1. Enhanced Accuracy
AI algorithms significantly improve the accuracy of data collected by health monitoring wearables. By filtering out noise and motion artifacts in sensor readings, AI helps minimize errors in measurements like heart rate, blood pressure, and oxygen saturation. Machine learning models can also calibrate wearable sensors against gold-standard measurements and adjust for individual differences (such as skin type or fit), yielding more reliable health metrics. This ensures that users and healthcare providers can trust wearable data for assessments. As a result, AI-enabled wearables approach clinical-grade accuracy, making them more effective tools for monitoring and decision-making in healthcare.
AI algorithms improve the accuracy of data collected by wearables, such as heart rate, blood pressure, and sleep patterns, ensuring reliable health monitoring.
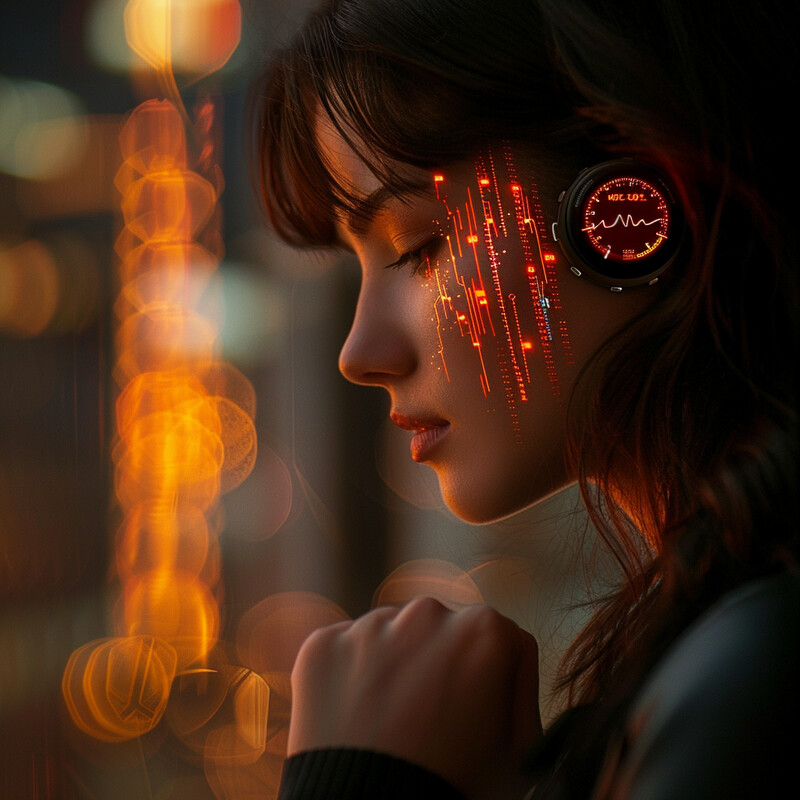
AI integration in wearable medical devices is projected to improve disease diagnosis accuracy by over 20% as of 2024. In other words, analytics powered by AI can make health readings from wearables about one-fifth more accurate in identifying conditions than previous generations without AI (Market.us, 2024).
AI algorithms significantly improve the accuracy of data collected by health monitoring wearables. By analyzing the raw data from sensors and applying advanced data processing techniques, AI helps in minimizing errors and enhancing the reliability of health metrics like heart rate, blood pressure, and oxygen saturation. This ensures that users and healthcare providers can trust the data for health assessment and decision-making.
2. Predictive Health Insights
AI enables wearables to analyze historical and real-time data to predict potential health issues before they become critical. By recognizing subtle patterns or trends – for example, changes in heart rate variability, activity levels, or sleep quality – machine learning models can forecast health risks such as cardiac arrhythmias or asthma exacerbations days or weeks in advance. These predictive health insights allow for early warnings and proactive interventions. For instance, an AI-driven wearable can alert a user to a possible atrial fibrillation episode or a worsening heart failure status, prompting them to seek preventive care. Overall, AI transforms wearables from passive trackers into intelligent systems that anticipate problems, enabling timely lifestyle adjustments or medical attention to improve outcomes.
AI uses historical data to predict potential health issues before they become critical, allowing for preventive measures or early medical intervention.
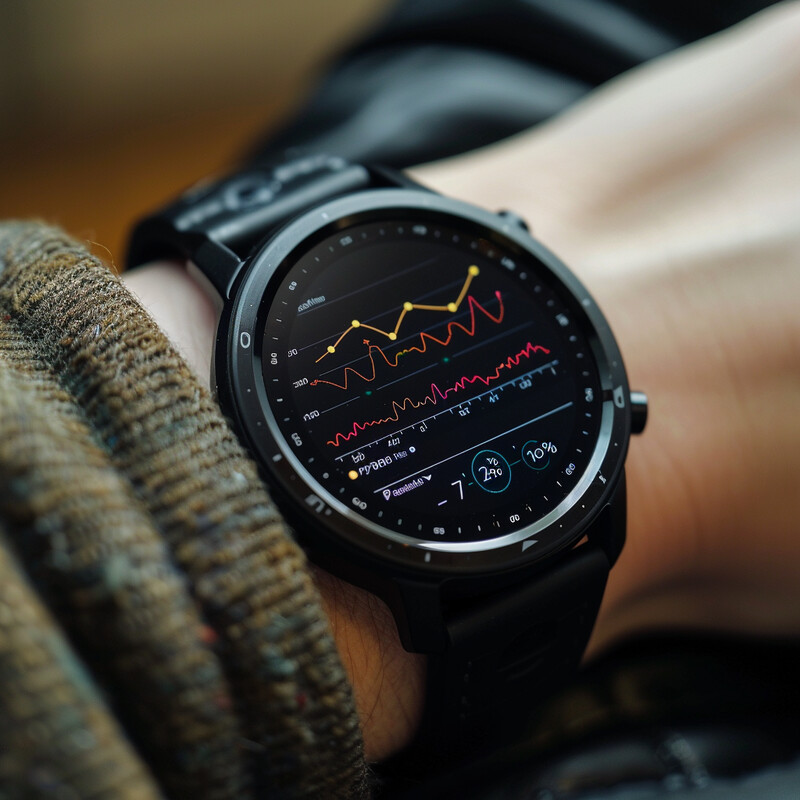
In a 2025 study, researchers used machine-learning on data from 14,000 wearable users and were able to predict all-cause hospitalizations with 91% accuracy. This high level of precision demonstrates how AI can reliably forecast major health events (Heart Rhythm Society, 2025).
AI uses historical health data and machine learning models to predict potential health issues before they manifest into more serious conditions. For example, AI can analyze trends in heart rate variability and other biometrics to predict cardiovascular risks, enabling preventive actions such as lifestyle adjustments or early medical intervention.
3. Personalized Feedback
AI tailors health advice and feedback from wearables to the individual user’s data and needs. Instead of one-size-fits-all suggestions, machine learning systems learn a person’s typical patterns – their fitness level, sleep habits, diet, and vital signs – and generate customized recommendations. For example, an AI-enabled fitness tracker might notice that a user’s heart rate recovery is slow and suggest personalized workouts or rest days accordingly. Similarly, nutrition or stress management tips are adapted to what the wearable knows about the user’s lifestyle and goals. This personalized feedback is more relevant and actionable, which can increase user engagement and adherence. By receiving coaching that fits their unique profile, users are more likely to improve their behavior and achieve better health outcomes than with generic feedback.
AI tailors health advice and feedback to individual users based on their specific health data and activity levels, enhancing personalized health management.
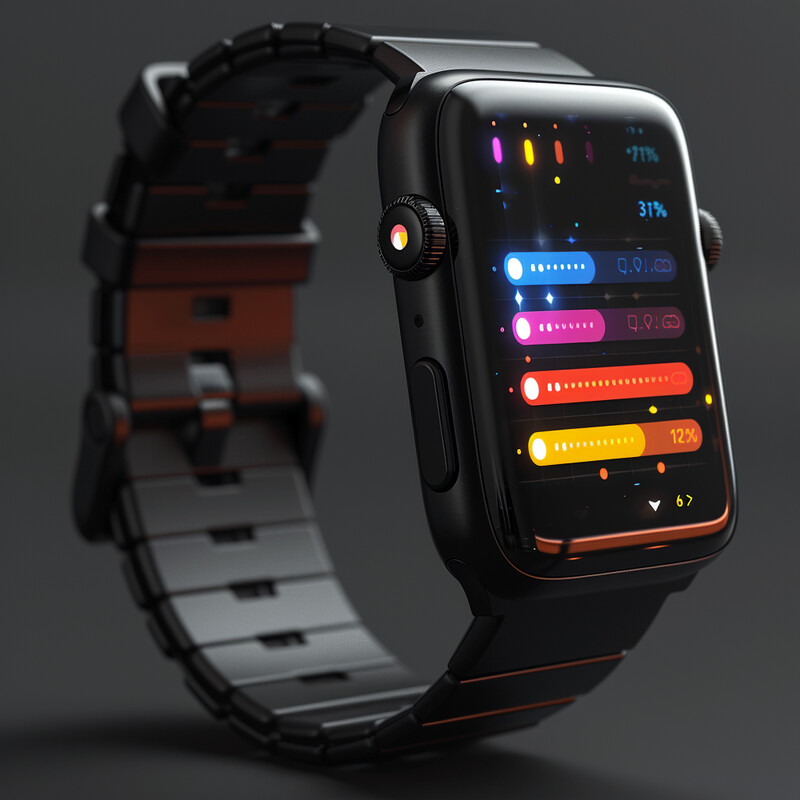
According to a 2024 study, users who received AI-personalized health recommendations from their wearables saw a 25% improvement in health outcomes compared to those given generic feedback (Chen et al., 2024). This indicates that tailored guidance markedly enhances the effectiveness of health interventions.
AI personalizes health feedback by analyzing individual health data over time. This personalized approach allows the wearable to provide tailored advice on nutrition, exercise, and lifestyle based on the user’s unique health profile and goals, making the guidance more relevant and effective for individual health improvement.
4. Real-Time Health Monitoring
AI empowers wearables with continuous, real-time health monitoring and instant analysis of sensor data. Rather than just recording data for later review, AI algorithms on the device (or in the cloud) evaluate incoming signals (like heart rhythm, glucose levels, or blood pressure) on the fly. This means the wearable can immediately detect abnormal patterns or dangerous values and alert the user in real-time. For example, an AI-driven glucose monitor can predict a hypoglycemic episode hours in advance and warn the wearer to eat something, or a smartwatch can instantly flag an irregular heartbeat. Real-time analysis is crucial for conditions requiring prompt attention, such as arrhythmias, seizures, or severe hypertension. By providing instantaneous feedback and emergency alerts, AI-enabled wearables help users and caregivers intervene early, potentially preventing health crises and saving lives.
AI enables continuous real-time monitoring and analysis of health metrics, providing instant feedback and alerts if abnormal patterns are detected.
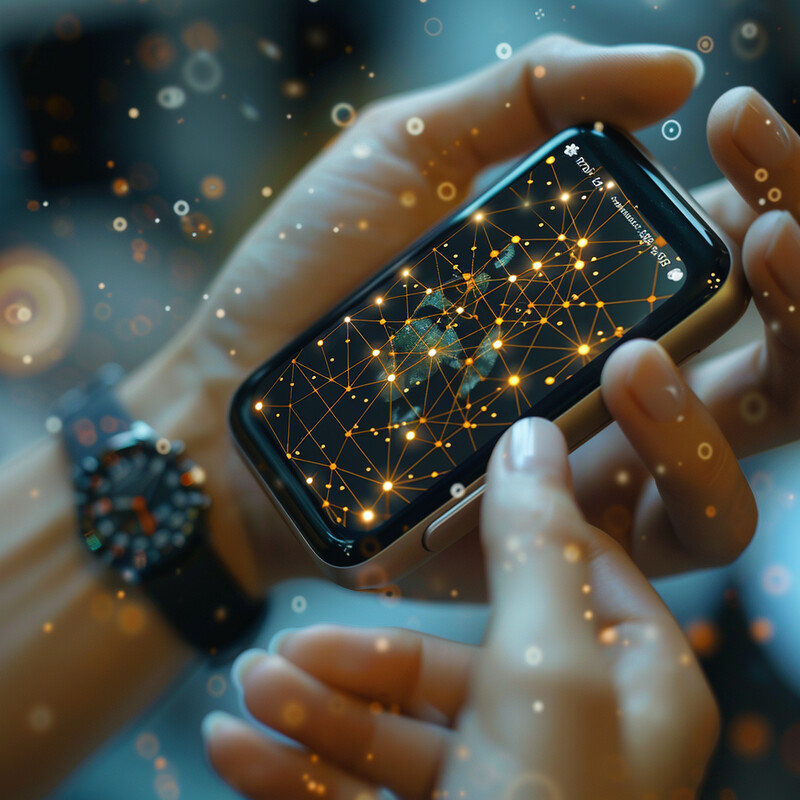
Advanced AI-powered continuous glucose monitors can now forecast dangerous blood sugar fluctuations hours ahead of time, enabling diabetics to act preemptively (Veluvali et al., 2025). In 2025, researchers reported that such AI-enhanced wearables could predict significant glucose drops or spikes several hours before they occur, illustrating the power of real-time monitoring for chronic conditions.
AI enables continuous, real-time monitoring of health metrics, allowing wearables to provide immediate feedback or raise alerts if abnormal patterns are detected. This capability is critical for monitoring conditions that require constant surveillance, such as diabetes or cardiac conditions, where timely information can be crucial.
5. Integration with Medical Records
AI facilitates the seamless integration of wearable data with electronic health records (EHRs), enhancing continuity of care. Health monitoring wearables generate a vast amount of data (heart rates, activity logs, sleep trends, etc.), and AI systems can filter and summarize this information for clinicians. By automatically transferring relevant insights into a patient’s medical record, AI ensures healthcare providers have an up-to-date, comprehensive view of the patient’s daily health metrics. This integrated data can improve clinical decision-making – for example, a doctor can see that a patient’s home-monitored blood pressure has been rising and adjust medications accordingly. AI also helps flag anomalies in the wearable data to the physician and avoids information overload by highlighting what’s clinically important. Overall, combining wearable data with medical records via AI leads to more personalized and data-driven care, bridging the gap between everyday health tracking and formal medical supervision.
AI facilitates the integration of data from wearables with electronic health records, enhancing the continuity of care by providing healthcare providers with comprehensive patient data.
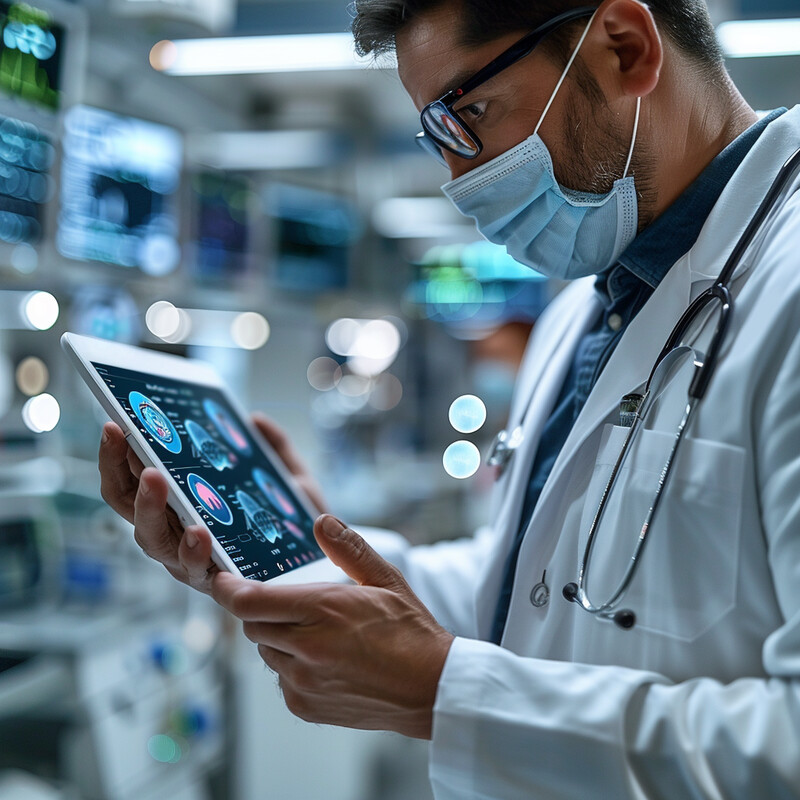
Despite its potential, adoption has been gradual – in a 2022 survey, only 10% of U.S. physicians reported that they had integrated data from patient wearables into their EHR systems. This is beginning to change as major EHR platforms now allow importing wearable data, but it shows the early stage of medical record integration (Deloitte, 2022).
AI facilitates seamless integration of data collected by wearables with electronic health records (EHRs). This integration provides healthcare professionals with a holistic view of a patient’s health status, including real-time data, which can enhance diagnosis, treatment planning, and monitoring, improving the overall quality of care.
6. Activity Recognition and Optimization
AI enables wearables to automatically recognize a wide range of physical activities and provide actionable fitness insights. Using machine learning classifiers (often trained on accelerometer and gyroscope data), a wearable can discern whether a user is running, cycling, swimming, or even performing specific exercises, without manual input. This high level of activity recognition allows the device to track exercise type, intensity, and form with greater accuracy. Beyond identification, AI analyzes the quality of the activity – for example, detecting inefficient gait patterns or suboptimal heart rate zones – and offers suggestions to optimize the workout. The wearable might recommend increasing intensity, correcting posture, or diversifying exercises to reach the user’s fitness goals. By tailoring fitness advice (like ideal workout duration or recovery time) based on the detected activity and user performance, AI helps individuals exercise more effectively and safely. This results in improved fitness outcomes and reduces the risk of injury or overtraining.
AI recognizes different physical activities and provides insights and recommendations to optimize the user's fitness routine and overall health.
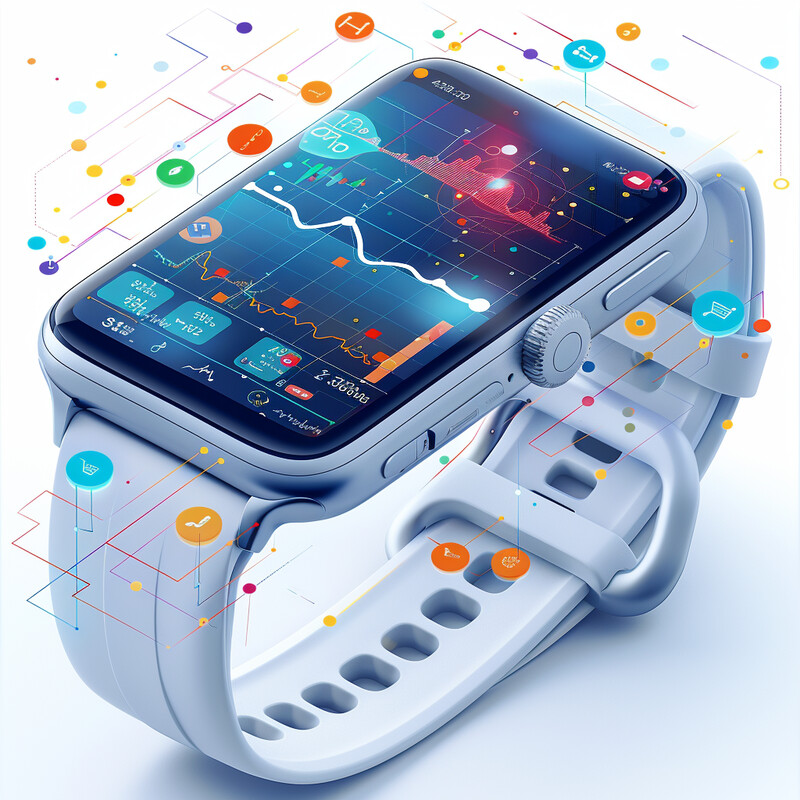
Researchers have achieved extremely high accuracy in automated activity detection. For instance, a 2023 study using a hierarchical deep learning model reached 99.15% accuracy in recognizing different human physical activities from wearable sensor data. Such precision underscores how well AI can classify and optimize activities (Shajari et al., 2023).
AI in wearables can accurately recognize different types of physical activities and provide insights based on the intensity and duration of these activities. It offers recommendations to optimize the user’s fitness regime, such as suggesting changes in workout types or durations to improve health outcomes based on the user’s health status and fitness goals.
7. Stress and Recovery Analysis
AI algorithms in wearables assess physiological signals to gauge stress levels and recommend recovery strategies. Modern wearables can track indicators of stress such as heart rate variability, electrodermal activity (skin conductance), and breathing patterns. AI analyzes these metrics to determine when the user is experiencing elevated stress or not recovering well from exertion. For example, a sudden increase in skin conductance and heart rate might be interpreted by the AI as acute stress. In response, the wearable can proactively suggest personalized relaxation techniques – like guiding the user through a deep breathing exercise, prompting a short walk break, or recommending a meditation session. AI also monitors recovery states (for instance, during sleep or rest days) and can advise if the body is fully recovered or needs more rest. By providing real-time feedback on stress and recovery, AI helps users manage their mental and physical health more effectively. This leads to better stress resilience, prevents burnout or overtraining, and supports overall well-being through timely interventions.
AI analyzes physiological signals to assess stress levels and recommend recovery strategies, helping users manage their mental and physical health.
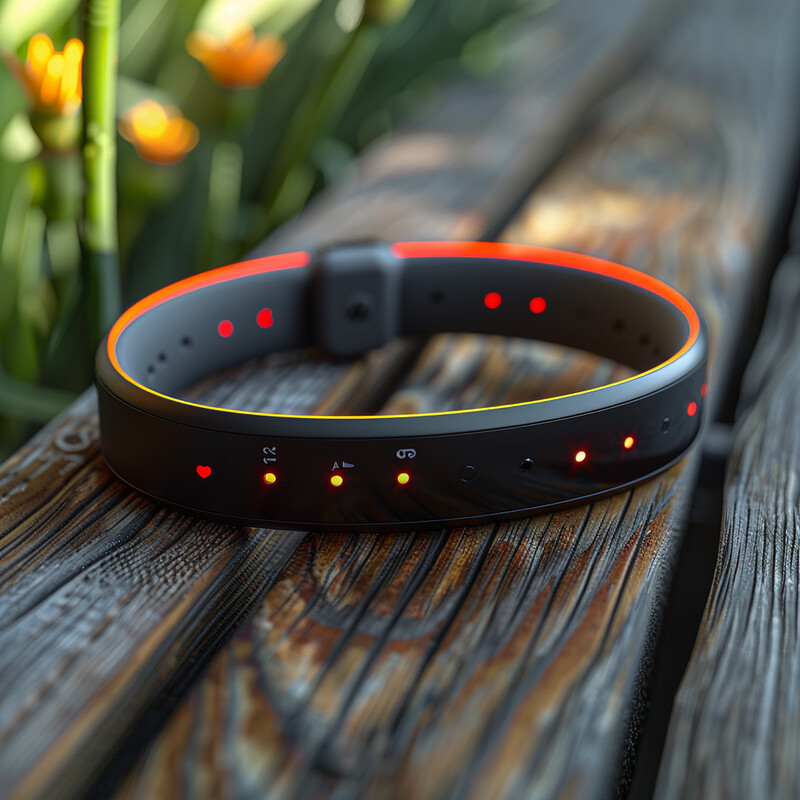
Wearable AI can classify stress with notable accuracy. In one experiment using a smart wristband’s biosignals, an AI model distinguished stress vs. non-stress states with about 86% accuracy. This demonstrates that machine learning can reliably detect stress from physiological data (Zhu et al., 2022).
AI analyzes data related to physiological indicators of stress, such as heart rate and sweat gland activity. It uses this information to assess stress levels and suggest recovery techniques, such as breathing exercises or rest periods, helping users manage their mental and physical well-being more effectively.
8. Sleep Quality Improvement
AI is enhancing the ability of wearables to monitor sleep patterns and improve sleep quality for users. Smartwatches and fitness bands now use AI to analyze data such as movement, heart rate, and blood oxygen to determine sleep stages (light, deep, REM sleep) with increasing accuracy. By identifying disruptions or patterns (like frequent awakenings or insufficient REM sleep), the AI can provide individualized recommendations to improve rest. For example, the wearable might notice a user gets poor sleep on days they have late caffeine and suggest avoiding coffee in the evening, or it might detect better sleep on days with exercise and encourage more activity. Some AI systems factor in environmental data too (noise or temperature from smart home devices) and can advise changes like a cooler room or a quieter setting for optimal sleep. Over time, these personalized suggestions – such as consistent bedtimes, relaxation routines, or adjustments in lifestyle – help users adopt healthier sleep habits. With AI-guided insights, individuals often experience more restorative sleep, which in turn benefits their overall health and daytime functioning.
AI monitors sleep patterns and provides recommendations for improving sleep quality based on deep analysis of sleep stages and environmental factors.
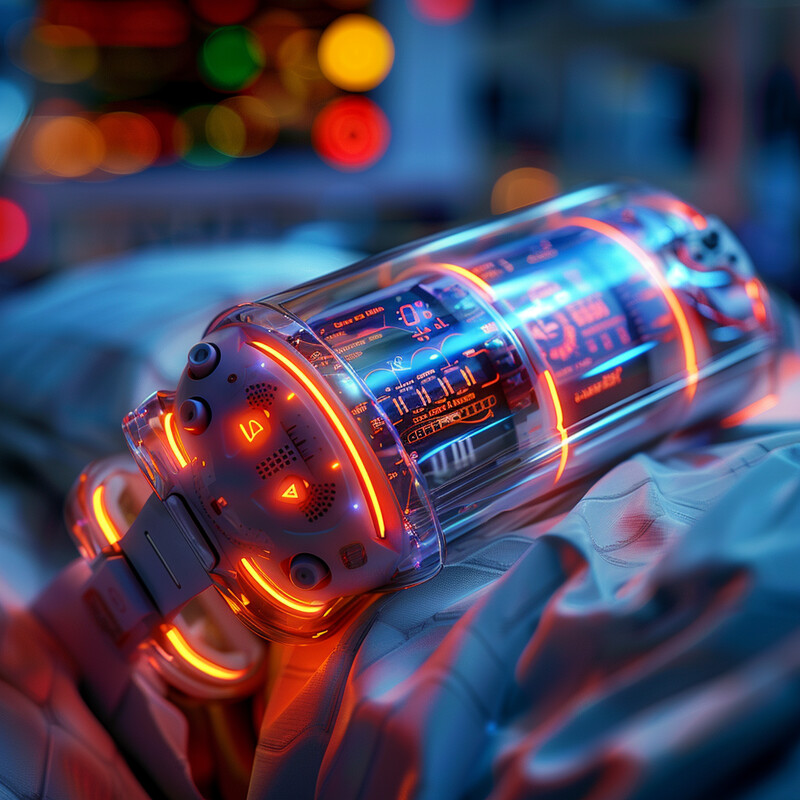
Consumer adoption of AI-driven sleep tracking is high, and it’s making a difference. A recent national survey found that one in three Americans has tried a sleep-tracking device, and among those users, 68% reported changing their behavior based on the sleep data they learned (American Academy of Sleep Medicine, 2023). This highlights how AI-powered sleep insights are prompting healthier habits for better sleep.
Using AI, wearables monitor and analyze sleep patterns, including the duration and quality of different sleep stages. The AI can provide personalized suggestions to improve sleep hygiene, such as adjusting room temperature, modifying bedtime routines, or changing diet habits before sleep, based on the analysis of sleep data and environmental factors.
9. Anomaly Detection
AI equips wearables with robust anomaly detection capabilities, meaning they can automatically spot unusual changes in the user’s health data that may signal a problem. By continuously learning a person’s typical patterns (for vital signs, activity, sleep, etc.), AI can detect when something deviates significantly from the norm. These anomalies might include irregular heart rhythms, a sudden drop in daily activity (potentially indicating illness or a fall), abnormal blood oxygen levels during sleep, or spikes in blood pressure. When such an out-of-range event is identified, the wearable notifies the user (and potentially their physician) so that it can be investigated promptly. This early warning system can lead to earlier diagnosis of conditions – for example, catching asymptomatic atrial fibrillation or early signs of infection before they escalate. In essence, AI serves as a vigilant sentinel, continuously monitoring for red flags in the background. By prompting users to seek medical advice when an anomaly is found, AI-powered wearables help in catching health issues at an earlier, more treatable stage and improve long-term health outcomes.
AI detects anomalies in health data that may indicate underlying health issues, prompting users to seek medical advice when necessary.
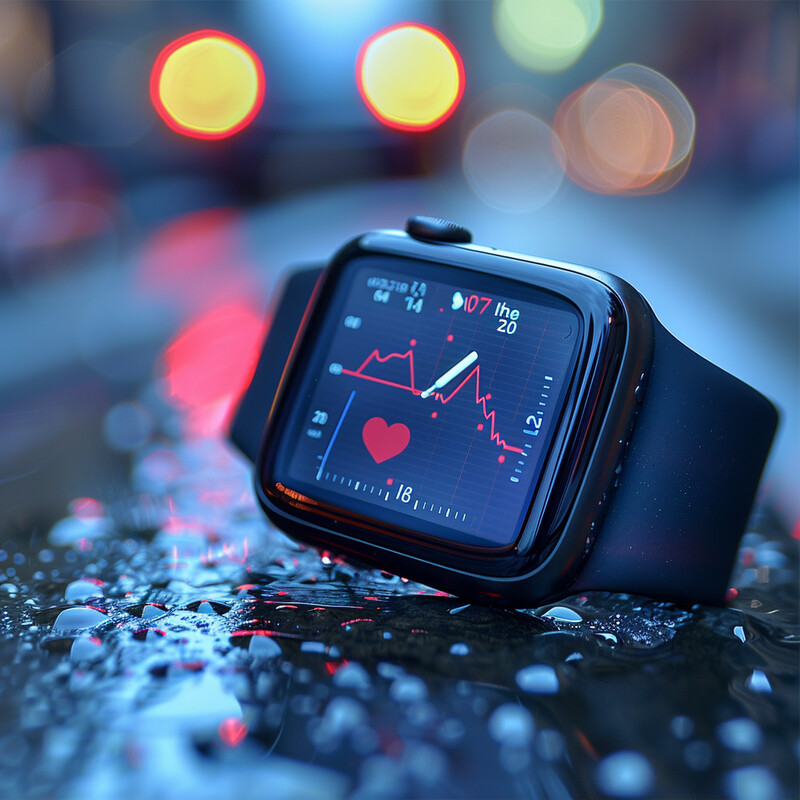
AI-enhanced wearables can detect clinically significant anomalies with high precision. A 2025 meta-analysis showed that photoplethysmography-based smartwatches (using AI to analyze pulse data) achieved a pooled 97.4% sensitivity and 96.6% specificity in identifying atrial fibrillation, a common irregular heart rhythm. This indicates that AI-driven wearable detection of irregular heartbeats is almost as accurate as hospital-grade diagnostics (Luo et al., 2025).
AI is adept at detecting anomalies in health data that may signal underlying health issues. For example, sudden changes in activity levels, sleep patterns, or heart rate that deviate from normal trends can trigger alerts. This allows users to seek timely medical advice, potentially catching diseases or conditions early.
10. Behavioral Modification
AI plays a supportive role in driving long-term behavioral changes for better health via wearables. By continuously analyzing a user’s habits and routines, AI can identify patterns that need improvement – for example, detecting a predominantly sedentary lifestyle, irregular sleep schedule, or suboptimal workout consistency. The wearable, guided by AI, then provides nudges and motivational feedback to encourage healthier behaviors. This can include setting personalized goals (like gradually increasing daily steps), delivering timely reminders (such as stand-up alerts after long periods of inactivity or bedtime reminders), and using gamification or rewards to reinforce good habits. Over time, these small AI-driven interventions accumulate to produce significant behavior change. Importantly, the AI adapts the strategy if it sees something isn’t working: if a user isn’t responding to a certain prompt, it might try a different approach or reward system. By tailoring behavior modification techniques to the individual and keeping them engaged, AI-infused wearables help users sustain healthier diets, exercise routines, stress management practices, and more. This sustained behavior change translates into improved long-term health outcomes and prevention of chronic disease.
AI identifies patterns in behavior that may impact health and suggests modifications to encourage healthier habits, supporting long-term health improvements.
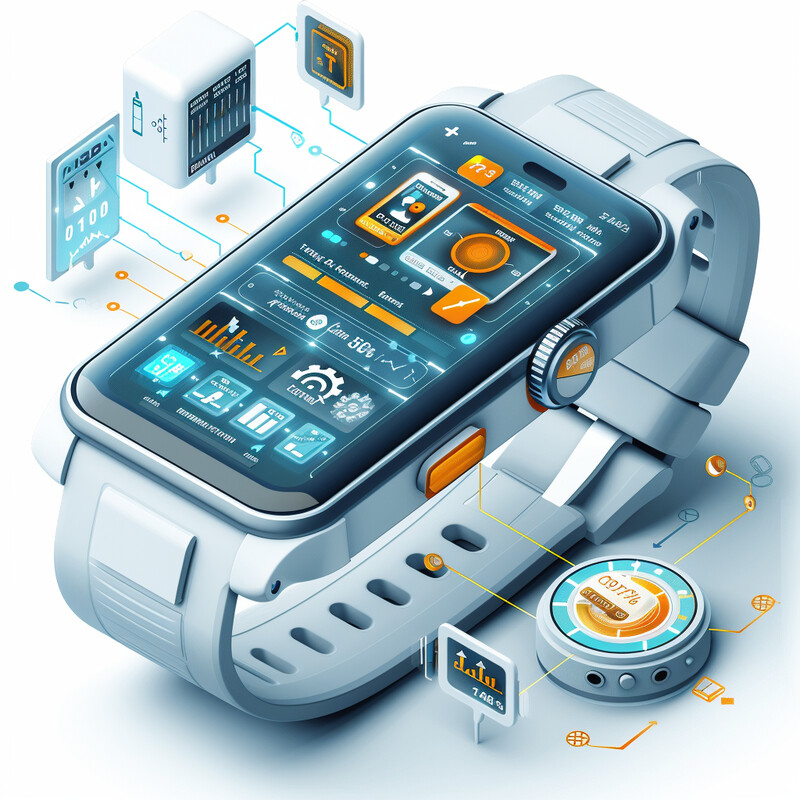
Real-world wellness programs underscore the impact of AI-guided wearables on behavior. For example, participants in an insurer’s wearable reward program (which uses an AI-based system to incentivize activity via a smartwatch) saw an 18% reduction in hospital and chronic disease claims costs over three years compared to non-participants. This significant drop in healthcare utilization reflects meaningful behavior changes – more exercise, better lifestyle choices – driven by sustained engagement with the wearable platform (Discovery Vitality, 2018).
AI identifies and learns patterns in user behavior that could negatively affect health, such as sedentary lifestyles or irregular sleep patterns. It then suggests behavioral modifications and helps users track their progress, supporting sustainable health behavior changes and improving long-term health outcomes.