1. Personalized Therapy Recommendations
AI enables mental health apps to deliver highly individualized therapy content by analyzing user data and behavior patterns. Machine learning models process inputs like self-reported mood, symptom severity, and in-app activities to tailor recommendations that fit a person’s unique needs. Instead of a one-size-fits-all approach, the app’s AI can suggest specific coping strategies, exercises, or therapy modules most relevant to the individual’s situation. This personalization mirrors the way a human therapist would adjust treatment to a client, but it can be done continuously and automatically within the app. In a neutral and data-driven manner, AI ensures that users receive guidance that aligns with their personal progress and preferences, potentially increasing engagement and efficacy of the therapy.
AI analyzes user inputs and behaviors to tailor therapy sessions and recommend specific interventions or coping strategies that match individual needs.
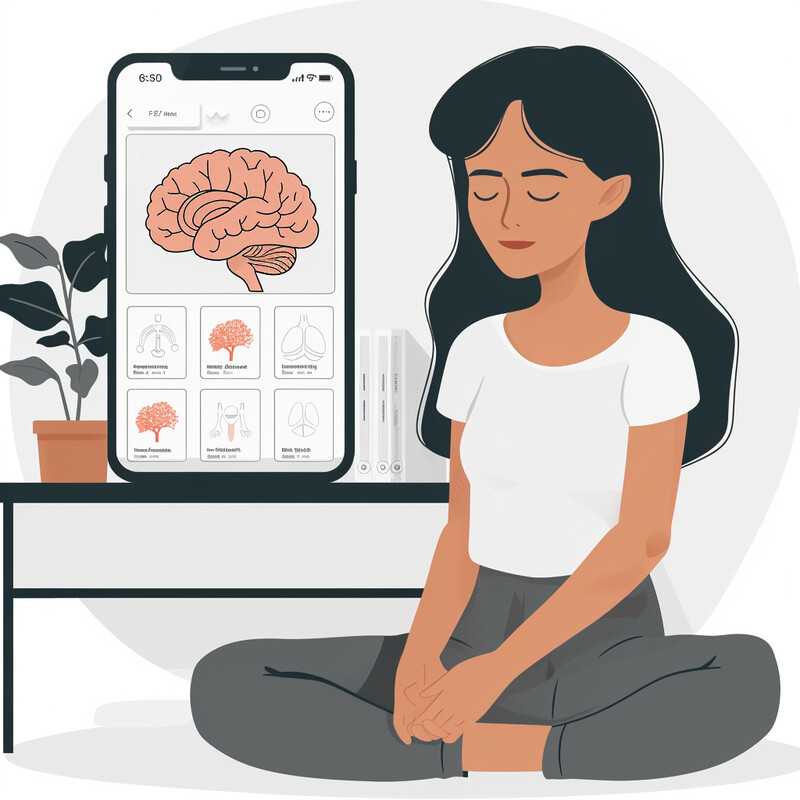
Early evidence suggests that AI-driven personalization can improve mental health outcomes. For example, a longitudinal study of over 4,500 users of an AI therapy app (“Youper”) reported moderate reductions in anxiety and depression symptoms after using the app’s tailored interventions. Users were engaged over several weeks, with 42.6% still active by week 4, indicating sustained use of the personalized program. Such findings lend credibility to AI-based personalized therapy, as the app’s recommendations were associated with meaningful symptom improvement in a large user base. The data from this 2021 study highlight how adapting therapeutic content to each user—through AI analysis of mood and behavior—can result in better emotional regulation and user satisfaction compared to generic advice.
AI algorithms in mental health apps analyze user inputs, such as self-reported mood, behavior logs, and interaction data, to tailor therapy recommendations. By understanding individual patterns and preferences, AI can suggest specific therapeutic interventions, coping mechanisms, or lifestyle adjustments that are most likely to benefit the user, making mental health support highly personalized and effective.
2. Mood Tracking and Analysis
AI-enhanced mood tracking in mental health apps allows users to record their emotions over time and receive insightful feedback. These apps often prompt users to log their mood daily (or even passively sense it via phone sensors), then use algorithms to detect patterns and correlations. In a neutral, factual way, the AI analyzes when and why mood fluctuations occur – for instance, identifying triggers like poor sleep, specific social interactions, or time of day associated with mood changes. By crunching large amounts of mood data, AI can alert users to trends they might not notice on their own, such as a dip in mood every Sunday night or improvement on days with exercise. Both users and clinicians can benefit from this analysis: it provides a data-driven understanding of an individual’s emotional cycles, enabling more targeted coping strategies or treatment adjustments. Overall, AI transforms raw mood logs into actionable insights, making mood tracking a more powerful tool for mental health self-awareness.
AI-enabled apps track mood fluctuations over time and analyze patterns to identify triggers, helping users and their therapists understand emotional cycles better.
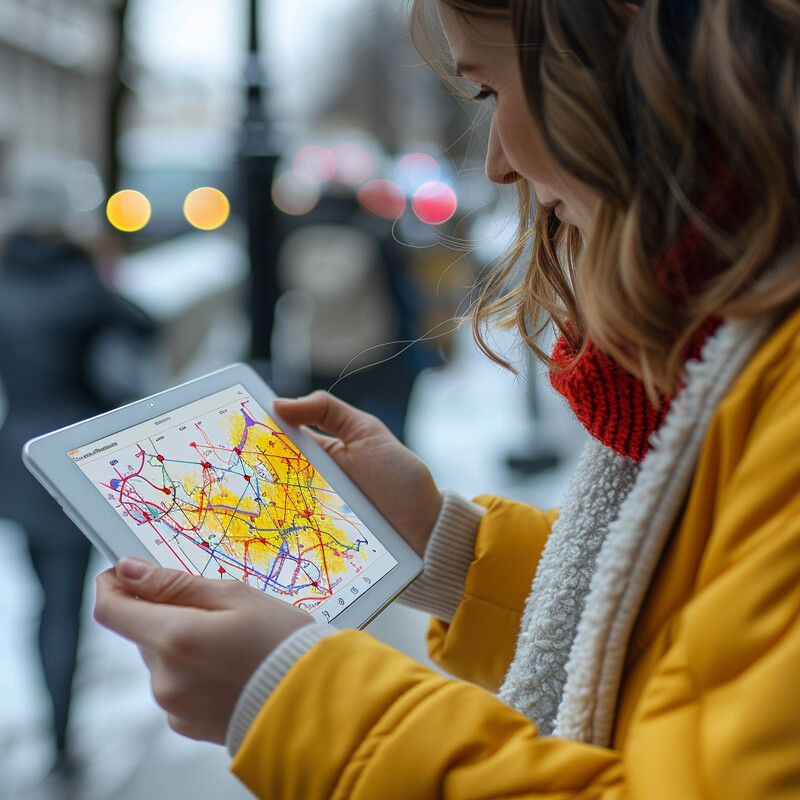
Adoption of AI-powered mood tracking is on the rise, especially among young people, underscoring its perceived value. A 2022 mixed-methods study surveyed 666 youths and found that more than two-thirds had experience using mental health apps, and 60% reported regular use of one or two such apps. Many of these apps include mood logging and AI analysis features, indicating that tracking one’s emotional state digitally is becoming common practice. Users appreciated how AI highlighted triggers and patterns; for example, the study noted generally positive attitudes toward AI in mental health, with only 19% expressing negativity about AI integration. This widespread usage suggests that millions are leveraging AI mood-analysis to better understand their emotions. While the effectiveness is still being evaluated, initial data show that AI can identify subtle mood trends (like the link between decreased physical activity and low mood) that help users and therapists manage mental health more proactively.
AI-powered mood tracking tools within apps systematically collect data on users' emotional states over time. By analyzing these mood logs, AI identifies patterns and potential triggers for emotional fluctuations, helping both users and therapists understand the underlying causes of mood swings and develop strategies to manage them more effectively.
3. Real-time Emotional Support
AI provides real-time emotional support through chatbots and virtual agents that are available 24/7 to those in distress. These AI chatbots use natural language processing to engage in text-based conversations that feel empathetic and supportive. In practice, when a user is feeling anxious, depressed, or overwhelmed at any hour, they can turn to the app’s chatbot which will respond immediately with understanding and evidence-based coping techniques. The AI is trained on therapeutic frameworks (like cognitive-behavioral responses or validation techniques) to offer calming advice, ask helpful questions, or guide the user through a grounding exercise. This kind of instant support is fact-driven in that it’s built on large datasets of counseling dialogues, enabling the bot to recognize crisis language or negative thought patterns and respond appropriately. AI chatbots serve as a first line of support during moments when a human therapist or friend might not be available, helping users navigate acute emotional episodes in real time and bridging the gap between therapy sessions with on-demand care.
AI-driven chatbots provide immediate emotional support and crisis intervention, offering conversational interactions that can guide users through distressing moments.
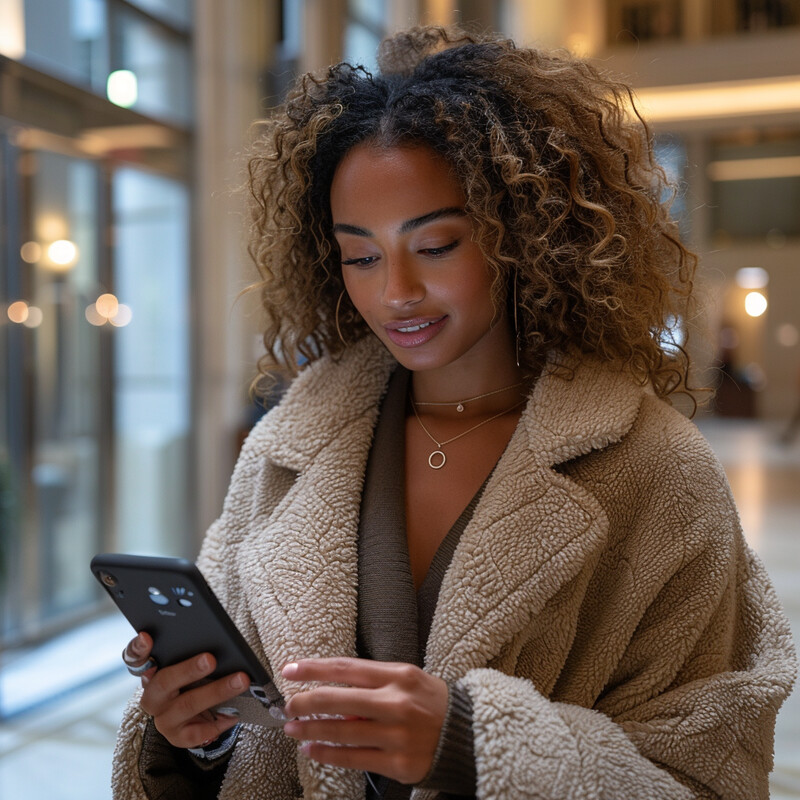
Studies have begun to validate the effectiveness of AI chatbots in reducing users’ distress and providing genuine support. In a randomized controlled trial, the AI chatbot “Woebot” (which interacts conversationally to deliver therapeutic content) significantly reduced symptoms of depression in young adults after just two weeks of daily engagement. Participants using Woebot reported lower depression scores than a control group, demonstrating measurable short-term benefits from the bot’s real-time support. Separately, a 2020 qualitative study found that users of a companion chatbot (Replika) felt it provided a safe, non-judgmental space to vent and receive encouragement, helping to alleviate loneliness and anxiety. Users described feeling heard by the AI and gaining emotional relief similar to talking with a supportive friend. These findings, though preliminary, indicate that AI-driven emotional support isn’t just a gimmick — it can yield real improvements in mood and serve as a valuable supplement to traditional mental health resources.
AI-driven chatbots offer real-time emotional support, acting as first-line responders during moments of distress. These chatbots use natural language processing to understand user queries and provide conversational support, offering coping strategies and soothing interactions to help users navigate through acute episodes of anxiety or depression.
4. Predictive Analytics for Risk Assessment
AI is increasingly used in mental health apps to predict serious risks (such as suicidality or relapse of a condition) before they fully manifest, allowing for proactive intervention. By examining a user’s historical data—mood trends, app usage patterns, messages typed, and even biometrics—advanced algorithms can identify red flags that a human might miss. For instance, a sudden drop in engagement combined with consistently low mood scores could signal a heightened risk of a depressive crisis. AI risk models continuously crunch these data points to estimate the probability of outcomes like a panic attack or self-harm, often giving an early warning score. This analysis is rooted in large-scale training data where the AI learned which patterns often preceded crises in others. As a result, the app can alert the user (or with permission, a clinician/caregiver) if their patterns match a high-risk profile—neutrally providing a “risk assessment” that something might be wrong. In essence, predictive analytics turns passive tracking into an active safety net: instead of waiting for a crisis to happen, the AI attempts to forecast it and prompt preventive measures or contact from professionals.
AI uses historical data and user interactions to predict potential mental health crises, enabling proactive management and timely intervention by healthcare providers.
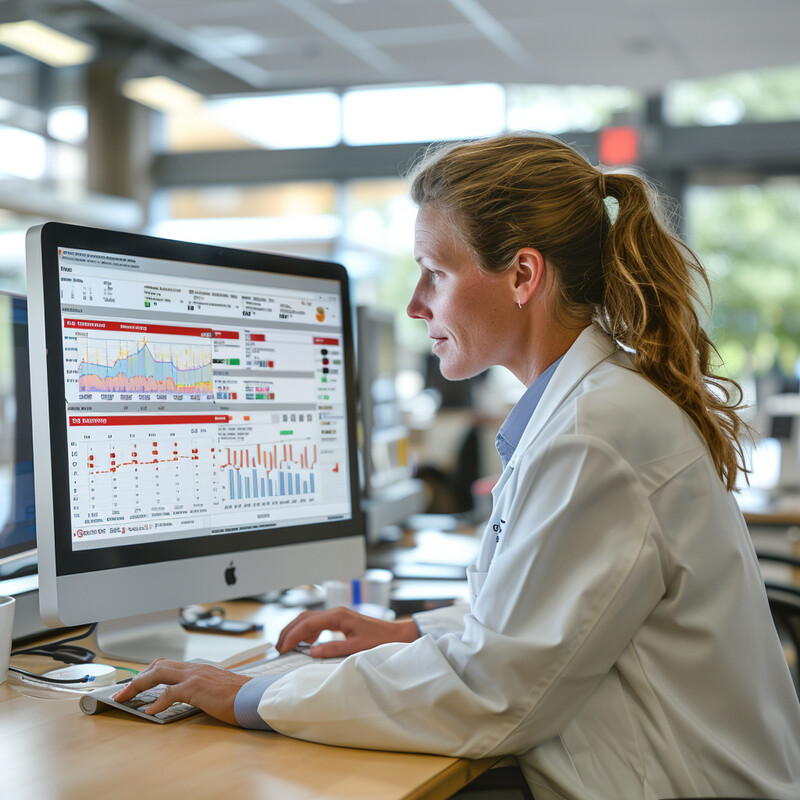
Data-driven risk prediction has shown notable accuracy improvements over traditional screening. A 2024 NIH-funded study developed AI models to predict suicide attempts by analyzing electronic health records and behavioral data; the AI correctly identified 82% of individuals who would go on to attempt or die by suicide within 90 days of a healthcare visit. In contrast, the standard screening methods (such as clinician assessments and questionnaires) only identified about 64% of those at-risk in the same sample. This means the AI approach significantly outperformed existing techniques, approaching a level far better than chance for such a complex prediction. The study, which involved data from over 16,000 patients, underscores AI’s potential in risk assessment. By catching more true positives, these predictive analytics tools can trigger timely interventions (like an app encouraging the user to seek help or alerting medical staff). It’s a compelling real-world example of how AI in mental health can move beyond reactive care to a more preventative, safety-focused model.
Using predictive analytics, AI models assess the risk of mental health crises by analyzing historical user data and ongoing interactions. This proactive approach enables timely interventions by alerting users, their support networks, or healthcare providers when there's a significant risk detected, thus preventing the escalation of mental health issues.
5. Cognitive Behavioral Therapy (CBT) Tools
AI is powering interactive Cognitive Behavioral Therapy tools in mental health apps, bringing structured therapeutic exercises to users anytime. These tools are grounded in CBT principles – identifying negative thought patterns, challenging and reframing those thoughts, and gradually changing behavior. An AI-driven CBT app might walk a user through a “thought record” exercise: the user types a distressing thought, and the AI responds with questions or alternative perspectives to gently dispute cognitive distortions. The experience is personalized and responsive; for example, if a user expresses a lot of self-criticism, the AI module might provide a tailored cognitive reframing exercise focusing on self-compassion. All of this is done in a neutral, guidance-oriented tone, often through a conversational interface or interactive worksheets. The AI adapts to the user’s inputs (skipping parts they seem to grasp well or reviewing areas they struggle with), making the exercise feel more like a guided coaching session than a static self-help worksheet. By simulating a therapist’s prompts and feedback, AI CBT tools help users practice proven techniques for managing anxiety or depression on their own, increasing access to these gold-standard therapy methods.
AI powers interactive tools that deliver cognitive behavioral therapy techniques, helping users challenge negative thoughts and change behavior patterns.
-tools-2.jpg)
Clinical trials indicate that AI-delivered CBT can yield tangible improvements in mental health. In a randomized controlled trial with young adults, using a fully automated CBT chatbot (named Woebot) led to a significant reduction in depressive symptoms within two weeks. Participants in the trial who engaged with the Woebot app reported lower depression and anxiety scores than those in the control group, who received only a self-help ebook, according to the published results. The chatbot guided users through CBT techniques via brief daily conversations, demonstrating that a purely digital intervention can replicate some benefits of traditional therapy in the short term. Another evaluation of an AI-based CBT app (Wysa) in 2018 found high user engagement and qualitative feedback suggesting reductions in stress and improvement in mood over several weeks of use. While long-term efficacy is still being studied, these early trials and user data support that AI-driven CBT tools are not only feasible but can measurably help reduce negative thoughts and feelings – validating the concept that therapeutic exercises don’t always require a human therapist present to be effective.
AI integrates cognitive behavioral therapy techniques into apps, providing users with interactive tools that help them recognize and alter negative thought patterns and behaviors. These AI-driven CBT tools guide users through exercises that challenge irrational thoughts, track progress, and gradually help in adopting healthier thinking habits.
6. Sleep Improvement
AI in mental health apps also targets sleep, recognizing that better sleep hygiene and quality can greatly improve mood and resilience. These apps often track sleep patterns either through user input (like a sleep diary) or via wearable devices and phone sensors (detecting movement and ambient light). The AI then analyzes this sleep data for issues such as irregular sleep schedules, insufficient duration, or frequent nighttime awakenings. In a neutral, coaching tone, the app provides personalized recommendations to improve sleep – for example, suggesting an earlier bedtime based on the user’s fatigue levels, or proposing a wind-down routine if it detects restless activity before bed. It may guide users through relaxation techniques like guided meditations or breathing exercises at night, adapting the suggestions over time as it learns what helps the individual fall asleep faster or sleep more soundly. Importantly, AI can also correlate sleep quality with the user’s reported mental health (noticing, say, that anxiety spikes after nights of poor sleep). By treating sleep as a key component of mental well-being, these AI tools help users make incremental changes (consistent wake times, reduced screen exposure at night, etc.) that cumulatively lead to better rest. In turn, improved sleep can reduce stress, stabilize mood, and enhance overall mental health.
AI analyzes sleep patterns and provides personalized recommendations and exercises to improve sleep quality, which is crucial for mental well-being.
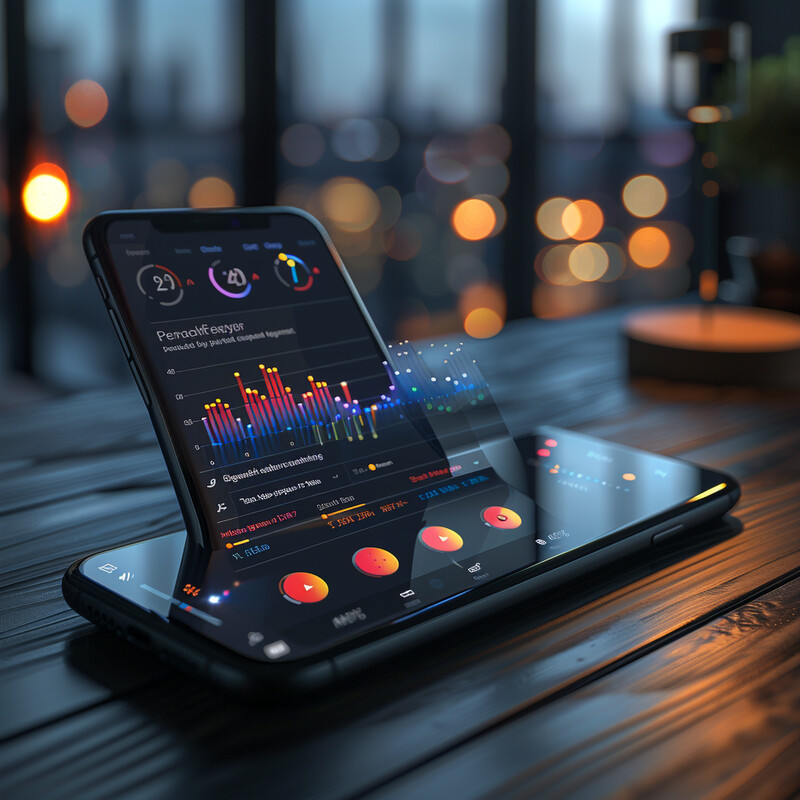
Research shows that digital CBT for insomnia, often delivered via AI-guided apps, can significantly improve sleep and even alleviate co-occurring mental health symptoms. A 2023 study pooled data from two large randomized trials (over 2,100 participants) testing an AI-driven online insomnia program (“Sleepio”). The results showed that those who used the AI sleep intervention had much greater improvements in sleep outcomes than those who received standard sleep hygiene advice – the effect size for insomnia severity reduction was around 0.8, which is considered large. Moreover, participants in the digital sleep program also saw meaningful reductions in anxiety symptoms (effect size ~0.4), highlighting that better sleep translated into better mental health. Another finding: in a subgroup of users with clinical anxiety, 76% no longer had significant insomnia after using the AI-guided sleep app, compared to only 30% in a control group, according to a 2024 analysis. These data underscore that AI-facilitated sleep improvement isn’t just theoretical – it produces real benefits. By helping users implement CBT-insomnia strategies (like adjusting sleep schedules and reducing pre-sleep worry), such apps have achieved high remission rates for insomnia and even secondary benefits like reduced depression and anxiety, all through a scalable digital format.
AI analyzes data related to users' sleep patterns collected through app inputs or wearable devices. It then provides personalized insights and recommendations to improve sleep hygiene, such as adjusting bedtime routines, meditation exercises, or environmental changes, recognizing that good sleep is foundational to mental well-being.
7. Stress Reduction Techniques
Many mental health apps now use AI to help users manage and reduce stress through personalized exercises. These apps can gauge a user’s stress level in real time – either through direct input (like the user reporting feeling “very stressed”) or by inferring it from patterns (such as physiological data from a smartwatch or the content of journal entries). The AI then suggests an appropriate stress reduction technique suited to that moment and user. For example, if the user is experiencing acute stress at work, the app might prompt a short guided breathing exercise, complete with on-screen visuals and a calm voice, adjusting the pace based on the user’s feedback. If the AI notices chronic stress patterns, it might recommend a daily mindfulness meditation of gradually increasing length, tailoring difficulty as the user progresses. The tone is encouraging and supportive, with the AI checking in (“Did this exercise help you feel calmer?”) to refine future suggestions. By continuously learning what strategies the individual responds to best – be it progressive muscle relaxation, visualization, or even a quick relaxing game – the AI provides a toolkit of coping methods. Over time, users practice these techniques regularly and can build resilience, with the AI acting as a personalized stress coach that’s available whenever tension spikes.
AI suggests and guides users through stress reduction exercises like guided meditation or breathing exercises based on their stress levels and preferences.
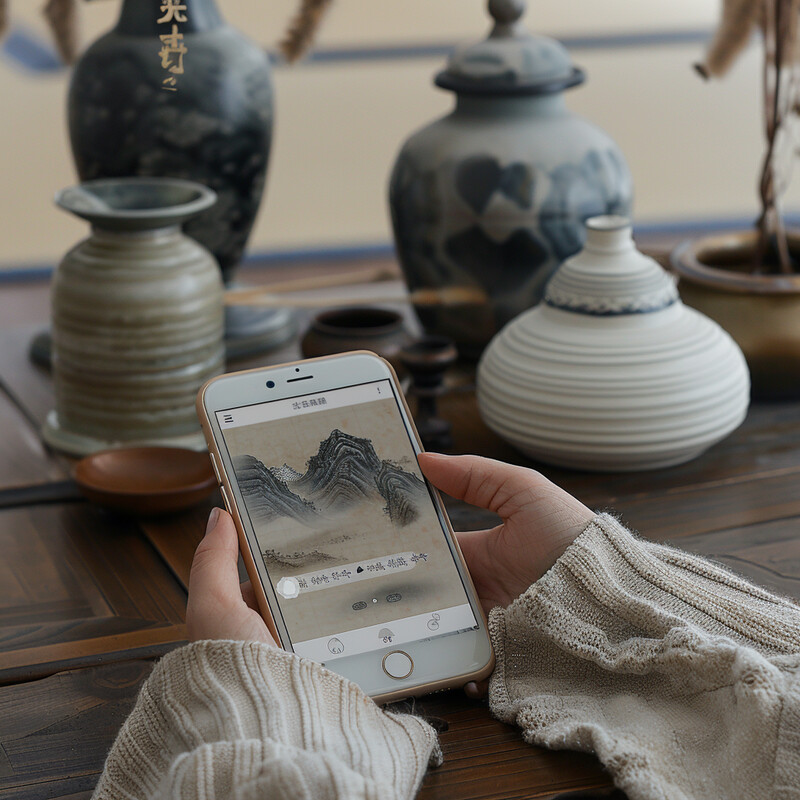
Evidence is emerging that AI-guided stress reduction can measurably improve users’ well-being. In a 2023 pilot study, an AI-driven “relational agent” (Woebot) provided an 8-week stress management program to adults, and the outcomes were promising. Participants reported significant reductions in perceived stress levels from baseline by the end of the program. The same study found burnout symptoms (often related to chronic workplace stress) also decreased substantially, and resilience scores increased, among those using the AI tool. Notably, individuals who started out with higher anxiety or mood symptoms benefited the most in terms of stress reduction, though improvements were seen across the board. These results indicate that even without live human coaching, a structured AI-based intervention can help users relax and cope better with daily pressures. Additionally, user engagement was high – suggesting people found the AI’s stress-relief exercises accessible and helpful. While larger trials are needed, this data aligns with countless anecdotal reports that guided meditation apps and breathing coaches (often powered by AI to some degree) are effective in lowering momentary stress. It provides a data-backed reassurance that AI can deliver clinically relevant stress relief techniques, not just generic advice.
Based on real-time assessments of users’ stress levels, AI suggests appropriate stress reduction techniques. Whether it’s through guided meditation, breathing exercises, or progressive muscle relaxation, AI guides users step-by-step, adapting the difficulty and duration based on their progress and feedback.
8. Behavior Modification Programs
AI helps power behavior modification programs in mental health apps by tracking user habits and providing timely, tailored feedback to encourage healthier behaviors. These apps monitor activities that can impact mental health – such as exercise frequency, screen time, social interaction, or even diet – often through phone sensors or connected wearables. The AI establishes a baseline for the user and then sets achievable goals (for instance, increasing daily steps by a certain amount, or reducing late-night phone use). What sets AI apart is its ability to personalize nudges: if a user tends to be sedentary in the afternoons, the app might send a friendly prompt at 3 PM suggesting a short walk, referencing the user’s own goal (“You’ve been sitting for 2 hours, how about a 5-minute stretch now?”). These prompts are data-driven, appearing at moments when they’re most needed. The AI also adapts to progress – if the user consistently meets a target, it might gently raise the bar, whereas if they struggle, it offers encouragement or adjusts the goal to be more attainable. Over time, such programs help replace detrimental habits (like prolonged inactivity or irregular routines) with positive ones, using reinforcement learning to figure out which motivational strategies (a congratulatory message, a visual progress chart, small rewards badges, etc.) work best for the individual. Through continuous monitoring and feedback, AI serves as a personal habit coach that supports behavior changes known to improve mental health (like regular exercise, adequate downtime, or routine activity levels).
AI tracks user behaviors and provides feedback and strategies to help modify habits that are detrimental to mental health, such as procrastination or lack of physical activity.
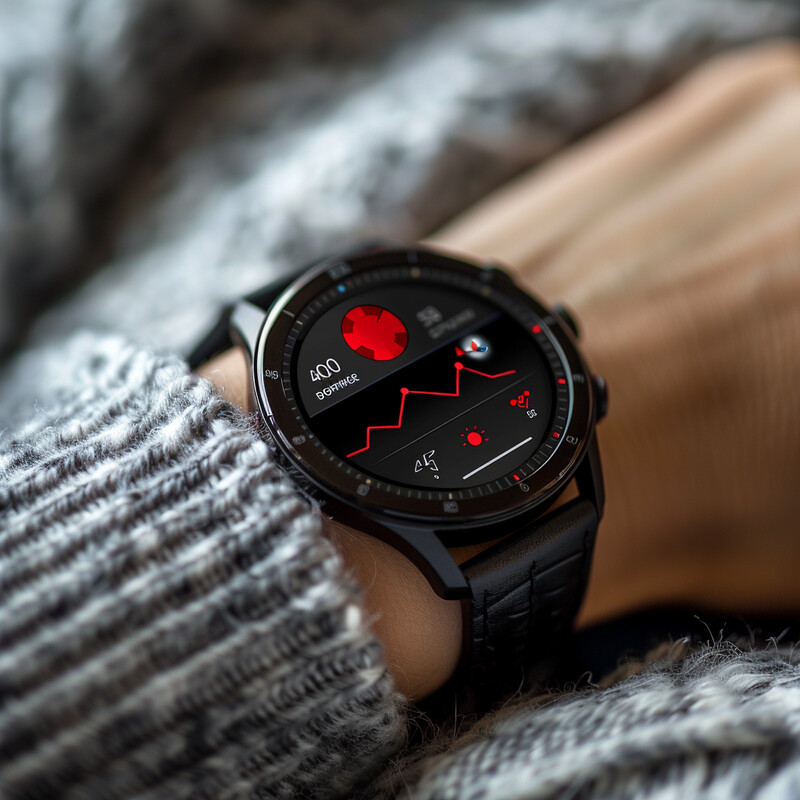
Personalization significantly boosts the effectiveness of digital behavior change interventions. A Stanford-led clinical trial in 2023 tested various app-based prompts to increase physical activity among 2,500 adults, and found that tailored coaching messages from an AI had the greatest impact. Participants who received personalized daily e-coaching (based on their own activity patterns) increased their step count by an average of over 400 steps per day – roughly a 10% improvement in activity. This gain was notably higher than in groups receiving generic prompts (for example, a non-personalized reminder to take 10,000 steps, which led to a smaller ~215 step increase). Another intervention in the study, which sent hourly prompts to stand up after prolonged inactivity, also boosted steps (by ~292 steps), but still less than the AI-customized approach. These results, published in European Heart Journal – Digital Health, demonstrate that an AI which “learns” an individual’s behavior and provides context-aware nudges can more effectively change habits than one-size-fits-all messaging. In practice, this means mental health apps using AI to, say, encourage regular exercise or consistent sleep schedules can achieve significantly better adherence. By dynamically adjusting to user behavior (in this case, increasing walking when one is typically idle), the AI made the healthy choice easier and more compelling — leading to measurable behavior change that supports mental well-being.
AI monitors user behaviors that impact mental health, such as physical activity levels, screen time, or social interactions. It provides personalized feedback and actionable strategies to modify these behaviors. By setting goals and tracking progress, AI helps users develop healthier habits that support their mental health.
9. Community and Peer Support
AI is enhancing community and peer support features in mental health apps by smartly matching users to relevant support networks and moderating those spaces for safety. When a user joins an app and perhaps fills out a profile or answers questions about what they’re going through, AI algorithms can recommend online peer groups or forums that align with their needs (for example, a young adult anxiety support chat, or a community for new mothers dealing with postpartum blues). This matching is based on data – the AI looks at the topics of discussion in various groups, the demographics, and the user’s expressed interests or conditions to find a good fit. Once in a peer support environment, AI tools help maintain a helpful atmosphere: they might flag potentially harmful posts (such as those indicating severe distress or use of triggering language) for human moderators or provide automatic gentle guidance according to community guidelines. Some apps deploy AI “facilitators” that can prompt discussion or check in if someone hasn’t posted in a while (“You mentioned feeling down last week – how are things now?”), thus keeping the support dynamic. In a neutral but compassionate manner, AI thus expands access to the age-old practice of peer support, making it easier for individuals to find and engage with others who have similar experiences. The result is a more inclusive, well-managed online support community where users can share, vent, and heal together, with AI working behind the scenes to ensure everyone finds the right group and that interactions remain positive and safe.
AI matches users with peer support groups or online communities based on their mental health conditions and preferences, facilitating a supportive environment for sharing and healing.
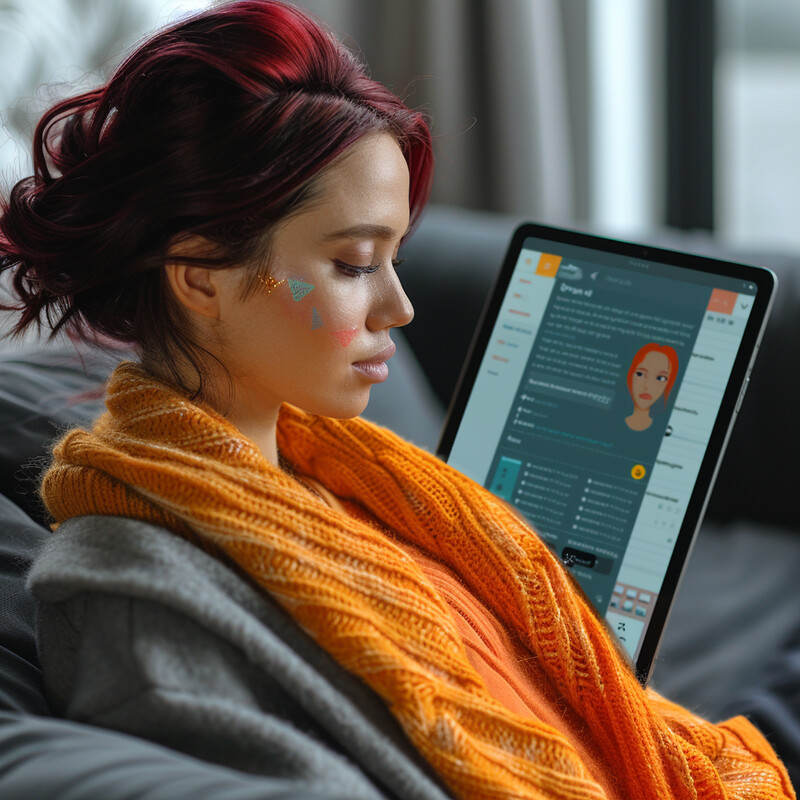
Structured digital peer support, when implemented with guidance (often facilitated by an app platform), has shown significant benefits for users’ mental health. In a 2023 randomized controlled trial involving 100 emerging adults in Singapore, those who participated in a digital peer support program (via a platform called Acceset) experienced greater improvements in well-being compared to a control group. Over the course of the intervention, measures of self-compassion, mindfulness, and perceived social support increased in the peer support group, while symptoms of depression and anxiety decreased, whereas the control group (waitlisted for the intervention) did not show such changes. The study also found that the positive effects were sustained beyond the active intervention period, suggesting lasting benefits from engaging with trained peer “befrienders” on the app. Importantly, the peer helpers in the app were given some training and the platform was structured – indicating that when AI or digital systems organize peer support in a purposeful way, it can be clinically effective. Another review of online support forums (2023) has noted that while open social media groups have mixed outcomes, dedicated peer support platforms with some moderation (often AI-assisted) show promise in reducing loneliness and imparting coping skills. Taken together, current data supports that AI-curated and overseen peer communities can be a powerful adjunct to traditional care, offering users empathy and understanding from those with lived experience, which in turn improves mental health outcomes.
AI facilitates connections between users and peer support communities by matching individuals with similar conditions or interests. This matching is based on detailed analysis of user profiles and interaction patterns, ensuring that users find supportive, understanding communities that can enhance their healing process.
10. Therapist Assistance Tools
AI is not only user-facing; it also works in the background of mental health apps to assist therapists in delivering better care. These AI-driven therapist assistance tools aggregate data from patients’ app usage, self-reports, and even session transcripts (if integrated with teletherapy) to give clinicians a clearer picture of patient progress. For example, an app might compile a weekly report for the therapist showing the patient’s mood charts, notable journal entries (flagging keywords like “hopeless” or “improved” using natural language processing), and whether the patient completed homework exercises. The AI can analyze this information to highlight trends – perhaps it notes, neutrally, that “Anxiety symptoms have steadily decreased 20% over 4 weeks, but sleep quality dropped in the last week.” This assists the therapist in quickly pinpointing areas to address in the next session or adjusting the treatment plan (like focusing on insomnia). Some AI tools even provide decision support by comparing the patient’s profile to large datasets (“patients with similar profiles tended to benefit from CBT combined with medication at this stage”) – though final decisions are of course left to the human clinician. Additionally, AI can handle routine tasks such as transcribing session notes or scoring assessment questionnaires automatically, reducing the administrative burden on therapists. By leveraging AI for data analysis and routine work, therapists can use session time more efficiently, focusing on empathy and strategy, armed with deeper insights and freed from some paperwork. This ultimately can improve the quality and personalization of care each client receives.
AI compiles and analyzes session notes, mood tracking data, and user feedback to assist therapists in understanding their clients' progress and adjusting treatment plans accordingly.
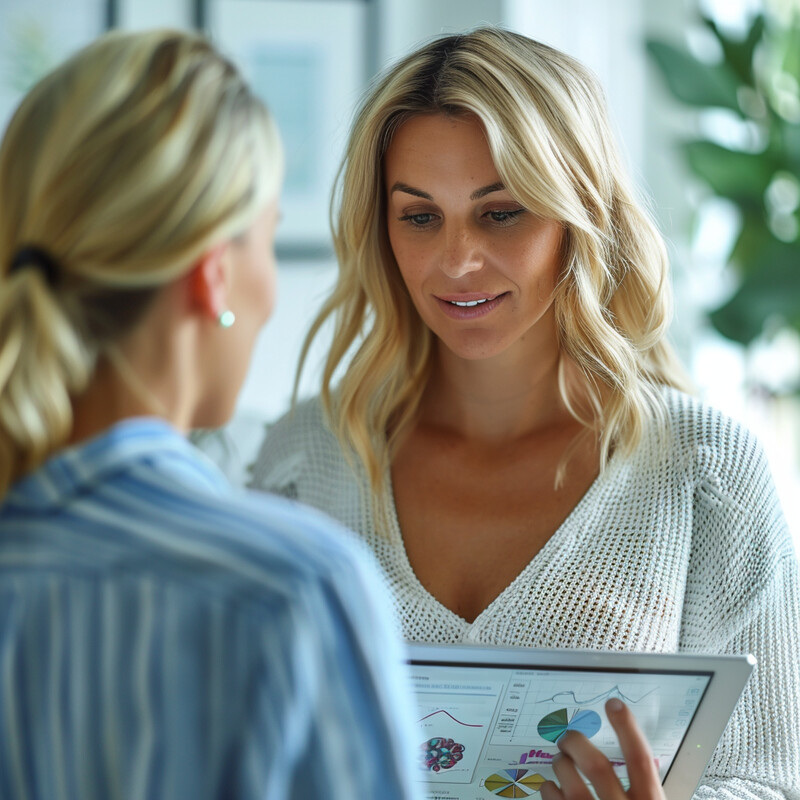
Mental health professionals are increasingly open to incorporating AI and digital tools into their practice, reflecting confidence in these assistants. In a survey conducted from 2020 to 2023 among psychiatrists, psychologists, and psychotherapists (primarily in Poland), 86% of respondents agreed that mobile health tools could support patients in managing their mental health, and 74% said they would recommend such apps to their patients. By 2023, about 84% of these professionals expressed readiness to use new digital technologies (like apps that track mood or AI-based diagnostic aids) as part of treatment. This trend is likely similar in the U.S. and other countries as digital therapeutics gain traction. The high acceptance suggests that therapists see value in AI-curated patient data and insights – many clinicians report that seeing objective mood data or AI summaries of patient progress helps inform their care decisions (for example, knowing a patient’s daily anxiety ratings can guide session focus). Moreover, pilot programs in U.S. healthcare systems have used AI to monitor therapy session quality (by analyzing recordings for adherence to CBT techniques) and found it can enhance training and supervision. While concerns like privacy remain, the overall attitude is that AI can act as a “second pair of eyes,” improving treatment personalization and outcomes. As one concrete example, the Department of Veterans Affairs has tested an AI note-taking tool that automatically flags PTSD symptoms from therapy sessions, saving clinicians time and ensuring important symptoms are not overlooked. Such real-world applications underscore the potential of therapist assistance tools to augment human care with data-driven support.
AI assists therapists by aggregating and analyzing data from session notes, mood tracking, and user feedback. This analysis helps therapists gain deeper insights into their clients' conditions, monitor treatment effectiveness, and adjust therapeutic approaches as needed, enhancing the overall quality of care.