1. Enhanced Precision and Accuracy
AI enables robots to perform tasks with higher precision and accuracy, reducing errors in processes like assembly, welding, and painting.
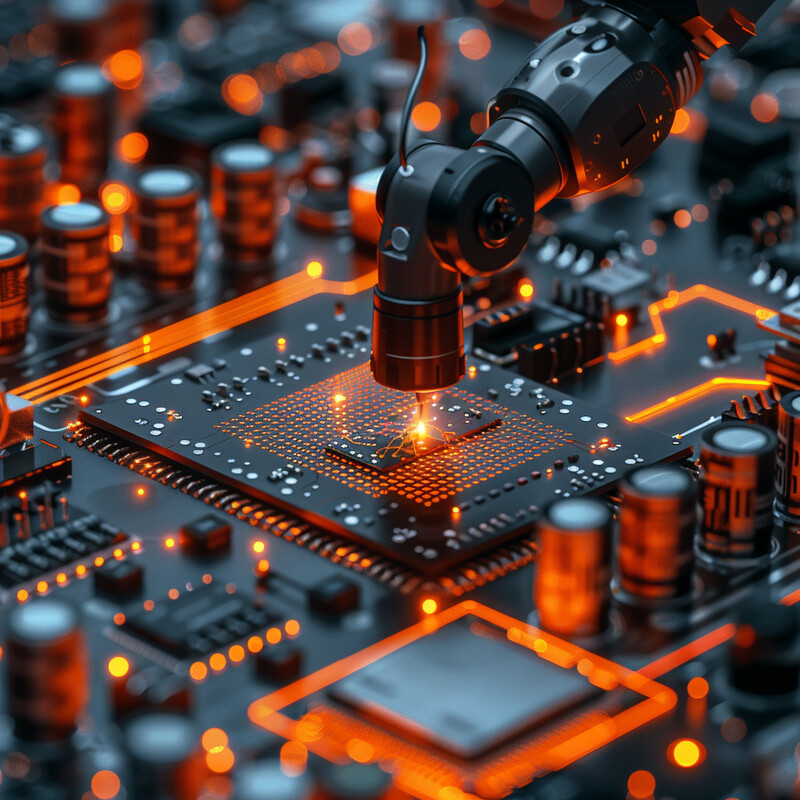
AI significantly enhances the precision and accuracy of industrial robots by utilizing advanced algorithms that allow for finer control over robotic actions. This is particularly important in tasks that require exact movements, such as assembling small components, performing intricate welding, or applying precise layers of paint. The improved accuracy reduces waste, improves product quality, and lowers the likelihood of defects.
2. Adaptive Learning
AI allows robots to learn and improve from each task they perform, adapting their operations based on new data to enhance productivity and quality over time.
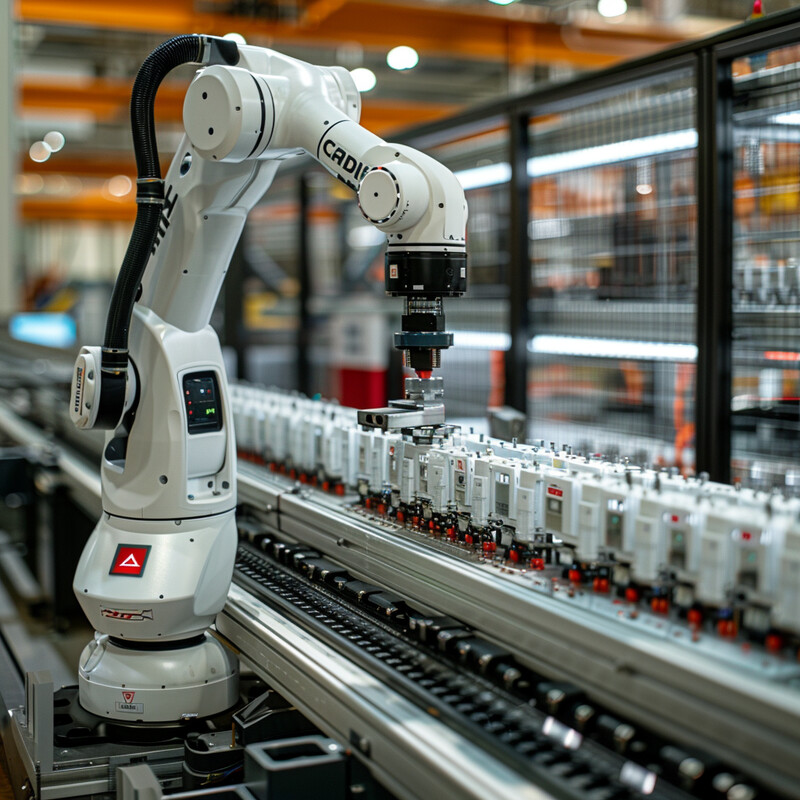
Through machine learning techniques, industrial robots can continually adapt and optimize their performance based on real-time feedback from their tasks. This adaptive learning enables robots to adjust their methods as they encounter different or unexpected situations, enhancing their efficiency and effectiveness in dynamic environments. As a result, they become more proficient over time, learning from each operation they perform.
3. Predictive Maintenance
By monitoring the condition of robotic equipment, AI predicts when maintenance is needed, reducing downtime and extending the lifespan of machinery.
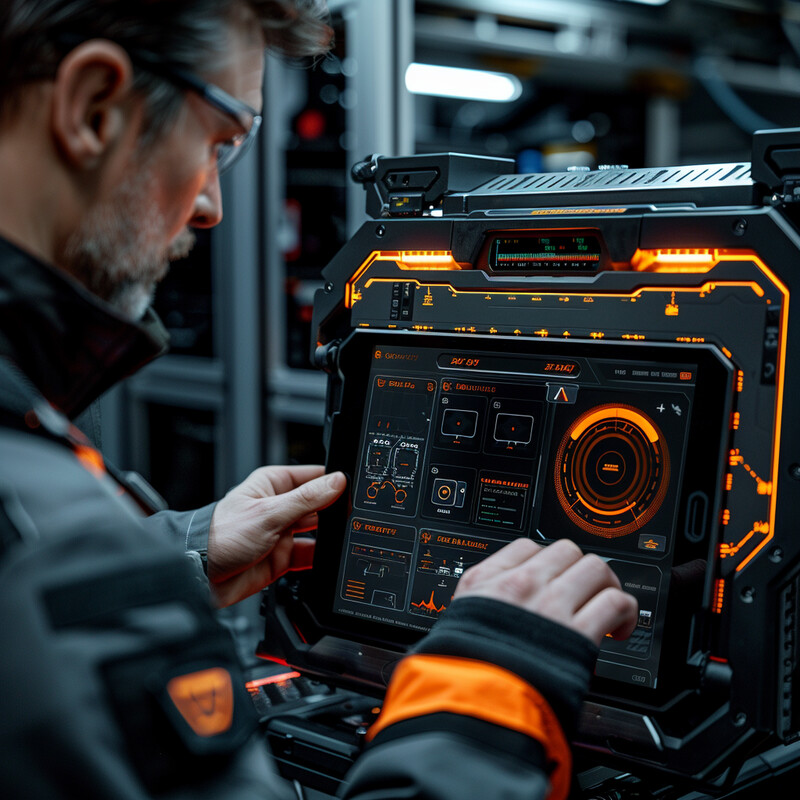
AI-driven predictive maintenance utilizes data collected from sensors embedded in robotic systems to monitor their health and predict potential failures before they occur. This approach allows maintenance to be scheduled at optimal times, minimizing unexpected downtime and extending the machinery's operational life. By preventing breakdowns, AI ensures continuous production flows and reduces maintenance costs.
4. Autonomous Decision-Making
AI equips robots with the capability to make decisions during complex tasks, allowing them to adjust operations dynamically based on real-time environmental inputs.
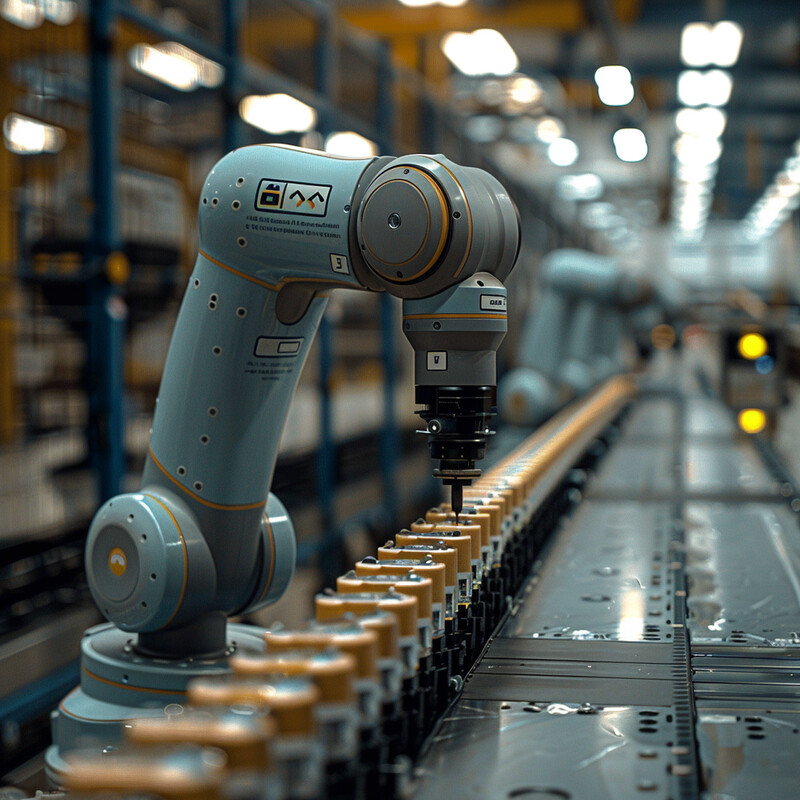
AI equips industrial robots with autonomous decision-making capabilities, enabling them to make critical operational decisions independently. For instance, in complex assembly lines, robots can decide how to handle irregular or unexpected conditions without human intervention. This autonomy is crucial for maintaining efficiency and continuity in fast-paced industrial settings.
5. Collaborative Robotics (Cobots)
AI improves the safety and effectiveness of cobots, which work alongside human operators, by enhancing their ability to sense and respond to human presence and actions.
-3.jpg)
Cobots, designed to work alongside humans, are enhanced by AI to better understand and respond to human actions and presence safely. AI algorithms help cobots detect human workers' locations and movements, allowing them to adjust their operations to avoid accidents and collaborate more effectively. This interaction enhances workplace safety and increases productivity by seamlessly integrating human skills with robotic precision.
6. Vision Systems
AI-powered vision systems enable robots to recognize and handle objects of different shapes, sizes, and orientations, expanding their range of tasks.
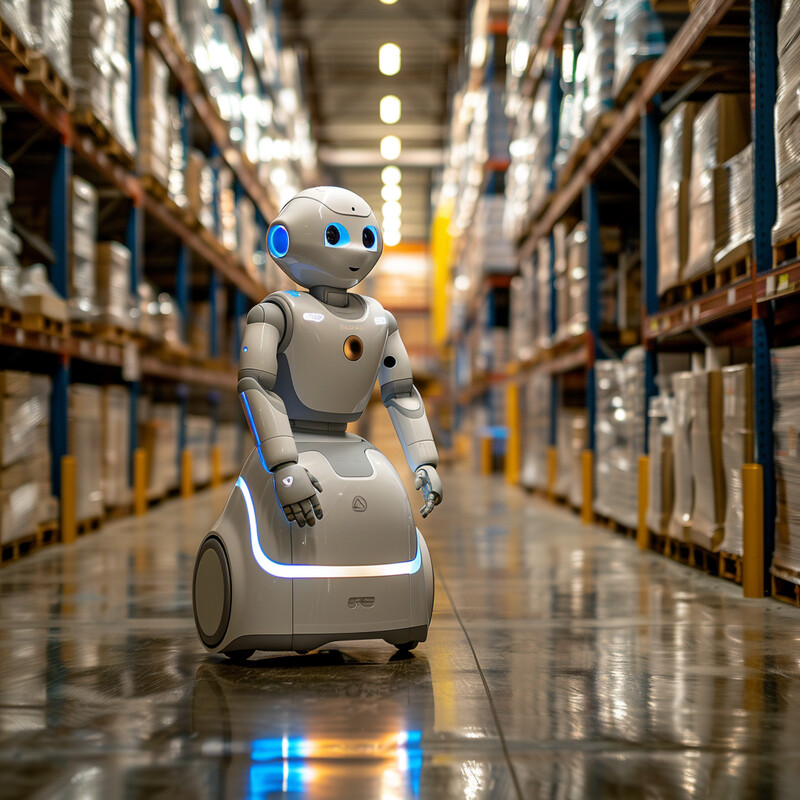
AI-powered vision systems grant robots the ability to identify and manipulate objects that vary in shape, size, and orientation. This capability is vital for tasks such as sorting mixed products on a conveyor belt or assembling parts from an unstructured bin. AI enhances the vision systems' accuracy in object recognition, even under varying lighting conditions and orientations, expanding the range of applications for robotic systems.
7. Increased Flexibility
AI enhances the flexibility of robots, allowing them to switch between different tasks without manual reprogramming, making them more adaptable to varying production needs.
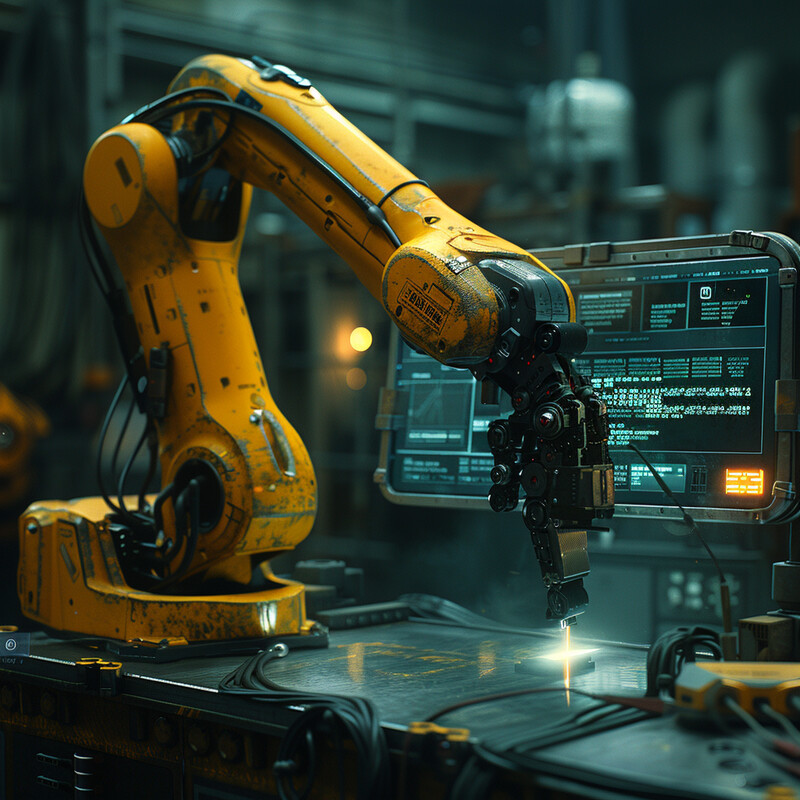
AI enables robots to quickly switch between different tasks without extensive manual reprogramming. This flexibility is achieved through AI models that can learn and adapt to new tasks by analyzing data on the fly. Such adaptability is essential for manufacturers who need to change production lines or introduce new products without significant downtime.
8. Integration with IoT
AI integrates robots with other IoT devices in the industrial setup, enabling a more cohesive and interconnected manufacturing environment.
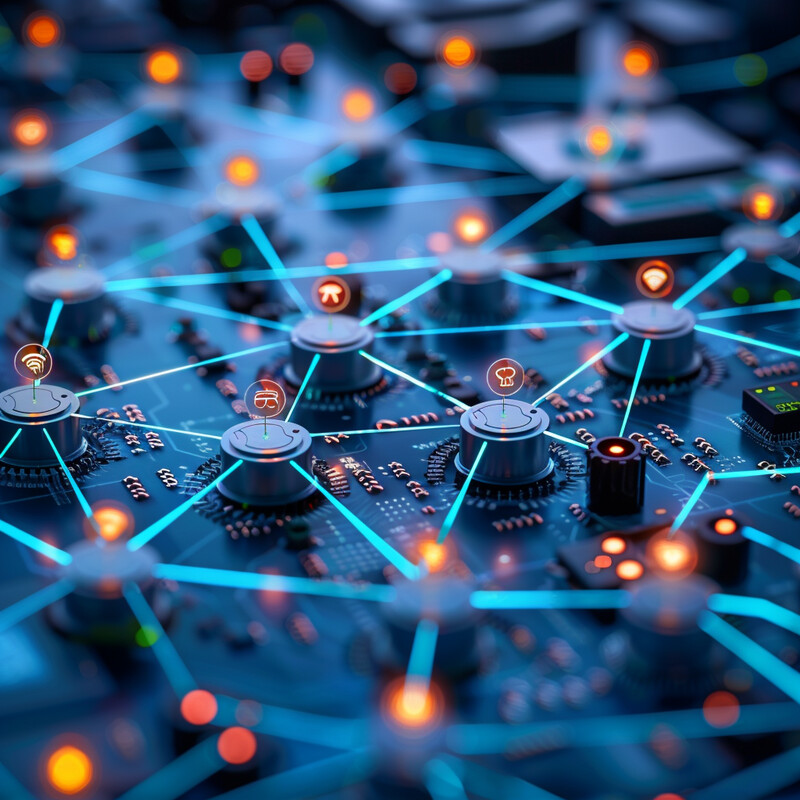
Integrating AI with the Internet of Things (IoT) allows robots to operate as part of a connected ecosystem within industrial settings. AI analyzes data from various IoT sensors and devices to optimize robot actions based on comprehensive environmental inputs, enhancing the synchronization of entire production lines and improving overall factory efficiency.
9. Energy Efficiency
AI optimizes the energy consumption of robots by determining the most efficient paths and speeds for task execution, reducing the overall energy use in operations.
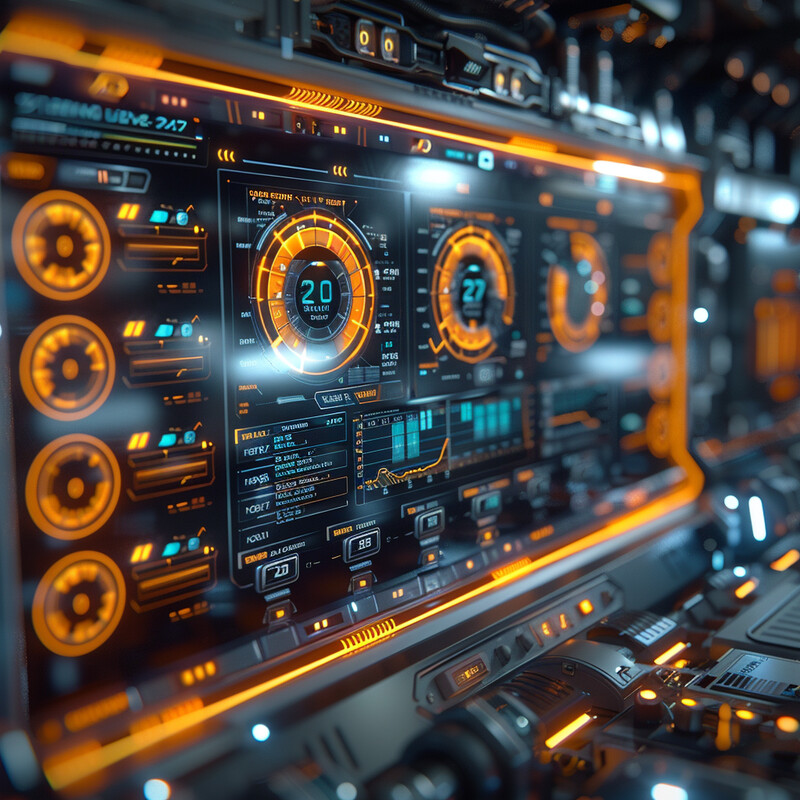
AI optimizes the energy consumption of robotic systems by calculating the most efficient routes and operational speeds. This optimization not only reduces the energy costs associated with robot operation but also contributes to more sustainable manufacturing practices by minimizing the overall energy footprint of industrial operations.
10. Data Analytics for Process Improvement
AI analyzes data collected by robots to identify inefficiencies and bottlenecks in production processes, suggesting improvements and innovations that can enhance overall operational efficiency.
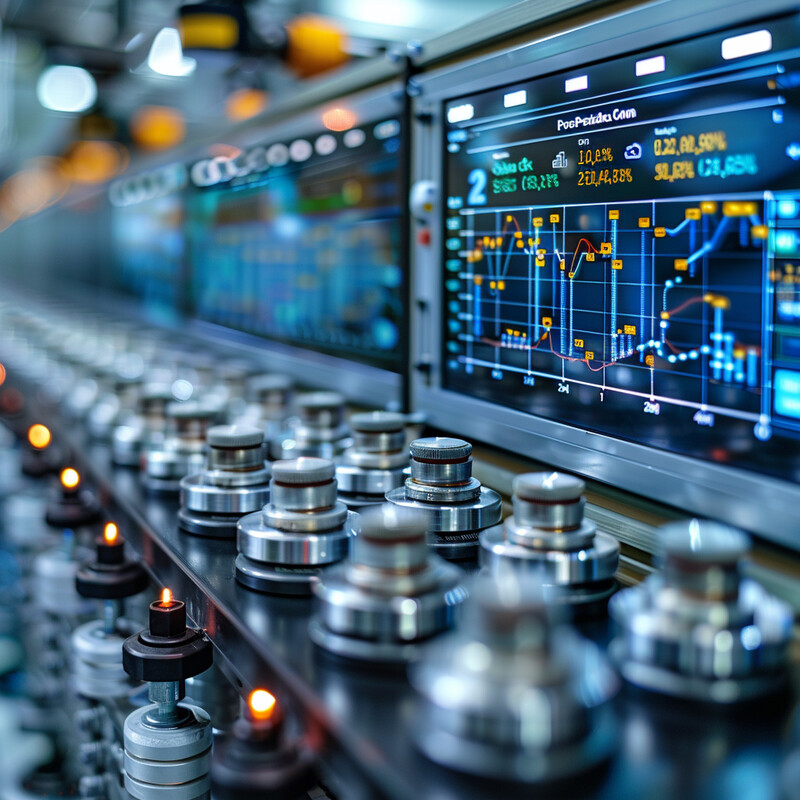
AI leverages data analytics to evaluate the performance of robotic operations, identifying inefficiencies and areas for improvement. By analyzing patterns in data collected by robots, AI can suggest modifications to enhance speed, quality, and cost-effectiveness, continuously driving innovations in production processes.