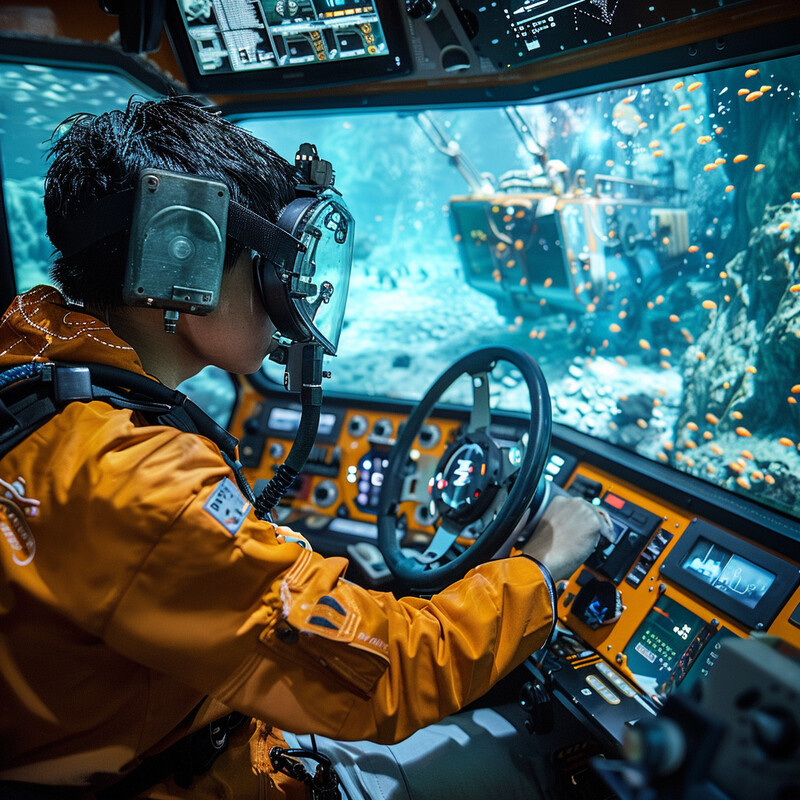
Human factors remain a leading cause of accidents at sea, which is why AI’s predictive safety capabilities are so valuable. Studies indicate that human error is a contributing factor in over 70–80% of maritime accidents. AI-driven safety systems aim to counter this by supporting crews both in training and in real-time operations. For instance, AI-based simulators can expose submarine pilots or ship crews to a wide range of simulated emergency scenarios (loss of power, rapid flooding, equipment malfunctions, etc.) and teach optimal responses, reinforcing emergency protocols in a risk-free setting. During actual missions, AI monitoring tools will continuously check the status of critical systems and the environment; if a parameter deviates from the safe range or a pattern emerges that matches a known hazard (e.g. early signs of equipment fatigue or an approaching weather anomaly), the AI will promptly alert the team or suggest preventative actions. By reducing reliance on human vigilance alone and providing data-driven decision support, these AI enhancements significantly lower the chances that an oversight or slow reaction could lead to an accident. The result is a higher safety margin for explorers, as AI helps catch potential problems that humans might miss and ensures that contingency plans are in place well in advance.