1. Demand Forecasting
AI-driven demand forecasting allows businesses to predict future inventory needs with much greater precision. Machine learning models can analyze historical sales data, market trends, seasonal patterns, and even external factors (like economic indicators or weather) to detect complex demand patterns that traditional methods might miss. These AI systems continuously update forecasts in real time as new data comes in, enabling companies to respond quickly to changing customer needs. The result is more accurate demand predictions that help prevent stockouts (running out of products) and avoid overstocking, thus optimizing inventory levels. By aligning inventory with actual demand, companies reduce excess holding costs and improve customer satisfaction through better product availability.
AI algorithms analyze historical sales data and market trends to predict future product demand, enabling more accurate stock planning and reducing overstock or stockouts.
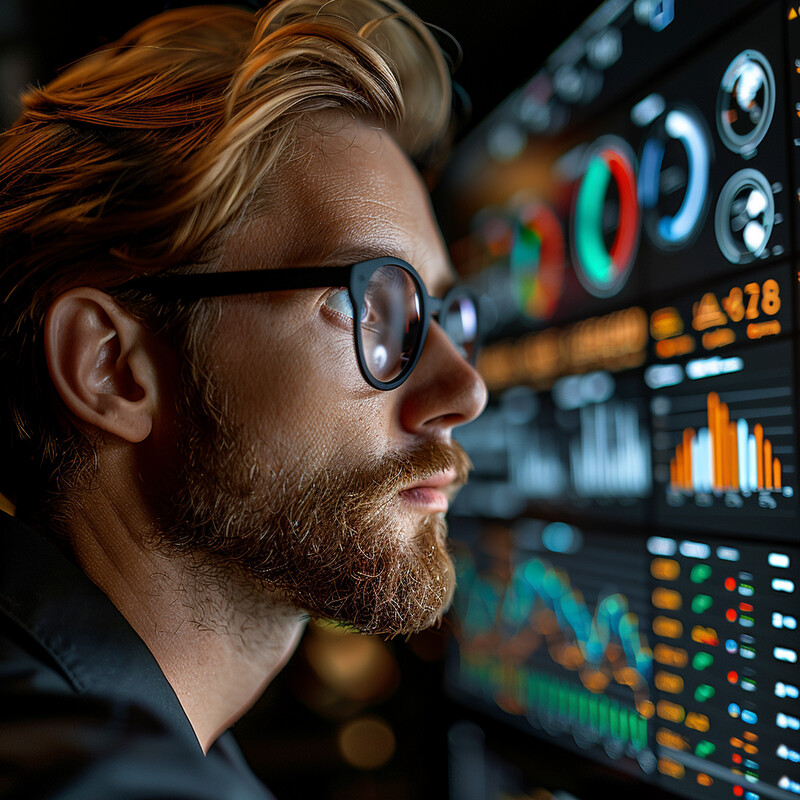
Research in 2024 highlighted that companies using AI in their forecasting processes have achieved up to a 30% improvement in forecast accuracy compared to conventional methods. For example, AI-based models can incorporate diverse data (like online search trends or social media sentiment) and adjust forecasts on the fly, something not feasible with manual forecasting. This boost in accuracy directly translates into leaner inventory – McKinsey notes that AI-driven forecasting can cut inventory levels by 20–30% by eliminating unnecessary safety stock. Retailers that adopted AI for demand planning reported not only fewer stockouts but also lower storage and spoilage costs. Overall, AI-enhanced demand forecasting leads to a more efficient supply chain, where inventory is kept at optimal levels to meet demand without waste.
AI leverages historical sales data, market trends, consumer behavior, and external factors like weather or economic conditions to accurately predict future product demand. This sophisticated analysis helps businesses optimize their inventory levels, ensuring they have enough stock to meet demand without overstocking, which can tie up capital and increase storage costs.
2. Automated Reordering
AI enables automated reordering systems that keep inventory replenished with minimal human intervention. These systems use real-time inventory data and predictive algorithms to decide when to reorder stock and how much to order. By continuously tracking sales rates, lead times from suppliers, and current stock levels, an AI-driven system can trigger purchase orders the moment inventory falls below optimized thresholds. This automation eliminates the delays and errors of manual reordering – no more forgetting to reorder a hot-selling item or misjudging the amount needed. Moreover, AI can dynamically adjust reorder points based on trends (like ramping up orders ahead of a predicted demand surge or pausing orders if demand softens). The benefits are significant: companies maintain optimal stock levels with much less effort, leading to fewer stockouts (lost sales due to empty shelves) and lower holding costs from over-ordering. Employees are freed from routine inventory checks and can focus on more strategic tasks since the AI handles the routine restock decisions.
AI systems automate the reordering process by monitoring stock levels in real-time and automatically placing orders when stock falls below predetermined thresholds.
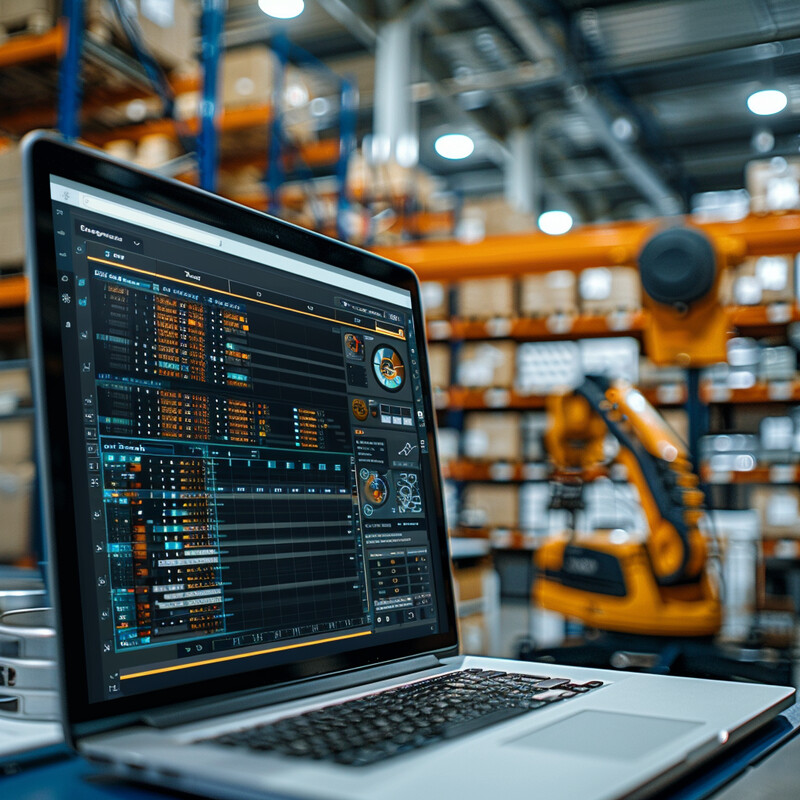
According to industry analysis, businesses that implement AI-powered automated reordering see dramatic improvements in inventory reliability. One report found that such companies reduced stockouts by over 40% on average by ensuring products are reordered at just the right time. In practice, this means customers are far less likely to encounter an “out of stock” message, as the AI has already replenished the item before it ran out. These systems also keep inventory lean – studies show automated reordering can lead to 20%+ reductions in inventory carrying costs by avoiding excess stock. For example, an AI-based replenishment tool might notice that a certain item’s sales are faster than usual and immediately raise its reorder frequency, preventing a stockout. Conversely, if demand slows, the AI will push back orders to avoid overstock. By reacting swiftly to real-world data, AI reordering systems maintain nearly 100% product availability while minimizing waste and storage costs.
AI systems monitor inventory levels in real time and automate the reordering process. By setting predetermined thresholds for stock levels, AI can trigger automatic purchase orders when supplies run low, ensuring continuous availability of products and minimizing the risk of stockouts that could result in lost sales.
3. Warehouse Optimization
AI helps optimize warehouse operations by making them more efficient and adaptable. In a warehouse, AI can analyze workflows – such as the movement of goods and the usage of storage space – and suggest improvements that a human manager might not spot. For instance, AI algorithms can determine the optimal layout of a warehouse, deciding where to place products so that the most frequently picked items are in the easiest locations, reducing travel time for workers. Machine learning can also optimize picking routes for order fulfillment: given a set of orders, an AI system can direct workers (or robots) along the shortest path that collects all items, saving time. Additionally, AI-driven “digital twin” simulations of warehouses allow companies to test different strategies (like shifting labor schedules or rearranging aisles) in a virtual model to see what yields the best throughput. Overall, AI improves warehouse capacity usage, speeds up order processing, and cuts operational costs by streamlining every step – from receiving inventory, to storing it efficiently, to picking and packing orders for shipment.
AI optimizes warehouse layouts and manages inventory placement based on frequency of access and other factors, improving the efficiency of storage and retrieval processes.
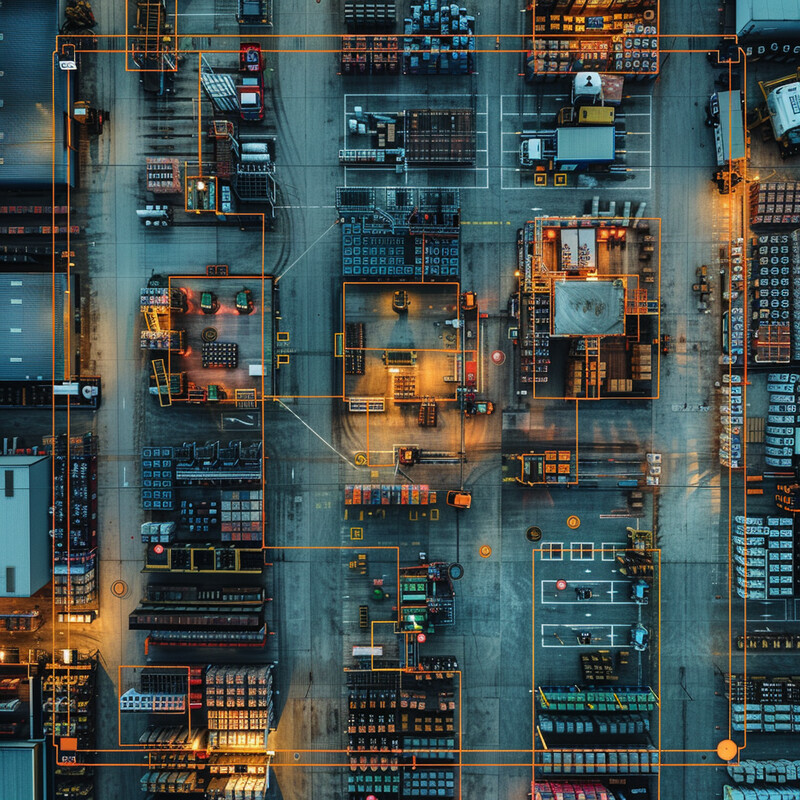
AI-driven tools have been shown to significantly boost warehouse capacity and efficiency. McKinsey reported that using AI to optimize warehouse operations can unlock 7% to 15% additional storage capacity in a network of warehouses. In one real-world example, a major logistics provider implemented an AI-powered “digital twin” of its warehouse network and achieved nearly a 10% increase in warehouse capacity without adding any new space. This AI system ran countless simulations to fine-tune how each facility used its labor and equipment hour-by-hour, revealing hidden spare capacity and more efficient ways to schedule tasks. The outcome was that the company could handle more inventory and orders in the same footprint. Similarly, AI optimization in warehouses has led to faster order fulfillment – companies report higher picking speeds and reduced worker travel distances after AI reorganizes their layout and processes. By maximizing use of space and time, AI warehouse optimization translates into lower costs and the ability to process more orders quickly.
AI optimizes warehouse operations by analyzing data on product movement and storage needs. It can suggest the most efficient placement of goods within a warehouse based on factors like sales velocity and picking logic. This optimization improves the speed and accuracy of order fulfillment processes, reducing operational costs and enhancing overall efficiency.
4. Supply Chain Management
AI enhances supply chain management by improving visibility and decision-making across the entire supply chain, from suppliers to end customers. Traditional supply chains often struggle with reacting to disruptions – for example, a sudden supplier delay or a spike in demand – but AI can help anticipate and mitigate these issues. AI systems analyze data from multiple sources (supplier production data, transit times, market trends, even news or social media signals about potential disruptions) to flag risks and opportunities early. This means a company can re-route shipments or find alternate suppliers proactively if a delay is predicted. Additionally, AI optimizes supply chain scheduling and logistics: it can determine the most efficient shipping routes, or dynamically allocate inventory to different regions based on where it predicts demand will be highest. These intelligent adjustments keep products flowing smoothly and cost-effectively. By coordinating manufacturing, inventory, and transportation plans under one AI-driven umbrella, businesses achieve a more resilient supply chain that responds in real time to changes, reduces waste (like overproduction or idle stock sitting in the wrong place), and ensures customers get products on time despite complexities.
AI enhances the entire supply chain by providing insights into supplier performance, transport conditions, and potential delays, facilitating better decision-making and planning.
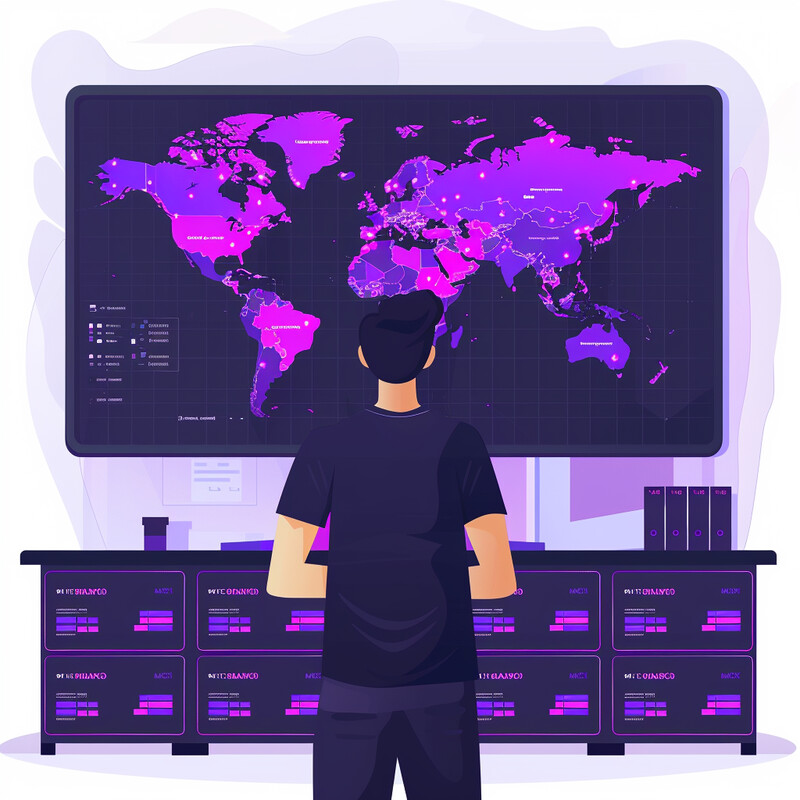
The impact of AI on supply chains is evident in performance metrics. Early adopters of AI-enabled supply chain management have significantly outperformed those using traditional methods. A 2024 report noted that companies using AI in supply chain planning reduced logistics costs by about 15%, improved inventory levels (i.e., having the right stock in the right place) by 35%, and enhanced their service levels (ability to meet customer demand on time) by 65%. These gains come from AI’s ability to better match supply with demand and to optimize transport and distribution routes. For example, by predicting demand more accurately, an AI-driven supply chain ensures that inventory is neither overstocked in low-need locations nor understocked in high-need ones, which cuts down both storage costs and lost sales. Similarly, AI can consolidate shipments or select more efficient delivery windows, trimming logistics expenses. The net result is a leaner, more agile supply chain. In fact, surveys indicate supply chain management is the business function seeing the highest cost-saving benefits from AI adoption, underlining how transformative AI has been in this domain.
AI provides comprehensive insights across the entire supply chain, from supplier performance analysis to logistics optimization. It can predict potential delays, assess risks, and suggest alternative solutions to mitigate supply chain disruptions. This proactive management helps maintain a smooth supply chain operation even in the face of unforeseen challenges.
5. Robotics in Warehousing
AI-powered robotics are revolutionizing warehousing by taking on tasks that used to require intensive manual labor. In modern warehouses, autonomous robots (guided by AI software) move and sort inventory. For example, robotic mobile units zip around aisles to pick items for orders or to replenish stock, following optimal routes decided by algorithms. These robots can work 24/7 without fatigue, dramatically increasing the speed of operations – orders can be picked and packed much faster, and with fewer errors, compared to a manual process. AI vision systems also enable robots to identify items and navigate safely, even in dynamic warehouse environments alongside human workers. Furthermore, robotics helps address labor shortages in warehouses and reduces the risk of injuries from repetitive or heavy lifting tasks. By deploying AI-driven robots for routine hauling, lifting, and sorting, warehouses become safer and more efficient. This automation scales up easily: if order volume spikes, companies can just deploy more robots or run them longer, maintaining smooth operations. Overall, AI and robotics together create highly automated “smart warehouses” with faster throughput, lower operating costs, and more reliability than traditional warehouses.
AI-driven robots automate physical tasks such as picking, packing, and sorting, increasing speed and reducing human error in warehouse operations.
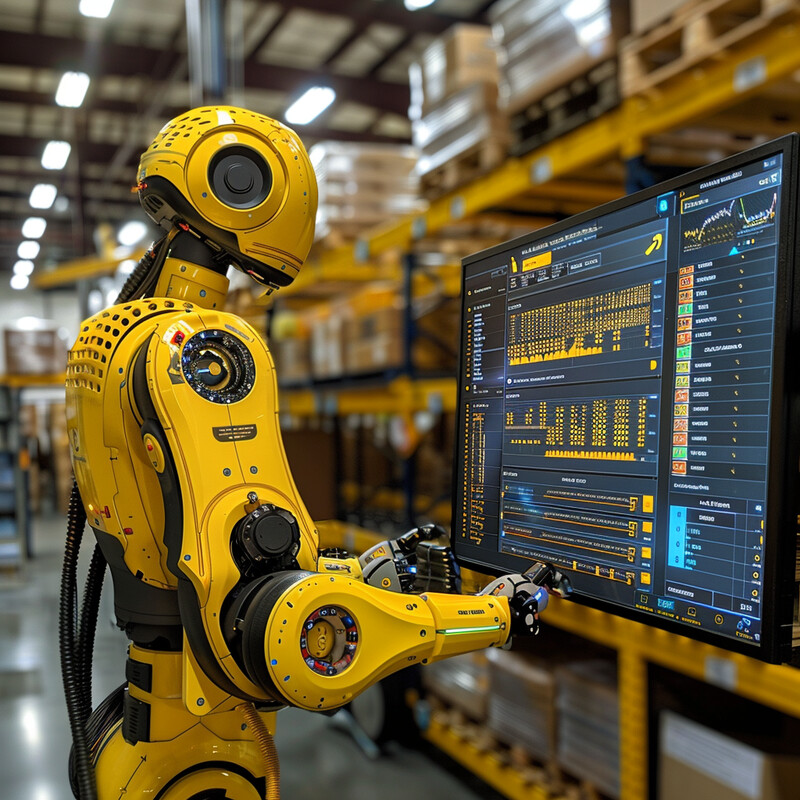
The adoption of warehouse robotics has grown explosively due to these efficiency gains. As of 2023, Amazon (a pioneer in warehouse robotics) had a fleet of over 750,000 robots working in its fulfillment centers, illustrating how central AI-guided robots have become in large-scale operations. Industry-wide, studies show that warehouse automation technology (which includes robots) can reduce labor costs by up to 60% while boosting labor productivity by about 30%. This means tasks that once took, say, 10 workers might be handled by a few robots supervised by a couple of technicians, at a fraction of the long-term cost. Additionally, robots perform tasks with near-perfect consistency – some AI inspection robots catch over 99% of errors or defects, far above human accuracy. By cutting errors, speeding up order processing, and operating around the clock, AI-driven robots help warehouses handle more volume in less time. One analysis noted that implementing autonomous robots and automated systems led to a 25–30% reduction in overall warehousing and logistics costs for businesses. These hard numbers explain why so many warehouses are investing in robotics to stay competitive.
AI-driven robots automate crucial warehouse tasks such as picking, packing, and sorting. These robots increase operational efficiency, speed up order processing, and reduce human errors. By handling repetitive and physically demanding tasks, AI robots also help optimize workforce allocation, focusing human efforts on more strategic activities.
6. Real-Time Inventory Tracking
AI, combined with IoT sensors, enables real-time inventory tracking throughout the supply chain. This means at any given moment, a company knows exactly how much stock it has and where it is – whether on store shelves, in a warehouse, or in transit. Technologies like RFID (radio-frequency identification) tags and smart barcode systems feed continuous data about inventory movements, and AI systems process this flood of information instantaneously. The benefit is greatly improved inventory accuracy: errors from manual stock counts or delayed database updates are eliminated. For example, an item removed from a warehouse for shipment is immediately deducted from inventory counts by the AI system, and if that causes the stock to hit a reorder threshold, the system can prompt a restock (as discussed in automated reordering). Real-time tracking also helps prevent shrinkage (loss of inventory due to theft or misplacement) because AI can quickly flag anomalies – like inventory that is “missing” in the data stream. Moreover, with up-to-the-minute inventory visibility, businesses can implement strategies like “omnichannel” retail, where a customer can order a product online and the system will locate the nearest store or warehouse that has it in stock for fastest delivery. Overall, AI-driven real-time tracking creates a single, accurate picture of inventory across all locations in real time, leading to smarter decisions and better customer service (since one can confidently promise a customer an item is available).
AI integrates with IoT devices to offer real-time tracking of inventory levels, providing up-to-date information that helps prevent discrepancies and improves inventory accuracy.
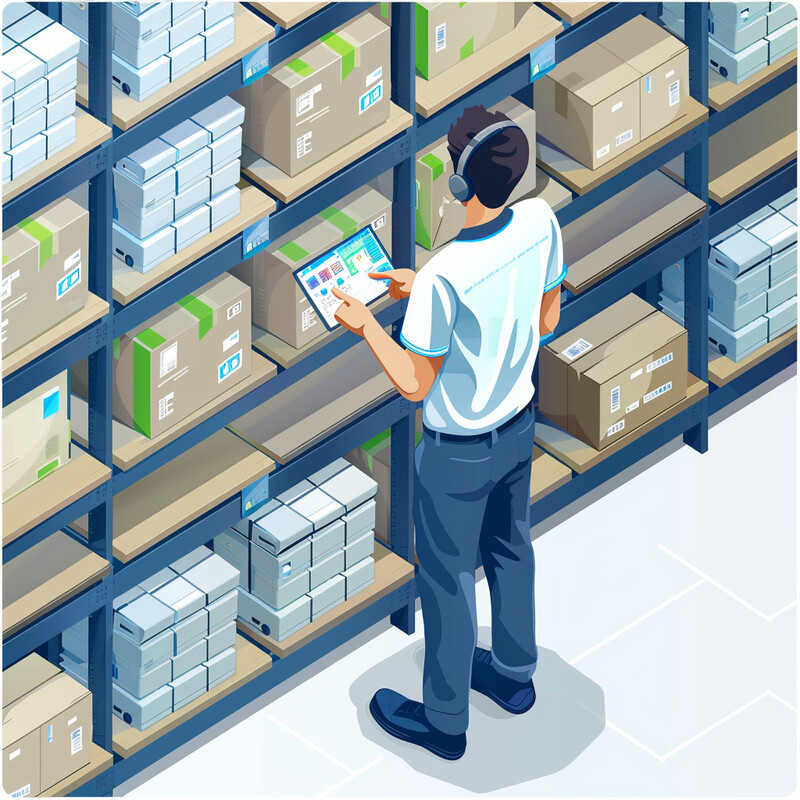
The use of AI and advanced tracking tech is dramatically improving inventory accuracy and reducing stock shortfalls. A study by Auburn University found that implementing RFID (an important real-time tracking tool) raised inventory accuracy from about 65% (with older manual methods) to 95% with an AI-monitored RFID system. In the same study, retailers saw out-of-stock incidents drop by 50% once real-time tracking via RFID was in place, and even recorded a 5% uptick in sales thanks to better product availability. In practice, this means shelves are correctly stocked and inventory records actually match reality – if a customer or store employee checks the system for an item, it’s very likely to be correct about whether it’s on-hand. Another industry expert noted that RFID and AI together give retailers 95–98% inventory accuracy, versus the roughly 45–65% accuracy levels of systems without such technology. This high accuracy is crucial for strategies like buy-online-pickup-in-store or ship-from-store; it prevents situations where an online order is accepted but the item isn’t actually available. With real-time inventory tracking, companies operate with far fewer surprises, leading to smoother operations and happier customers who can trust that products listed as “in stock” truly are available.
Integrating AI with IoT devices enables real-time tracking of inventory items. This connectivity ensures that inventory data is always current, reducing discrepancies between recorded and actual stock levels, and providing businesses with accurate insights for decision-making.
7. Price Optimization
AI is transforming price optimization by allowing retailers and suppliers to set product prices more intelligently and dynamically. Traditionally, pricing might be updated infrequently and based on broad rules or past experience. AI, however, can continuously analyze a variety of factors – competitor pricing, demand fluctuations, inventory levels, customer buying patterns, and even time of day or local events – to determine the ideal price for each product at each moment. This practice, known as dynamic pricing, ensures that prices are high enough to maximize revenue or profit but not so high that they deter customers. For instance, if demand for a product is surging and inventory is limited, an AI system might raise the price slightly to manage the rush (and improve margins); conversely, for a slow-moving item or during a clearance period, the AI will markdown prices to boost sales and clear inventory. These adjustments happen quickly and at scale, often product-by-product, which is far beyond human capabilities for large catalogs. The outcome is that businesses capture more sales and margin that would otherwise be lost with static pricing – they don’t leave money on the table when they could have charged a bit more, and they don’t lose impatient customers by sticking to a high price when competitors have a sale. In short, AI-driven price optimization aligns prices with real-time market conditions and business goals, improving both top-line and bottom-line performance.
AI analyzes market conditions, competitor pricing, and inventory levels to dynamically adjust prices, helping businesses maximize their profit margins while remaining competitive.
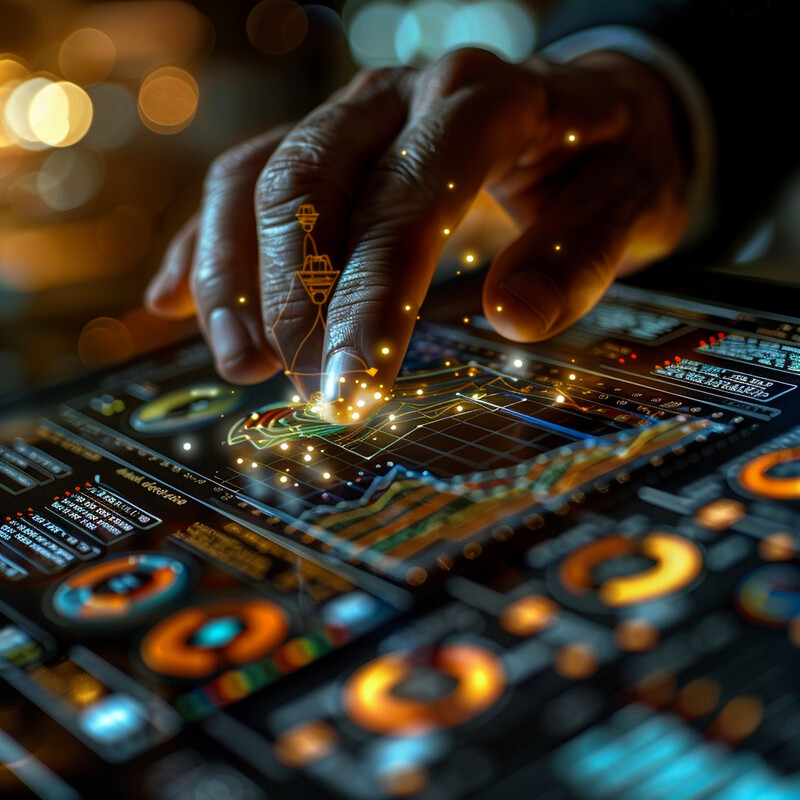
The financial benefits of AI-based price optimization are substantial. Consulting studies have found that using AI for dynamic pricing can increase a retailer’s profits by roughly 10% to 20%. For example, a McKinsey analysis showed that companies adopting AI pricing tools enjoyed double-digit percentage improvements in gross profit, thanks to better pricing precision. In another case, Boston Consulting Group reported that retailers leveraging AI-powered pricing saw gross margin gains of 5–10% while simultaneously growing their sales volumes. These gains come from capturing higher willingness-to-pay on some transactions and stimulating additional demand on others through targeted discounts. A concrete illustration is online retail: an AI might recognize that a certain customer segment is less price-sensitive for overnight shipping and adjust those fees accordingly, boosting revenue, whereas it might drop the price on a product sitting in inventory to entice a sale before the item becomes obsolete. Over time, these granular optimizations have a huge cumulative effect. Retailers also note improved customer perception – by using AI to adjust prices and promotions in a customer-centric way (for instance, offering personalized discounts), some have seen customer satisfaction scores rise, creating a win-win of happier customers and better financial outcomes.
AI analyzes various factors such as market demand, competitor pricing, and current stock levels to dynamically adjust product prices. This price optimization strategy helps businesses maximize their profits while staying competitive in the market, ensuring they can react quickly to market changes.
8. Quality Control
AI is greatly improving quality control in manufacturing and distribution by automating and enhancing the inspection of products. Traditionally, quality control relied on human inspectors to spot defects or issues in products – a process that can be slow and prone to human error or fatigue. Now, AI systems (especially those using computer vision) can examine products at high speed and with remarkable accuracy. For example, on a production line, an AI-driven camera system can inspect each item or component in milliseconds, identifying tiny flaws or deviations from specification that a person might miss. These systems “learn” what a perfect product looks like and can flag anything that falls outside the norm. Beyond visual inspection, AI sensors monitor things like temperature, dimensions, or performance metrics in real time to catch any quality issues immediately. Moreover, AI doesn’t just detect defects; it can also analyze patterns of defects to pinpoint the root cause (maybe a particular machine is miscalibrated at certain times, or a certain supplier’s material has inconsistencies). This predictive insight allows companies to fix problems earlier in the process, preventing large batches of defective products. The overall effect is that products coming out of factories have far more consistent quality, with less waste and rework. AI-driven quality control ensures customers receive reliable, well-made goods, while manufacturers benefit from lower costs associated with defects, returns, or warranty claims.
AI systems use image recognition and other sensor data to identify damaged or defective products during handling and storage, ensuring quality control and reducing waste.
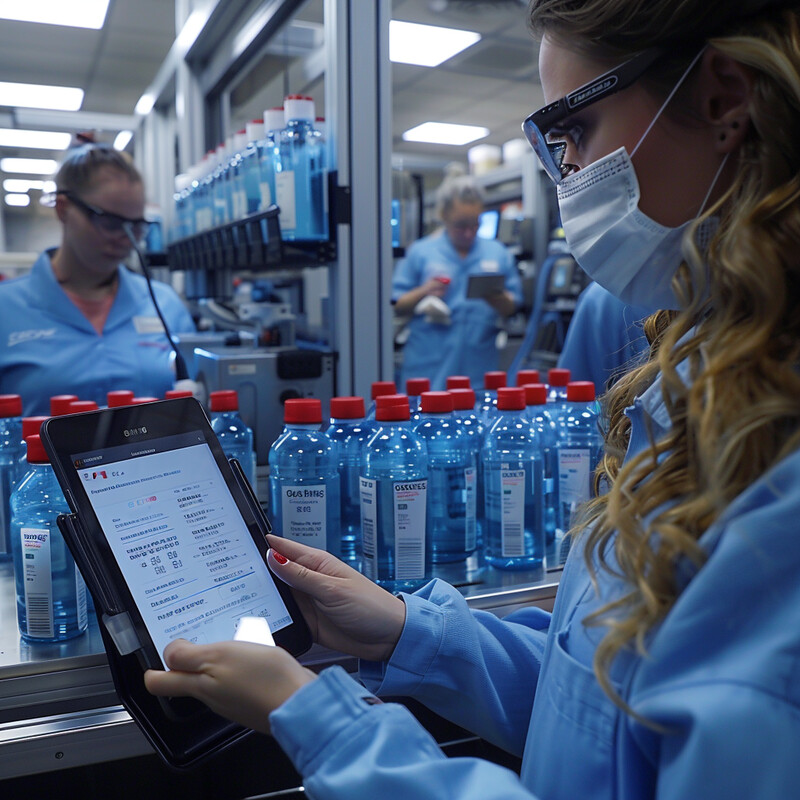
The efficiency of AI in quality inspection far exceeds manual methods. AI-based vision systems can detect defects with over 90% accuracy, compared to roughly 70–80% accuracy for human inspectors on the same tasks. This jump in accuracy means far fewer defective items slip through. For instance, in electronics manufacturing, an AI might catch a hairline crack in a circuit board that a person would overlook, thus preventing a faulty product from ever reaching a customer. In addition to improved detection, AI speeds up the process – it can check hundreds of parts per minute, which would be impossible manually. Importantly, better quality control has financial payoffs: a McKinsey study estimated that AI-driven quality programs can reduce manufacturing costs by up to 20% (by cutting down scrap, rework, and warranty repairs). Companies also report that AI quality systems quickly pay for themselves; for example, a factory that introduced AI inspection saw defect rates drop so much that their re-inspection labor and scrap material costs were slashed in the first year. Furthermore, AI can help ensure regulatory compliance (especially in industries like pharmaceuticals or automotive where safety is critical) by maintaining consistent quality standards. In short, AI-enabled quality control catches more problems earlier, saves money, and protects the end consumer – a triple benefit that is driving rapid adoption of these technologies in manufacturing.
Using image recognition and sensor data, AI systems can identify damaged or defective products during the warehousing process. This capability ensures that only products meeting quality standards reach the consumer, reducing returns and enhancing customer satisfaction.
9. Customer Service
AI is improving customer service in the realm of inventory management by providing faster and more precise responses to customer inquiries about products and orders. One big application is AI chatbots – these are virtual assistants on websites or messaging apps that can answer customer questions 24/7. For example, if a customer wants to know “Is this item in stock in my size?” or “Where is my package right now?”, an AI chatbot connected to the inventory system can instantly retrieve that information and respond. This immediate service is something human representatives would struggle to provide around the clock or at high volumes. AI in customer service also extends to phone calls, where AI-powered voice agents can handle routine requests (like tracking a shipment or initiating a return) without making the customer wait on hold. Beyond direct Q&A, AI helps customer service by proactively notifying customers about inventory-related issues – such as sending an alert if an item they were interested in is back in stock, or if an order is delayed. These improvements lead to customers getting the information they need quickly, which increases satisfaction. Meanwhile, the human customer service staff can be reserved for more complex issues that truly need a personal touch, since AI filters out the simple repetitive questions. Overall, AI creates a more responsive and efficient customer service experience related to inventory and order management.
AI improves customer service by providing precise inventory information, enabling businesses to respond quickly to customer inquiries about product availability.
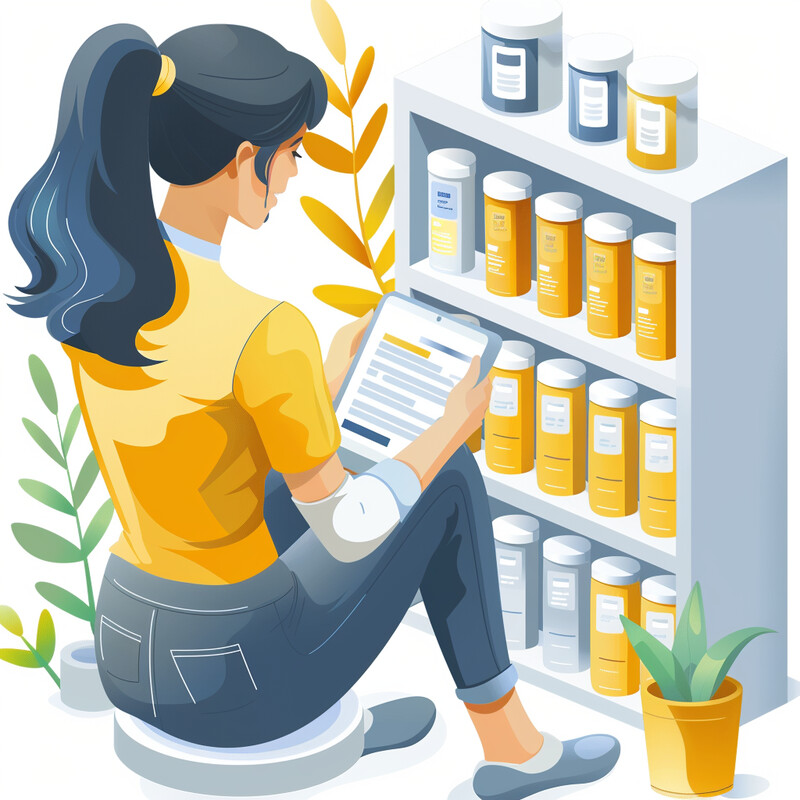
Companies adopting AI chatbots and virtual agents in customer service are seeing tangible benefits in responsiveness and cost. It’s reported that AI chatbots can handle up to 80% of routine customer inquiries (such as product availability checks, order status updates, or FAQ-type questions) without human intervention. This high rate of automated resolution means customers get answers almost instantly via AI, rather than waiting minutes or hours for an email or a call back. As a result, more than half of consumers (around 51%) now say they actually prefer interacting with a bot if they need quick service, since the speed is often greater than with a human agent. On the business side, these AI systems significantly cut costs – one study found a 25% reduction in customer service costs for companies that implemented AI assistants, largely due to lower labor requirements and faster handling of inquiries. Additionally, predictions for the near future suggest that by 2025, AI could be handling as much as 95% of all customer interactions in some form, showing the trend toward heavy automation. For example, a major retailer using an AI chatbot saw its customer satisfaction scores rise because customers were getting immediate, accurate answers about product stock and order issues at any hour. In short, AI-driven customer service is making support more scalable and responsive, which is a win for both the customer and the company’s efficiency.
AI improves customer service by providing accurate and up-to-date inventory information. This transparency enables customer service representatives to offer immediate, informed responses to customer inquiries regarding product availability, expected restock dates, and more, enhancing the overall customer experience.
10. Returns Processing
AI helps manage and streamline returns processing, which is an increasingly important part of inventory management due to the growth of e-commerce and easy return policies. Handling returns (reverse logistics) efficiently is challenging: it involves getting products back from customers, deciding what to do with them (put back into stock, refurbish, recycle, or dispose), and doing all this cost-effectively while keeping the customer experience positive. AI comes into play by predicting and reducing returns and by optimizing the process when returns do happen. Predictively, AI can analyze which products are likely to be returned frequently and why – for example, clothing of a certain brand that often doesn’t fit as expected – and flag these insights to the business. This can lead to actions like improving product descriptions or sizing charts (to help customers choose the right item and avoid the return altogether) or tightening return policies for habitual returners. When returns are initiated, AI can automate the steps: providing customers with instant return authorizations and shipping labels via chatbots, deciding the best return location (e.g., direct to a store vs. a warehouse) based on where that inventory is needed, and even inspecting returned items using computer vision when they arrive. By sorting returned products intelligently, AI ensures that items in good condition go back to stock quickly to be resold, while others might go to secondary markets or recycling. All these improvements mean the returns process becomes faster, less costly, and more sustainable, converting what used to be a pure loss center into a more controlled, optimizable part of the inventory cycle.
AI automates and streamlines the processing of returns, analyzing reasons for returns and reintegrating items into inventory efficiently, thereby enhancing the overall customer experience.
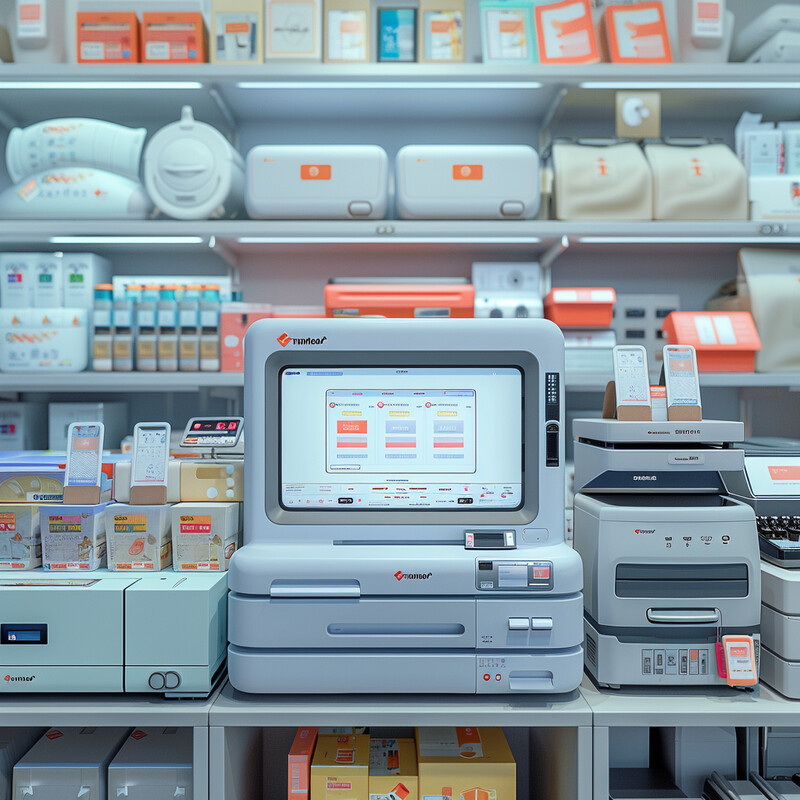
With retail product returns expected to reach an astonishing $890 billion in value in 2024, merchants are turning to AI to cut down the associated costs and hassles. A recent industry report noted that 36% of retailers are now using AI to analyze customers’ purchase and return history in order to predict if a specific customer is likely to return items, allowing them to take proactive steps. Similarly, 39% of retailers are employing AI to identify products that have high return rates and to understand the reasons (such as sizing issues or quality problems) so they can address those root causes. These measures can significantly reduce the volume of returns by preventing avoidable ones – for instance, if AI shows a particular garment is often returned due to fit, the retailer might adjust the sizing info or cut. AI is also used to optimize the returns process itself: about a quarter of retailers (25%) have AI-driven programs to help customers choose the right size before purchase, which directly lowers return rates. For the returns that do happen, companies like Amazon have algorithms to decide if it’s cheaper to have the customer keep an inexpensive item rather than ship it back, or to route a return to the nearest warehouse that actually needs that stock. These AI-driven strategies are saving retailers millions – by one estimate, AI-based improvements in returns handling and fraud detection can save large retailers around 10–15% of their return costs annually. Ultimately, AI is turning returns into a more manageable part of the inventory lifecycle, minimizing unnecessary losses and even improving customer loyalty (since a smoother, smarter returns process makes customers more confident in their purchases).
AI automates the returns processing by efficiently handling the logistics of returned items. It quickly assesses the condition of returned goods, decides whether they can be resold or should be scrapped, and updates inventory levels accordingly. This streamlined process reduces the administrative burden of returns, speeds up the resolution for customers, and helps recapture value from returned items.