1. Target Identification
AI algorithms analyze vast biological data sets to identify potential drug targets by understanding disease mechanisms at a molecular level.
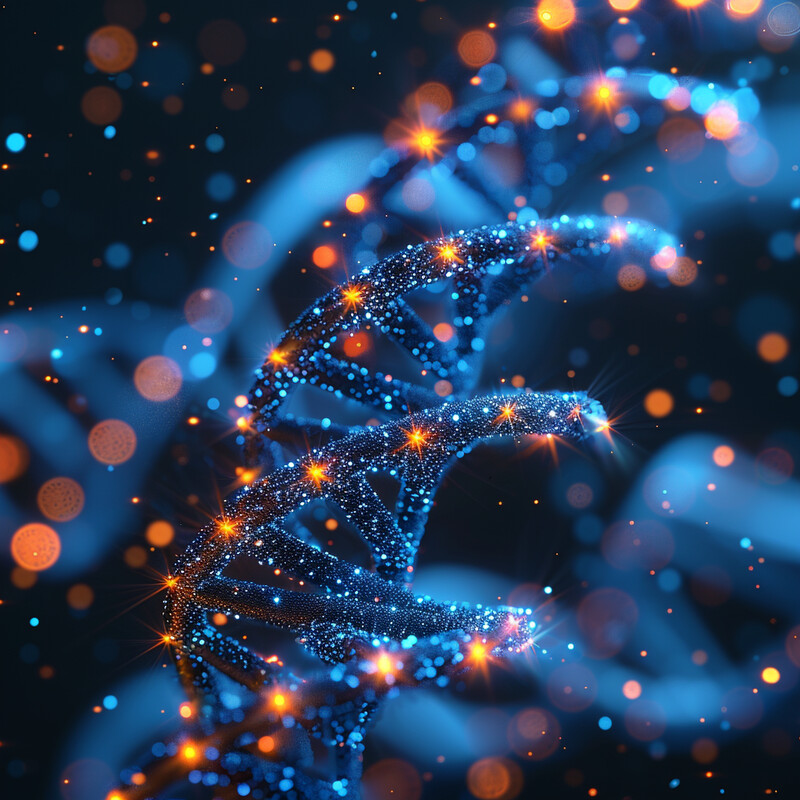
AI algorithms analyze vast biological datasets, including genomic, proteomic, and metabolomic information, to uncover potential drug targets. By understanding disease pathways and mechanisms at a molecular level, AI can identify proteins or genes that are promising targets for therapeutic intervention. This approach not only speeds up the initial phase of drug discovery but also increases the likelihood of success in later stages.
2. Hit Discovery
AI speeds up the hit discovery phase by predicting which molecules are likely to bind to a specific target, thus streamlining the screening process
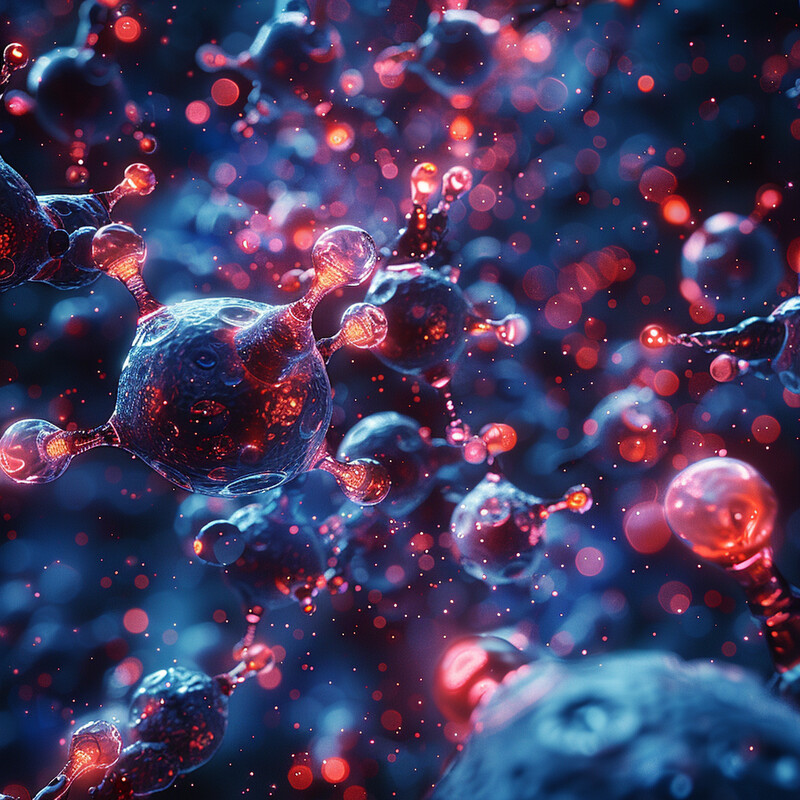
During the hit discovery phase, AI models predict the likelihood of molecules interacting effectively with specific biological targets. By assessing vast libraries of compounds quickly, AI significantly reduces the time and resources needed for experimental screening, allowing researchers to focus on the most promising candidates from the outset.
3. Lead Optimization
AI models simulate how modifications to chemical structures might affect a drug’s behavior, helping chemists optimize drug candidates for effectiveness and safety.
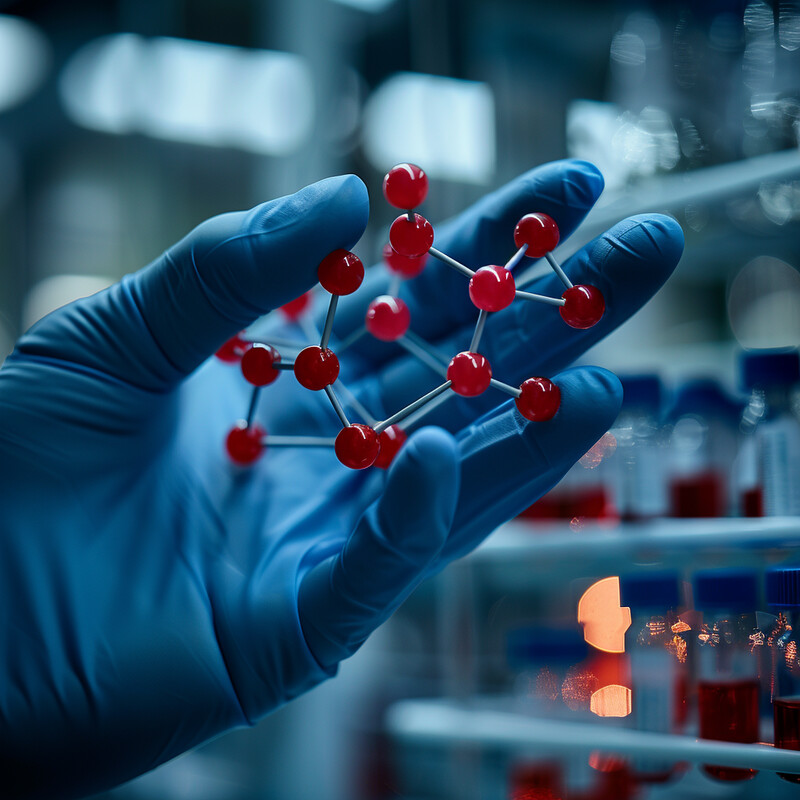
AI-driven models simulate potential modifications to chemical structures and predict their effects on a drug's pharmacological properties. This predictive capability enables chemists to refine molecules to enhance their efficacy, reduce side effects, and improve overall safety profiles before moving into costly clinical trials.
4. Prediction of Drug-like Properties
AI predicts pharmacokinetic properties of molecules, such as solubility and permeability, which are crucial for determining a compound's suitability as a drug.
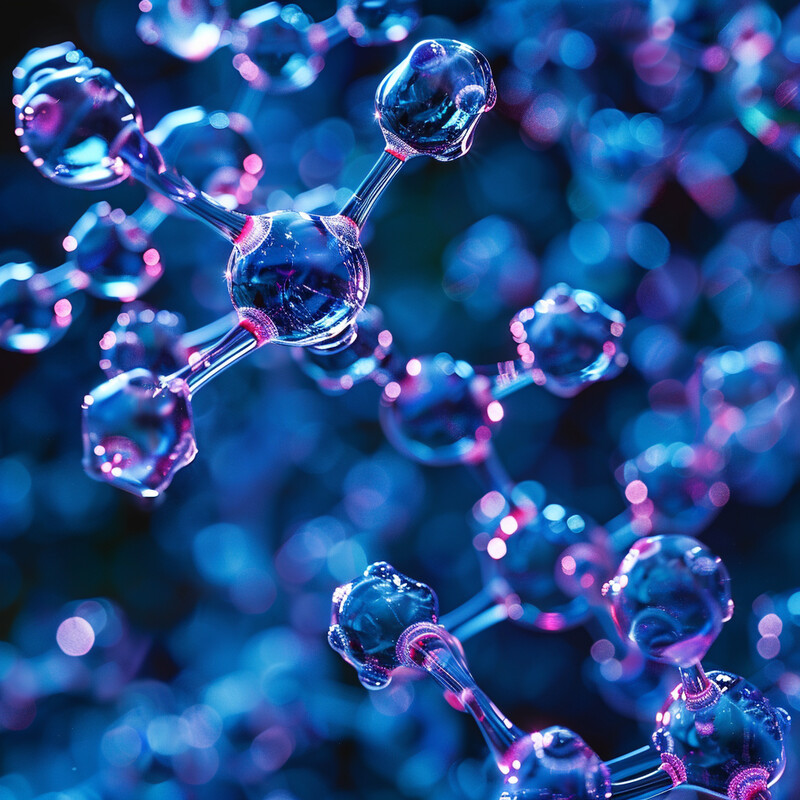
Determining a molecule's drug-like properties, such as absorption, distribution, metabolism, excretion, and toxicity (ADMET), is critical. AI predicts these properties early in the drug design process, helping researchers assess the feasibility of molecules as viable drug candidates and tailoring molecules to meet specific pharmacokinetic criteria.
5. Toxicity Prediction
AI uses historical data and pattern recognition to predict potential toxicity of new compounds early in the drug development process, reducing the risk of late-stage failures.
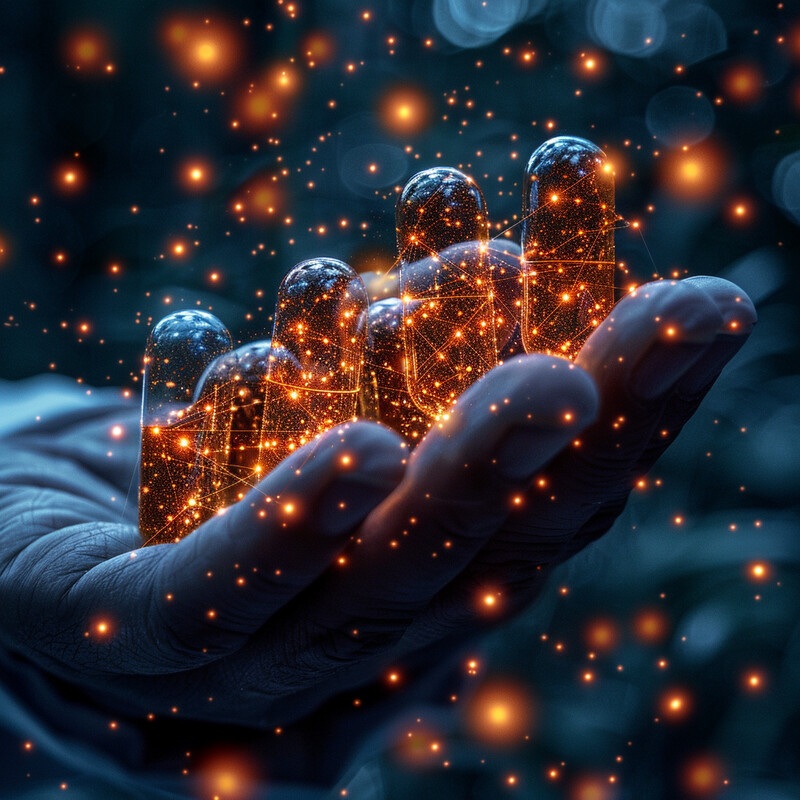
AI leverages historical data and advanced pattern recognition techniques to predict the potential toxicity of new compounds. Early identification of toxic properties allows researchers to modify or halt development on problematic molecules, significantly reducing the risk of failures in more advanced phases of drug testing.
6. Synthesis Prediction
AI assists in the synthesis of new drugs by suggesting possible synthetic pathways that are less costly and more efficient, and by predicting the success rate of these synthetic routes.
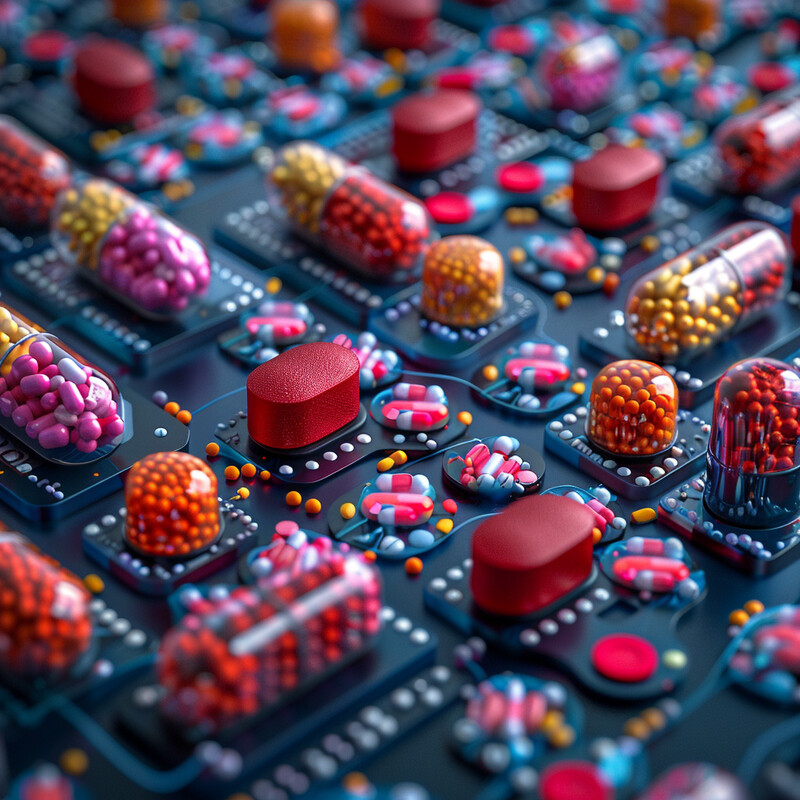
AI assists chemists in devising efficient synthetic pathways for new drugs. By predicting the most feasible routes of synthesis and the likelihood of successful outcomes, AI reduces experimental trial and error, decreases synthesis time, and lowers production costs.
7. Biased Library Design
AI designs focused libraries of compounds that are more likely to yield successful outcomes by analyzing trends in successful drug discovery projects.
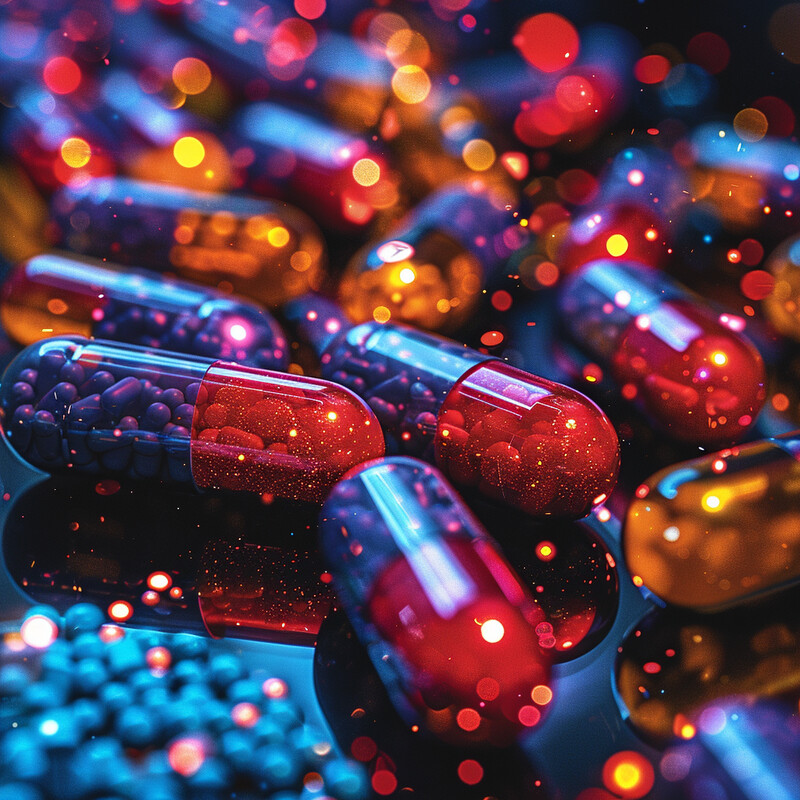
AI analyzes data from previous successful drug discovery projects to design libraries of compounds that are more likely to be effective. This approach focuses resources on exploring the most promising chemical spaces, enhancing the efficiency of discovery efforts and increasing the yield of successful outcomes.
8. Enhanced Drug Repurposing
AI analyzes existing drugs and their effects to identify new therapeutic uses, expanding the potential applications of known compounds.
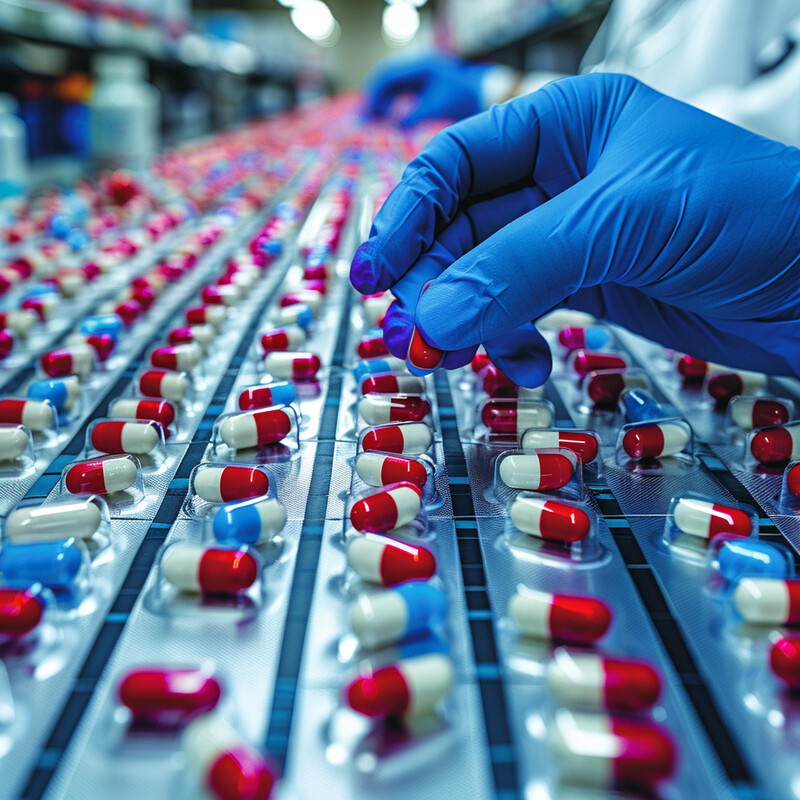
AI examines the known effects and mechanisms of existing drugs to identify potential new uses outside their original medical indications. This method of drug repurposing can significantly shorten development timelines and reduce costs by leveraging prior knowledge and approval of compounds.
9. Personalized Medicine
AI designs molecules tailored to individual genetic profiles, paving the way for more personalized and effective treatments.
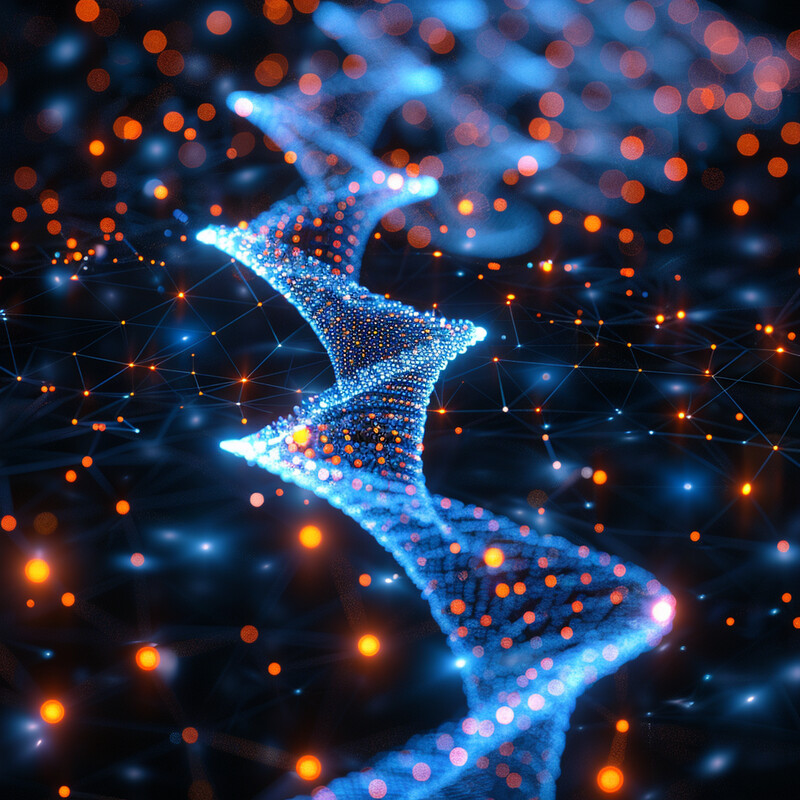
In the realm of personalized medicine, AI designs molecules that are specifically tailored to an individual’s genetic makeup. This precision approach promises more effective and tailored treatments, reducing the trial and error often associated with traditional drug prescriptions.
10. Automated Literature Review
AI continuously scans scientific literature to keep drug developers updated on the latest research findings, ensuring that molecular designs are informed by the most current knowledge.
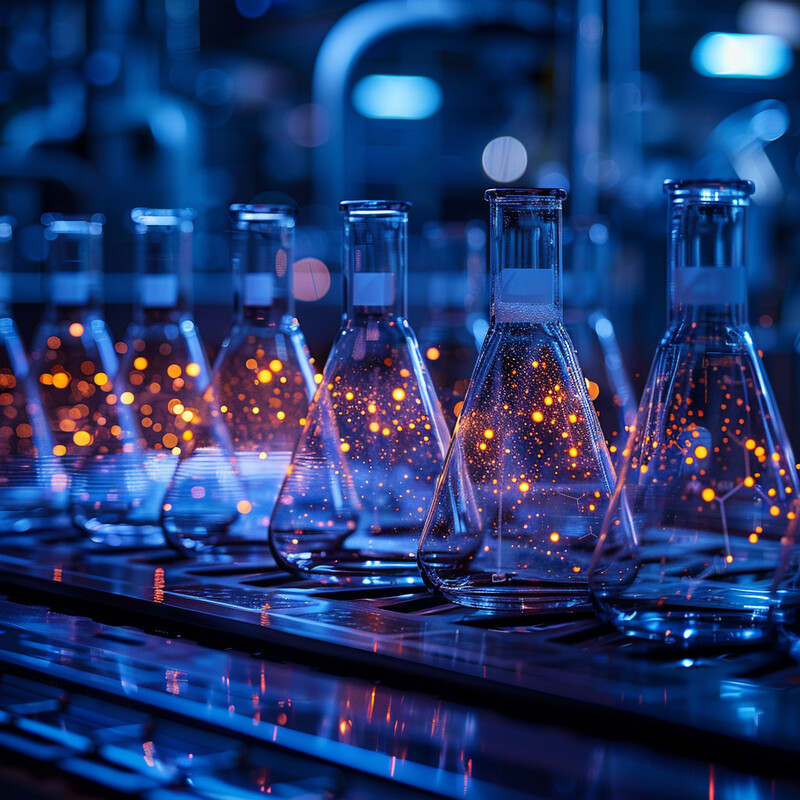
AI continuously scans and analyzes new research publications, extracting relevant information and keeping pharmaceutical researchers up to date with the latest scientific findings. This automation ensures that drug development is based on the most current knowledge, optimizing research and development strategies.