1. Enhanced Machine Translation
AI-driven models are greatly improving the quality and speed of machine translation between languages, enabling more accurate and contextually appropriate translations that consider cultural nuances and idiomatic expressions.
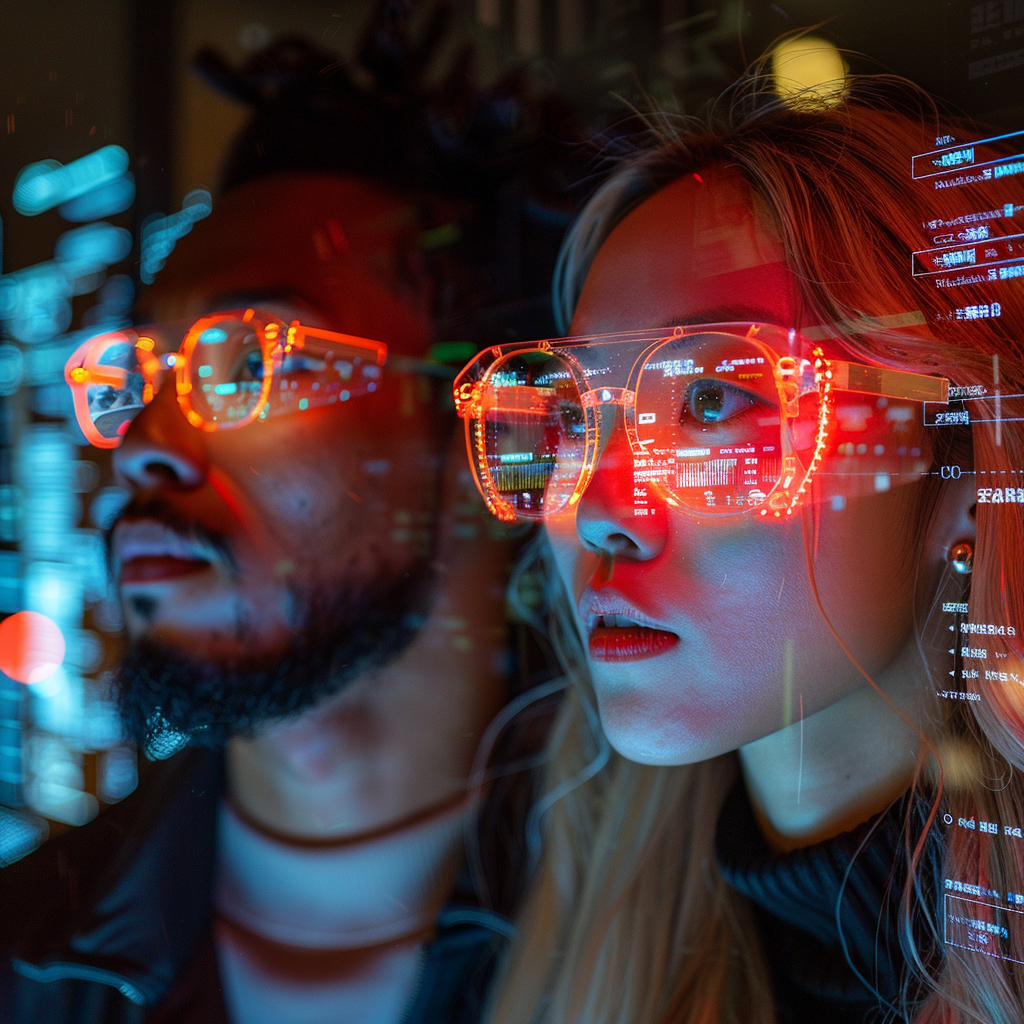
AI has revolutionized machine translation by employing sophisticated models like neural networks, which mimic human brain functions to understand and translate languages with impressive accuracy. These AI systems consider the context, cultural nuances, and even idiomatic expressions, greatly improving the fluency and appropriateness of translated text. This advancement is particularly beneficial in global communication, enabling clearer and more effective cross-cultural exchanges.
2. Better Speech Recognition
AI technologies are enhancing speech recognition systems, making them more accurate in understanding and transcribing spoken language across a variety of accents and dialects, even in noisy environments.
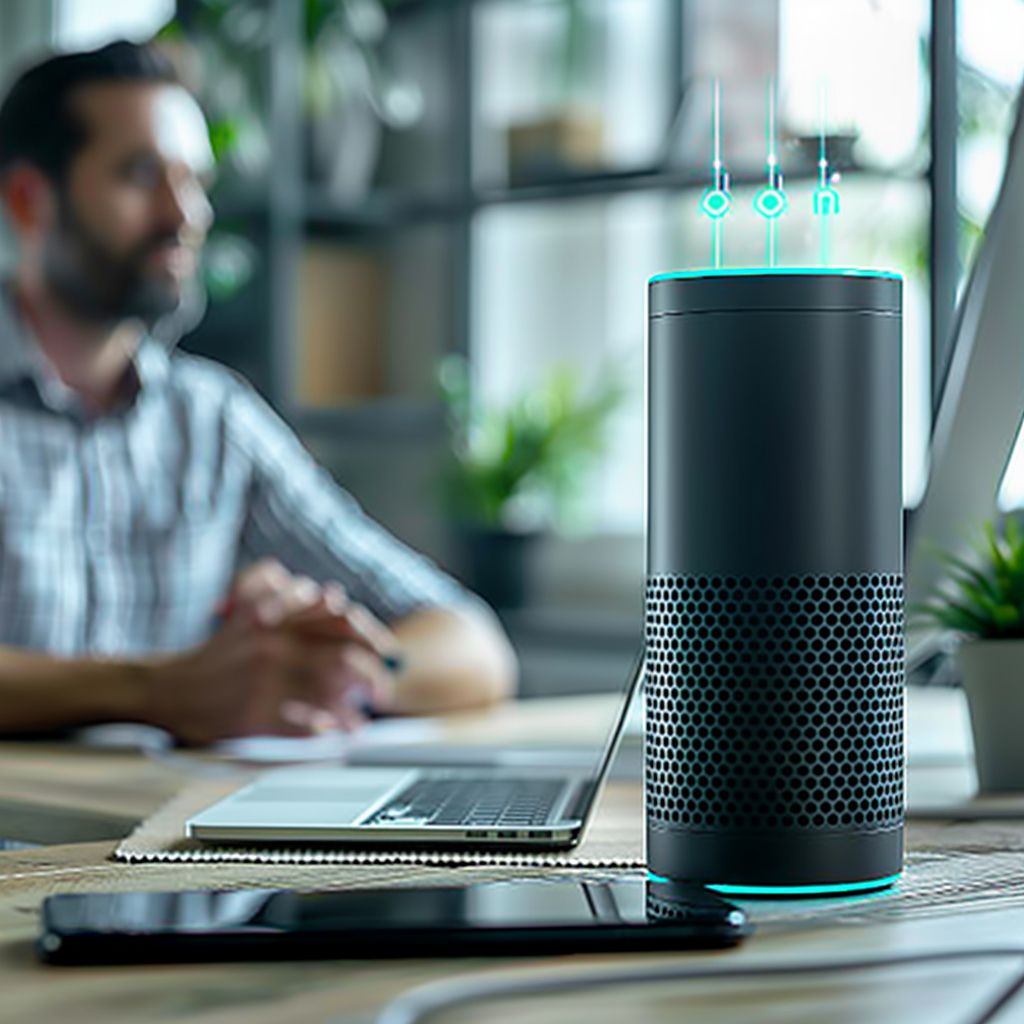
AI-driven speech recognition technologies have significantly improved in recognizing and transcribing spoken language accurately. By using deep learning algorithms, these systems can adapt to various accents, dialects, and speech patterns, and filter out background noises, enhancing their utility in real-world applications such as virtual assistants, dictation software, and interactive voice response systems.
3. Sentiment Analysis
AI helps in analyzing text data to determine the sentiment expressed in it, whether positive, negative, or neutral. This is particularly useful for businesses to understand customer opinions and market trends.
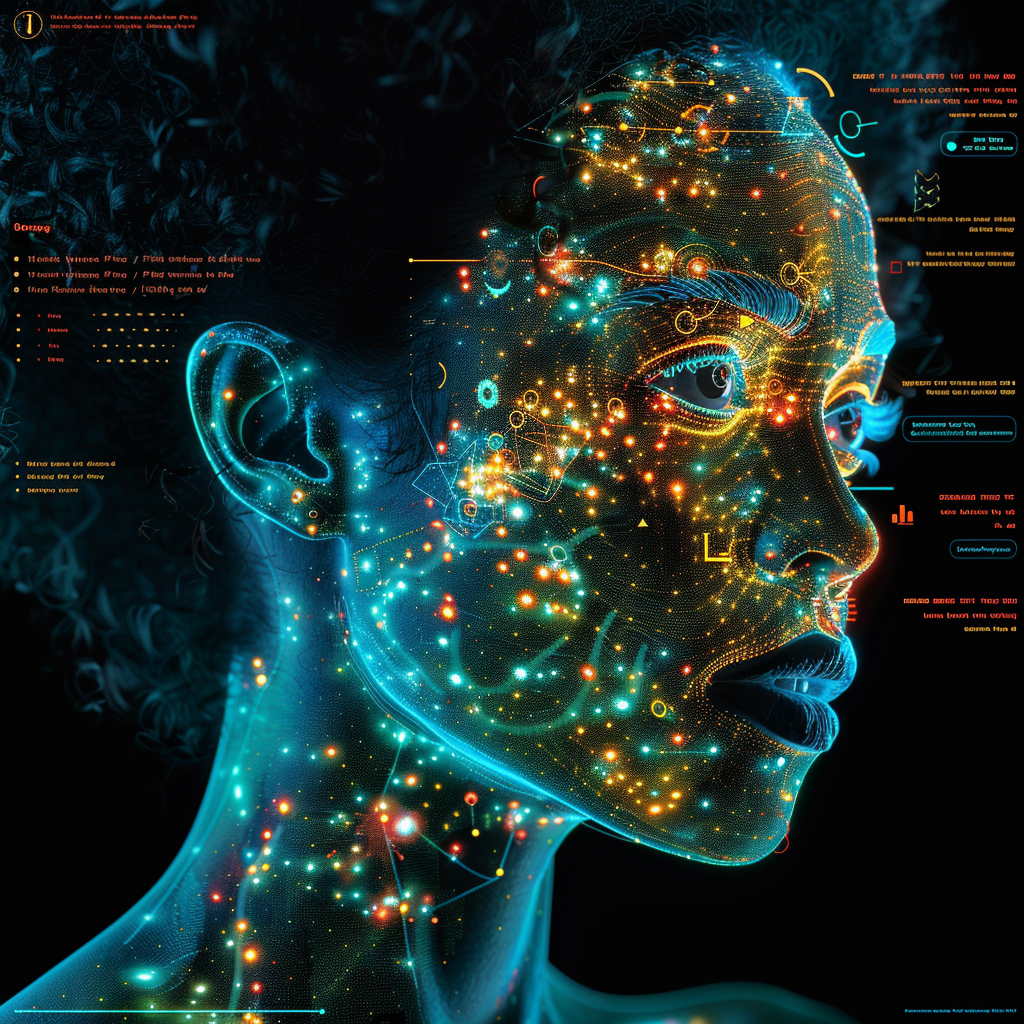
Sentiment analysis powered by AI examines text data to determine the emotional tone behind words. This technology is invaluable for businesses to gauge customer sentiment from reviews, social media, and other feedback channels, enabling them to better understand consumer preferences and adjust their strategies accordingly. AI algorithms are adept at picking up subtle cues in language to classify sentiments effectively.
4. Automated Content Generation
AI is being used to automatically generate written content for reports, news articles, and more, saving time and effort while maintaining a high level of quality and relevance.
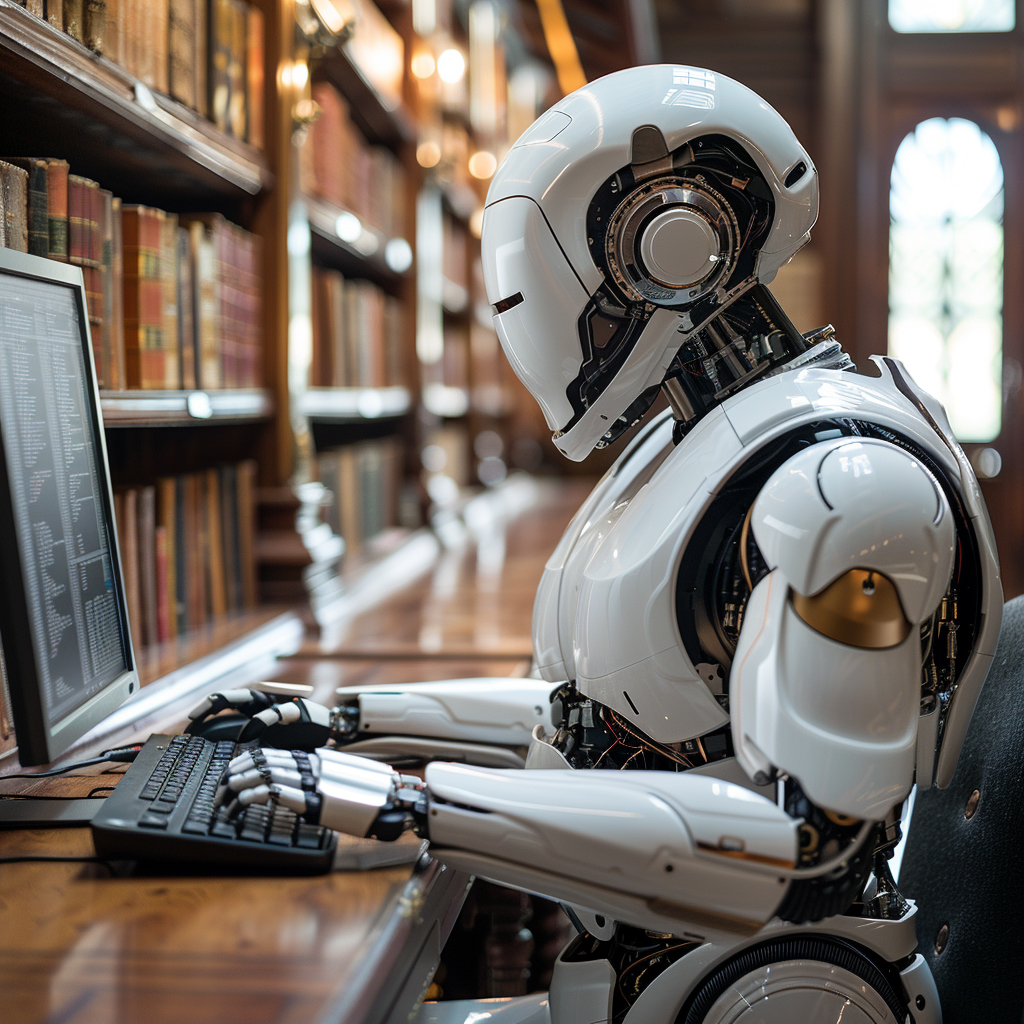
AI technologies are being used to automatically generate content for various applications, from drafting simple reports to creating entire articles or even books. These AI systems leverage data and pre-existing content to produce new material that is coherent and contextually relevant, drastically reducing the time and effort required for content creation while maintaining a high standard of quality.
5. Text Summarization
AI algorithms can summarize long documents into concise versions, retaining key information and points. This technology is invaluable for digesting large volumes of text quickly.
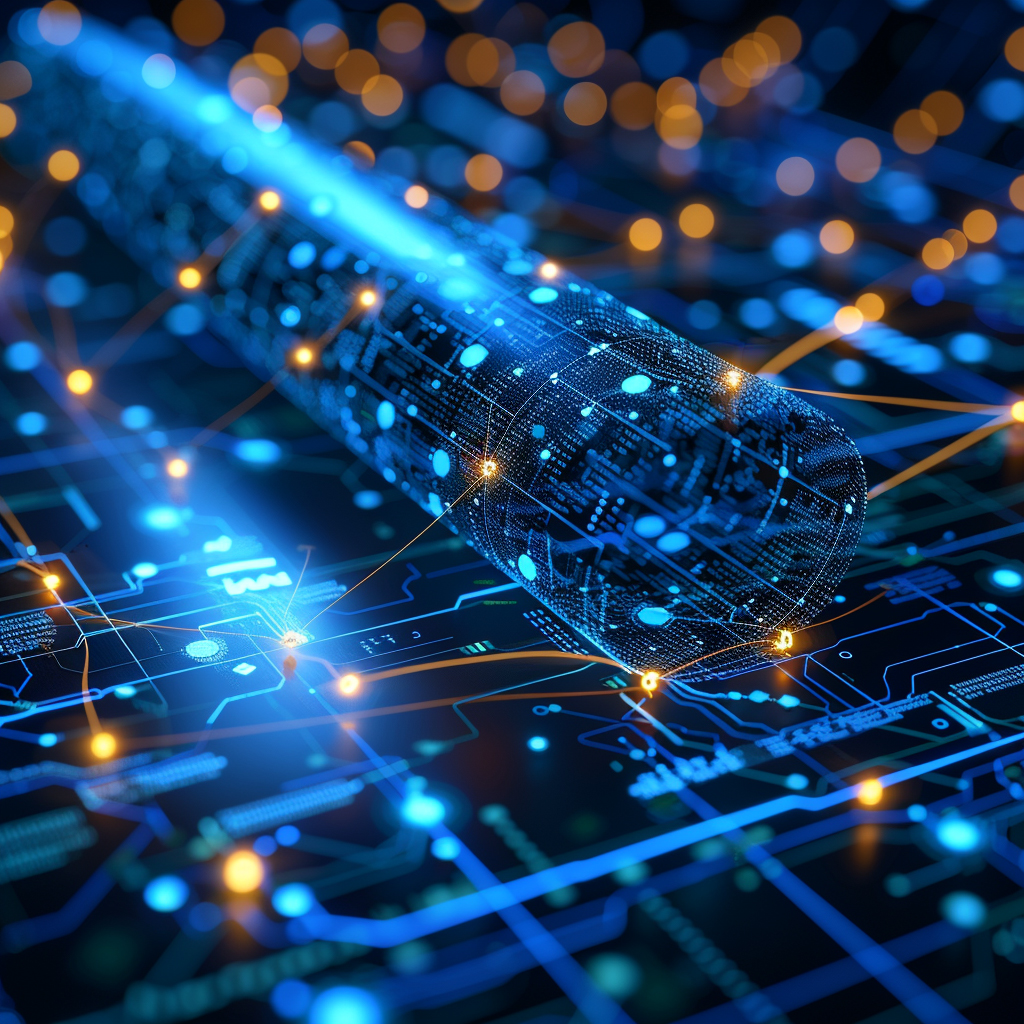
AI is improving the ability to condense lengthy documents into concise summaries without losing critical information. Using techniques like extraction and abstraction, AI models identify key points and essential data, presenting them in a shortened form. This technology is particularly useful for professionals and researchers who need to process large volumes of information efficiently.
6. Chatbots and Virtual Assistants
AI powers sophisticated chatbots and virtual assistants that can understand and respond to human queries with high accuracy, providing customer support and personal assistance.
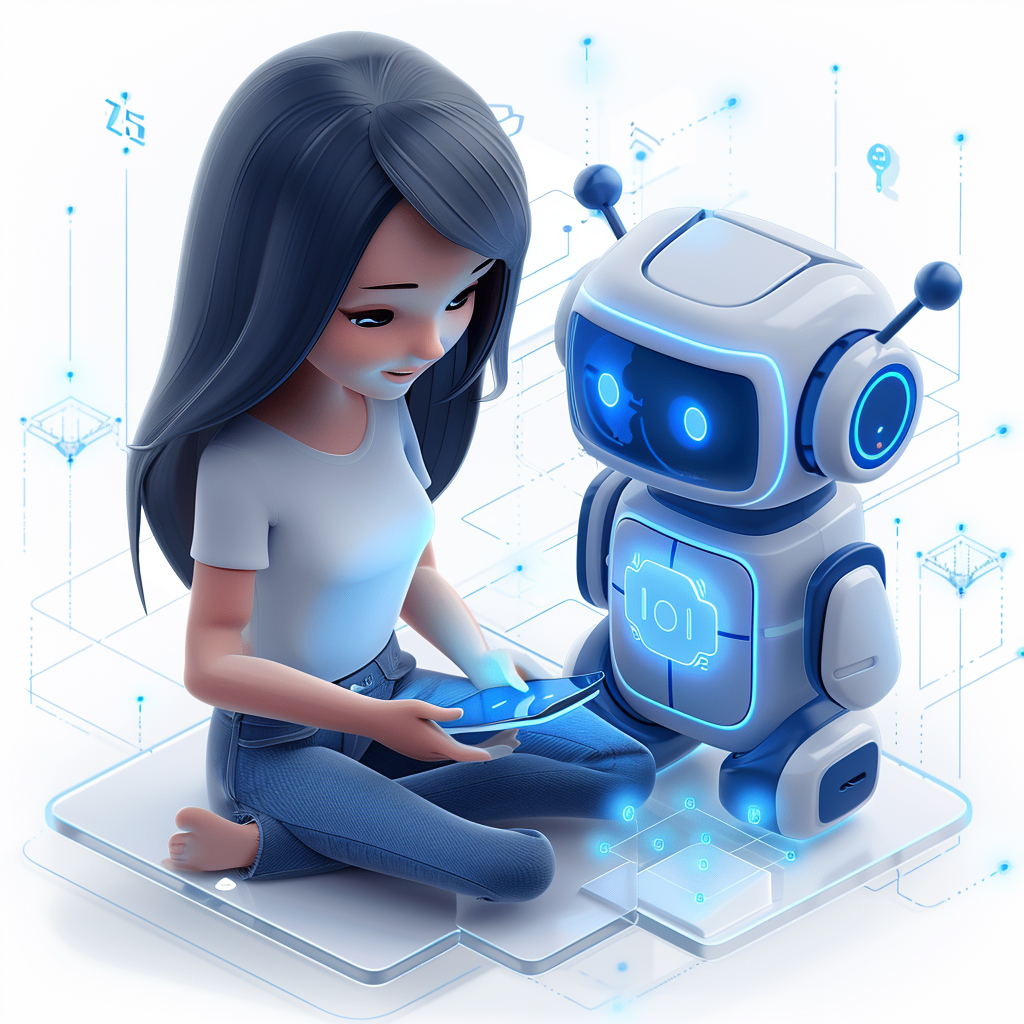
Chatbots and virtual assistants powered by AI are becoming increasingly sophisticated in how they understand and respond to user queries. These systems use NLP to parse human language, determine intent, and generate natural-sounding responses, enhancing user experience in customer service, personal assistance, and interactive applications.
7. Improved Information Retrieval
AI enhances search engines and other information retrieval systems to better understand and interpret user queries, returning more relevant results based on a deeper understanding of the content.
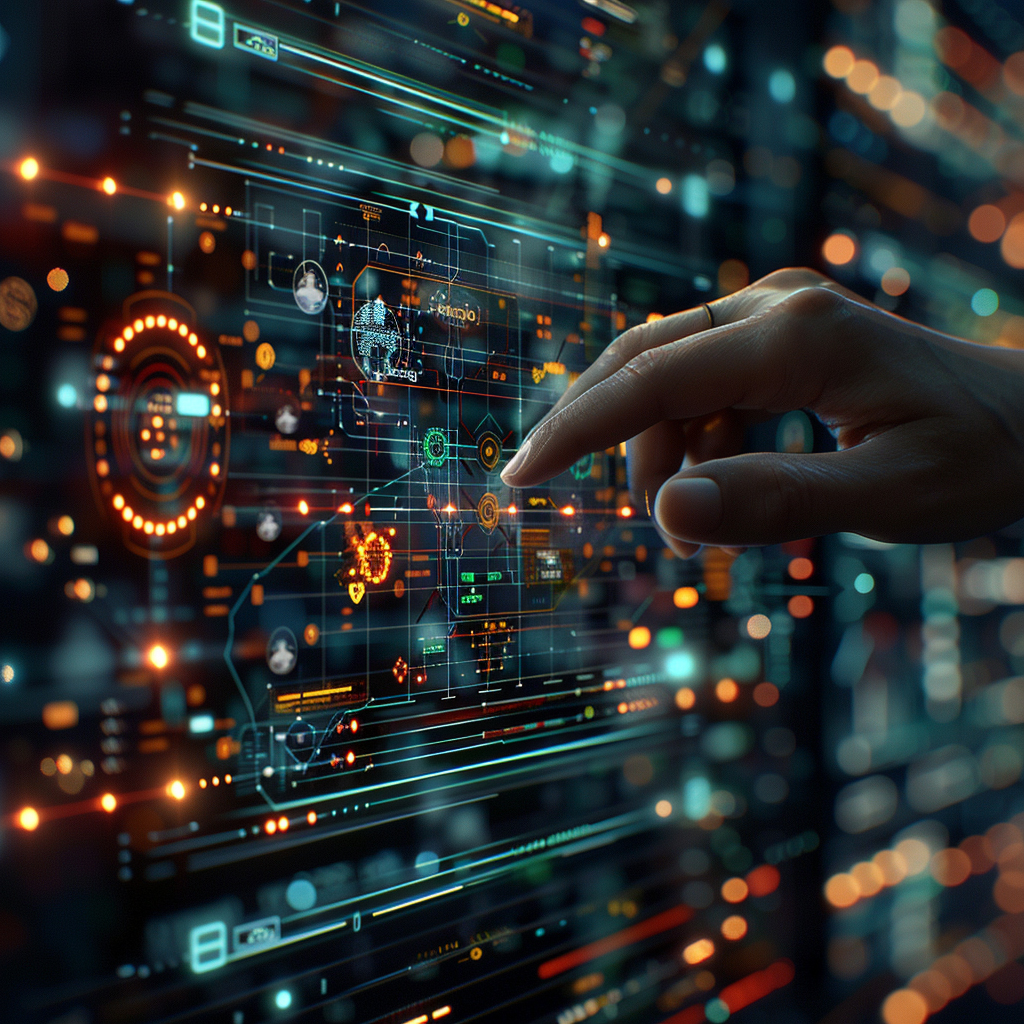
AI has enhanced information retrieval systems to better interpret the intent behind user queries, enabling more effective searches. By understanding natural language queries, AI can analyze and rank content relevance more accurately, providing users with results that are more aligned with their informational needs. This capability is crucial for search engines, academic research, and any application where users need to find specific information quickly.
8. Contextual Understanding
AI models are getting better at understanding context and ambiguity in language, which helps in more accurate responses and interactions, particularly in conversational AI.
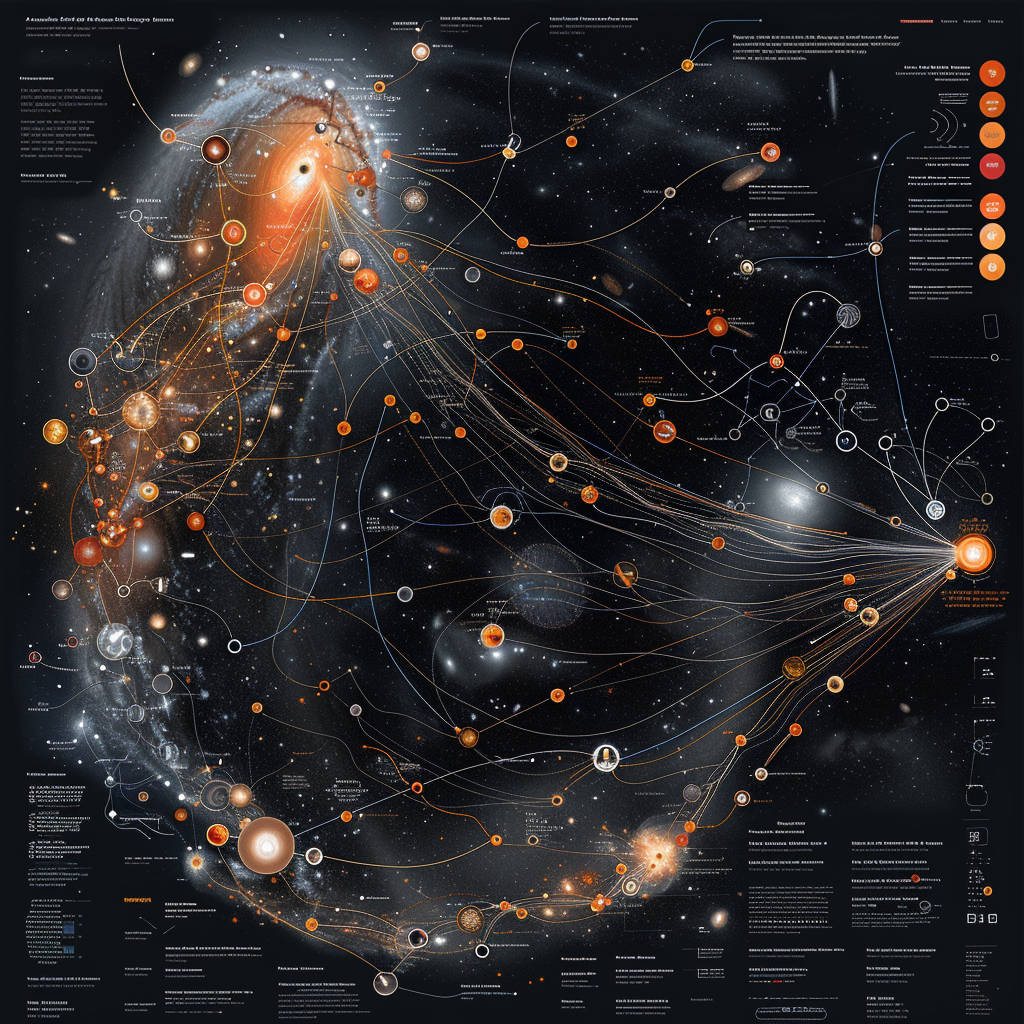
AI models have become adept at deciphering context and reducing ambiguity in communication. This improvement is crucial for applications like conversational AI, where understanding the context of a discussion can influence the response. By considering the broader conversation or text, AI can provide more appropriate and accurate interpretations and reactions.
9. Named Entity Recognition (NER)
AI is used for identifying and classifying key information in text, such as names of people, organizations, locations, dates, and other specifics, which can be crucial for data extraction and analysis.
-3.jpg)
Named Entity Recognition (NER) is an NLP task where AI identifies and classifies proper nouns and other specific information in text into predefined categories such as names of people, companies, locations, dates, etc. This functionality is essential for organizing and extracting valuable data from unstructured text, aiding in information management, and facilitating deeper data analysis.
10. Language Modeling and Prediction
AI is improving language models that predict text, enhancing the fluency and coherence of text in applications like auto-complete features in keyboards or writing assistants.
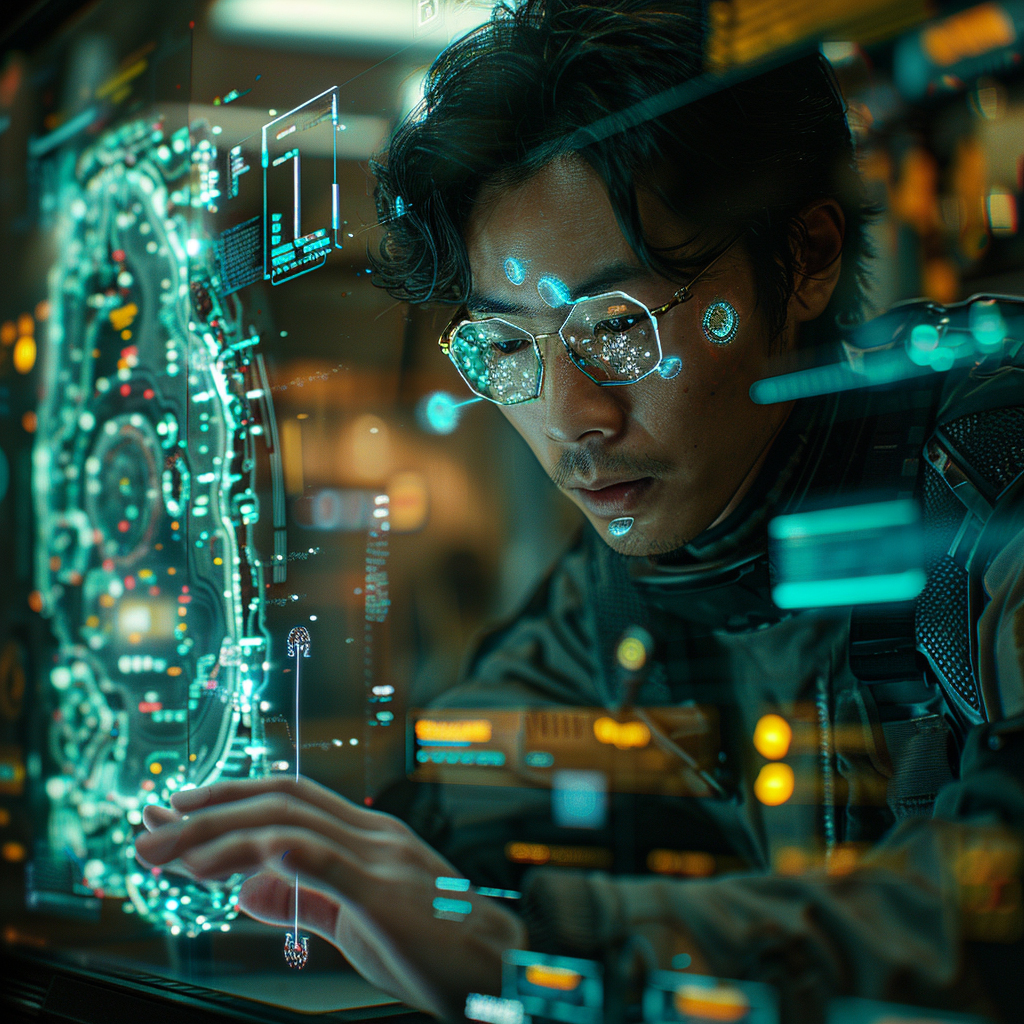
AI is enhancing language models that predict the next word or sequence of words during text input. This technology not only improves user experience in typing and text-based interfaces by suggesting contextually appropriate continuations but also helps in generating coherent and fluent text in various AI writing and communication applications.