1. Real-Time Detection
AI enables real-time monitoring and detection of suspicious activities, allowing companies to respond instantly to potential fraud incidents.
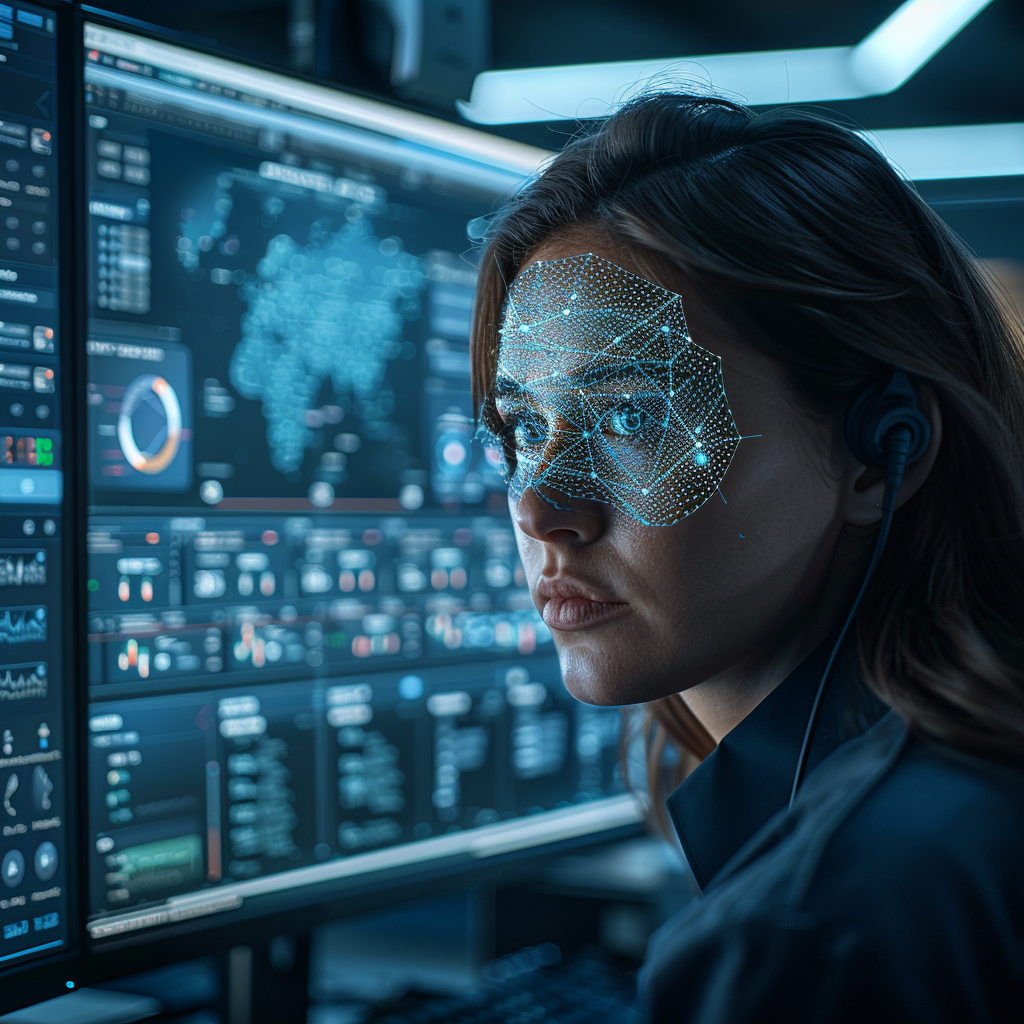
AI significantly boosts the capability of fraud detection systems to monitor transactions and user behaviors in real-time. By analyzing activities as they occur, AI can instantly identify and flag actions that appear suspicious, allowing organizations to intervene promptly before significant damage is done. This immediacy is crucial for industries like banking and e-commerce, where the speed of response can prevent substantial financial losses.
2. Pattern Recognition
AI algorithms excel at identifying and learning from patterns and anomalies in large datasets, which helps in spotting fraudulent behaviors that deviate from the norm.
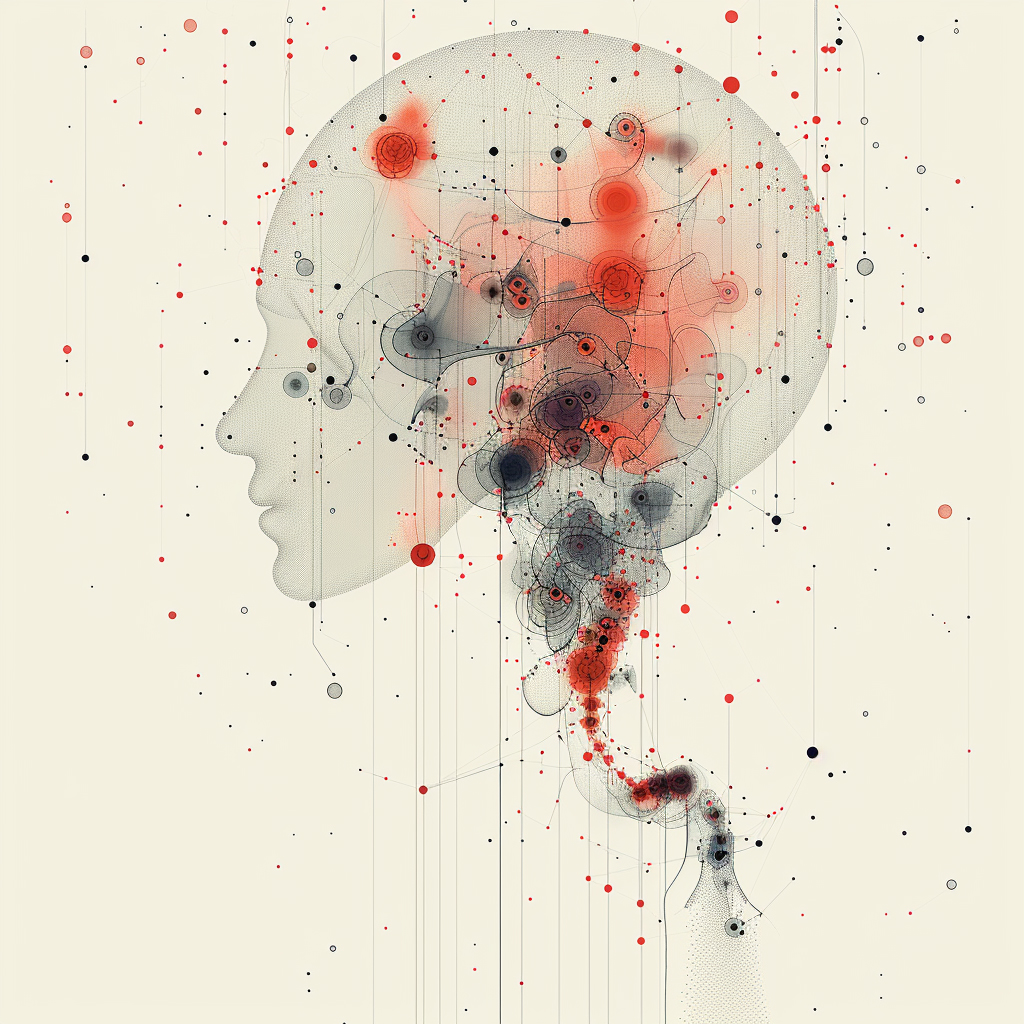
AI algorithms are adept at identifying patterns and anomalies within vast datasets. These systems can recognize deviations from normal transaction behaviors by learning from historical data, enabling them to detect potential fraud. For example, if a user suddenly makes a high-value transaction in an unusual location, AI can flag this as atypical based on learned user patterns.
3. Predictive Analytics
AI uses historical data and predictive analytics to foresee potential fraud scenarios before they occur, enabling proactive measures to prevent them.
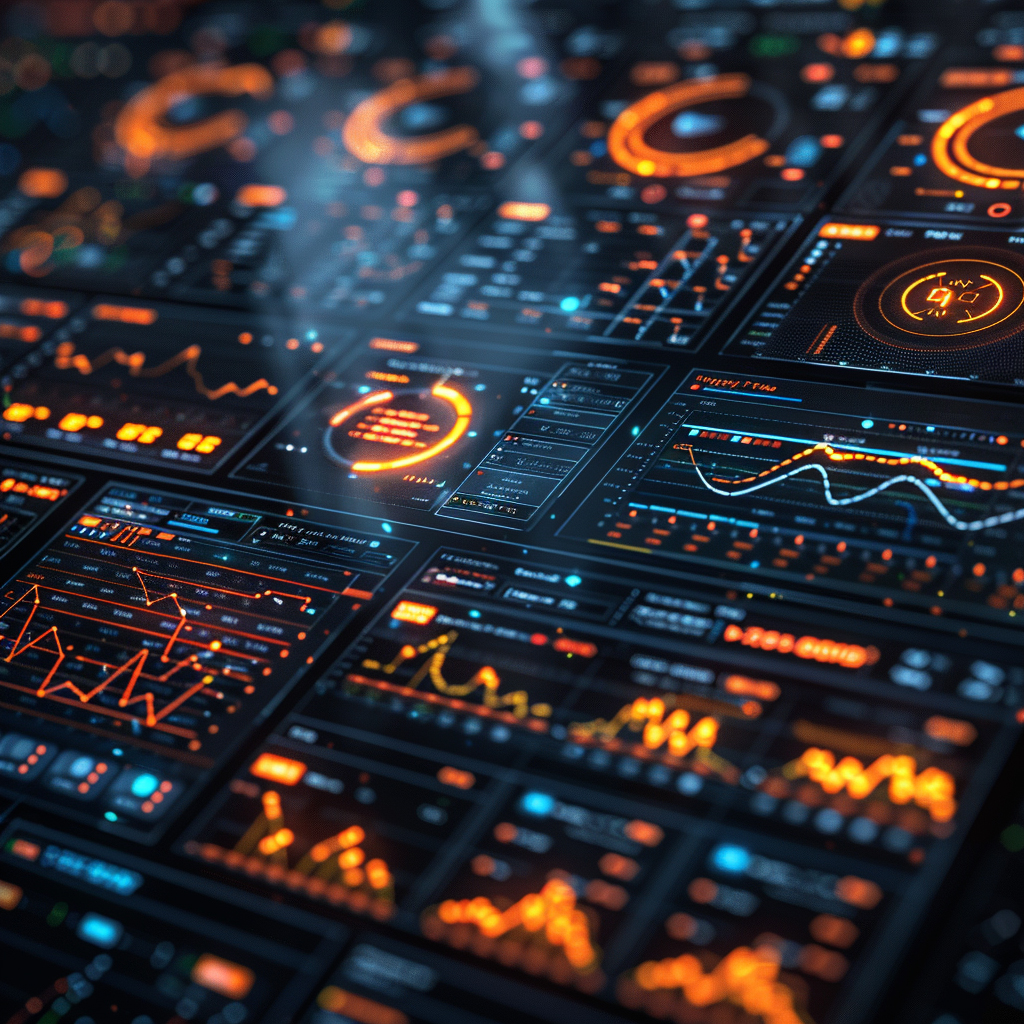
Using predictive analytics, AI anticipates potential fraud by analyzing trends and patterns that have historically been indicative of fraudulent activity. This foresight allows organizations to implement preventative measures even before a fraud attempt is made. AI models can predict the likelihood of fraud in various scenarios, guiding preemptive actions to tighten security or review potentially risky transactions more closely.
4. Adaptive Learning
AI systems continually learn and adapt based on new fraud tactics and techniques, keeping detection methods current and effective against evolving threats.
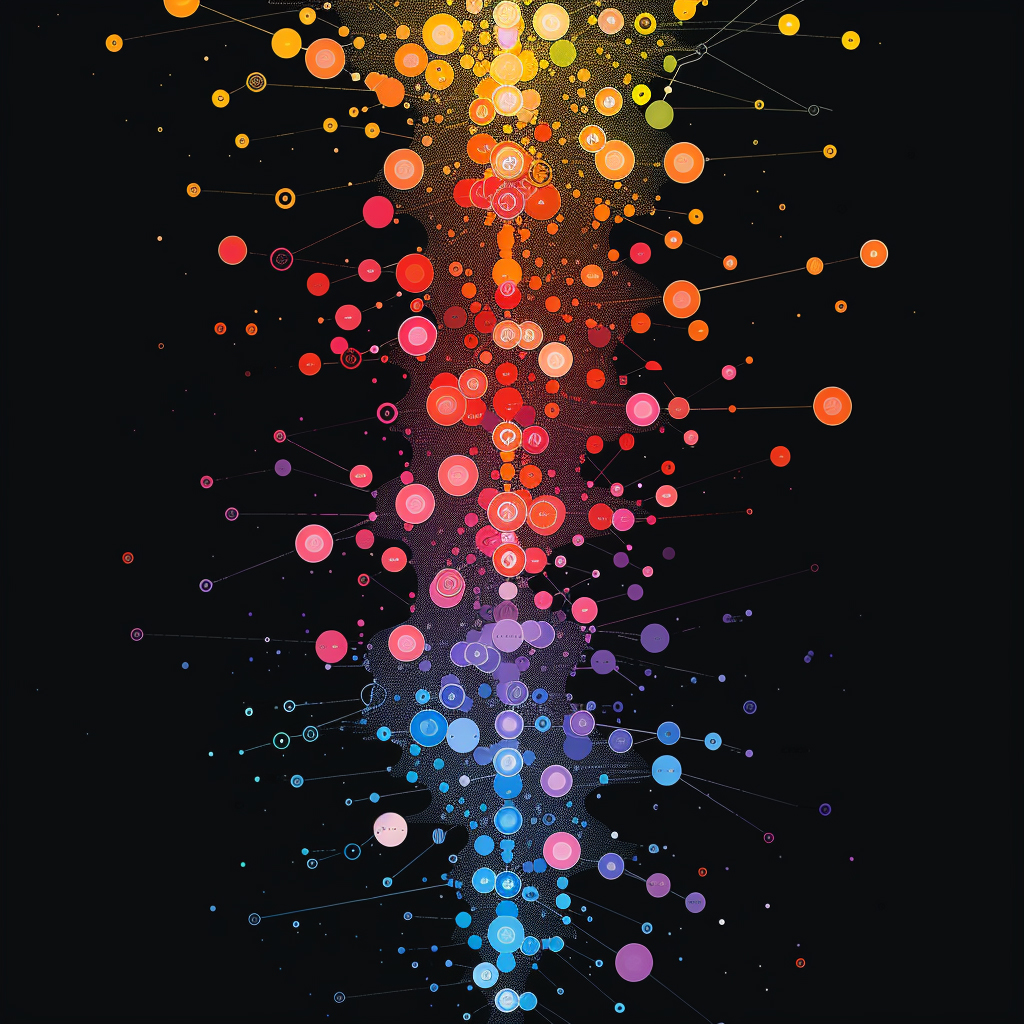
AI systems equipped with machine learning continually evolve by learning from the latest data, including new methods of fraud. This adaptability ensures that fraud detection methods stay effective over time, continually adjusting to counter new tactics employed by fraudsters as they evolve.
5. Enhanced Data Integration
AI can integrate and analyze data across multiple platforms and systems, providing a comprehensive view that is crucial for detecting complex fraud schemes.
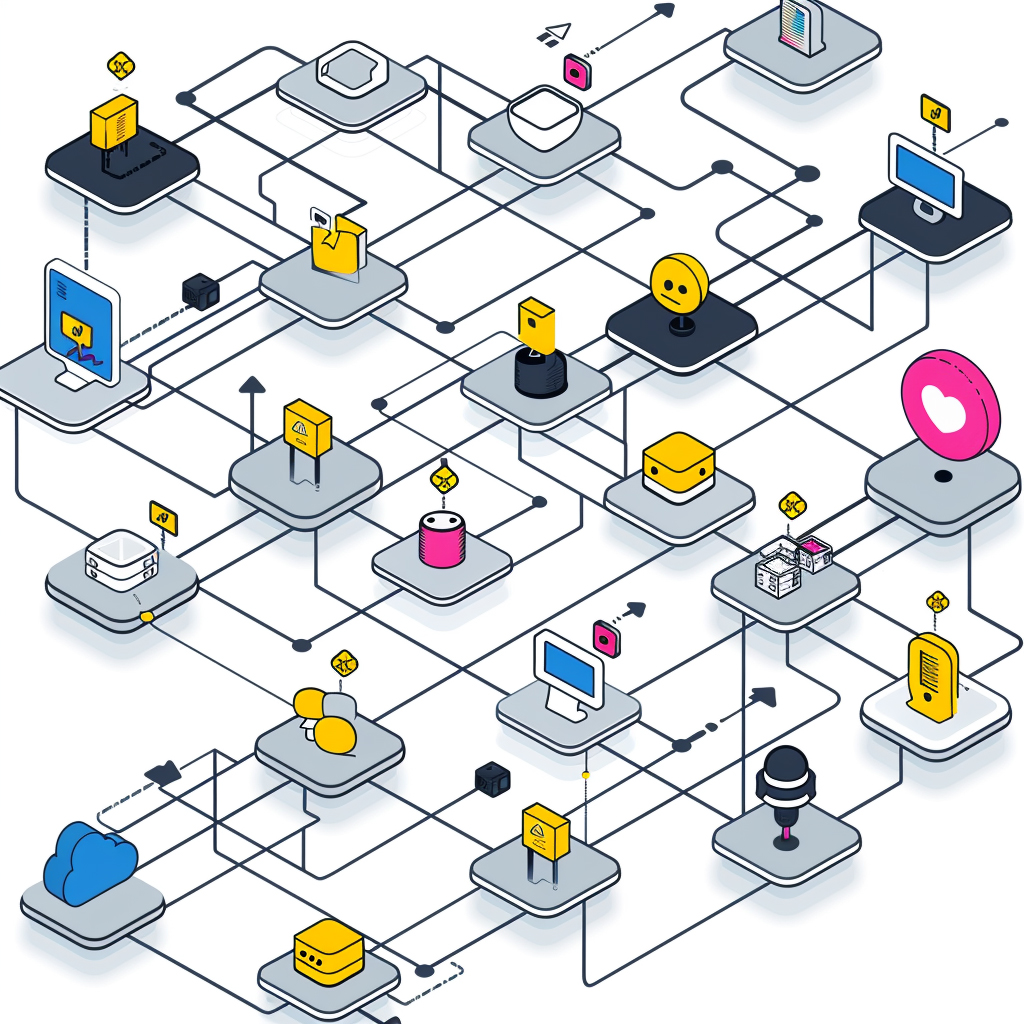
AI can seamlessly integrate and analyze data from multiple sources and systems, creating a unified view that enhances the detection of sophisticated fraud schemes. For instance, by correlating data from customer transactions, social media, and other external data sources, AI can provide a more comprehensive understanding of a user’s profile and identify discrepancies that may indicate fraud.
6. Automated Alerts
AI systems automatically generate alerts for suspicious activities, streamlining the process and ensuring no potential fraud goes unnoticed.
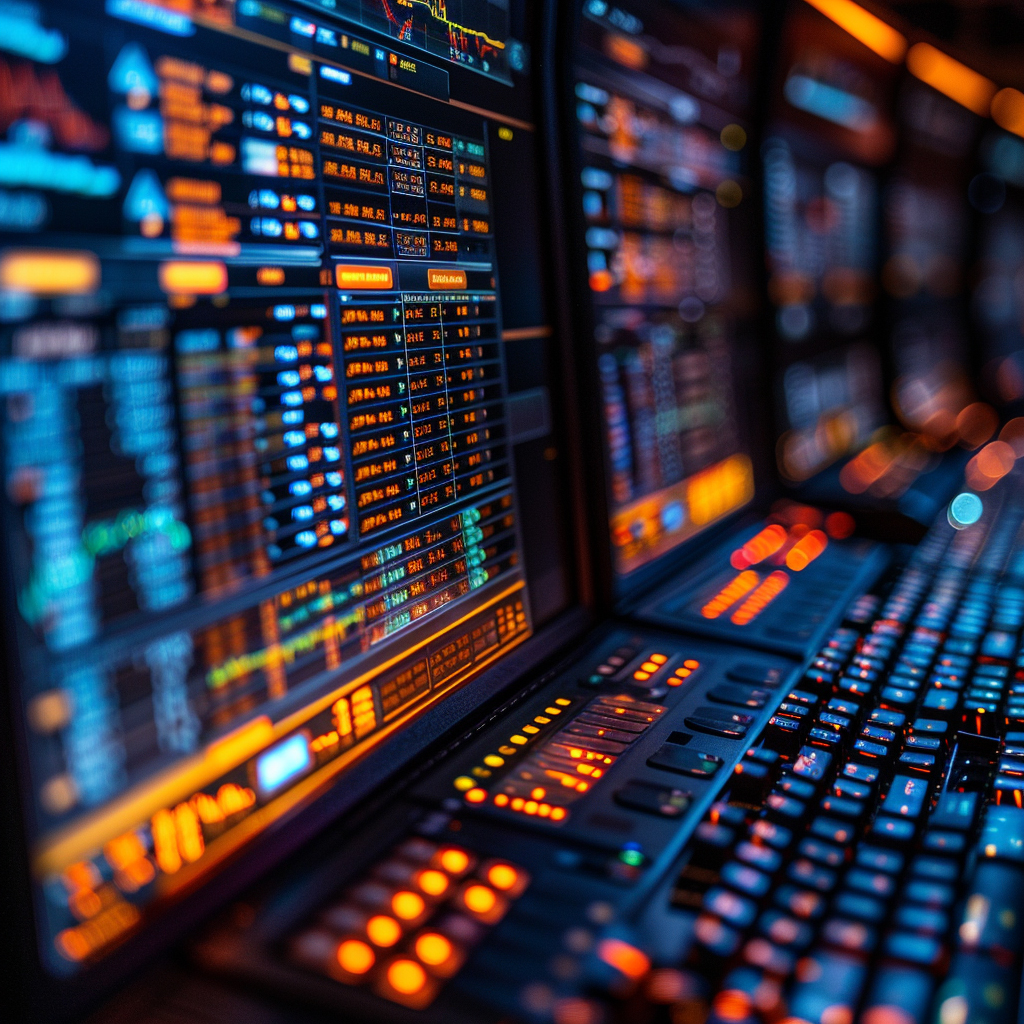
AI-driven systems streamline the fraud detection process by automatically generating alerts when suspicious activities are detected. These automated alerts ensure that human analysts can focus on investigating and responding to legitimate threats more efficiently, rather than sifting through all transactions manually.
7. Risk Assessment
AI helps in assessing the risk levels of transactions or activities based on historical data, improving decision-making processes related to fraud prevention.
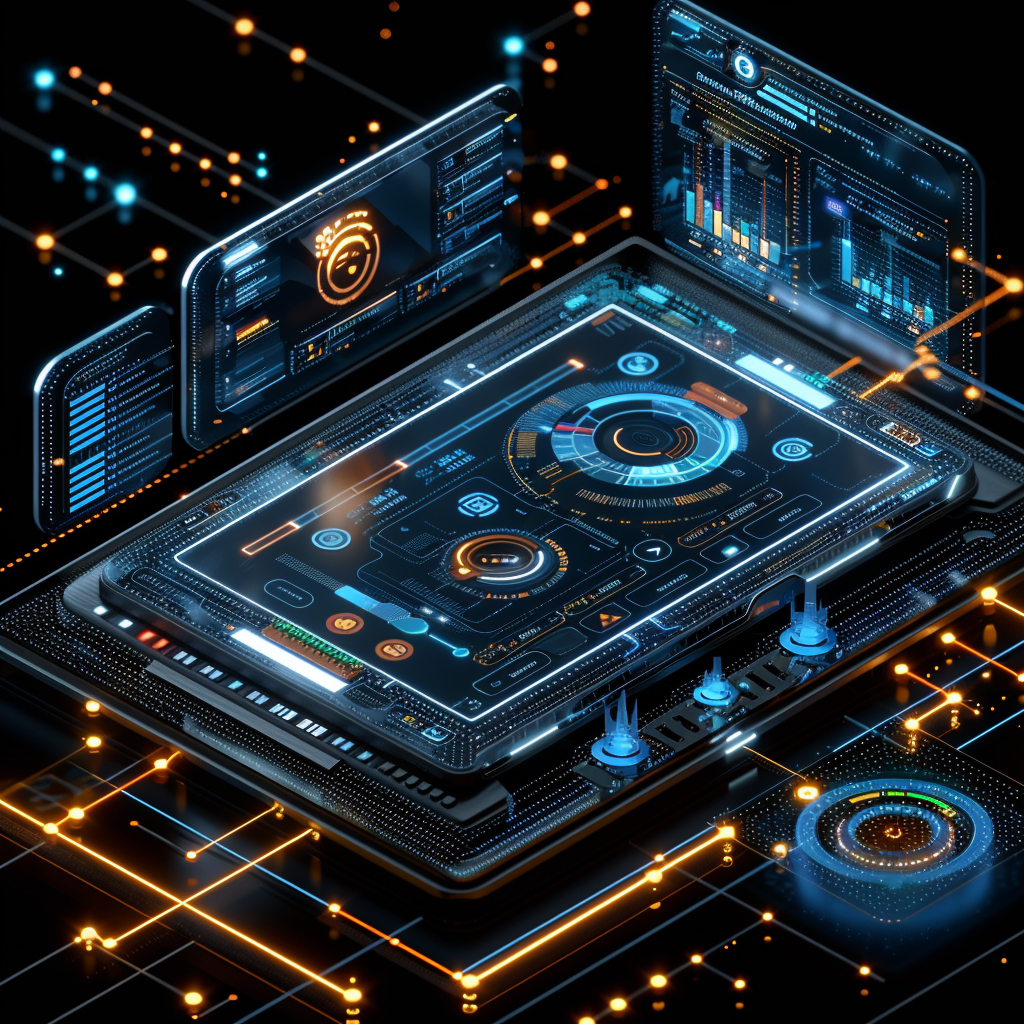
AI enhances the assessment of risk associated with particular transactions or user activities. By analyzing past behaviors and outcomes, AI models can assign risk scores to different actions, helping organizations make informed decisions about which transactions to allow, block, or review further.
8. Text Analysis
AI leverages natural language processing to analyze textual content for signs of fraud in communications, such as phishing emails or fake reviews.
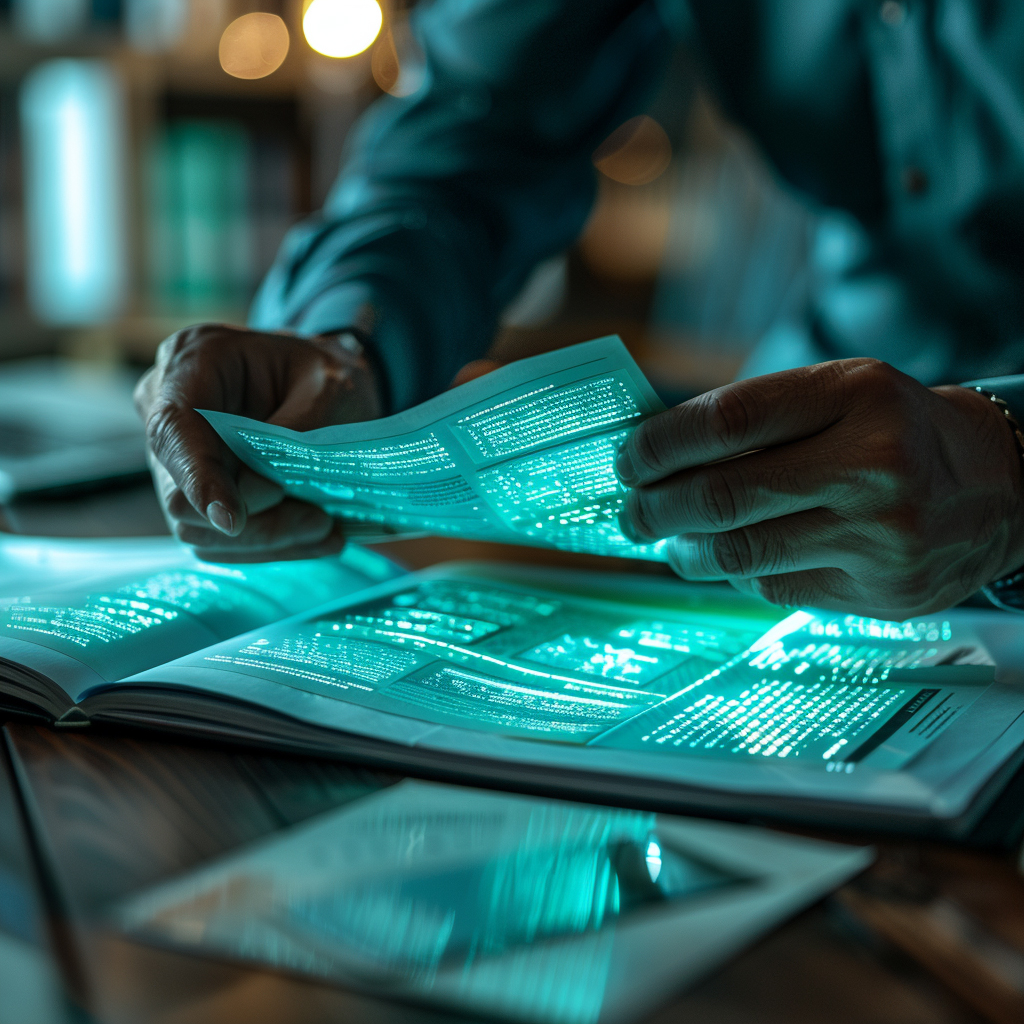
Utilizing natural language processing (NLP), AI analyzes textual content to detect fraud. This includes identifying phishing attempts in emails, fraudulent claims in insurance documents, or deceptive product reviews online. AI’s ability to parse and understand the nuances of language helps in pinpointing text-based fraud efficiently.
9. Image and Video Analysis
Advanced AI technologies are used to detect fraudulent activities through analysis of images and videos, such as identifying altered documents or counterfeit goods.
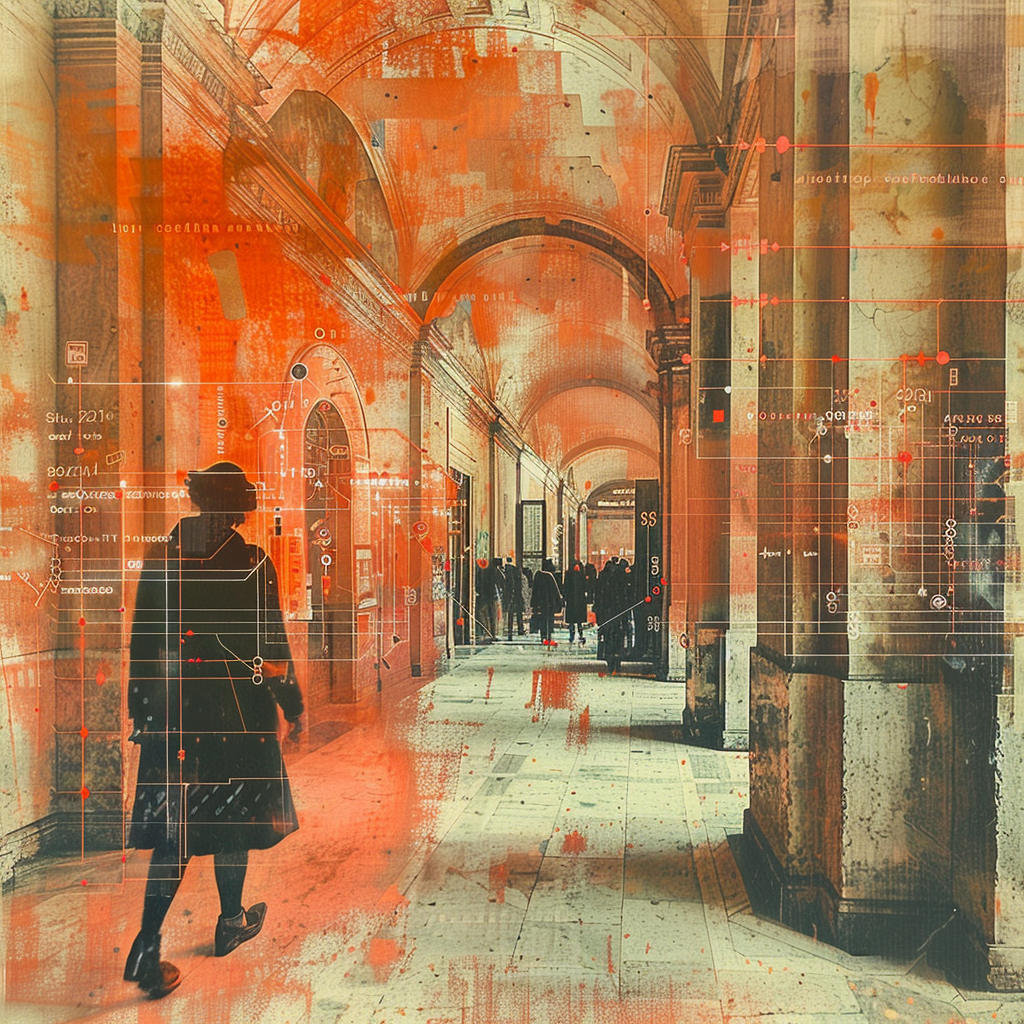
AI technologies also apply to visual content by analyzing images and videos for signs of fraud. This can include detecting alterations in documents, identifying counterfeit products through visual discrepancies, or even analyzing security footage for suspicious behaviors.
10. Network Analysis
AI employs techniques like social network analysis to identify and visualize networks of fraudulent activities, helping in uncovering organized fraud rings.
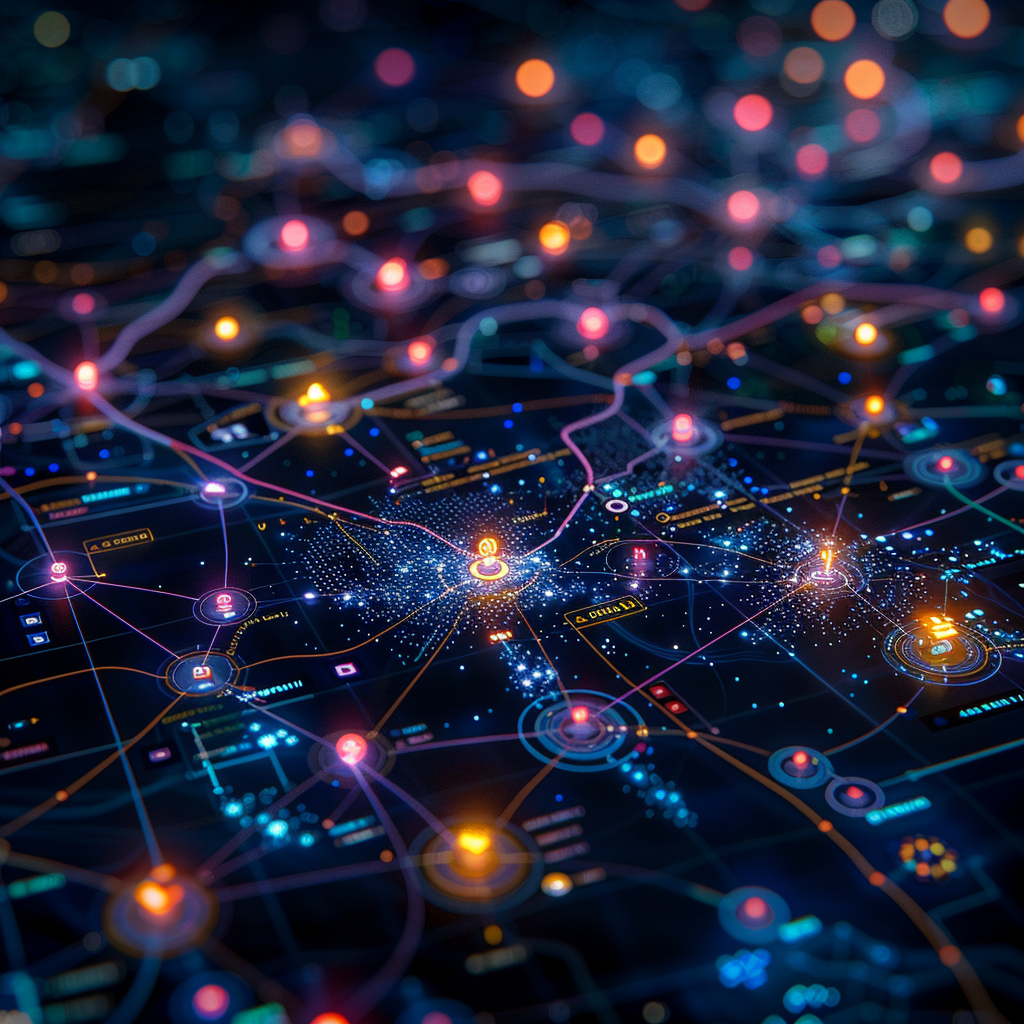
AI employs techniques like social network analysis to examine the connections between entities and transactions. This method is particularly effective in identifying complex fraud schemes involving multiple parties, such as organized crime rings or large-scale financial fraud networks, by visualizing relationships and patterns that might not be evident from isolated data points.