1. Enhanced Data Analytics
Artificial intelligence is elevating data analytics in IoT by handling the massive volumes of sensor data that devices generate. Machine learning algorithms can sift through streams of unstructured IoT data to find patterns and correlations that humans might miss. This leads to smarter insights in sectors like manufacturing (where AI analyzes production line data to improve yield) and smart cities (where traffic and environmental sensors are analyzed for real-time optimizations). By enhancing data analytics, AI enables predictive modeling – for example, forecasting equipment performance or consumer behavior based on IoT inputs. Overall, AI-driven analytics turn raw IoT data into actionable intelligence faster and more accurately, helping organizations make informed decisions and optimize operations.
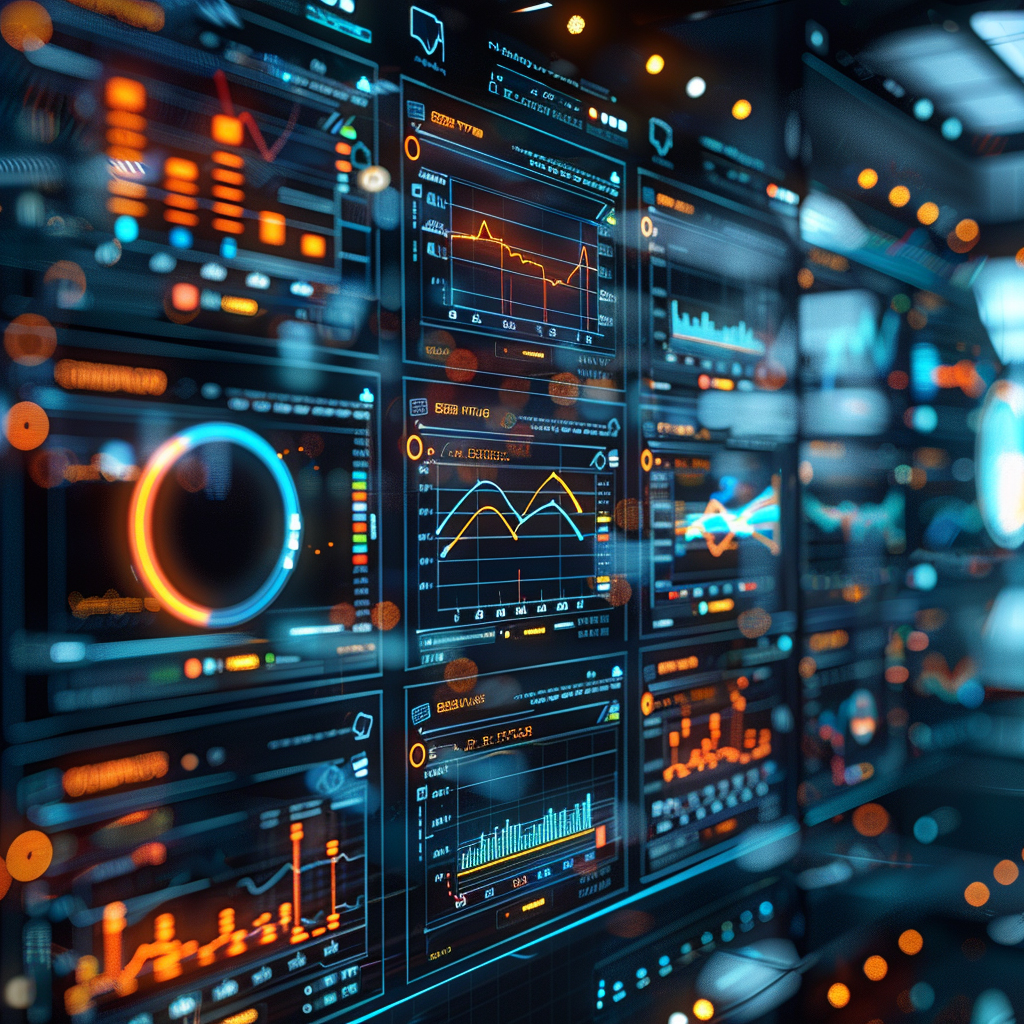
The impact of AI on IoT data analysis is evident in market growth. The global market for AI-enabled IoT analytics was valued at roughly $77.2 billion in 2023, and it is projected to grow at over 20% annually. North America leads this trend, accounting for about 40% of that market as of 2023. This rapid growth reflects how widely companies are adopting AI for IoT data processing. In practice, more organizations are investing in AI-powered analytics tools to cope with IoT data – contributing to a market expected to reach several hundred billion dollars over the next decade. The strong North American share underscores that U.S. industries are at the forefront of leveraging AI to derive insights from IoT data.
2. Predictive Maintenance
AI-powered predictive maintenance is transforming how industries maintain equipment by using IoT sensor data to foresee problems before they cause downtime. In manufacturing and utilities, IoT devices like vibration or temperature sensors continuously monitor machinery health. Machine learning models analyze this data to detect early warning signs of wear or anomalies. This allows companies to service or replace components just-in-time, avoiding unexpected breakdowns. For example, in the transportation sector, AI can predict when a fleet vehicle’s engine is likely to fail and schedule maintenance proactively. The result is that AI-enhanced IoT systems minimize unplanned outages, extend the lifespan of assets, and reduce maintenance costs by fixing issues when they are minor rather than after a major failure.
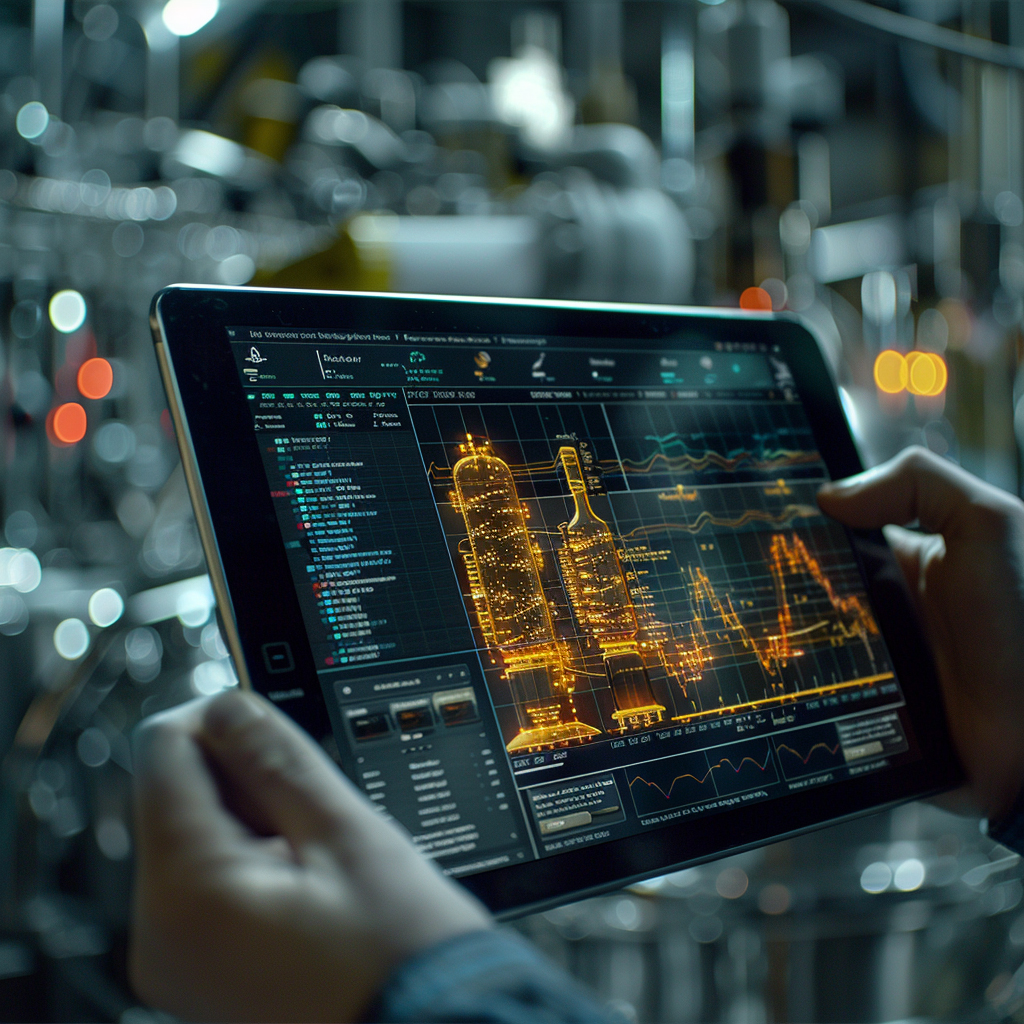
The benefits of AI-driven predictive maintenance are reflected in overwhelmingly positive outcomes for adopters. According to recent industry research, 95% of organizations implementing IoT-based predictive maintenance reported a positive return on investment, and 27% achieved payback in under one year. These systems can sharply cut downtime – studies by McKinsey have found machine downtime is typically reduced by 30–50% with predictive maintenance practices. In one analysis of heavy industries, the median cost of unplanned downtime was about $125,000 per hour, so catching failures in advance has enormous financial rewards. The high ROI and fast payback reported underscore that AI-enhanced maintenance is not just technically effective but also financially compelling for companies.
3. Improved Security
Security is a critical concern in IoT, and AI is bolstering defenses for the billions of connected devices now online. Traditional security tools struggle to monitor IoT networks due to the sheer scale and diversity of devices, from smart home cameras to industrial sensors. AI addresses this by learning the normal pattern of IoT device behavior and network traffic, then flagging anomalies in real time. For instance, an AI system can detect if a normally quiet sensor suddenly starts transmitting large amounts of data – a sign it might be compromised – and trigger an alert or autonomous response. In enterprise settings, AI-powered IoT security platforms can rapidly identify malware or intrusion attempts targeting IoT endpoints (like thermostats or medical devices) that would evade manual oversight. By continuously adapting to new threats, AI enhances IoT security with faster threat detection, automated responses (such as isolating a breached device), and smarter authentication methods, thereby reducing the risk of cyberattacks across smart homes, healthcare, industrial control systems, and other IoT-rich environments.
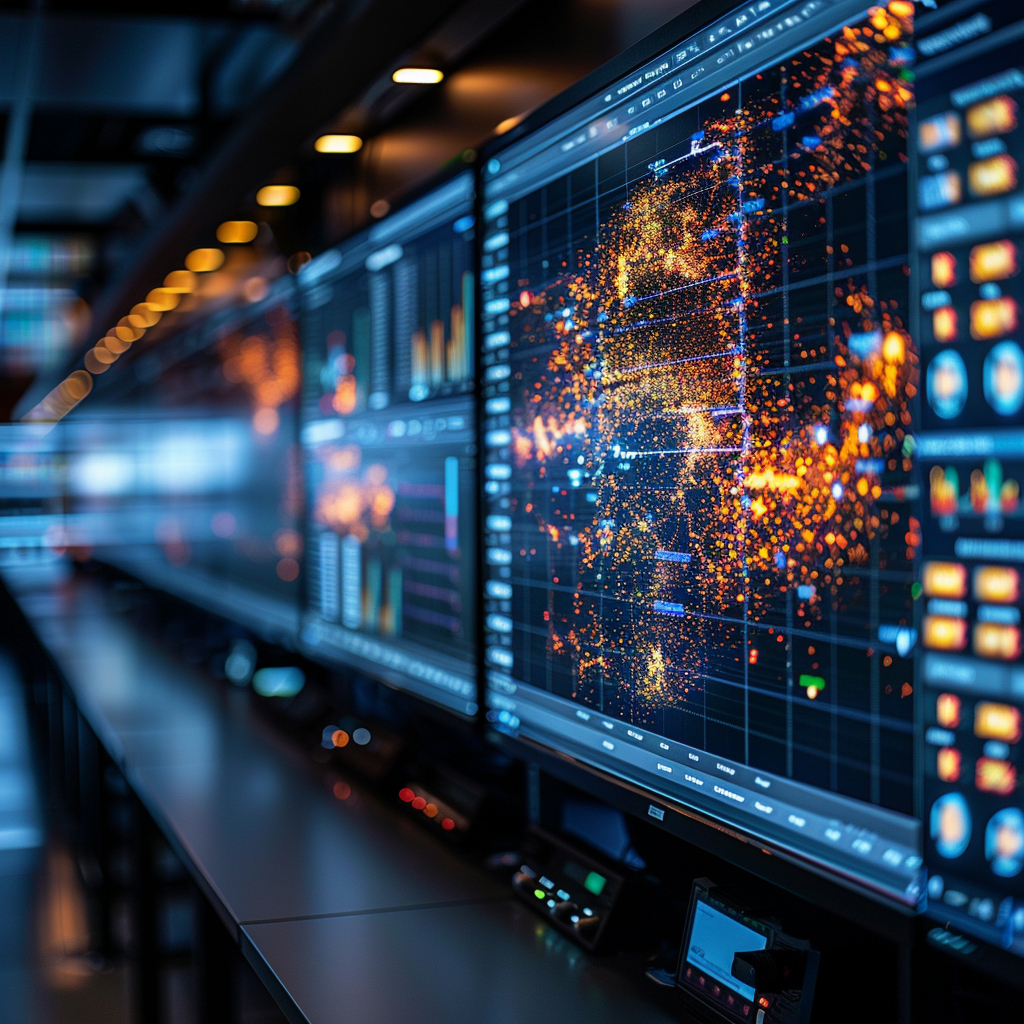
The importance of AI in IoT security is highlighted by the scale and cost of IoT-related breaches. Research in 2024 found that the average cost of a successful attack on an IoT device exceeds $330,000, once factors like remediation, downtime, and fines are included. Moreover, over a third of companies that suffered a breach via IoT devices faced cumulative costs of $5–10 million, significantly higher than the costs of breaches not involving IoT endpoints. These statistics underline how attacks on unsecured IoT devices can amplify damage compared to typical cyber incidents. Consequently, organizations are increasingly deploying AI-driven security analytics that monitor IoT networks for suspicious patterns 24/7. For example, AI systems can analyze nearly 70 IoT attack attempts per organization per week in Europe – the region with the highest activity – and help filter out false alarms from true threats. By leveraging AI to manage this volume of security data, companies aim to mitigate the outsized financial risks associated with IoT breaches.
4. Energy Efficiency
AI is helping IoT networks become far more energy-efficient by intelligently managing energy consumption across devices and systems. In smart buildings, AI algorithms process data from IoT sensors (temperature, occupancy, lighting) to adjust HVAC systems and lighting in real time, minimizing waste – for example, by dimming lights and reducing cooling in unoccupied areas. Industrial facilities use AI-driven IoT platforms to optimize machine operations and schedule energy-intensive tasks during off-peak hours. Utilities deploy AI with IoT on the electric grid to balance load and integrate renewable energy smoothly, improving overall grid efficiency. A representative sector is commercial real estate: many modern office towers use AI-based building management systems that learn patterns (like when people arrive or leave) and automatically tune energy settings, often cutting power usage substantially. By using predictive control (such as anticipating a temperature rise and pre-cooling a building), AI enables IoT devices to run more efficiently than simple rule-based control, leading to significant energy savings and a smaller carbon footprint.
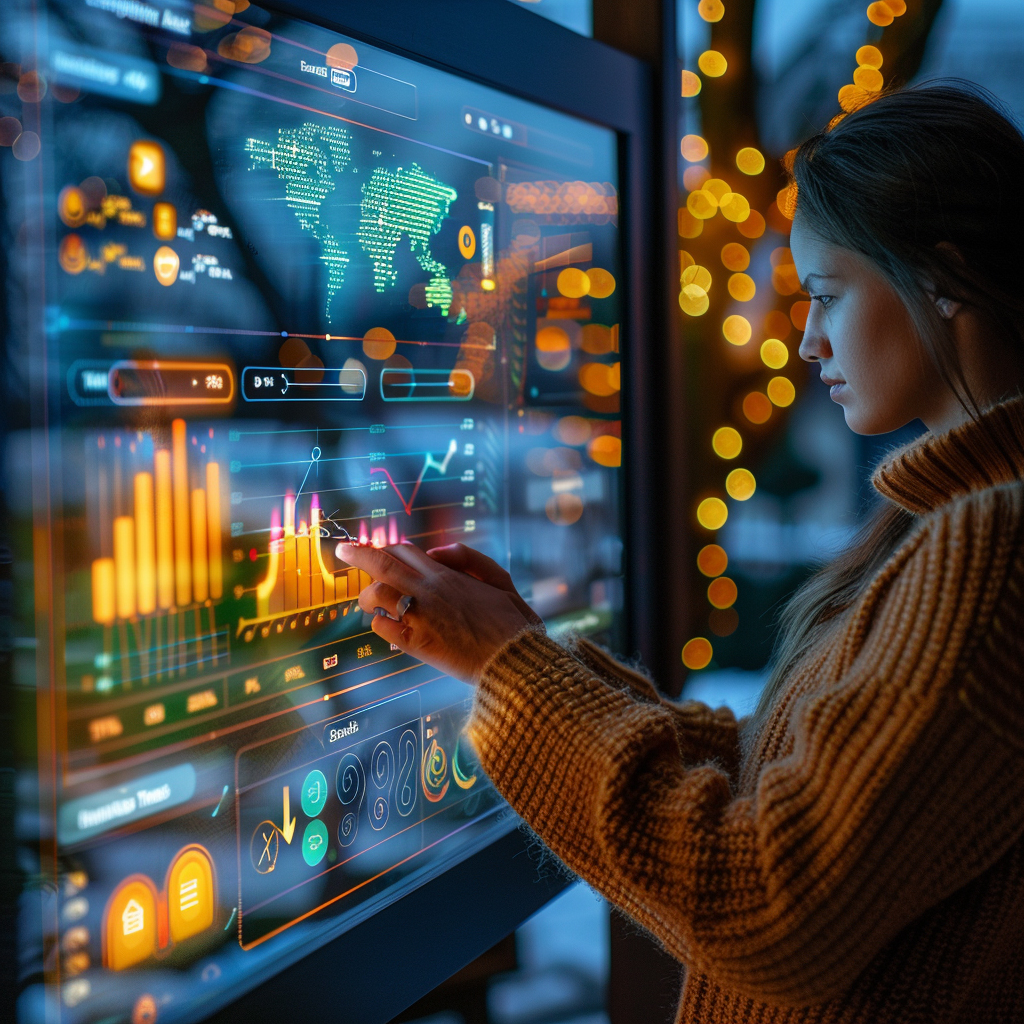
The introduction of AI into IoT energy management has yielded remarkable efficiency gains. In advanced implementations, companies have documented energy consumption reductions on the order of 40% after integrating AI with IoT building systems. In fact, a Deloitte analysis reports that organizations which deployed IoT-enabled sensors and AI-driven controls saw energy savings of up to 70% over three years in their facilities. Such dramatic improvements, while at the high end, illustrate the potential of AI-optimized IoT systems (for instance, in smart offices and factories). Even more typical results are impressive: academic reviews of smart building projects find AI techniques can improve energy efficiency by 10% to 40% on average. These statistics are backed by real-world cases like Google’s data centers, where AI-based IoT controls cut cooling energy use by about 40%, and by numerous pilot programs in which smart grids and AI have trimmed peak demand by 10–15%. Overall, the data shows that combining AI with IoT can deliver double-digit percentage energy savings, validating energy efficiency as a key strength of AI-enhanced IoT deployments.
5. Personalization
AI is enabling IoT devices to deliver highly personalized experiences by learning from user behavior and preferences. In the consumer realm, this means smart home devices can adapt to individual lifestyles – for example, a smart thermostat learns a household’s schedule and comfort preferences, then adjusts heating and cooling automatically. Wearable IoT health devices use AI to analyze a person’s unique fitness or medical data and provide tailored coaching or alerts (such as personalized exercise goals or medication reminders). Even in retail, IoT sensors combined with AI can personalize the in-store experience, like smart shelves recommending products based on a customer’s past behavior. Personalization is also key in connected cars: AI in automotive IoT remembers a driver’s seat position, music choices, and driving style, customizing the vehicle settings accordingly. By leveraging machine learning on the data IoT devices collect about their users, companies across sectors – from healthcare to entertainment – create more user-centric services. This individualized approach enhances user satisfaction and engagement, as devices feel more intuitive and responsive to each person’s needs.
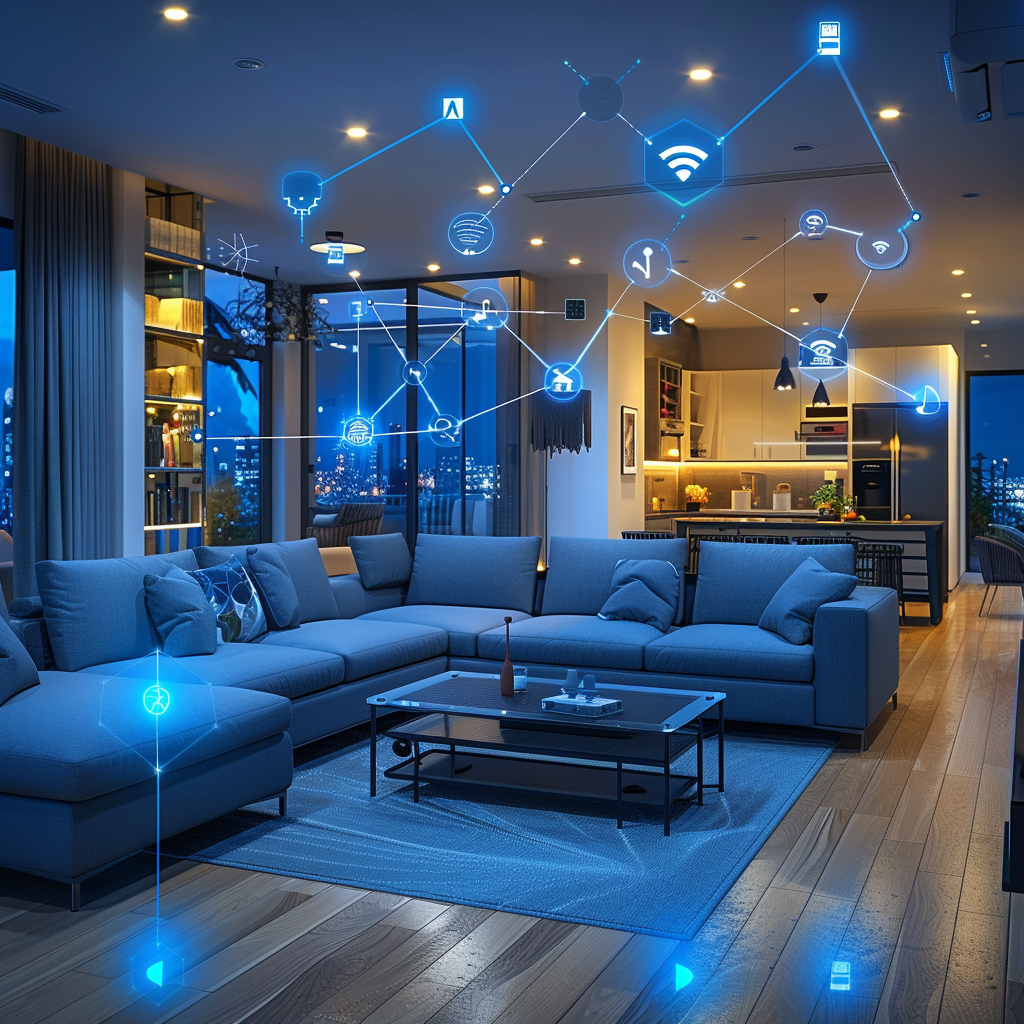
Personalized IoT devices are having a measurable impact on user engagement and outcomes, especially in health and wellness. In healthcare, for instance, a 2023 study reported that 75% of healthcare providers believe wearable IoT devices (like smartwatches with health apps) enhance patient engagement, and 60% have observed improved patient health outcomes among those using such personalized monitoring tools. Consumers similarly report greater satisfaction when IoT gadgets adapt to them: user surveys find that fitness tracker wearers increased their activity levels by up to 50% once the device personalized their goals and feedback. Additionally, around 80% of individuals using AI-driven health wearables feel these devices give valuable insights into their personal health, prompting preventative actions. In the smart home domain, personalization is a selling point as well – a Deloitte survey found 77% of smart home adopters say the technology (often powered by AI learning their routines) improves their quality of life. These statistics underscore that personalization through AI is not just a novelty but a functional benefit of IoT, leading to more engaged users and tangible improvements in their daily lives.
6. Enhanced Automation
By combining AI with IoT, businesses are achieving levels of automation that go far beyond what was previously possible with fixed scripts or human monitoring. IoT devices provide real-time data from the physical world, and AI algorithms can instantly decide on actions – effectively creating self-regulating systems. In manufacturing, this manifests as smart factories where assembly lines autonomously adjust their operating parameters through AI feedback loops (often called Industry 4.0). Robots on the factory floor coordinate via IoT sensors and AI vision systems to handle materials with minimal human intervention. In warehousing and logistics, AI-driven IoT networks guide fleets of autonomous mobile robots and drones for picking and inventory management, increasing efficiency. Even agriculture benefits: automated irrigation systems use AI to interpret soil sensor data and weather forecasts, then water crops optimally without human input. AI enhances automation by handling complex decision-making – for example, an AI can decide to shut off a machine if multiple sensor readings indicate an impending failure – which traditional rule-based automation might miss. The result is IoT systems that run with greater autonomy, speed, and precision, delivering higher productivity and consistency in sectors from manufacturing to supply chain to smart cities.
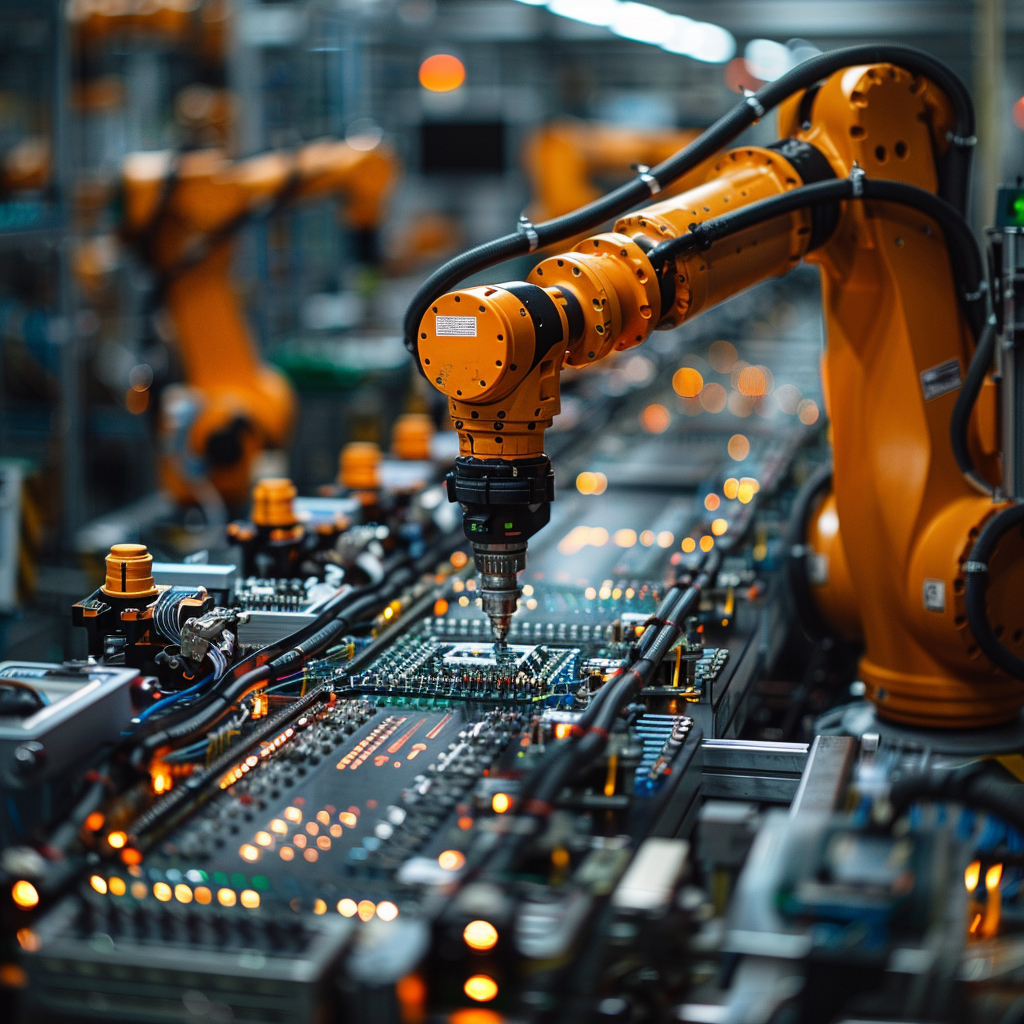
The adoption of AI-enhanced automation in industry has become nearly ubiquitous. A recent survey of manufacturers found that 95% are now using or actively evaluating “smart manufacturing” technologies that integrate IoT and AI to automate processes, up from 83% just one year prior. This surge reflects a consensus that AI+IoT automation is critical for staying competitive. Notably, companies aren’t using automation to cut workers, but to augment them – about 94% of manufacturers in the survey expected to maintain or grow their workforce as they implement smart factory systems, suggesting AI is handling routine tasks while humans focus on higher-level work. Outside of manufacturing, the trend is similar: in utilities and energy, more than 50% of new projects involve AI-based IoT automation for tasks like grid balancing and outage response. The manufacturing sector leads in AI-in-IoT investment – accounting for roughly 24% of the AI+IoT market by one estimate – indicating how central enhanced automation has become. Overall, the data shows that virtually all major industrial players are embracing IoT-driven automation with AI, highlighting a widespread shift toward autonomous operations.
7. Real-time Decision Making
AI is empowering IoT systems to make decisions in real time, which is crucial for time-sensitive applications. In the past, IoT devices might simply collect data to be analyzed later in a cloud server or by humans, causing delays. Now, with AI algorithms running either on the device (edge AI) or nearby, IoT setups can interpret data and act on it in milliseconds. This enhancement is evident in scenarios like autonomous vehicles – a self-driving car’s IoT sensors (cameras, LIDAR, radar) feed into onboard AI that instantly recognizes hazards and makes steering or braking decisions on the spot. Similarly, in smart factories, if an IoT sensor detects a dangerous pressure spike in a boiler, an AI system can trigger an immediate shutdown or alert, preventing an accident. Edge computing often plays a role here: placing AI processing close to IoT devices (such as in a factory or cell tower) cuts the latency of sending data to the cloud, enabling near-instant responses. Real-time AI decisions are also used in healthcare IoT – think of wearable heart monitors with AI that can detect an arrhythmia and promptly alert doctors or call an ambulance. By integrating AI, IoT networks become far more responsive, handling events on the fly without waiting for human input, which is essential for safety and efficiency in dynamic environments.
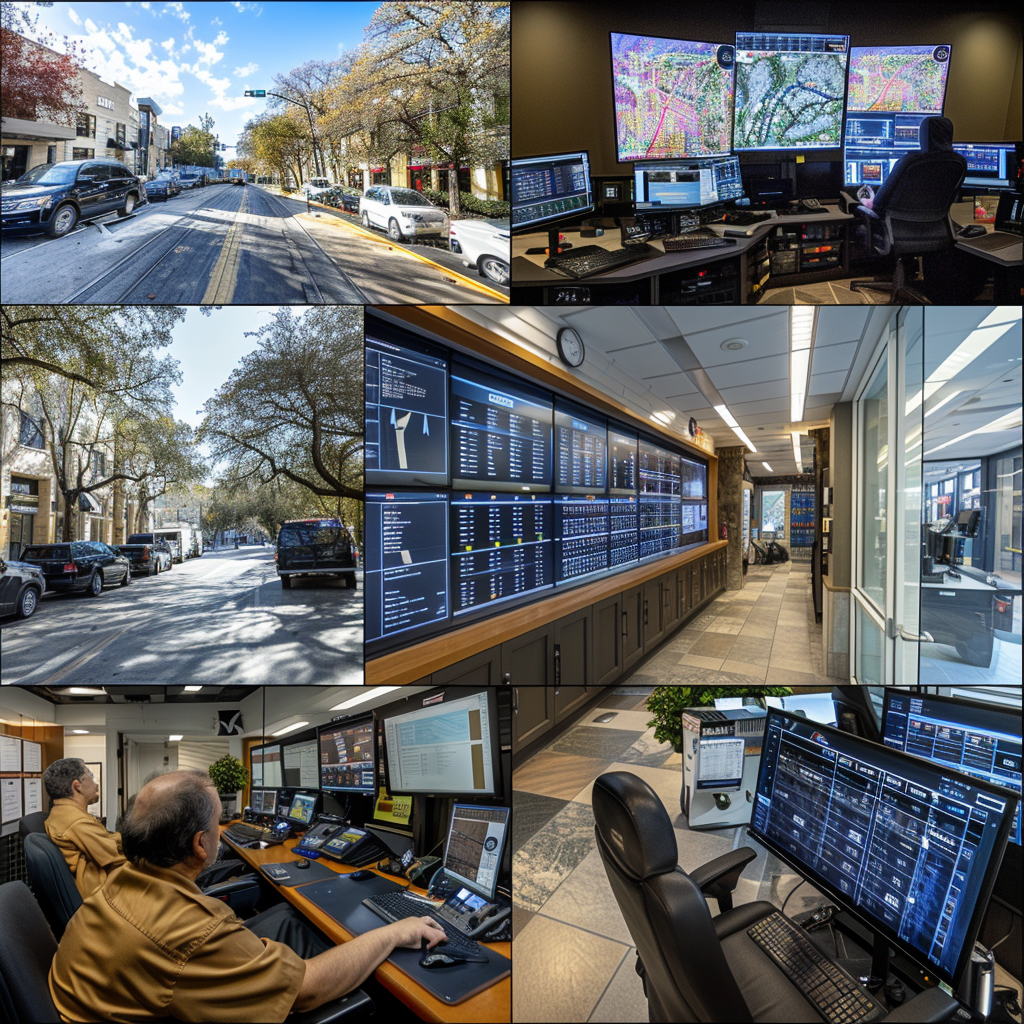
Industry forecasts underscore the growing emphasis on real-time, on-site data processing in IoT. Gartner projects that by 2025, 75% of enterprise-generated data will be created and processed outside of traditional data centers or cloud – i.e. at the network edge, closer to where data originates. This is a sharp rise from only 25% in 2018, reflecting how companies are redesigning systems to enable immediate decision-making on IoT data. The proliferation of 5G networks and edge computing infrastructure supports this shift, as they dramatically reduce transmission delays. In practical terms, such a trend means the majority of IoT data (from factory machines, vehicles, sensors, etc.) will soon be analyzed by AI in real time at the source. We already see this in numbers: global spending on edge computing (often paired with AI analytics for IoT) is expected to reach $261 billion by 2025, driven largely by demand for real-time automation and analytics. By pushing intelligence to the edge, organizations achieve decision latencies down to milliseconds – for example, an AI-powered surveillance camera can now detect an intruder and send an alert instantaneously, rather than after video is reviewed in a central cloud. The data trends make it clear that real-time AI decision-making is becoming a standard feature of IoT deployments moving forward.
8. Seamless Integration
AI is helping to knit together the diverse components of IoT ecosystems, creating more seamless integration of devices, platforms, and data. One of the challenges with IoT has been that different devices (from different manufacturers or using different protocols) don’t always communicate well or share data readily. AI can mitigate this by serving as an intelligent intermediary – for instance, using machine learning to normalize data from disparate sensors so that it can be analyzed in a unified way. In enterprise settings, AI-powered integration hubs can ingest data from various IoT sources (machinery sensors, security cameras, environmental monitors, etc.) and automatically map relationships or dependencies between them, which traditionally required manual configuration. This means an AI might learn that a spike in vibration sensor data and a fluctuation in power draw, though from two separate systems, together indicate a single event like a machine fault. Additionally, AI can manage IoT device interoperability in smart homes: modern smart assistants use AI to discover new IoT devices and configure them with minimal user input, effectively plug-and-play. By simplifying compatibility issues and performing real-time translation between protocols, AI allows previously siloed IoT devices to operate as part of a cohesive network. This seamless integration is essential for large-scale IoT deployments such as smart factories or smart cities, where thousands of heterogeneous devices must work in concert.
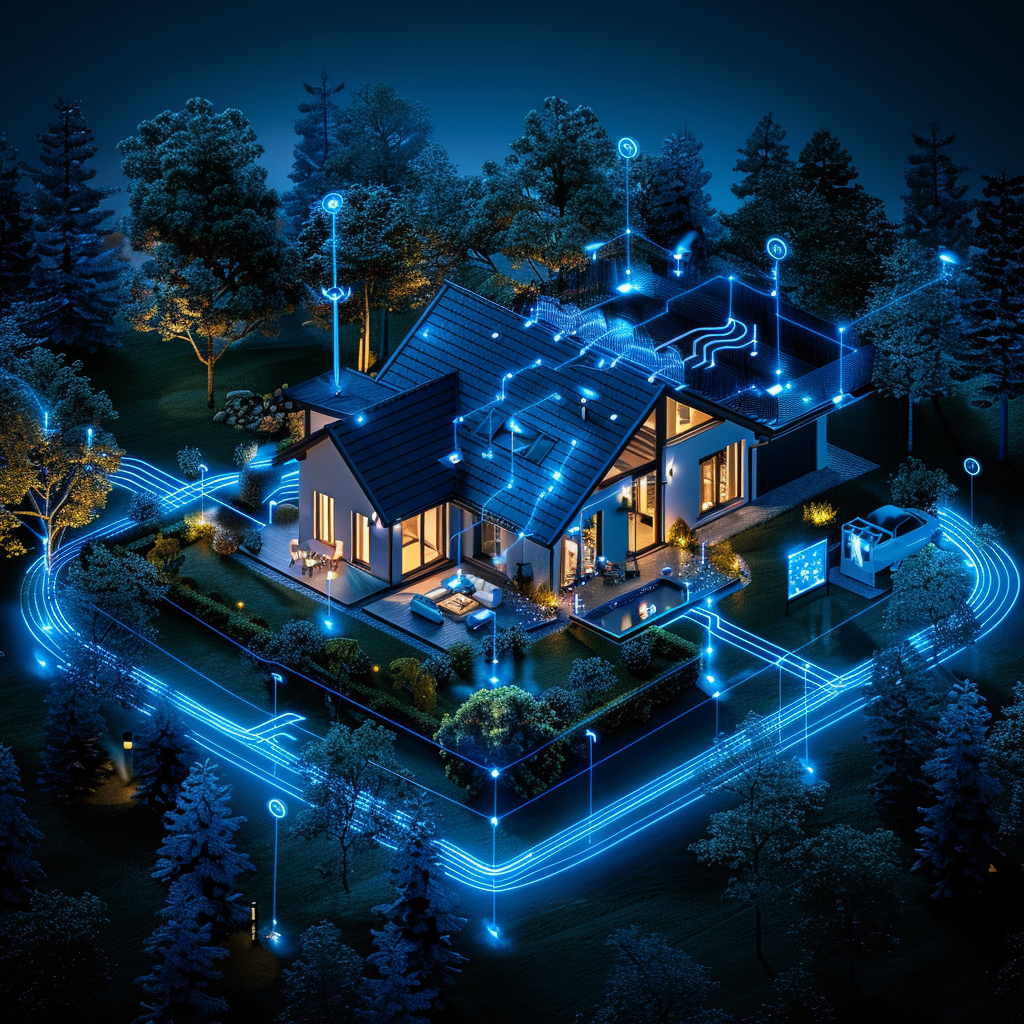
Despite improvements, integrating IoT systems remains a major hurdle – one that AI aims to overcome. A 2024 survey of industrial IoT projects revealed that as many as 80% of IoT projects fail to fully scale beyond prototypes due to the complexity of integration and inability to get different systems working together smoothly. This startling failure rate shows how difficult it can be to connect myriad devices, data sources, and legacy systems in practice. It also explains why organizations are investing in AI-driven integration solutions. Analysts note that companies struggle with siloed IoT deployments and data – in one IDC survey, 70% of firms said they still find it challenging to manage and integrate IoT data across the enterprise. AI can help by automating much of this integration work: for example, intelligent IoT platforms use machine learning to auto-detect device types and configure data pipelines, reducing manual coding. The need for seamless integration is further underscored by the fact that 76% of organizations plan to increase IoT investments (often in tandem with AI) but remain wary of integration difficulties. With integration issues causing such high failure rates historically, the use of AI to intelligently bridge gaps between IoT devices and IT systems is becoming critical to ensure IoT deployments succeed and scale company-wide.
9. Improved User Interfaces
AI is significantly improving the way users interact with IoT devices by enabling more natural and user-friendly interfaces. Rather than relying on complex control panels or smartphone apps for every device, users can increasingly use voice commands, chatbots, or gestures to control their smart environments, thanks to AI. Voice assistants like Amazon’s Alexa, Google Assistant, or Apple’s Siri are prime examples: they use speech recognition and natural language processing (AI techniques) to let users verbally instruct IoT devices (turning lights on/off, querying sensor information, setting thermostats, etc.). This makes smart homes and cars much more accessible – even those not tech-savvy can simply “talk” to their devices. In industrial or professional contexts, augmented reality (AR) is an emerging AI-driven interface for IoT: technicians wearing AR glasses can see overlays of sensor data or receive AI-guided instructions while looking at a machine, rather than consulting manuals. AI also enables personalization of UIs – a smart fridge’s display might learn which information (weather, recipes, etc.) the user checks most and prioritize that on its screen. By handling complex input recognition (voice, vision) and predictive personalization, AI-driven interfaces reduce the friction between humans and the IoT devices around them. This leads to IoT systems that are easier to use and more engaging, ultimately driving higher adoption and user satisfaction.
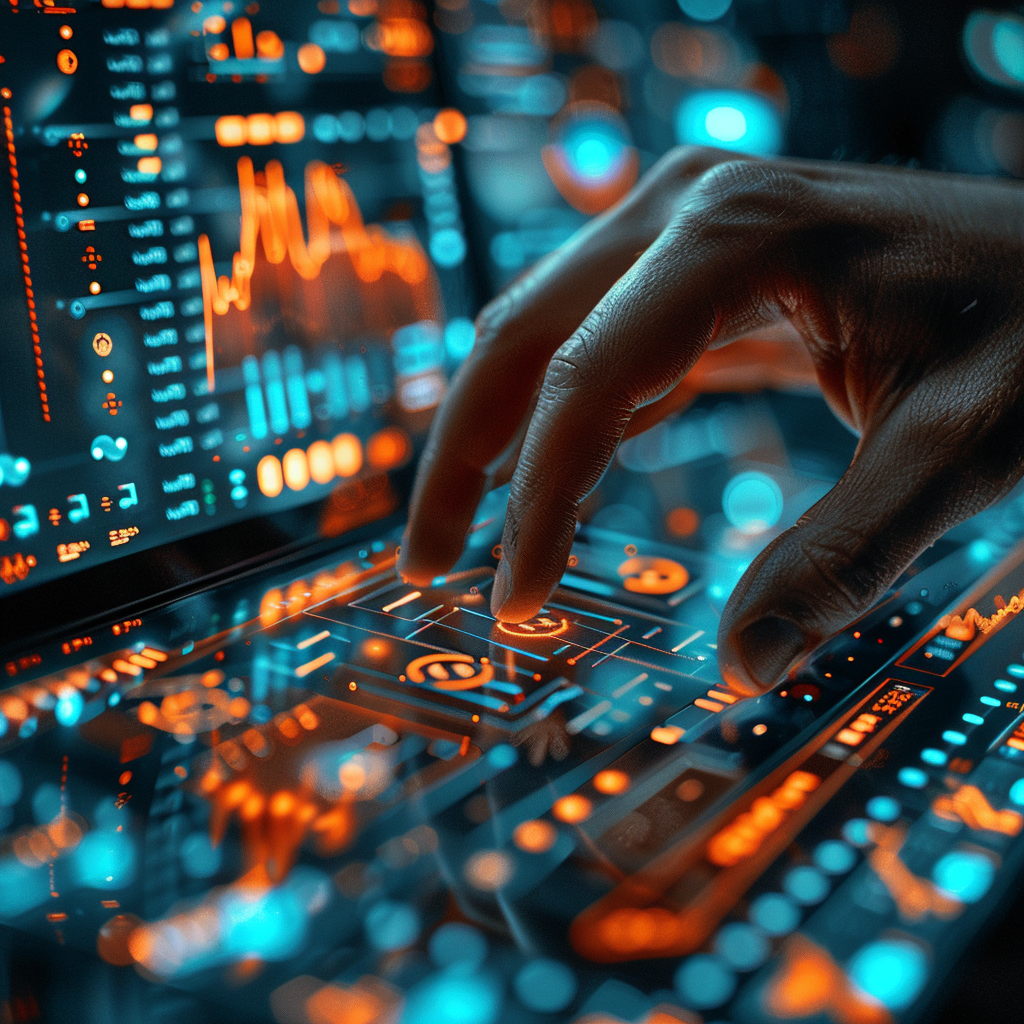
The rise of AI-driven voice interfaces in IoT is evident from their widespread adoption. As of 2023, an estimated 100 million Americans (about 35% of the U.S. population aged 12 and up) own a smart speaker device (such as an Amazon Echo or Google Nest), which they can use to interact with IoT services via voice commands. In households with these devices, studies show that a majority of users give voice commands daily – everything from asking for the news to controlling smart lights. Overall, about 45% of U.S. households now have at least one “smart home” device (like smart thermostats, cameras, or speakers) that often features AI-based interfaces. This penetration is projected to keep rising, with forecasts expecting over half of households to be equipped in the next couple of years. User behavior also reflects the convenience of AI UIs: nearly 72% of Americans use digital voice assistants in some form, whether on speakers or phones, to interact with their apps and smart devices. In industrial settings, while harder to quantify, there is a growing use of voice-activated controls for machinery and AI chatbots for facility management. These trends demonstrate that AI-enhanced user interfaces – particularly voice – have become a mainstream way people engage with IoT technology, lowering barriers to entry and making the experience more intuitive.
10. Fault Detection
AI improves the ability of IoT systems to detect faults and anomalies in equipment or infrastructure at an early stage, preventing minor issues from escalating into major failures. In practice, IoT sensors monitor various parameters (vibration, temperature, pressure, voltage, etc.), and AI models learn what “normal” looks like for those signals. When something deviates – say a machine vibrates more than usual or a pipeline’s pressure fluctuates abnormally – the AI can immediately flag it as a potential fault. This is crucial across sectors: for example, in power utilities, AI-enabled IoT sensors on the grid can pinpoint a failing transformer or line issue, allowing for rapid repairs before an outage spreads. In manufacturing, if an assembly robot or motor shows signs of mechanical stress, AI algorithms can detect the anomaly and schedule maintenance or shutdown to avoid a breakdown and production halt. Fault detection extends to building management as well: IoT-based systems watch HVAC performance, and AI can alert facility managers if an air handler or compressor is starting to malfunction (often before occupants even feel a change). By catching issues early, AI-driven fault detection not only averts downtime but also enhances safety (preventing accidents), and optimizes maintenance (fixing things exactly when needed). It essentially gives organizations a kind of “digital sixth sense” about the health of their connected devices and infrastructure.
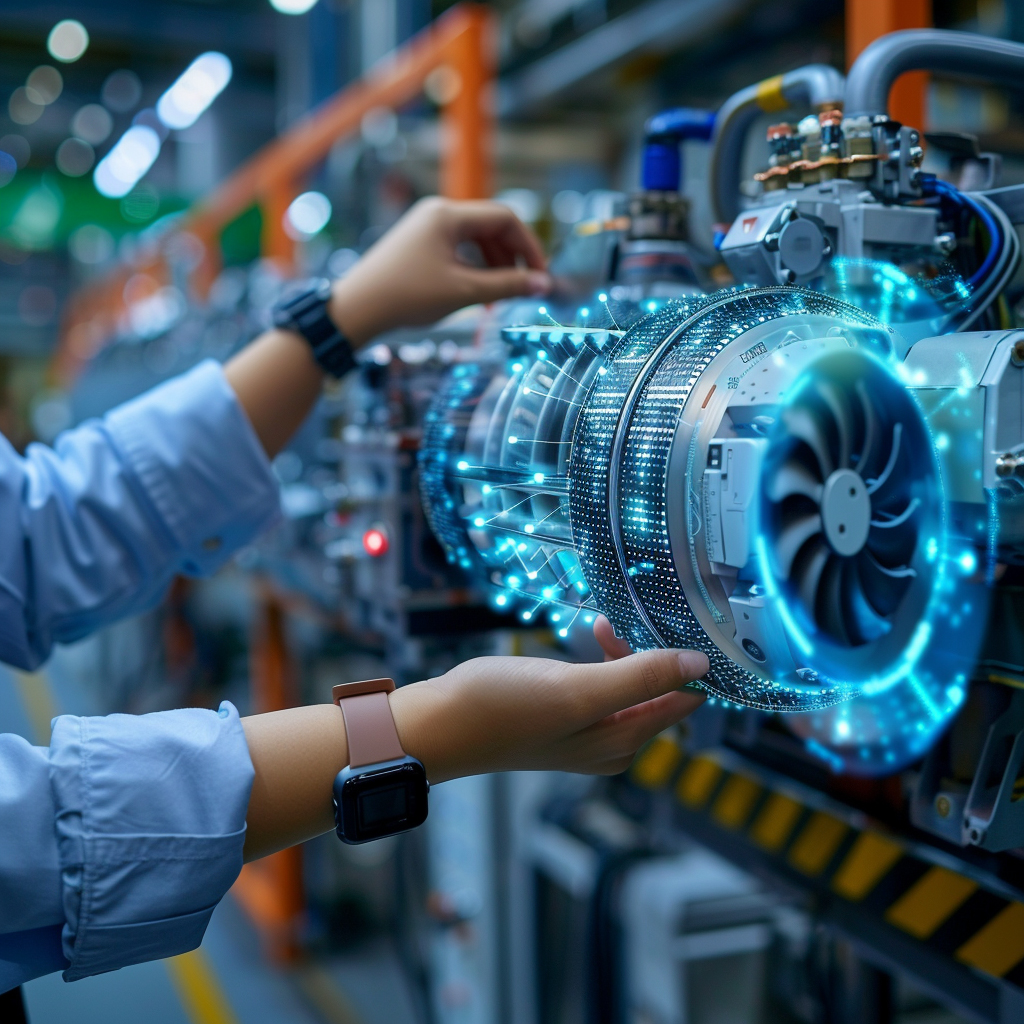
Early fault detection through AI is proving its value by significantly reducing waste and downtime. Studies indicate that IoT systems equipped with AI diagnostics can reduce energy waste due to equipment faults by roughly 15% on average. This is because failing equipment often draws extra power or operates inefficiently; catching those issues sooner avoids weeks of energy being wasted on an underperforming machine. In industrial settings, those energy losses from undetected faults can account for a substantial slice of usage (on the order of one-sixth of total consumption), so eliminating them yields major savings. Furthermore, various field results show that AI-based fault detection lowers unplanned downtime notably – for instance, some manufacturing firms have reported up to 70% fewer unexpected breakdowns after implementing predictive fault analytics, as minor anomalies are fixed during scheduled maintenance instead. In power grids, deploying smart fault-detection IoT sensors has led to utilities isolating and repairing outages 50% faster than before, minimizing customer disruption. These figures demonstrate that AI-driven fault detection is not just theoretically useful but is tangibly improving operational reliability and efficiency, saving companies significant costs and improving service uptime by identifying problems that would previously have gone unnoticed until failure.